NIH Clinical Center Releases CT Image Dataset
By HospiMedica International staff writers Posted on 28 Aug 2018 |
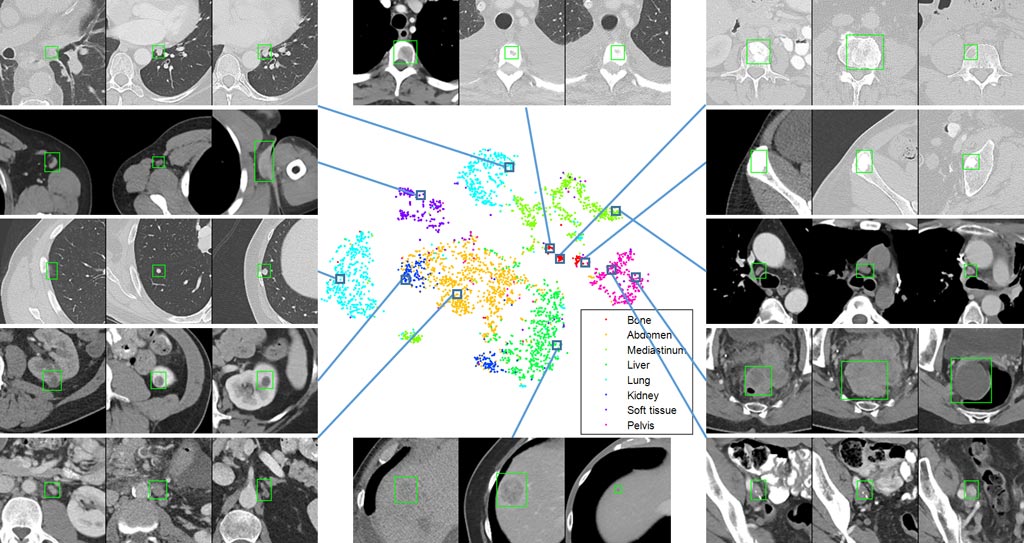
Image: Lesion embedding visualized on the DeepLesion test set (Photo courtesy of NIH).
DeepLesion, a large-scale dataset of CT images compiled by the U.S. National Institutes of Health (NIH, Bethesda, MD, USA) Clinical Center, has been made publicly available to help the scientific community improve detection accuracy of lesions. DeepLesion includes a dataset with 32,735 lesions in 32,120 CT slices from 10,594 studies of 4,427 unique anonymized patients whose CT images were sent to radiologists at the NIH Clinical Center for interpretation.
The NIH radiologists measured and marked clinically meaningful findings with the aid of a complex electronic bookmark tool that provides arrows, lines, diameters, and text that can tell the exact location and size of a lesion so experts can identify growth or new disease. The bookmarks, including a range of retrospective medical data, were used to develop the DeepLesion dataset. Unlike most lesion medical image datasets currently available, which can only detect one type of lesion, the database contains all critical radiology findings, such as lung nodules, liver tumors, enlarged lymph nodes, and so on.
The dataset released is large enough to train a deep neural network, which could enable the scientific community to create a large-scale universal lesion detector with one unified framework that could eventually serve as an initial screening tool for other specialist systems trained on certain types of lesions. In addition, DeepLesion marks multiple findings in one CT exam image, allowing researchers to analyze their relationship to make new discoveries, enabling whole body assessment of cancer burden. DeepLesion was introduced in a study published on July 20, 2018, in the Journal of Medical Imaging.
“Vast amounts of clinical annotations have been collected and stored in hospitals’ picture archiving and communication systems. These types of annotations, also known as bookmarks, are usually marked by radiologists during their daily workflow to highlight significant image findings that may serve as reference for later studies,” said senior author Ronald Summers, MD, PhD, and colleagues. “We propose to mine and harvest these abundant retrospective medical data to build a large-scale lesion image dataset.”
“In the future, the NIH Clinical Center hopes to keep improving the DeepLesion dataset by collecting more data, thus improving its detection accuracy,” stated the NIH in a press release. “The universal lesion detecting capability will become more reliable once researchers are able to leverage 3D and lesion type information. It may be possible to further extend DeepLesion to other image modalities such as MRI and combine data from multiple hospitals, as well.”
Related Links:
U.S. National Institutes of Health
The NIH radiologists measured and marked clinically meaningful findings with the aid of a complex electronic bookmark tool that provides arrows, lines, diameters, and text that can tell the exact location and size of a lesion so experts can identify growth or new disease. The bookmarks, including a range of retrospective medical data, were used to develop the DeepLesion dataset. Unlike most lesion medical image datasets currently available, which can only detect one type of lesion, the database contains all critical radiology findings, such as lung nodules, liver tumors, enlarged lymph nodes, and so on.
The dataset released is large enough to train a deep neural network, which could enable the scientific community to create a large-scale universal lesion detector with one unified framework that could eventually serve as an initial screening tool for other specialist systems trained on certain types of lesions. In addition, DeepLesion marks multiple findings in one CT exam image, allowing researchers to analyze their relationship to make new discoveries, enabling whole body assessment of cancer burden. DeepLesion was introduced in a study published on July 20, 2018, in the Journal of Medical Imaging.
“Vast amounts of clinical annotations have been collected and stored in hospitals’ picture archiving and communication systems. These types of annotations, also known as bookmarks, are usually marked by radiologists during their daily workflow to highlight significant image findings that may serve as reference for later studies,” said senior author Ronald Summers, MD, PhD, and colleagues. “We propose to mine and harvest these abundant retrospective medical data to build a large-scale lesion image dataset.”
“In the future, the NIH Clinical Center hopes to keep improving the DeepLesion dataset by collecting more data, thus improving its detection accuracy,” stated the NIH in a press release. “The universal lesion detecting capability will become more reliable once researchers are able to leverage 3D and lesion type information. It may be possible to further extend DeepLesion to other image modalities such as MRI and combine data from multiple hospitals, as well.”
Related Links:
U.S. National Institutes of Health
Latest Health IT News
- Printable Molecule-Selective Nanoparticles Enable Mass Production of Wearable Biosensors
- Smartwatches Could Detect Congestive Heart Failure
- Versatile Smart Patch Combines Health Monitoring and Drug Delivery
- Machine Learning Model Improves Mortality Risk Prediction for Cardiac Surgery Patients
- Strategic Collaboration to Develop and Integrate Generative AI into Healthcare
- AI-Enabled Operating Rooms Solution Helps Hospitals Maximize Utilization and Unlock Capacity
- AI Predicts Pancreatic Cancer Three Years before Diagnosis from Patients’ Medical Records
- First Fully Autonomous Generative AI Personalized Medical Authorizations System Reduces Care Delay
- Electronic Health Records May Be Key to Improving Patient Care, Study Finds
- AI Trained for Specific Vocal Biomarkers Could Accurately Predict Coronary Artery Disease
Channels
Critical Care
view channel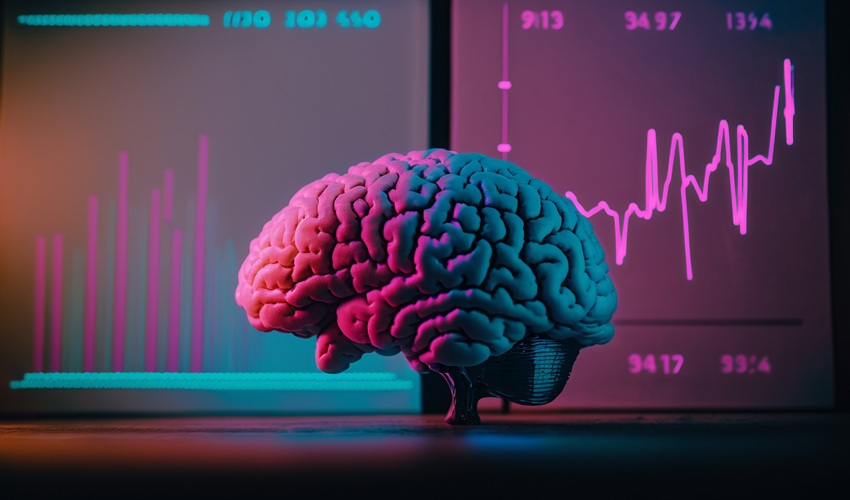
AI Model Analyzes Patient Data to Diagnose Multiple Sclerosis With 90% Accuracy
Multiple sclerosis (MS) is a chronic inflammatory condition affecting the central nervous system. Most patients initially experience the relapsing-remitting form (RRMS), characterized by periods of symptom... Read more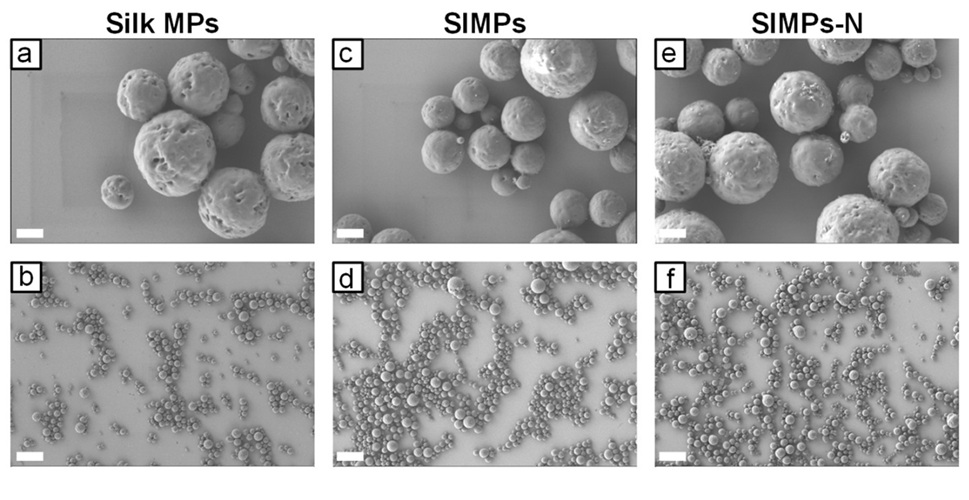
Magnetically Navigable Microparticles Enable Targeted Drug Delivery
Abdominal aortic aneurysms (AAA) can be life-threatening if not treated and result in nearly 10,000 deaths annually. Researchers working to improve treatments for AAA could now make it possible for doctors... Read more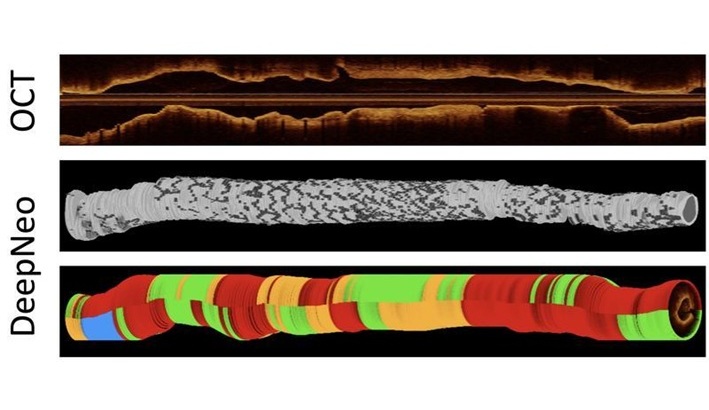
AI-Powered Algorithm Automates Analysis of Coronary Stents After Implantation
Every year, over three million people globally receive stents to open blocked blood vessels caused by heart disease. However, monitoring the healing process after stent implantation remains a significant challenge.... Read moreSurgical Techniques
view channel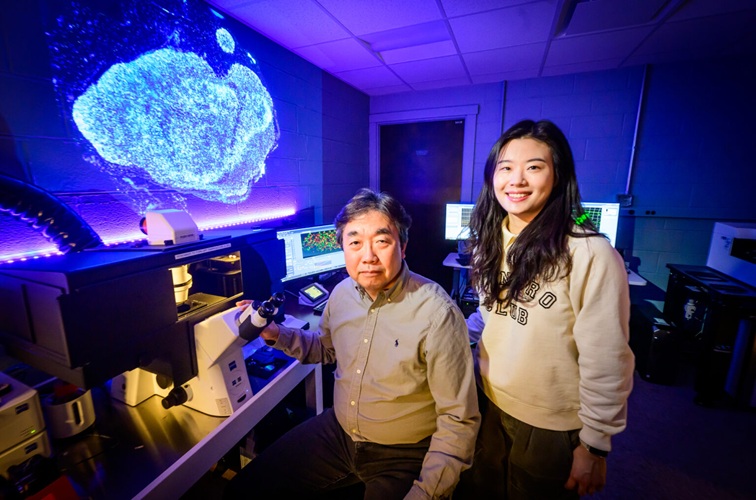
DNA Origami Improves Imaging of Dense Pancreatic Tissue for Cancer Detection and Treatment
One of the challenges of fighting pancreatic cancer is finding ways to penetrate the organ’s dense tissue to define the margins between malignant and normal tissue. Now, a new study uses DNA origami structures... Read more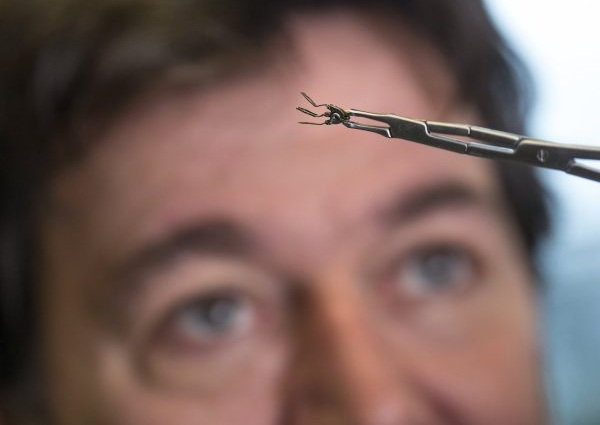
Pioneering Sutureless Coronary Bypass Technology to Eliminate Open-Chest Procedures
In patients with coronary artery disease, certain blood vessels may be narrowed or blocked, requiring a stent or a bypass (also known as diversion) to restore blood flow to the heart. Bypass surgeries... Read more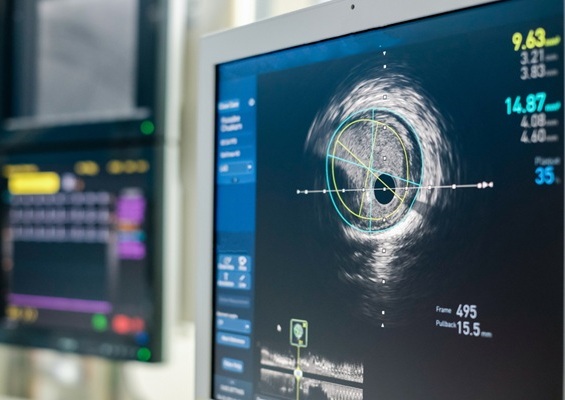
Intravascular Imaging for Guiding Stent Implantation Ensures Safer Stenting Procedures
Patients diagnosed with coronary artery disease, which is caused by plaque accumulation within the arteries leading to chest pain, shortness of breath, and potential heart attacks, frequently undergo percutaneous... Read more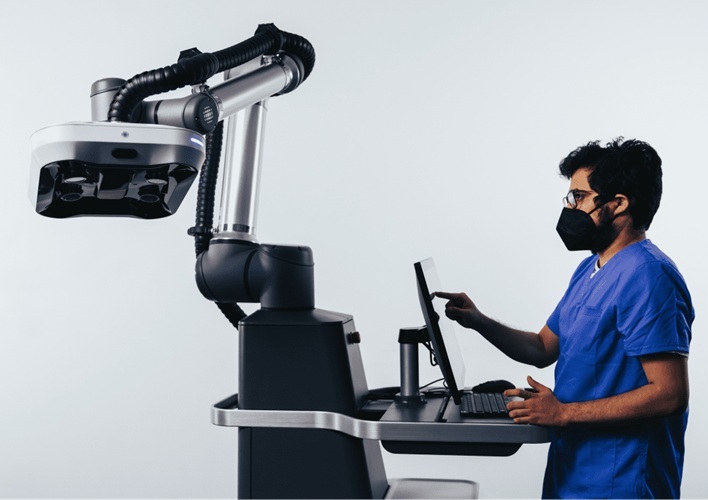
World's First AI Surgical Guidance Platform Allows Surgeons to Measure Success in Real-Time
Surgeons have always faced challenges in measuring their progress toward surgical goals during procedures. Traditionally, obtaining measurements required stepping out of the sterile environment to perform... Read morePatient Care
view channel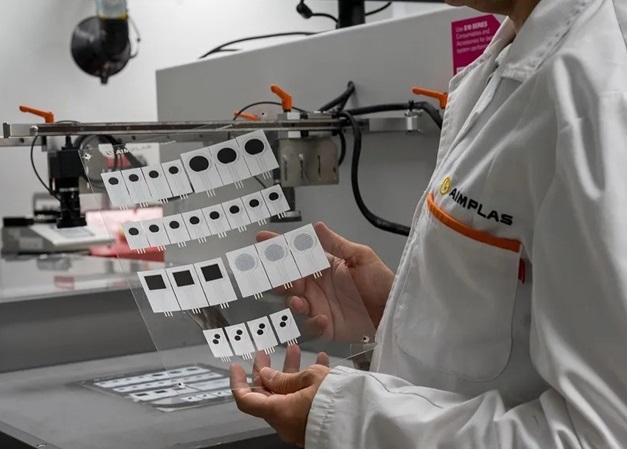
Portable Biosensor Platform to Reduce Hospital-Acquired Infections
Approximately 4 million patients in the European Union acquire healthcare-associated infections (HAIs) or nosocomial infections each year, with around 37,000 deaths directly resulting from these infections,... Read more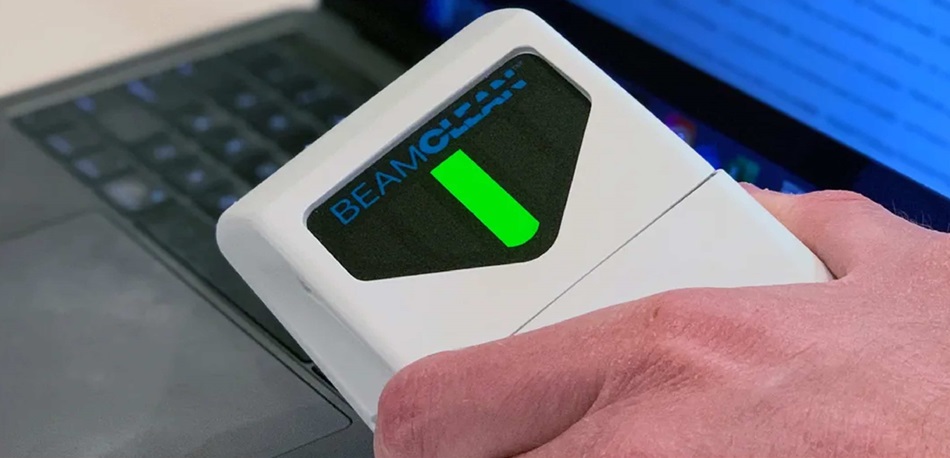
First-Of-Its-Kind Portable Germicidal Light Technology Disinfects High-Touch Clinical Surfaces in Seconds
Reducing healthcare-acquired infections (HAIs) remains a pressing issue within global healthcare systems. In the United States alone, 1.7 million patients contract HAIs annually, leading to approximately... Read more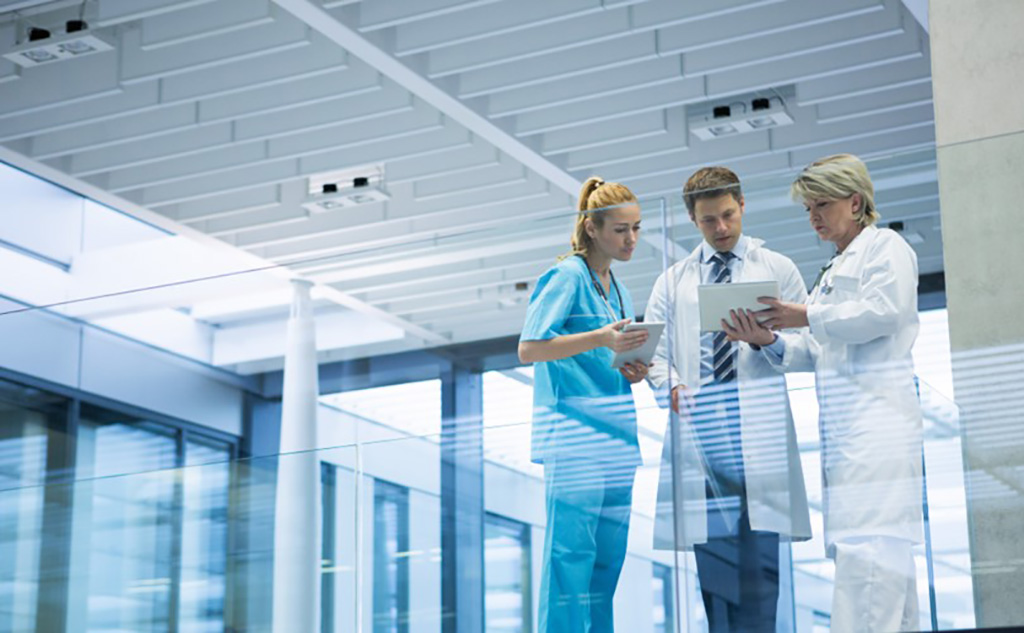
Surgical Capacity Optimization Solution Helps Hospitals Boost OR Utilization
An innovative solution has the capability to transform surgical capacity utilization by targeting the root cause of surgical block time inefficiencies. Fujitsu Limited’s (Tokyo, Japan) Surgical Capacity... Read more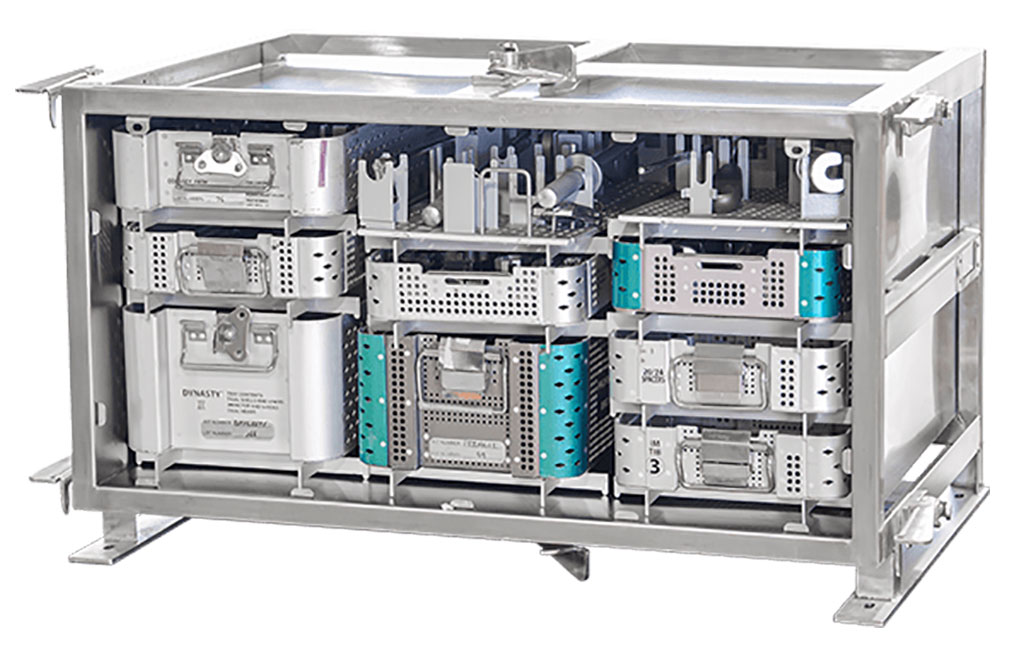
Game-Changing Innovation in Surgical Instrument Sterilization Significantly Improves OR Throughput
A groundbreaking innovation enables hospitals to significantly improve instrument processing time and throughput in operating rooms (ORs) and sterile processing departments. Turbett Surgical, Inc.... Read moreHealth IT
view channel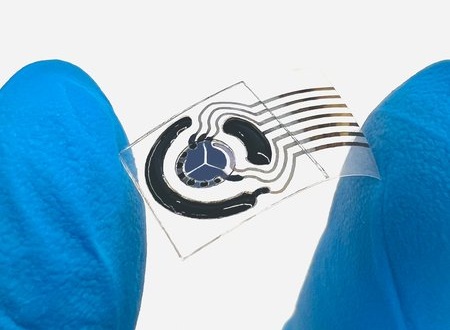
Printable Molecule-Selective Nanoparticles Enable Mass Production of Wearable Biosensors
The future of medicine is likely to focus on the personalization of healthcare—understanding exactly what an individual requires and delivering the appropriate combination of nutrients, metabolites, and... Read more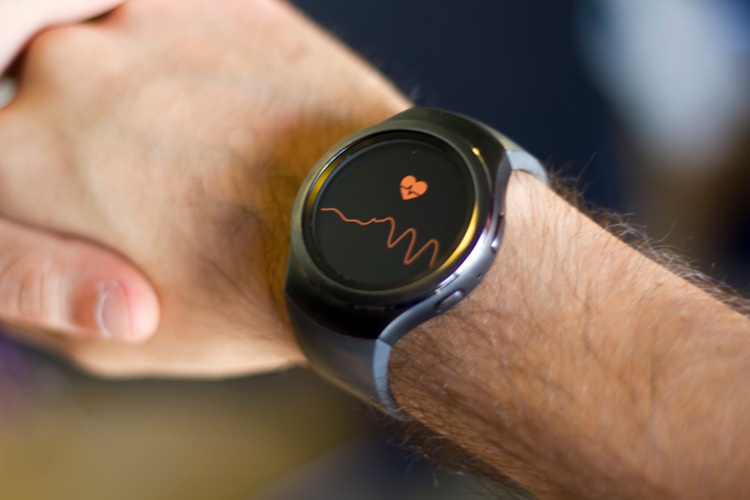
Smartwatches Could Detect Congestive Heart Failure
Diagnosing congestive heart failure (CHF) typically requires expensive and time-consuming imaging techniques like echocardiography, also known as cardiac ultrasound. Previously, detecting CHF by analyzing... Read moreBusiness
view channel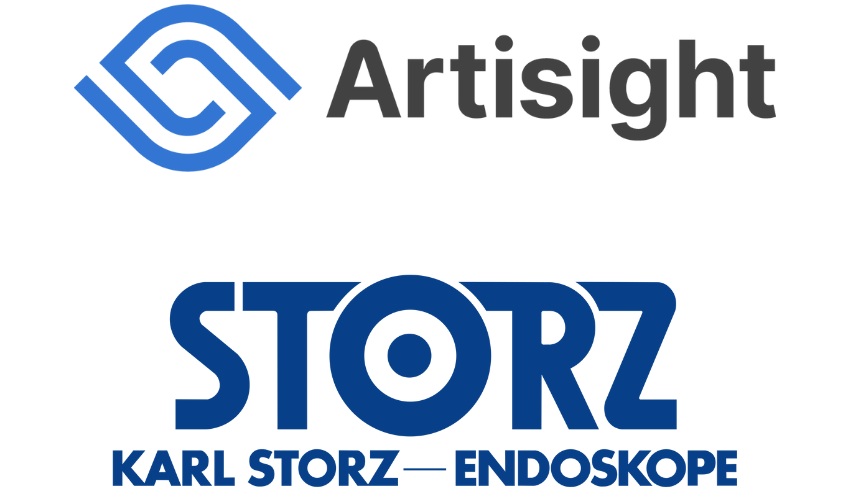
Expanded Collaboration to Transform OR Technology Through AI and Automation
The expansion of an existing collaboration between three leading companies aims to develop artificial intelligence (AI)-driven solutions for smart operating rooms with sophisticated monitoring and automation.... Read more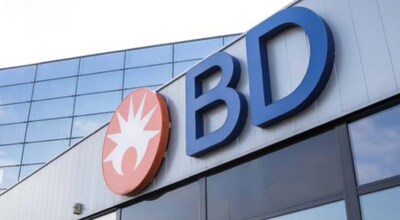