Artificial Intelligence Accurately Detects Fractures on X-Rays
By HospiMedica International staff writers
Posted on 13 Jan 2022
A new study reveals that artificial intelligence (AI) assistance improves the sensitivity and specificity of radiology readers searching for skeletal fractures.Posted on 13 Jan 2022
Researchers at Boston University School of Medicine (BUSM; MA, USA), Stony Brook University (SBU; NY, USA), and other institutions conducted a study of the Gleamer (Paris, France) AI BoneView algorithm, which can detect fractures of the limbs, pelvis, torso, lumbar spine, and rib cage. Six types of readers (radiologists, orthopedic surgeons, emergency physicians, physician assistants, rheumatologists, and family physicians) examined set 480 data sets, both with and without AI BoneView.
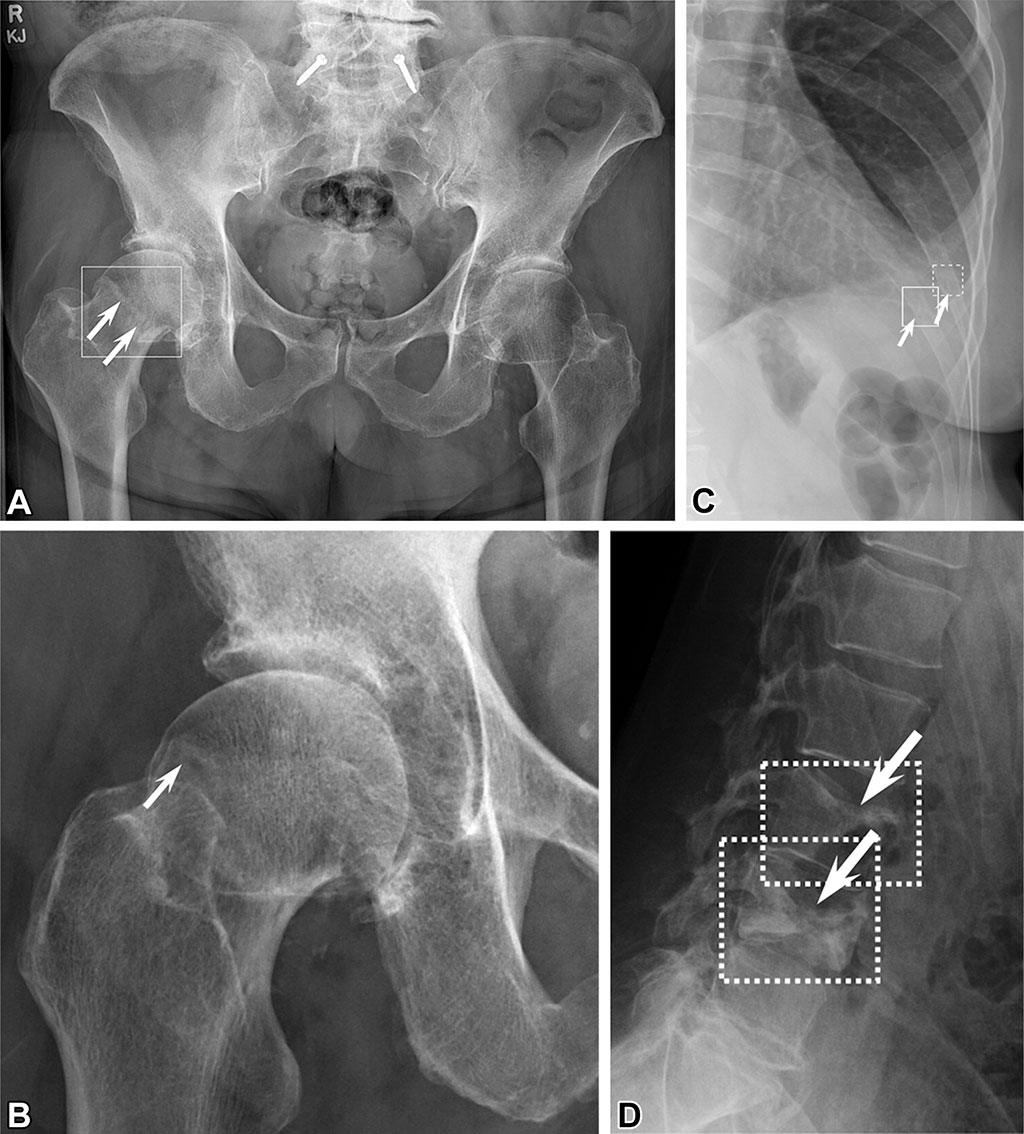
Image: Examples of fractures detected using the AI BoneView algorithm (Photo courtesy of BUSM)
The results revealed that using AI assistance helped reduce missed fractures by 29% and increased readers' sensitivity by 16% for a single fracture, and by 30% for exams with more than one fracture, while improving specificity by 5%. The improvement in sensitivity was significant in all locations, but especially in the shoulder, clavicle, and thoracolumbar spine. AI assistance also shortened X-ray reading time by an average of 6.3 seconds per patient. The study was published on December 21, 2021, in Radiology.
“Our AI algorithm can quickly and automatically detect x-rays that are positive for fractures and flag those studies in the system so that radiologists can prioritize reading x-rays with positive fractures,” said corresponding author Professor Ali Guermazi, MD, PhD, of BUSM. “The system also highlights regions of interest with bounding boxes around areas where fractures are suspected. This can potentially contribute to less waiting time at the hospital or clinic before patients can get a positive diagnosis of fracture.”
Missed fractures on radiographs are one of the most common causes of diagnostic discrepancies between initial interpretations by non-radiologists or residents and the final read by board-certified radiologists, leading to preventable harm or delay in care to the patient. In addition, inconsistencies in radiographic diagnosis of fractures are more common during the evening and overnight hours, likely related to non-expert reading and fatigue. In patients with multiple traumas, the proportion of missed injuries, including fractures, can be high on the forearm and hands (6.6%) and feet (6.5%).
Related Links:
Boston University School of Medicine
Stony Brook University
Gleamer