AI Tool Combines Lung Screening CT with Clinical Data to Predict Lung Cancer without Radiologist Assistance
Posted on 28 Oct 2021
A new artificial intelligence (AI)-based model that combines lung screening computed tomography (CT) information with clinical data has been shown to better predict lung cancer without the need for manual reading.
The deep learning tool built by scientists at the Vanderbilt University (Nashville, TN, USA) integrates CT features such as nodule size and risk factors including age, pack-years smoked, cancer history, among others. The scientists developed and tested the co-learning model on exams of more than 23,000 patients and found that it outperformed risk models utilizing clinical or imaging data alone, including the popular Brock model.
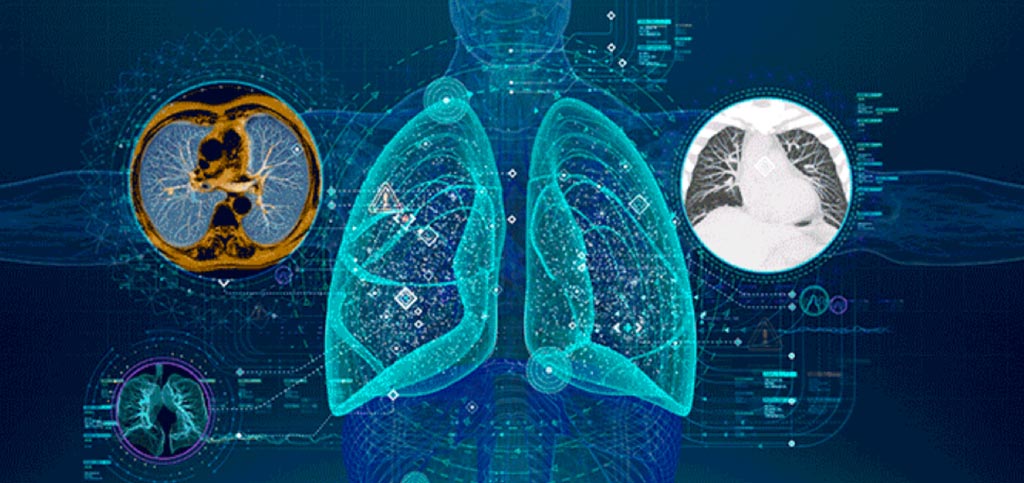
The scientists developed the deep learning tool by applying a five-fold cross-validation approach to data of 23,505 patients from the National Lung Screening Trial. The team used screening data from close to 150 patients in an in-house program for external testing. The scientists found that the deep learning tool notched an area under the receiver operating characteristic curve score of 0.88, which was higher than published models dependent completely on imaging data (0.86) and clinical risk factors (0.69).
More importantly, the deep learning tool automatically pulls high-risk regions from CT exams without the need for any effort by the radiologist. However, gathering clinical data does involve manual effort from radiologists and physicians. The scientists believe that their co-learning approach could be particularly beneficial as more patients start to qualify for screening exams and the insights from the tool could find low-risk individuals to be actually high-risk.
“Risk estimation among lung screening participants will become even more important with the impending expansion of screening guidelines to include those patients who are considered lower risk only based on age and history of tobacco use,” stated Riqiang Gao, a PhD student in computer science at Vanderbilt University. “The role of [the] radiologist is still irreplaceable in terms of looking for and reporting clinically significant findings (emphysema, pulmonary fibrosis, atelectasis, etc.).”
Related Links:
Vanderbilt University