New Self-Learning AI-Based Algorithm Reads Electrocardiograms to Spot Unseen Signs of Heart Failure
By HospiMedica International staff writers Posted on 20 Oct 2021 |
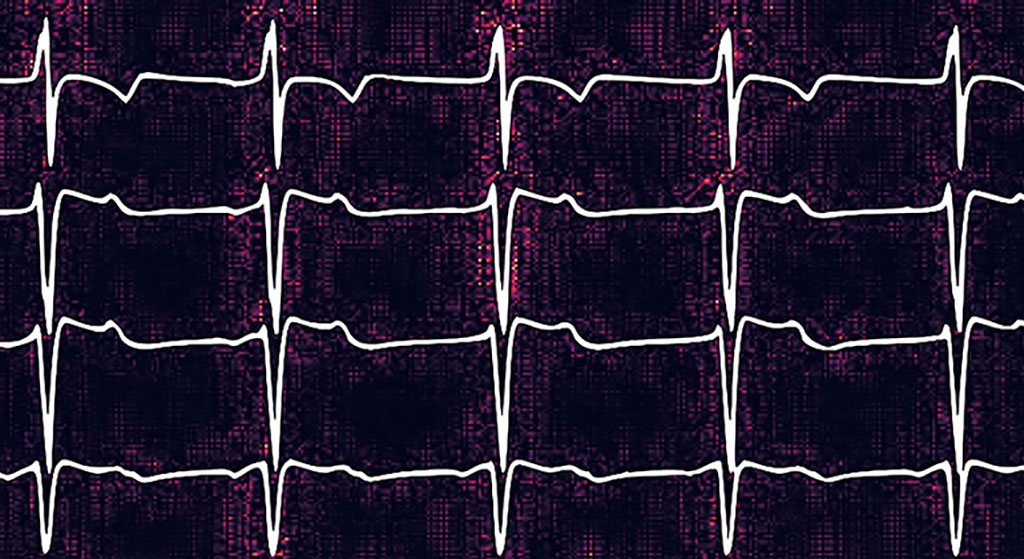
A new self-learning algorithm can detect blood pumping problems by reading electrocardiograms (also known as ECGs or EKGs) to predict whether a patient was experiencing heart failure.
The special artificial intelligence (AI)-based computer algorithm created by researchers at Mount Sinai (New York, NY, USA) was able to learn how to identify subtle changes in electrocardiograms (also known as ECGs or EKGs) to predict whether a patient was experiencing heart failure. Heart failure, or congestive heart failure, occurs when the heart pumps less blood than the body normally needs. For years, doctors have relied heavily on an imaging technique called an echocardiogram to assess whether a patient may be experiencing heart failure. While helpful, echocardiograms can be labor-intensive procedures that are only offered at select hospitals.
However, recent breakthroughs in AI suggest that electrocardiograms - a widely used electrical recording device - could be a fast and readily available alternative in these cases. For instance, many studies have shown how a “deep-learning” algorithm can detect weakness in the heart’s left ventricle, which pushes freshly oxygenated blood out to the rest of the body. In this study, the researchers described the development of an algorithm that not only assessed the strength of the left ventricle but also the right ventricle, which takes deoxygenated blood streaming in from the body and pumps it to the lungs.
Typically, an electrocardiogram involves a two-step process. Wire leads are taped to different parts of a patient’s chest and within minutes a specially designed, portable machine prints out a series of squiggly lines, or waveforms, representing the heart’s electrical activity. These machines can be found in most hospitals and ambulances and require minimal training to operate. For this study, the researchers programmed a computer to read patient electrocardiograms along with data extracted from written reports summarizing the results of corresponding echocardiograms taken from the same patients. In this situation, the written reports acted as a standard set of data for the computer to compare with the electrocardiogram data and learn how to spot weaker hearts.
Natural language processing programs helped the computer extract data from the written reports. Meanwhile, special neural networks capable of discovering patterns in images were incorporated to help the algorithm learn to recognize pumping strengths. The computer then read more than 700,000 electrocardiograms and echocardiogram reports obtained from 150,000 Mount Sinai Health System patients from 2003 to 2020. Data from four hospitals was used to train the computer, whereas data from a fifth one was used to test how the algorithm would perform in a different experimental setting. Initial results suggested that the algorithm was effective at predicting which patients would have either healthy or very weak left ventricles. Here strength was defined by left ventricle ejection fraction, an estimate of how much fluid the ventricle pumps out with each beat as observed on echocardiograms. Healthy hearts have an ejection fraction of 50% or greater while weak hearts have ones that are equal to or below 40%.
The algorithm was 94% accurate at predicting which patients had a healthy ejection fraction and 87% accurate at predicting those who had an ejection fraction that was below 40%. However the algorithm was not as effective at predicting which patients would have slightly weakened hearts. In this case, the program was 73% accurate at predicting the patients who had an ejection fraction that was between 40% and 50%. Further results suggested that the algorithm also learned to detect right valve weaknesses from the electrocardiograms. In this case, weakness was defined by more descriptive terms extracted from the echocardiogram reports. Here the algorithm was 84% accurate at predicting which patients had weak right valves. Finally, additional analysis suggested that the algorithm may be effective at detecting heart weakness in all patients, regardless of race and gender.
“We showed that deep-learning algorithms can recognize blood pumping problems on both sides of the heart from ECG waveform data,” said Benjamin S. Glicksberg, PhD, Assistant Professor of Genetics and Genomic Sciences, a member of the Hasso Plattner Institute for Digital Health at Mount Sinai, and a senior author of the study published in the Journal of the American College of Cardiology: Cardiovascular Imaging. “Ordinarily, diagnosing these type of heart conditions requires expensive and time-consuming procedures. We hope that this algorithm will enable quicker diagnosis of heart failure.”
“This study represents an exciting step forward in finding information hidden within the ECG data which can lead to better screening and treatment paradigms using a relatively simple and widely available test,” said Girish N. Nadkarni, MD, MPH, CPH, Associate Professor of Medicine at the Icahn School of Medicine at Mount Sinai, Chief of the Division of Data-Driven and Digital Medicine (D3M), and a senior author of the study. “A potential advantage of this study is that it involved one of the largest collections of ECGs from one of the most diverse patient populations in the world.”
Related Links:
Mount Sinai
Latest Health IT News
- Printable Molecule-Selective Nanoparticles Enable Mass Production of Wearable Biosensors
- Smartwatches Could Detect Congestive Heart Failure
- Versatile Smart Patch Combines Health Monitoring and Drug Delivery
- Machine Learning Model Improves Mortality Risk Prediction for Cardiac Surgery Patients
- Strategic Collaboration to Develop and Integrate Generative AI into Healthcare
- AI-Enabled Operating Rooms Solution Helps Hospitals Maximize Utilization and Unlock Capacity
- AI Predicts Pancreatic Cancer Three Years before Diagnosis from Patients’ Medical Records
- First Fully Autonomous Generative AI Personalized Medical Authorizations System Reduces Care Delay
- Electronic Health Records May Be Key to Improving Patient Care, Study Finds
- AI Trained for Specific Vocal Biomarkers Could Accurately Predict Coronary Artery Disease
Channels
Critical Care
view channel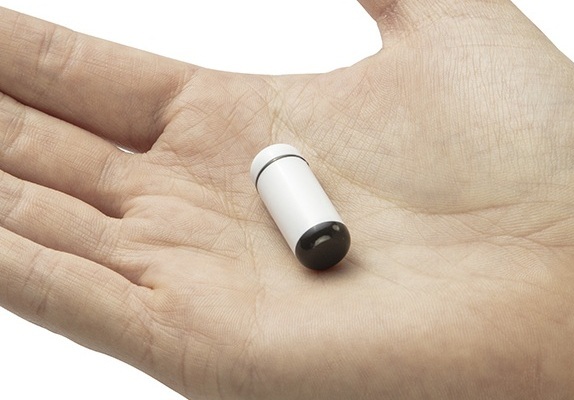
Ingestible Smart Capsule for Chemical Sensing in the Gut Moves Closer to Market
Intestinal gases are associated with several health conditions, including colon cancer, irritable bowel syndrome, and inflammatory bowel disease, and they have the potential to serve as crucial biomarkers... Read more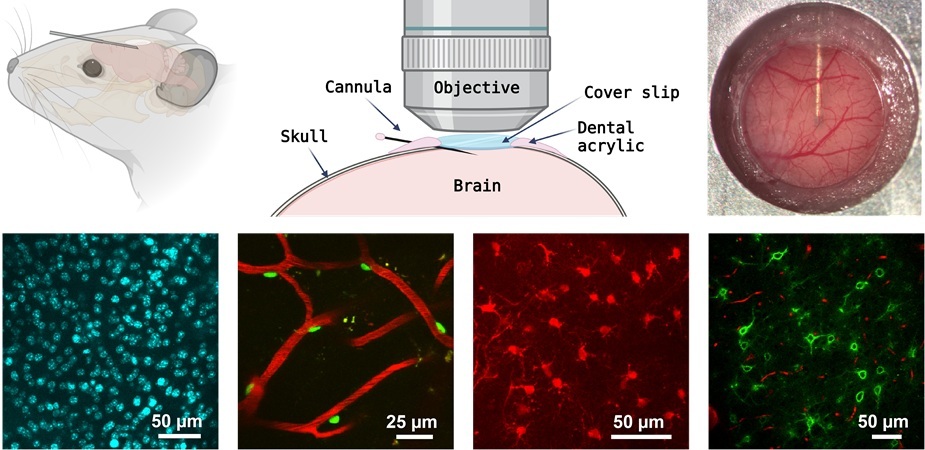
Novel Cannula Delivery System Enables Targeted Delivery of Imaging Agents and Drugs
Multiphoton microscopy has become an invaluable tool in neuroscience, allowing researchers to observe brain activity in real time with high-resolution imaging. A crucial aspect of many multiphoton microscopy... Read more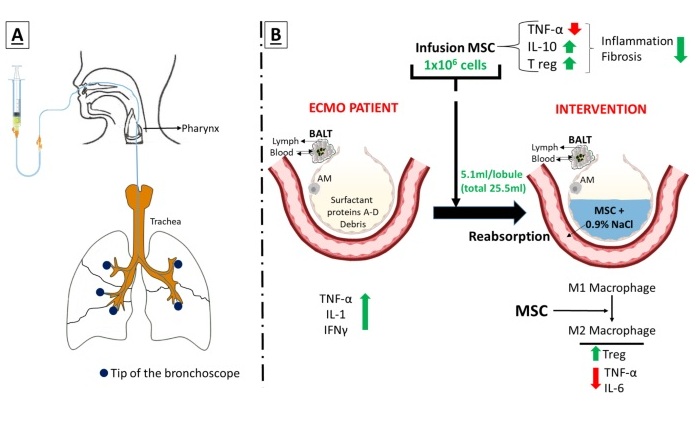
Novel Intrabronchial Method Delivers Cell Therapies in Critically Ill Patients on External Lung Support
Until now, administering cell therapies to patients on extracorporeal membrane oxygenation (ECMO)—a life-support system typically used for severe lung failure—has been nearly impossible.... Read moreSurgical Techniques
view channel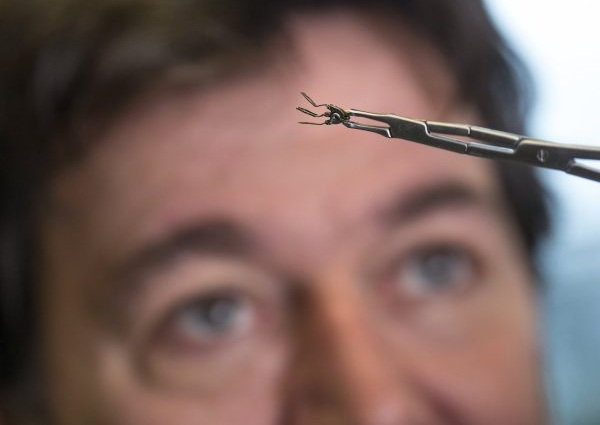
Pioneering Sutureless Coronary Bypass Technology to Eliminate Open-Chest Procedures
In patients with coronary artery disease, certain blood vessels may be narrowed or blocked, requiring a stent or a bypass (also known as diversion) to restore blood flow to the heart. Bypass surgeries... Read more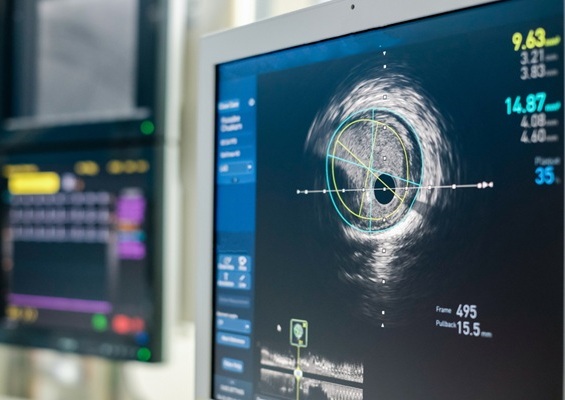
Intravascular Imaging for Guiding Stent Implantation Ensures Safer Stenting Procedures
Patients diagnosed with coronary artery disease, which is caused by plaque accumulation within the arteries leading to chest pain, shortness of breath, and potential heart attacks, frequently undergo percutaneous... Read more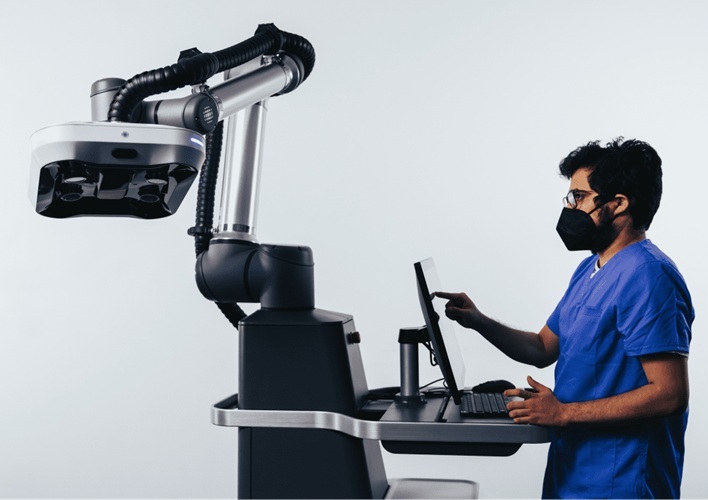
World's First AI Surgical Guidance Platform Allows Surgeons to Measure Success in Real-Time
Surgeons have always faced challenges in measuring their progress toward surgical goals during procedures. Traditionally, obtaining measurements required stepping out of the sterile environment to perform... Read morePatient Care
view channel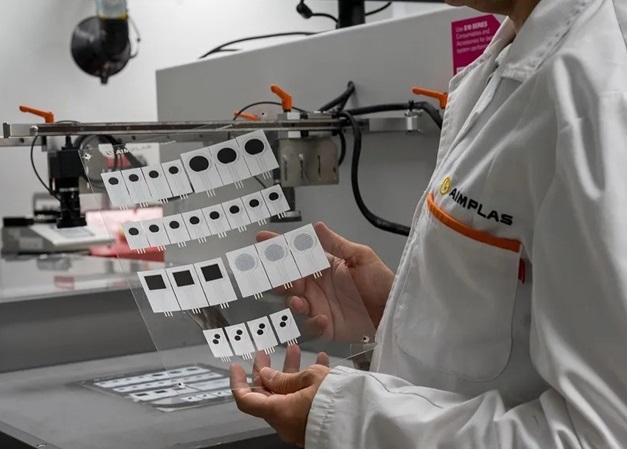
Portable Biosensor Platform to Reduce Hospital-Acquired Infections
Approximately 4 million patients in the European Union acquire healthcare-associated infections (HAIs) or nosocomial infections each year, with around 37,000 deaths directly resulting from these infections,... Read more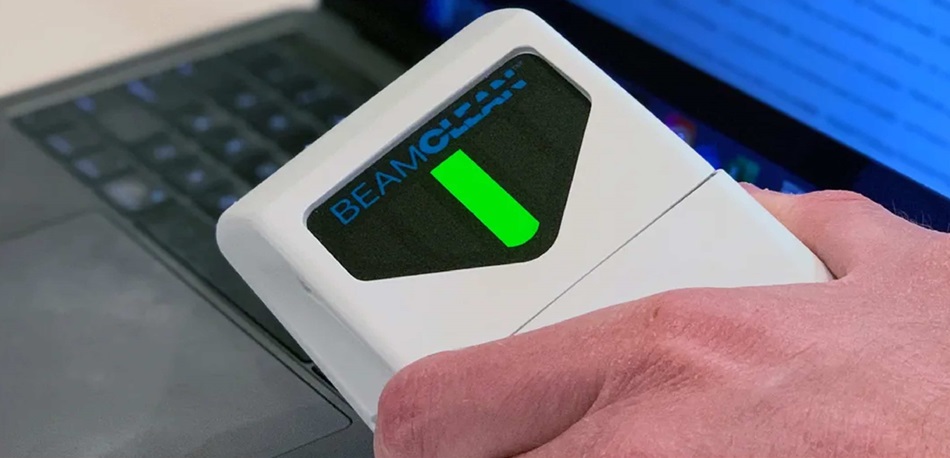
First-Of-Its-Kind Portable Germicidal Light Technology Disinfects High-Touch Clinical Surfaces in Seconds
Reducing healthcare-acquired infections (HAIs) remains a pressing issue within global healthcare systems. In the United States alone, 1.7 million patients contract HAIs annually, leading to approximately... Read more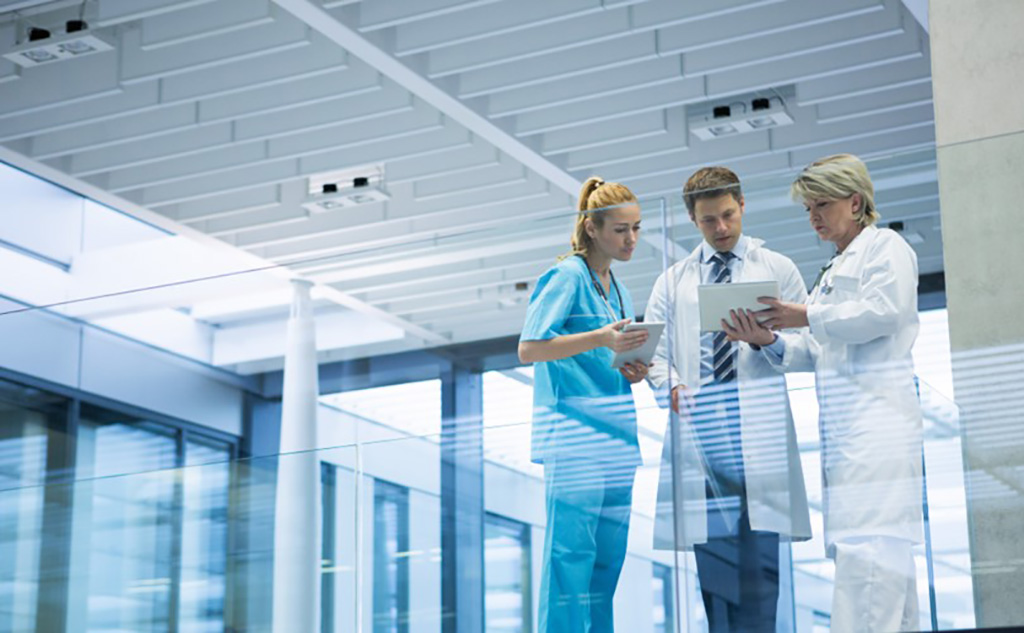
Surgical Capacity Optimization Solution Helps Hospitals Boost OR Utilization
An innovative solution has the capability to transform surgical capacity utilization by targeting the root cause of surgical block time inefficiencies. Fujitsu Limited’s (Tokyo, Japan) Surgical Capacity... Read more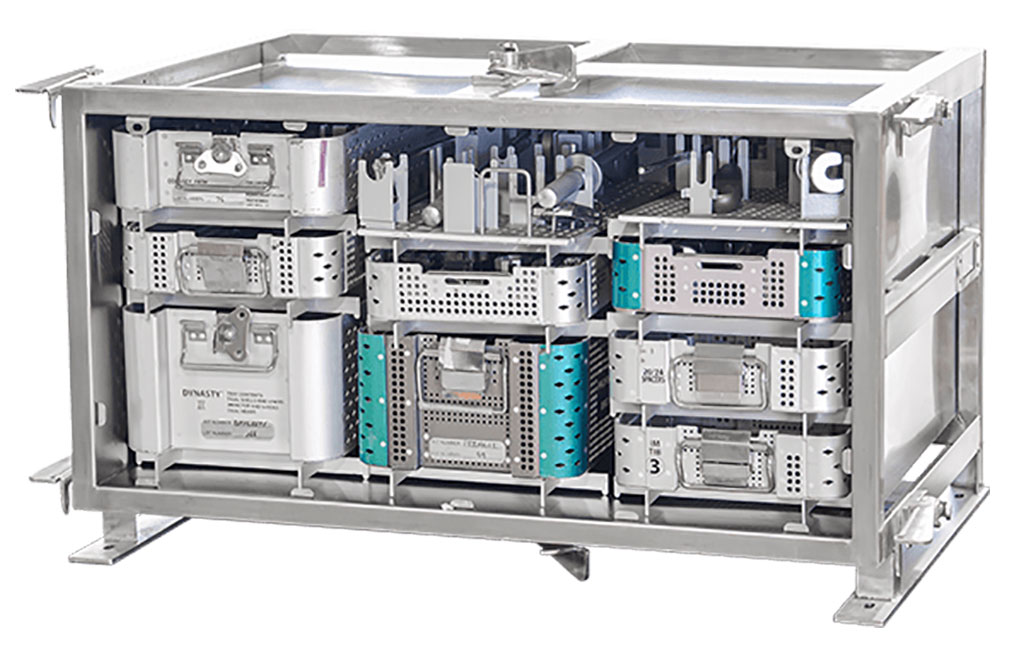
Game-Changing Innovation in Surgical Instrument Sterilization Significantly Improves OR Throughput
A groundbreaking innovation enables hospitals to significantly improve instrument processing time and throughput in operating rooms (ORs) and sterile processing departments. Turbett Surgical, Inc.... Read moreBusiness
view channel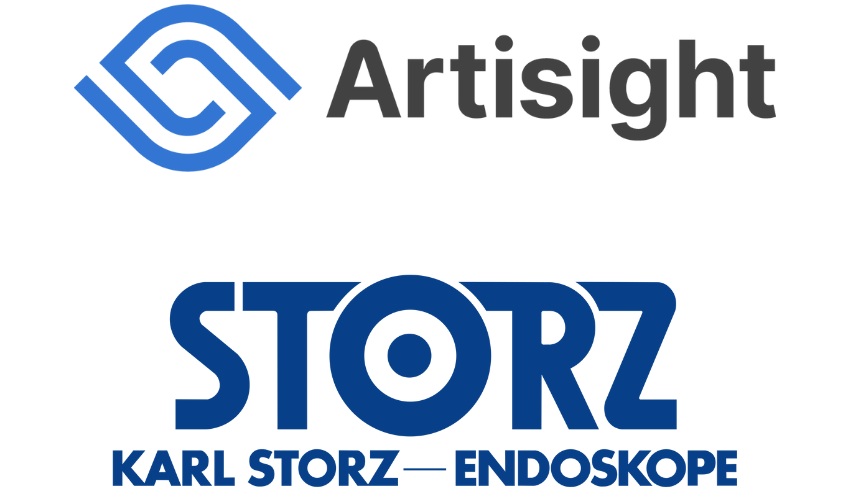
Expanded Collaboration to Transform OR Technology Through AI and Automation
The expansion of an existing collaboration between three leading companies aims to develop artificial intelligence (AI)-driven solutions for smart operating rooms with sophisticated monitoring and automation.... Read more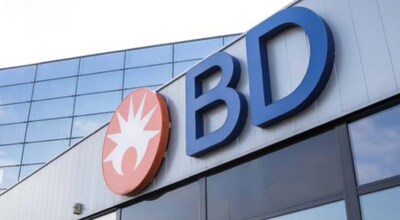