Wearable Technology Combined with AI Algorithms Offers More Accurate Way to Diagnose Parkinson’s
Posted on 24 Sep 2024
Parkinson's disease typically begins with mild symptoms, like a slight tremor in one hand, but can progress to more severe conditions such as muscle stiffness and difficulty walking without assistance. Diagnosing this debilitating movement disorder, particularly in its early stages, usually requires patients to perform various mobility tasks, with clinicians observing walking patterns and testing reflexes. This process is time-consuming and labor-intensive for both doctors and patients. Experts believe many individuals remain undiagnosed or are misdiagnosed, indicating that more people may have the disease than current estimates suggest. This highlights one of the primary challenges faced by clinicians and patients: the difficulty in accurately diagnosing Parkinson's disease. A more accurate diagnostic method could alleviate the physical and emotional strain patients experience by reducing the need for multiple clinic visits and preventing misdiagnoses.
Researchers at the University of Maryland, College Park’s Center for Bioinformatics and Computational Biology (CBCB, College Park, MD, USA) are collaborating with colleagues to address this issue using machine learning algorithms to analyze data from wearable movement-tracking sensors, aiming to automate parts of the diagnostic process. According to the researchers, this approach could lead to earlier and more accurate diagnoses, enabling timely therapeutic interventions. While wearable sensors for diagnosing Parkinson’s disease have been developed in the past, their complexity has hindered widespread clinical adoption. This new research simplifies the use of sensors and machine learning in clinical settings. The study demonstrated that a single sensor placed on the lower back, combined with a single mobility task involving multiple movements, could effectively differentiate individuals with Parkinson’s disease from healthy controls.
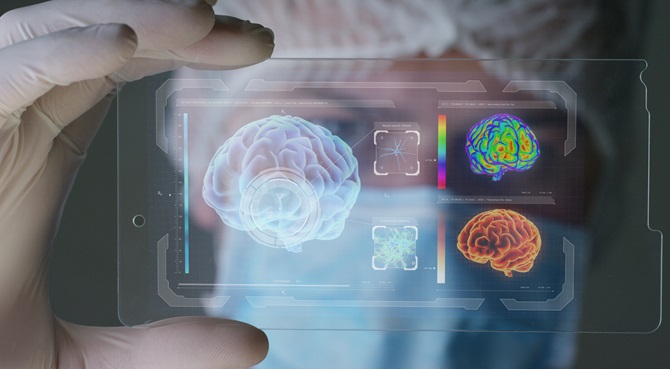
The research team then created an advanced machine-learning framework to analyze patterns and variations in the collected data. As detailed in the journal Sensors, this approach significantly improved the detection of disease symptoms and diagnostic accuracy, achieving an accuracy rate of 92.6% in identifying participants across various stages of Parkinson’s disease—surpassing the 81% accuracy previously reported for clinical diagnoses by movement disorder experts. The team is now expanding its research to distinguish Parkinson’s from other movement disorders, aiming to further increase diagnostic accuracy and reduce the likelihood of misdiagnosis.
Related Links:
CBCB