Robotics Combined with AI Improves Safety in Emergency Stroke Surgeries
Posted on 20 Jun 2024
Cardiovascular diseases remain the leading cause of death throughout Europe, resulting in over 4 million fatalities annually. Mechanical thrombectomies (MT), which involve the surgical removal of blood clots from large blood vessels, have become a standard treatment for patients experiencing such blockages, particularly strokes. The time between the first onset of stroke symptoms to the initiation of treatment is critical; the sooner the clot is removed, the greater the chance that the patient will regain independence after a stroke. Researchers are now focusing on how surgical robots, autonomously guided by artificial intelligence (AI), might improve the safety and efficiency of these procedures.
Researchers at King’s College London (KCL, London, UK) utilized computer modeling to demonstrate that the initial step of the MT procedure, which involves navigating catheters and wires from the groin into the neck vessels, can be performed autonomously using AI navigation. The team adopted inverse reinforcement learning (IRL) to train new AI models. In their studies to assess the viability of IRL for navigation, they compared the effectiveness of single-device tracking (guidewire alone) versus dual-device tracking (catheter and guidewire together), finding both methods highly successful with success rates of 95% and 96%, respectively.
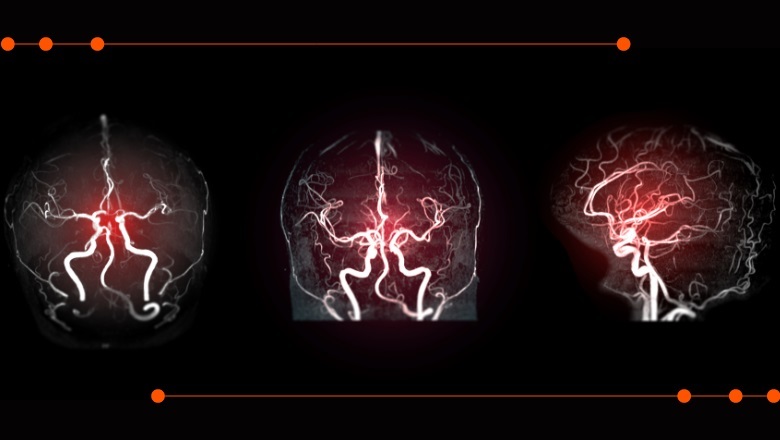
However, the dual-device tracking, which simulates the actions of an expert, showed that integrating IRL with a dense reward function, known as reward shaping, leads to higher overall success rates and reduced procedure times compared to existing methods. The model developed through reward shaping leverages demonstrator data via IRL to navigate towards the target effectively, while the dense reward function encourages quick and efficient progress towards the target, minimizing the number of steps needed.
"Our research uses AI to show, for the first time, how to autonomously navigate medical instruments from the groin to the neck in blood vessels. This is an important part of MT, which removes clots from blood vessels. We also explored various methods to teach the AI,” said King’s PhD student Harry Robertshaw. “We found that using real-life examples to guide the AI, a technique known as 'inverse reinforcement learning', improves its performance compared to the best current methods. Moving forward we can use these new techniques to create models that may be able to navigate unseen patient blood vessels, moving us closer to realizing the full benefits of robotic MT with autonomous assistance.”
“Our work is another step forwards towards improved procedural accessibility and precision of autonomous endovascular navigation tasks,” added Dr. Thomas Booth, Reader in Neuroimaging, School of Biomedical Engineering & Imaging Sciences. “For mechanical thrombectomy, the work plausibly lays the foundation for potentially transformative patient care - for example by treating patients more safely by using AI assistive navigation technologies.”
Related Links:
King’s College London