New Evidence-Based Algorithms Could Improve Diagnosis of Pediatric Tuberculosis
Posted on 16 Mar 2023
Tuberculosis (TB) continues to be one of the most prevalent causes of death among younger populations worldwide. Research indicates that over 96% of the deadly TB cases in children under the age of 15 occur due to the lack of any treatment being provided. However, there is now hope for a reversal in this trend with the development of two novel evidence-based algorithms for diagnosing pediatric TB.
A research team led by scientists at Yale School of Public Health (New Haven, CT, USA) has devised algorithm-based scoring mechanisms that could allow healthcare professionals to make more informed decisions when diagnosing TB, a condition that is notoriously challenging to recognize in low-resource settings. The team at Yale, working alongside a global network of scientists, analyzed medical data related to over 4,000 pediatric TB cases from across the world. Using statistical analyses, the researchers developed the most robust algorithms ever devised for pediatric TB. Following their analysis, the World Health Organization (WHO) has endorsed the implementation of these algorithms as part of its newest comprehensive guidelines for TB management. Additionally, the WHO is encouraging the utilization of the Yale-led team's algorithms.
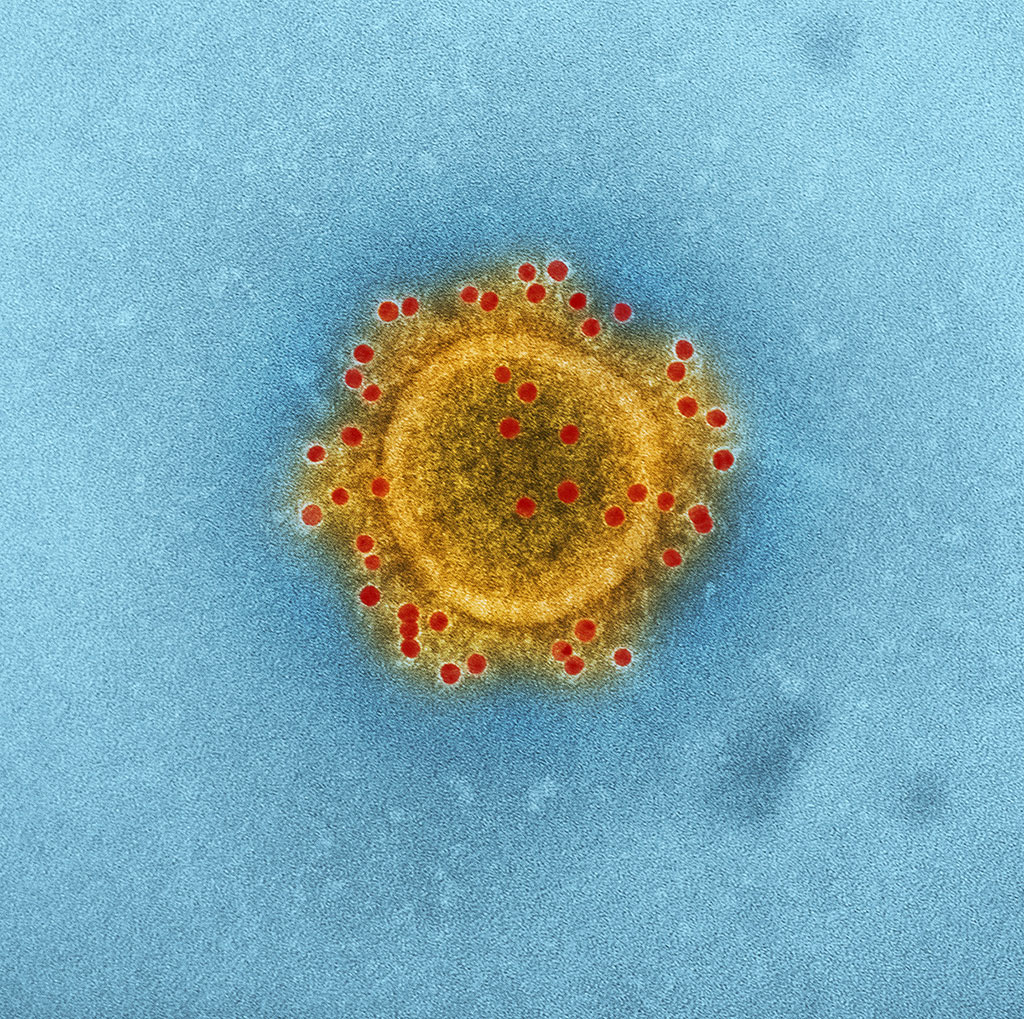
Using algorithms to assist in pediatric TB diagnosis is hardly new. Similar algorithms have been devised to improve disease diagnosis, although they rely mostly on expert opinion, instead of on rigorous interpretation of data. Furthermore, some evidence-based algorithms were developed using limited sample size, thereby limiting their generalizability. In order to create more robust scoring systems, the Yale scientists combined data from 13 studies involving 4,718 children, spanning 12 countries. The team developed two algorithms depending on the healthcare workers' ability to obtain chest X-rays of their patients. With both algorithms, patients are assigned a specific number of points based on their presenting symptoms and other relevant details, which healthcare professionals can add up to receive the recommendation. Generally, correct diagnoses are made approximately 85% of the time if the score is above 10. Patients may also receive scores above 10 if they're known to have been exposed to TB, regardless of the presence of other symptoms.
The researchers anticipate that their algorithms will facilitate more timely treatment for pediatric TB patients, but have warned about some limitations to their scoring systems, particularly due to their inability to formally test the algorithms. However, the team will conduct future studies to assess the capability of their algorithms to diagnose TB in real-world settings. Combining data from multiple studies can improve statistical analyses and create more robust data sets, although varying inclusion criteria and study definitions might result in slightly skewed data. Additionally, given the difficulty in diagnosing TB, some conclusions might be incorrect, according to the researchers. Further studies on pediatric TB, additional data and better diagnostic tools could help significantly refine the new algorithms. Nonetheless, the WHO's conditional recommendation of these algorithms as an aid to pediatric TB diagnosis, and its encouragement of the use of the Yale-led research group's algorithm paves the way for future research.
“I’m really looking forward to seeing the results of the prospective evaluation of these algorithms when others are actually going to use them in a study setting to aid in treatment decision-making for children being investigated for TB,” said Kenneth Gunasekera, a Yale M.D.-Ph.D. student who was first author on the study. “We built the algorithms based on evidence, but it will be exciting to see how well they will actually perform.”
Related Links:
Yale School of Public Health