AI Technology for Automated Assessment of Coronary Angiograms to Reduce Invasive Testing
Posted on 17 May 2023
Coronary heart disease is the primary cause of death in adults globally. Coronary angiography is a standard diagnostic procedure that influences virtually all relevant clinical choices, from medication prescriptions to coronary bypass surgery. In many instances, quantifying the left ventricular ejection fraction (LVEF) at the time of coronary angiography is essential for enhancing clinical decisions and treatment plans, especially when the angiography is carried out due to potentially fatal acute coronary syndromes (ACS). As the left ventricle is the main pumping part of the heart, assessing the ejection fraction in this chamber offers crucial details about the percentage of blood leaving the heart with each contraction. Currently, an extra-invasive procedure, known as left ventriculography, is required to measure LVEF during angiography, which involves inserting a catheter into the left ventricle and injecting a contrast dye. This procedure carries additional risks and increases contrast exposure. Now, researchers have developed automated assessment of coronary angiograms to reduce risk and minimize the need for invasive testing.
In a new study, researchers at University of California San Francisco (San Francisco, CA, USA) and the Montreal Heart Institute (Montreal, Canada) aimed to examine whether deep neural networks (DNNs), a type of AI algorithm, could predict cardiac pump function from standard angiogram videos. They created and tested a DNN named CathEF to estimate LVEF from coronary angiograms of the heart's left side. The team conducted a cross-sectional study of 4042 adult angiograms matched with corresponding transthoracic echocardiograms (TTEs) from 3679 UCSF patients. They trained a video-based neural network to estimate reduced LVEF (equal to or less than 40%) and to predict the LVEF percentage from standard angiogram videos of the left coronary artery.
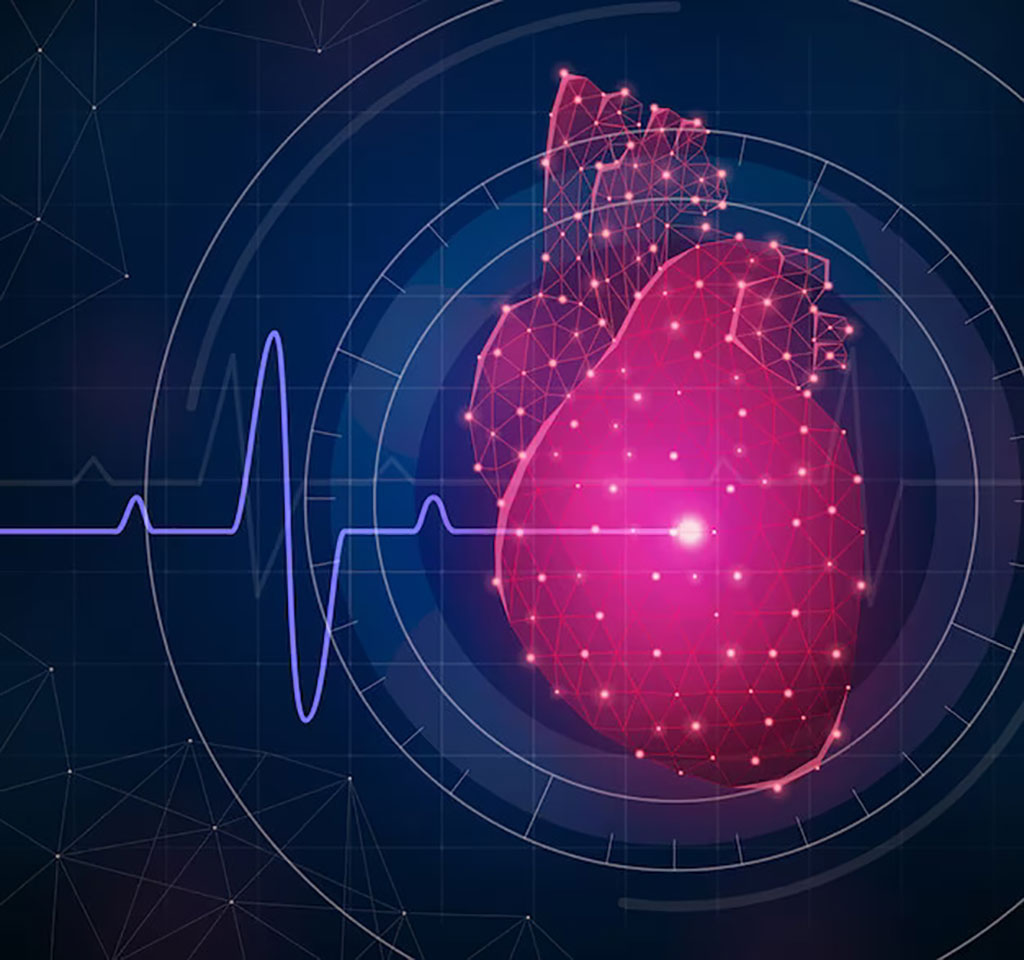
The findings indicated that CathEF accurately predicted LVEF, displaying strong correlations with echocardiographic LVEF measurements, which is the typical noninvasive clinical method. The model was also externally validated in real-world angiograms. It performed well across diverse patient demographics and clinical conditions, including acute coronary syndromes and varying degrees of renal function - groups of patients who may be less suitable for the standard left ventriculogram procedure. The researchers are now conducting further research to test this algorithm at the point of care and assess its influence on the clinical workflow in patients experiencing heart attacks. To that end, they have initiated a multi-center prospective validation study in patients with ACS to compare the performance of CathEF and the left ventriculogram with TTEs performed within 7 days of ACS.
“This work demonstrates that AI technology has the potential to reduce the need for invasive testing and improve the diagnostic capabilities of cardiologists, ultimately improving patient outcomes and quality of life,” said senior author and UCSF cardiologist Geoff Tison, MD, MPH.
Related Links:
UC San Francisco
Montreal Heart Institute