Machine Learning Detects Cardiovascular Diseases Before Symptoms Appear
Posted on 14 Aug 2024
Cardiovascular diseases rank among the leading causes of mortality globally, often remaining undetected until symptoms manifest and the condition becomes advanced, necessitating surgical intervention over medication. Researchers have devised a method to enhance the early detection of these diseases, bypassing expensive diagnostics like MRI or CT, through the use of a digital twin of the patient, which also allows for more in-depth disease investigation. This innovation promises to ease the strain on patients, doctors, and medical facilities alike.
Developed by the team at Graz University of Technology (TU Graz, Styria, Austria), this new approach leverages the principle that any disease altering cardiovascular mechanics also modifies the externally applied electrical field in specific ways, affecting conditions such as arteriosclerosis, aortic dissection, aneurysms, and heart valve defects. Researchers can utilize standard electrical, bio-impedance, or optical signals—from ECGs, PPGs, or smartwatches—which are analyzed through a self-developed machine learning model. This model detects potential diseases from the signals and assesses the likelihood of their presence, enabling earlier intervention when medication might still be viable over surgery.
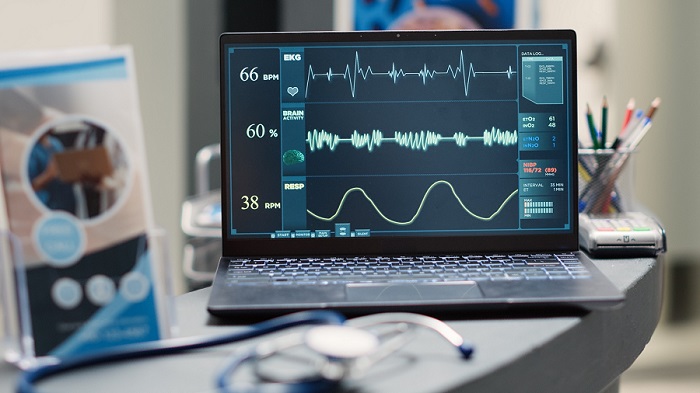
The machine learning model's training incorporated real clinical bio-impedance data and simulation values from cardiovascular system models. With numerous cardiovascular parameters and extensive simulation needs for statistically significant results, machine learning enables the achievement of results with more than 90% accuracy swiftly. Another benefit of this machine learning analysis is its capacity to identify changes in ECG data that are not easily visible to even seasoned physicians.
For instance, this technology can assess the extent of arterial stiffening, often a precursor to aortic dissection, thus serving as an early warning sign. Once a significant change is detected, the diagnostic data can be used to construct a multi-physical simulation model or a digital twin, which not only predicts the disease's progression but also facilitates deeper analysis by medical professionals. The researchers are actively refining this technology in collaboration with healthcare industry partners to enhance the accuracy of their algorithms and further tailor them for clinical application.
“There is a lot of information that can be collected from outside the body with little effort,” said Vahid Badeli from the Institute of Fundamentals and Theory in Electrical Engineering at TU Graz. “So far, it has been difficult to find out exactly what this information means. But with our computer models and the help of machine learning, we can understand it better and find correlations.”
Related Links:
TU Graz