AI-Assisted Imaging to Assist Endoscopists in Colonoscopy Procedures
Posted on 04 Dec 2024
Colorectal cancer is a major health concern in the United States, with the likelihood of developing the disease being 1 in 25 for women and 1 in 23 for men. Polyps, which are precursors to cancer, can be identified and removed through colonoscopies, of which 15 million are conducted annually in the U.S. These procedures are crucial for diagnosing not only cancer but also conditions like Crohn’s disease, ulcerative colitis, and other colon and rectal disorders. Approximately 30% of polyps are adenomas, a type of precancerous growth, yet the detection rate of adenomas ranges from 7% to 60%. This variation in detection may be attributed to factors such as the skill of the endoscopist, inadequate colon preparation for the procedure, or the challenging locations of some polyps that may be missed. It is also vital that adenomas are fully removed, as any remaining tissue could develop into a cancerous tumor. In a bid to improve the detection of adenomas and other colorectal diseases, a team of interdisciplinary researchers is turning to artificial intelligence (AI) to assist doctors in spotting polyps that even the best current technology may miss.
While AI tools for adenoma detection already exist, they have not significantly improved the identification of smaller or more subtle polyps. These tools mostly identify the adenomas that are already visible to most endoscopists. Researchers from the University of Texas at Austin (Austin, TX, USA) are focusing on detecting the more challenging, smaller polyps by analyzing each colonoscopy image pixel by pixel. Their AI algorithm is being trained to locate adenomas and precisely outline them, ensuring the tumor is detected and completely removed. The pixel-based segmentation helps address variations in colon contours, lighting issues, and even incomplete colon preparations during procedures. This advanced AI is designed to assist endoscopists in distinguishing between healthy and diseased tissue, even when the differences are subtle.
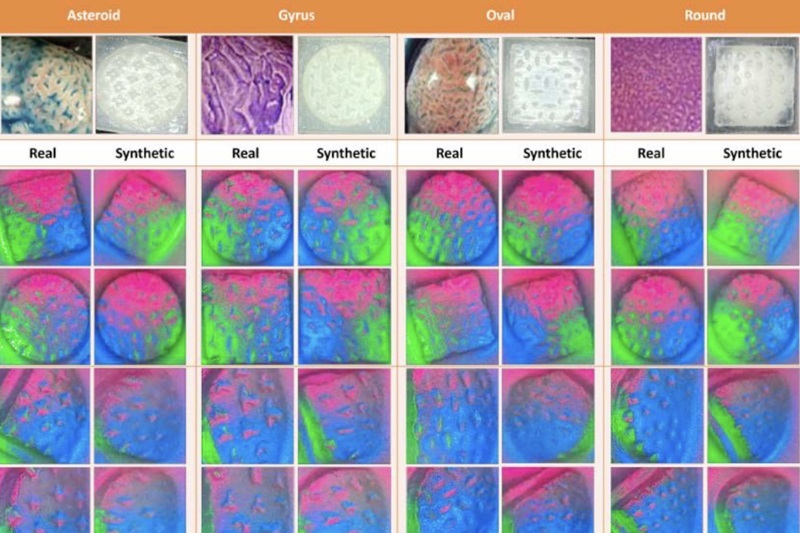
Currently, the only way to evaluate a polyp is through an endoscope, which provides a limited front view. However, the researchers are also developing a device that would allow endoscopists to “feel” the colon lining or a potential polyp before deciding whether to remove it. This device, an inflatable tactile sensor, would be attached to the endoscope. Once a polyp is identified, the endoscopist could move a donut-shaped balloon, about two inches in diameter, over the suspicious area. Using a joystick, the balloon would be inflated with air or liquid, and a sensor covering the balloon would provide tactile feedback about whether the area feels hard or soft, rough or smooth. (Hard or rough surfaces typically indicate a more problematic area.) This tactile sensor functions like a virtual fingertip on any surface and generates its own image, known as a vision-based tactile sensor. The team aims to have the tactile sensor ready for clinical trials within five years.
Related Links:
University of Texas at Austin