Machine Learning Delivers Personalized Oxygenation for Patients on Ventilators
Posted on 10 Jun 2024
Supplemental oxygen is one of the most commonly prescribed treatments globally, with between 13 and 20 million patients each year needing oxygen supplied via mechanical ventilation. Mechanical ventilation is a critical life support technology that facilitates the movement of air into and out of the lungs, functioning similarly to a bellows. Modern ventilators are a significant advancement from the typically envisioned old “iron lung” machines; today's devices are sophisticated, compact digital machines that administer oxygen through a small plastic tube inserted into the throat. Despite these technological improvements, determining the appropriate oxygen level for each patient continues to be largely based on estimation. Clinicians set oxygen levels using devices that measure SpO2 saturation, indicating the oxygen saturation in a patient's blood, yet previous studies have not conclusively determined whether higher or lower SpO2 targets are more beneficial for patients.
To remove the guesswork out of ventilation, a team from The University of Chicago Medicine (Chicago, IL, USA) has employed a machine learning model to explore how different oxygen levels might affect outcomes based on individual patient characteristics. Their findings suggest that personalized oxygenation targets could significantly lower mortality rates, potentially revolutionizing critical care practices. Earlier studies by various research groups attempted to determine if higher or lower oxygen levels were more advantageous, but generally, these studies did not yield definitive results. The researchers at UChicago Medicine proposed that the neutral outcomes of these trials might not mean oxygen levels are irrelevant to patient outcomes but rather that the effects of different oxygen levels vary from patient to patient, averaging out to zero effect in randomized trials.
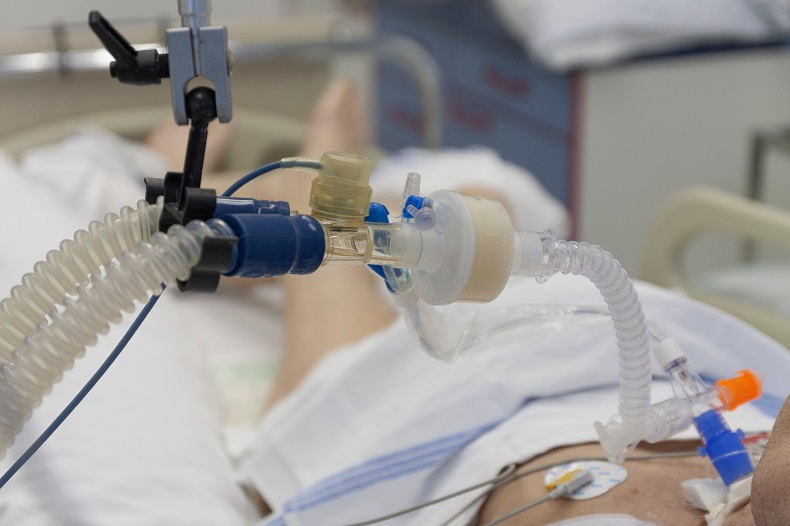
As personalized medicine gains popularity, there is an increasing interest in leveraging machine learning to predict optimal treatments for individual patients. In the area of mechanical ventilation, these predictive models could potentially determine the ideal oxygen saturation for a patient based on specific characteristics such as age, gender, heart rate, body temperature, and the reason for ICU admission. The team and their colleagues utilized data from previous randomized trials to develop and refine their machine learning model. After initial development with data from the U.S., the model was applied to patient data from Australia and New Zealand. According to their findings, for patients who achieved the oxygenation levels deemed optimal by the model, there was an overall mortality reduction of 6.4%. It’s important to note that outcomes cannot be universally predicted based on a single characteristic — for instance, not all patients with brain injuries will benefit from lower oxygen levels, despite data trends suggesting this — creating the need for a comprehensive tool like the machine learning model that integrates diverse patient data.
Despite the complexity of the algorithm, the input variables are common clinical parameters, making it simple for healthcare teams to use such tools in the future. At UChicago Medicine, algorithms are already integrated directly into electronic health record (EHR) systems to support various clinical decisions. The researchers envision that mechanical ventilation could be managed in a similar manner. For hospitals lacking the resources to integrate such advanced machine learning tools into EHRs, there is also the possibility of developing a web-based application that would allow clinicians to input patient characteristics and receive predictions, functioning like an online calculator. Extensive validation, testing, and refinement are required before these applications can be clinically implemented, but the potential benefits justify the investment in further research.
“If the results are true and generalizable, then the consequences are staggering,” stated Derek Angus, MD, a critical care expert. “If one could instantly assign every patient into their appropriate group of predicted benefit or harm and assign their oxygen target accordingly, the intervention would theoretically yield the greatest single improvement in lives saved from critical illness in the history of the field.”
Related Links:
The University of Chicago Medicine