AI Tool Accurately Identifies Patients Who Could Require Emergency Hospital Admission
Posted on 24 Oct 2024
Emergency admissions (EA), where patients require urgent in-hospital care, pose a significant challenge for healthcare systems. Developing risk prediction models can help alleviate this issue by aiding primary care interventions and public health planning. Researchers have now utilized machine learning to create an update for a tool designed to identify individuals at high risk of requiring urgent hospital care within the next 12 months.
The SPARRAv4 – Scottish Patients At Risk of Readmission and Admission – is an algorithm that predicts the risk of EA within the next 12 months. SPARRA was developed using Scotland’s electronic health records (EHR) databases and has been used since 2006 in the country. The current version of the algorithm (SPARRAv3) was implemented in 2012/13 and is calculated monthly by Public Health Scotland (PHS) for nearly the entire Scottish population. General practitioners (GPs) can access individual-level SPARRA scores to help them devise mitigation strategies for patients with complex care needs. Additionally, aggregate SPARRA scores can be utilized to estimate future demand, aiding in planning and resource allocation. SPARRA has also been widely employed in public health research.
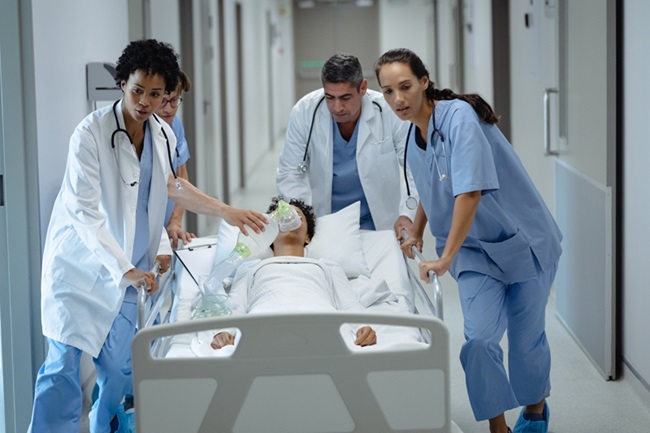
A research team that included investigators from The University of Edinburgh (Edinburgh, UK) has updated the SPARRA algorithm to version 4 (SPARRAv4) using contemporary supervised and unsupervised machine learning (ML) methods. They analyzed health records from 4.8 million residents of Scotland, collected between 2013 and 2018 and maintained by PHS. These records included routinely gathered information by healthcare providers, such as patient history, prescription details, and previous hospital admissions. By applying machine learning techniques to this dataset, the researchers developed SPARRAv4 to predict which patients might need emergency hospital care within a 12-month timeframe.
In tests, the team discovered that SPARRAv4 was more effective at identifying emergency admissions compared to the previous version. According to results published in npj Digital Medicine, SPARRAv4 also demonstrated improved accuracy in assessing individual patients’ risk levels for needing urgent hospital care. The researchers assert that the AI-powered update will assist healthcare providers in Scotland in anticipating and planning for emergency cases more effectively while managing healthcare resources more efficiently. They emphasize that although the tool will be a vital aid, it will not replace the critical clinical judgment of medical professionals.