Bedside AI Sepsis Detection System Can Cut Hospital Deaths by 20%
Posted on 22 Jul 2022
Sepsis occurs when an infection triggers a chain reaction throughout the body. Inflammation can lead to blood clots and leaking blood vessels, and ultimately can cause organ damage or organ failure. About 1.7 million adults develop sepsis every year in the US and more than 250,000 of them die. Sepsis is easy to miss because symptoms such as fever and confusion are common in other conditions. The faster it's caught, the better a patient's chances for survival. Now, patients are 20% less likely to die of sepsis because a new AI system catches the symptoms hours earlier than traditional methods
The AI system developed by researchers at Johns Hopkins University (Baltimore, MD, USA) scours medical records and clinical notes to identify patients at risk of life-threatening complications. The work could significantly cut patient mortality from one of the top causes of hospital deaths worldwide. The machine-learning system, named Targeted Real-Time Early Warning System, combines a patient's medical history with current symptoms and lab results to show clinicians when someone is at risk for sepsis and suggests treatment protocols, such as starting antibiotics. The AI tracks patients from when they arrive in the hospital through discharge, ensuring that critical information isn't overlooked even if staff changes or a patient moves to a different department.
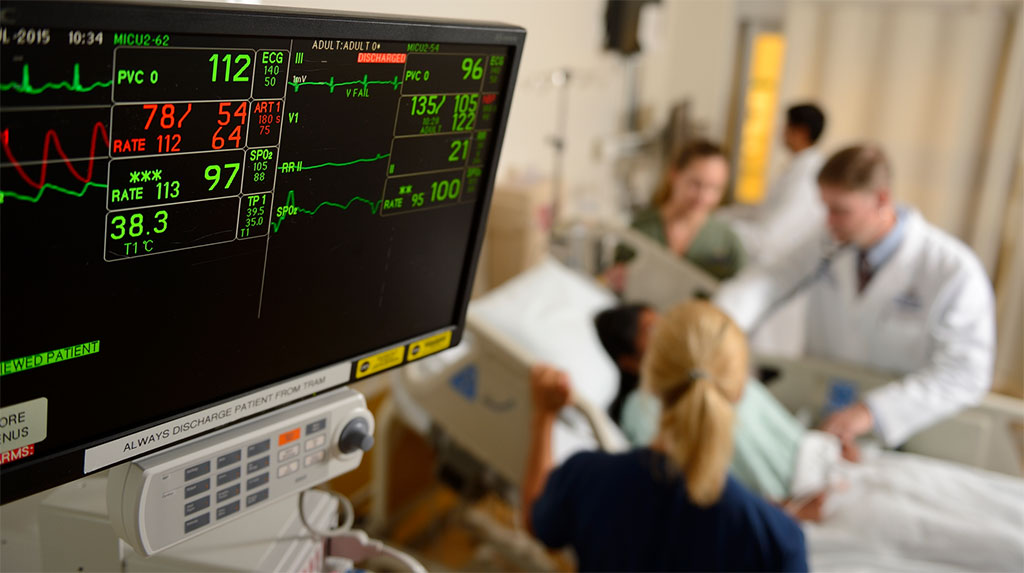
In an extensive hospital study, more than 4,000 clinicians from five hospitals used the AI in treating 590,000 patients. The system also reviewed 173,931 previous patient cases. In 82% of sepsis cases, the AI was accurate nearly 40% of the time. Previous attempts to use electronic tools to detect sepsis caught less than half that many cases and were accurate 2% to 5% of the time. All sepsis cases are eventually caught, but with the current standard of care, the condition kills 30% of the people who develop it. In the most severe sepsis cases, where an hour delay is the difference between life and death, the AI detected it an average of nearly six hours earlier than traditional methods. The team has now adapted the technology to identify patients at risk for pressure injuries, commonly known as bed sores, and those at risk for sudden deterioration caused by bleeding, acute respiratory failure, and cardiac arrest.
"It is the first instance where AI is implemented at the bedside, used by thousands of providers, and where we're seeing lives saved," said Suchi Saria, founding research director of the Malone Center for Engineering in Healthcare at Johns Hopkins and lead author of the studies, which evaluated more than a half million patients over two years. "This is an extraordinary leap that will save thousands of sepsis patients annually. And the approach is now being applied to improve outcomes in other important problem areas beyond sepsis."
Related Links:
Johns Hopkins University