Novel Algorithms Predict Cardiovascular Outcomes at Point-Of-Care Using ECG Data
Posted on 28 Nov 2024
A significant number of strokes with an unknown cause are linked to underlying, subclinical, paroxysmal atrial fibrillation (AFib), a condition where the heart beats irregularly for brief periods without causing noticeable symptoms. These AFib-related strokes can be prevented with blood-thinning medications. The standard approach for detecting AFib is the implantation of a loop recorder, a device placed under the skin to monitor heart activity and detect AFib. This allows healthcare providers to decide whether patients should use blood thinners to prevent future strokes. Now, researchers are leveraging machine learning and existing electrocardiogram (ECG) data to assist doctors in making more accurate predictions.
Researchers at Penn State (University Park, PA, USA) are developing new algorithms capable of predicting cardiovascular outcomes at the point-of-care. In an initial pilot study published in Heart Rhythm, they presented a model that could predict whether a patient with an unexplained stroke would develop AFib by analyzing a single heartbeat from a common and inexpensive heart test: the standard 12-lead ECG, which records the electrical activity of the heart. The research team sought to explore whether they could predict AFib using a 12-lead ECG rather than relying on a loop recorder.
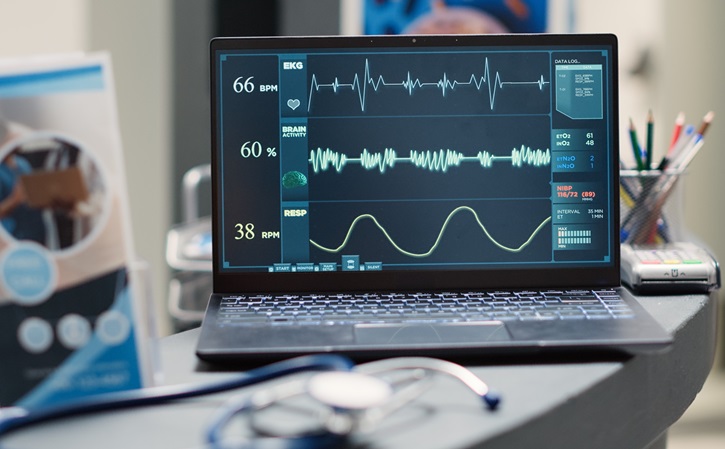
The team used a small dataset of existing ECG data from Penn State, which included patients with cryptogenic stroke—stroke without a known cause—who had previously had loop recorders implanted, as well as data from standard 12-lead ECGs. Using machine learning algorithms, they created a model that could analyze a patient’s 12-lead ECG and predict the likelihood of developing AFib. The model correctly predicted the outcome for 80% of the patients in the test cohort. Moving forward, the team plans to expand their database to enhance the model's applicability. The researchers achieved these high levels of accuracy by utilizing data augmentation techniques, which helped improve the predictive performance.
“With stronger algorithms and a larger database, we can predict cardiovascular outcomes at significantly less cost,” said Ankit Maheshwari, assistant professor of medicine at Penn State and lead researcher on the project. “This pilot study shows that even with a smaller group of 200 to 300 patients, we could create a useful predictive model. Our goal is to organize the 1.8 million ECGs in the University’s medical record system into a searchable database to facilitate large volume ECG analysis to support future projects aimed at utilizing a 12-lead ECG to predict cardiovascular outcomes and improve patient care.”