Implantable Neuro-Chip Uses Machine Learning Algorithm to Detect and Treat Neurological Disorders
Posted on 31 Jan 2023
Using a combination of low-power chip design, machine learning algorithms, and soft implantable electrodes, researchers have produced a neural interface that can identify and suppress symptoms of different types of neurological disorders.
NeuralTree, a closed-loop neuromodulation system-on-chip, developed by researchers at EPFL (Lausanne, Switzerland) can detect and alleviate disease symptoms. By utilizing a 256-channel high-resolution sensing array and an energy-efficient machine learning processor, the system can extract and classify a wide range of biomarkers from real patient data and animal models of disease in-vivo, resulting in highly accurate prediction of symptoms. NeuralTree works by extracting neural biomarkers – patterns of electrical signals believed to be associated with specific neurological disorders – from brain waves. It classifies the signals and indicates the possibility of an approaching epileptic seizure or Parkinsonian tremor, for instance. Upon detection of a symptom, a neurostimulator located on the chip becomes activated and sends out an electrical pulse to block it.
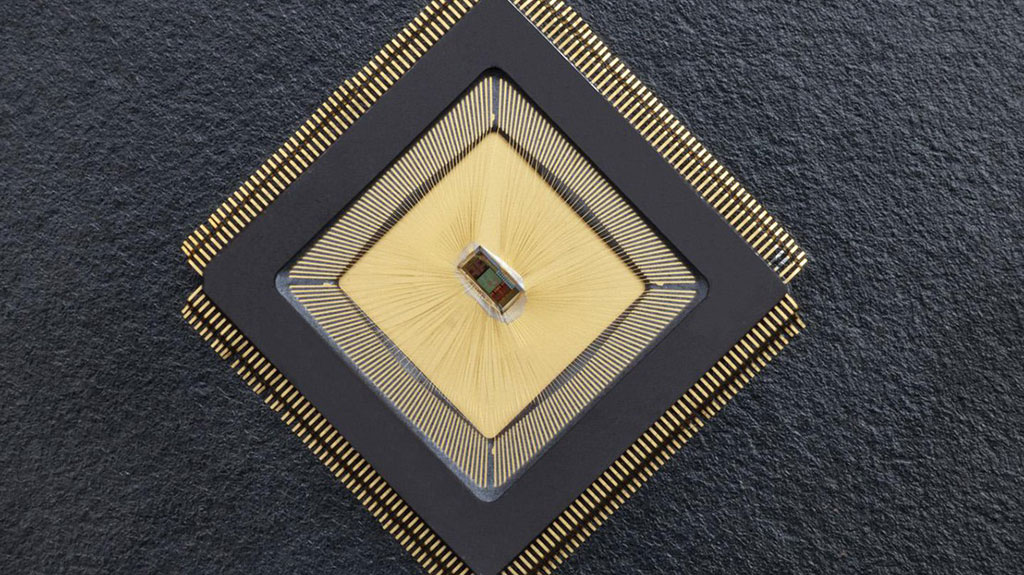
NeuralTree’s unique design provides the highest levels of efficiency and versatility as compared to the state-of-the-art. The chip features 256 input channels, as compared to 32 for previous machine-learning-embedded devices, enabling the implant to process more high-resolution data. The chip’s area-efficient design makes it extremely small (3.48mm2), creating significant potential for scalability to additional channels. The integrated ‘energy-aware’ learning algorithm that penalizes features consuming a lot of power also makes NeuralTree extremely energy efficient.
The system can also detect a wider range of symptoms than other devices, which focus mainly on the detection of epileptic seizures. The researchers trained the chip’s machine learning algorithm on datasets from both epilepsy and Parkinson’s disease patients, and accurately classified pre-recorded neural signals from both the categories. With the aim of making neural interfaces more intelligent for more effective disease control, the researchers are already looking ahead to innovate further. As a next step, the team plans to enable on-chip algorithmic updates in order to keep up with the evolution of neural signals.
“To the best of our knowledge, this is the first demonstration of Parkinsonian tremor detection with an on-chip classifier,” said Mahsa Shoaran of the Integrated Neurotechnologies Laboratory in the School of Engineering. “Eventually, we can use neural interfaces for many different disorders, and we need algorithmic ideas and advances in chip design to make this happen.”
Related Links:
EPFL