Deep-Learning Model that Can Predict How Human Genes and Medicines Will Interact Identifies Promising COVID-19 Treatments
By HospiMedica International staff writers
Posted on 03 Feb 2021
A new deep-learning model that can predict how human genes and medicines will interact has identified at least 10 compounds that may hold promise as treatments for COVID-19.Posted on 03 Feb 2021
The computer model, which the researchers call “DeepCE” - pronounced “Deep Sea” was created by computer scientists at The Ohio State University (Columbus, OH, USA) and helps find drug repurposing candidates for new diseases. All but two of the drugs identified as potential COVID-19 treatments by the deep-learning model are still considered investigational and are being tested for effectiveness against hepatitis C, fungal disease, cancer and heart disease. The list also includes the approved drugs cyclosporine, an immunosuppressant that prevents transplant organ rejection, and anidulafungin, an antifungal agent.
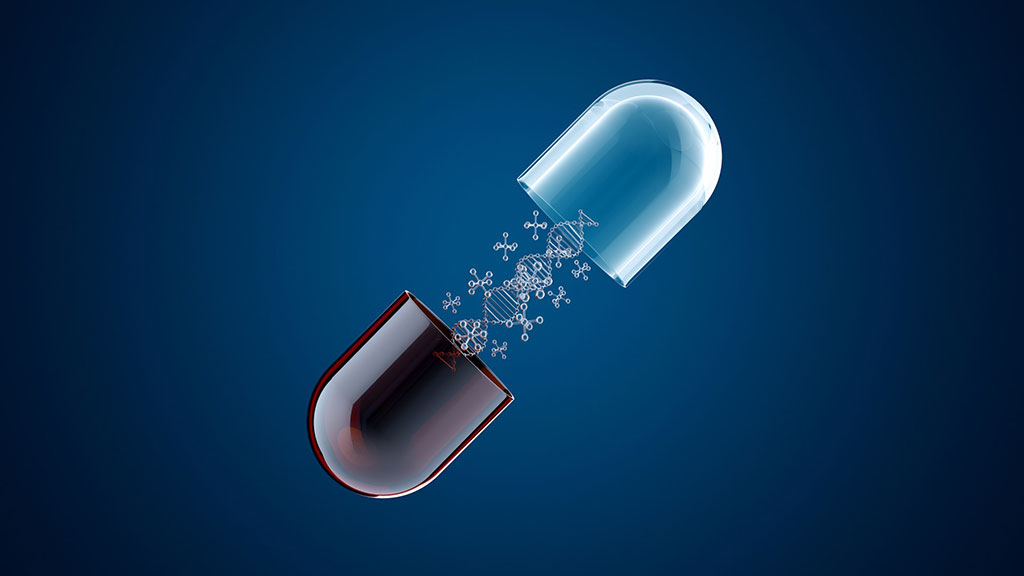
Image: The computer model can predict how human genes and medicines will interact (Photo courtesy of Getty Images)
Much more work needs to be done before any of these medications would be confirmed as safe and effective treatments for people infected with SARS-CoV-2. But by using artificial intelligence to arrive at these options, the scientists have saved pharmaceutical and clinical researchers the time and money it would take to search for potential COVID-19 drugs on a piecemeal basis. The researchers have noted that a few of the repurposing candidates the model generated have already been studied for their potential use in COVID-19 patients.
To make predictions about how genes and medicines will interact and yield drug repurposing candidates, DeepCE relies on two primary sources of publicly available data: L1000, a National Institutes of Health-funded repository of human cell-line data showing how gene expression changes in response to drugs, and DrugBank, which contains information on the chemical structures and other details on about 11,000 approved and investigational drugs.
L1000 displays side-by-side cell-line comparisons of standard gene expression activity with gene expression changes produced by interactions with specific drugs. The cell lines represent diseases, such as melanoma, and organs, like kidneys and lungs. It is an ongoing project, with data being added as experiments in animals or humans supplement the gene expression profiles produced in cell-line experiments.
The Ohio State researchers trained the DeepCE model by running all of the L1000 data through an algorithm against specific chemical compounds and their dosages. To fill in data gaps, the model converts chemical compound descriptions into figures, allowing for automatic consideration of their separate components’ effects on genes. And for genes not represented in L1000, the team used a deep learning approach called an “attention mechanism” to increase the model’s “learned” sample of gene-chemical compound interactions, which improves the framework’s performance.
The team applied DeepCE’s gene expression prediction matrix - focusing on data from lung and airway cell lines and the entire DrugBank catalog of compounds - to the genetic information provided from the early COVID-19 papers and additional government data. The COVID-19 data demonstrated how human gene expression had responded to being infected with SARS-CoV-2, creating a “disease signature.”
“When no one has any information on a new disease, this model shows how artificial intelligence can help solve the problem of how to consider a potential treatment,” said senior author Ping Zhang, assistant professor of computer science and engineering and biomedical informatics at The Ohio State University. “Great minds think alike - some lead compounds identified by machine intelligence coincide with later discoveries by human intelligence.”
Related Links:
The Ohio State University