Machine Learning Model Improves Mortality Risk Prediction for Cardiac Surgery Patients
By HospiMedica International staff writers Posted on 26 May 2023 |
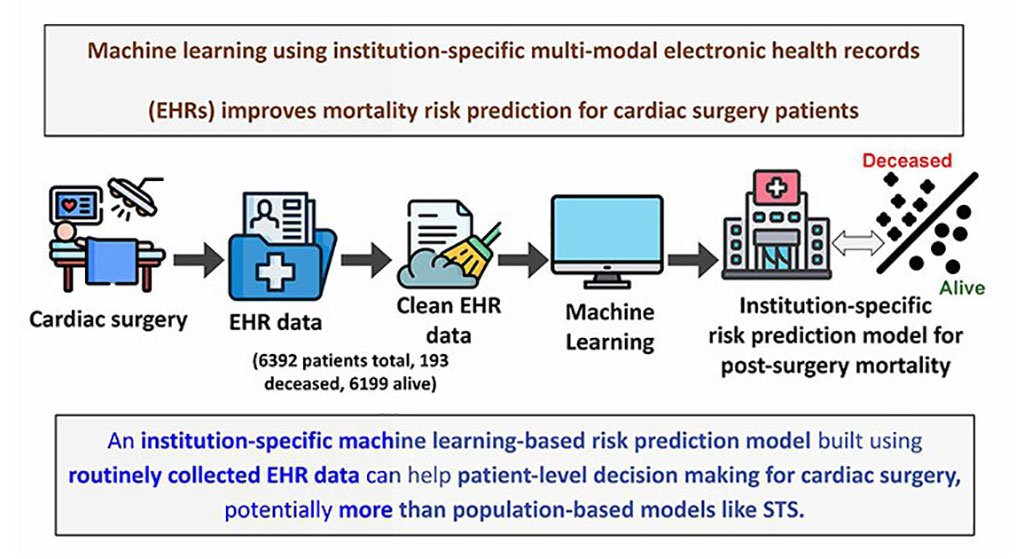
Machine learning algorithms have been deployed to create predictive models in various medical fields, with some demonstrating improved outcomes compared to their standard-of-care counterparts. In cardiac surgery, risk scores provided by The Society of Thoracic Surgeons (STS) are often used to evaluate a patient's procedural risk. While these scores remain vital for hospitals to assess and improve their performance, they are drawn from population-wide data, which can fall short of accurately predicting risk for specific patients with complex pathologies.
Now, cardiovascular surgeons and data science specialists at Mount Sinai (New York, NY, USA) have developed a machine learning-based model that predicts mortality risk for individual cardiac surgery patients, offering a considerable performance advantage over current population-based models. This data-driven algorithm, built on extensive electronic health records (EHR), is the first institution-specific model of its kind for pre-surgery cardiac patient risk assessment. It allows healthcare providers to determine the optimal treatment strategy for each patient.
The team theorized that models based on EHR data from their own institution, created via machine learning, could provide a useful solution. Using routinely gathered EHR data, they developed a robust machine learning framework to generate a risk prediction model for post-surgery mortality that is customized to both the patient and the hospital. This model incorporates vital data about Mount Sinai’s patient population, including demographic, socioeconomic, and health characteristics. This is in contrast to population-based models like STS, which rely on data from various health systems across the U.S. The effectiveness of this approach is further enhanced by an efficient open-source prediction algorithm called XGBoost, which assembles a group of decision trees by progressively focusing on harder-to-predict segments of training data.
The research team utilized XGBoost to model 6,392 cardiac surgeries conducted at The Mount Sinai Hospital from 2011 to 2016, encompassing heart valve procedures, coronary artery bypass grafts, aortic resections, replacements, or anastomoses, and reoperative cardiac surgeries, which significantly increase mortality risk. The team then compared the performance of their model to STS models for the same patient sets. The study found that the XGBoost model outshone STS risk scores for mortality in all frequently performed cardiac surgery categories for which STS scores were designed. The predictive performance of the XGBoost model across all types of surgeries was also high, indicating the potential of machine learning and EHR data for constructing effective institution-specific models.
“The standard-of-care risk models used today are limited by their applicability to specific types of surgeries, leaving out significant numbers of patients undergoing complex or combination procedures for which no models exist,” said senior author Ravi Iyengar, PhD, the Dorothy H. and Lewis Rosenstiel Professor of Pharmacological Sciences at the Icahn School of Medicine at Mount Sinai, and Director of the Mount Sinai Institute for Systems Biomedicine. “Our team rigorously combined electronic health record data and machine learning methods to demonstrate for the first time how individual institutions can build their own risk models for post-cardiac surgery mortality.”
Related Links:
Mount Sinai
Latest Health IT News
- Printable Molecule-Selective Nanoparticles Enable Mass Production of Wearable Biosensors
- Smartwatches Could Detect Congestive Heart Failure
- Versatile Smart Patch Combines Health Monitoring and Drug Delivery
- Strategic Collaboration to Develop and Integrate Generative AI into Healthcare
- AI-Enabled Operating Rooms Solution Helps Hospitals Maximize Utilization and Unlock Capacity
- AI Predicts Pancreatic Cancer Three Years before Diagnosis from Patients’ Medical Records
- First Fully Autonomous Generative AI Personalized Medical Authorizations System Reduces Care Delay
- Electronic Health Records May Be Key to Improving Patient Care, Study Finds
- AI Trained for Specific Vocal Biomarkers Could Accurately Predict Coronary Artery Disease
Channels
Critical Care
view channel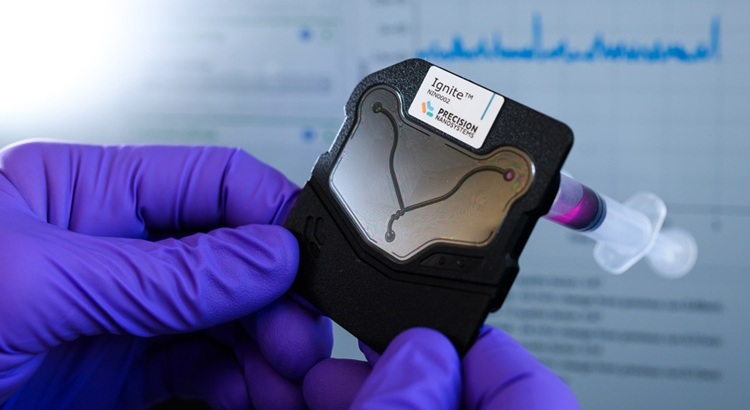
Mass Manufactured Nanoparticles to Deliver Cancer Drugs Directly to Tumors
Polymer-coated nanoparticles loaded with therapeutic drugs hold significant potential for treating cancers, including ovarian cancer. These particles can be precisely directed to tumors, delivering their... Read more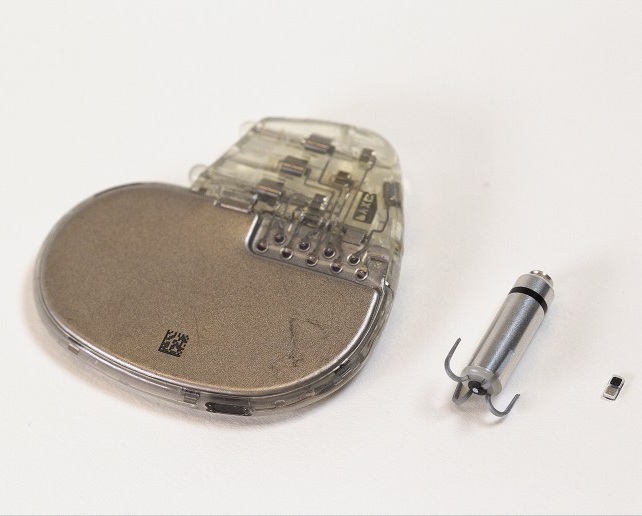
World’s Smallest Pacemaker Fits Inside Syringe Tip
After heart surgery, many patients require temporary pacemakers either to regulate the heart rate while waiting for a permanent pacemaker or to support normal heart rhythm during recovery.... Read more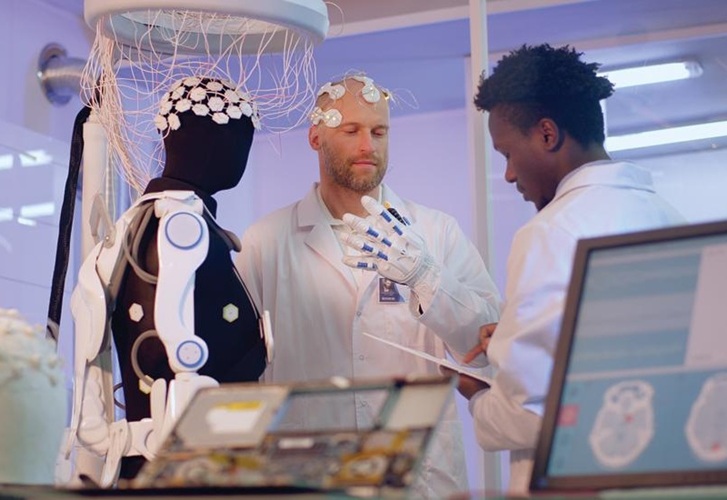
AI-Powered, Internet-Connected Medical Devices to Revolutionize Healthcare, Finds Study
A new study suggests that artificial intelligence (AI)-powered, internet-connected medical devices have the potential to transform healthcare by enabling earlier detection of diseases, real-time patient... Read moreSurgical Techniques
view channel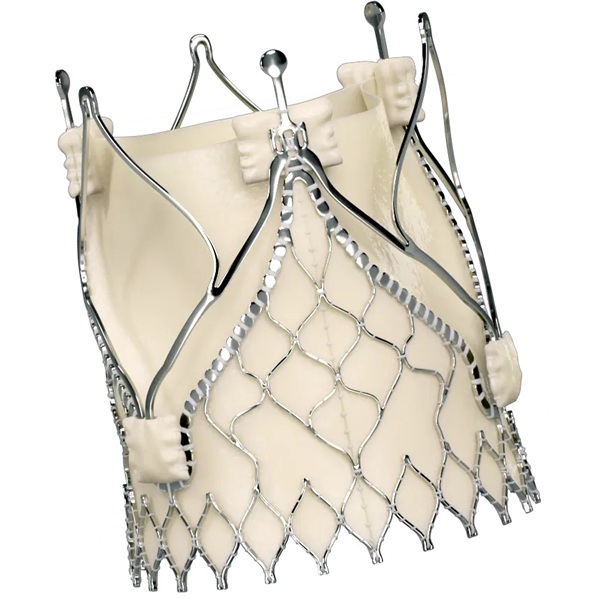
New Transcatheter Valve Found Safe and Effective for Treating Aortic Regurgitation
Aortic regurgitation is a condition in which the aortic valve does not close properly, allowing blood to flow backward into the left ventricle. This results in decreased blood flow from the heart to the... Read more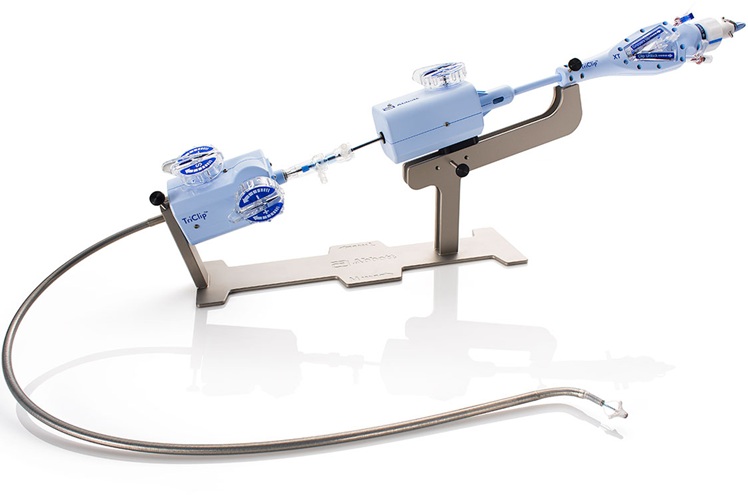
Minimally Invasive Valve Repair Reduces Hospitalizations in Severe Tricuspid Regurgitation Patients
The tricuspid valve is one of the four heart valves, responsible for regulating blood flow from the right atrium (the heart's upper-right chamber) to the right ventricle (the lower-right chamber).... Read morePatient Care
view channel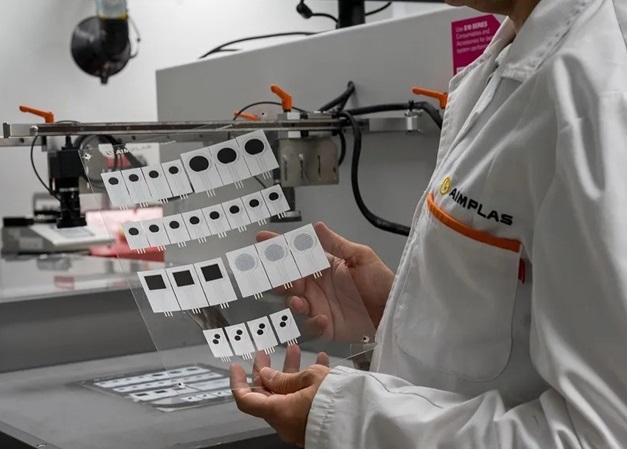
Portable Biosensor Platform to Reduce Hospital-Acquired Infections
Approximately 4 million patients in the European Union acquire healthcare-associated infections (HAIs) or nosocomial infections each year, with around 37,000 deaths directly resulting from these infections,... Read more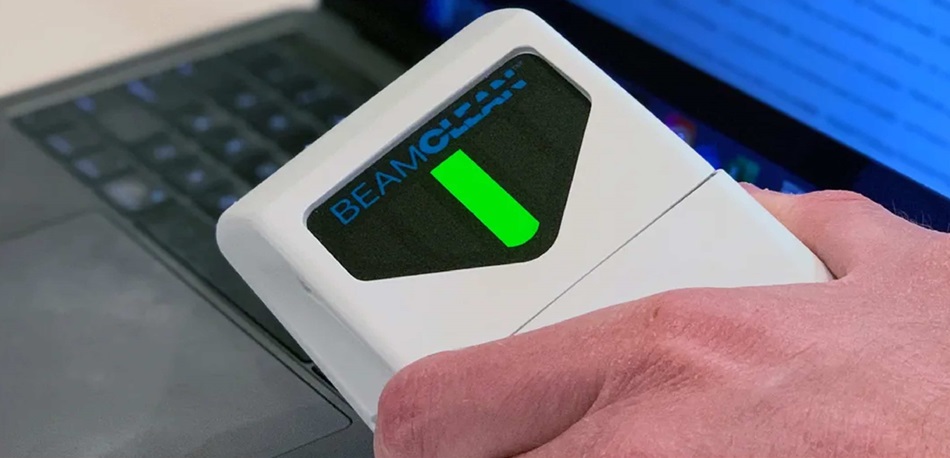
First-Of-Its-Kind Portable Germicidal Light Technology Disinfects High-Touch Clinical Surfaces in Seconds
Reducing healthcare-acquired infections (HAIs) remains a pressing issue within global healthcare systems. In the United States alone, 1.7 million patients contract HAIs annually, leading to approximately... Read more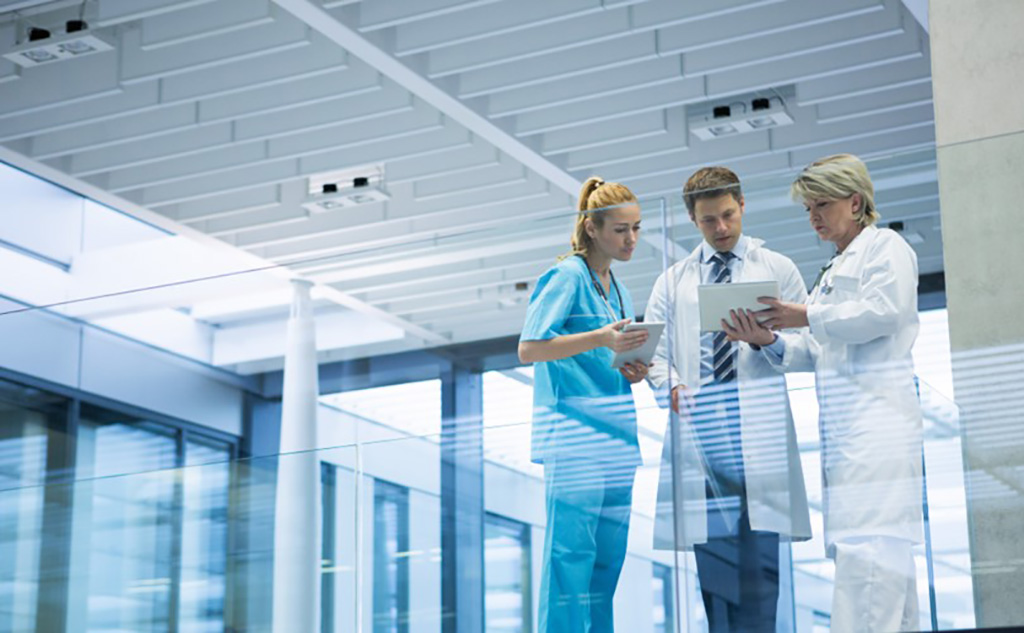
Surgical Capacity Optimization Solution Helps Hospitals Boost OR Utilization
An innovative solution has the capability to transform surgical capacity utilization by targeting the root cause of surgical block time inefficiencies. Fujitsu Limited’s (Tokyo, Japan) Surgical Capacity... Read more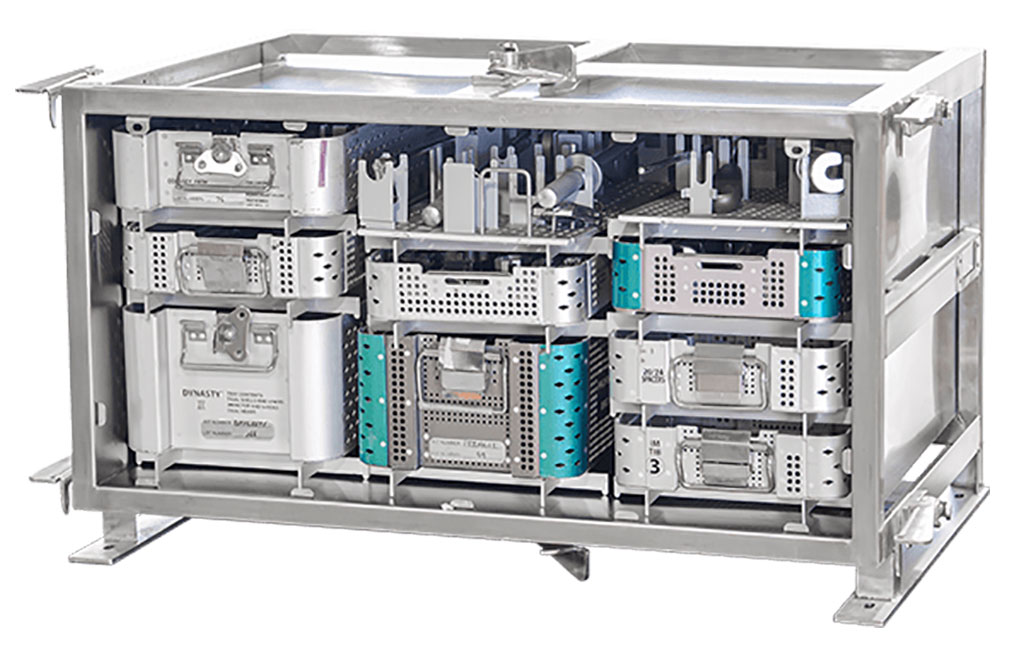
Game-Changing Innovation in Surgical Instrument Sterilization Significantly Improves OR Throughput
A groundbreaking innovation enables hospitals to significantly improve instrument processing time and throughput in operating rooms (ORs) and sterile processing departments. Turbett Surgical, Inc.... Read morePoint of Care
view channel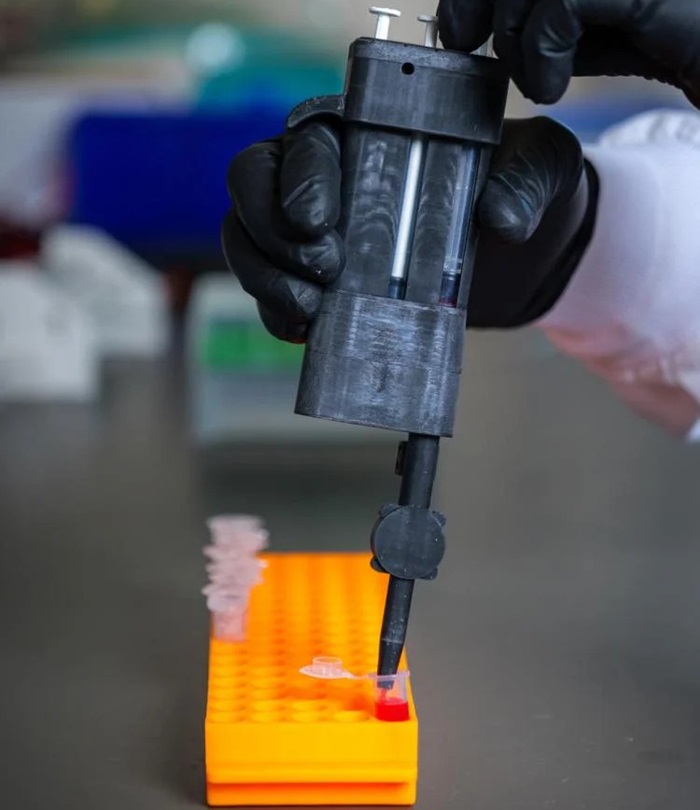
Handheld, Sound-Based Diagnostic System Delivers Bedside Blood Test Results in An Hour
Patients who go to a doctor for a blood test often have to contend with a needle and syringe, followed by a long wait—sometimes hours or even days—for lab results. Scientists have been working hard to... Read moreBusiness
view channel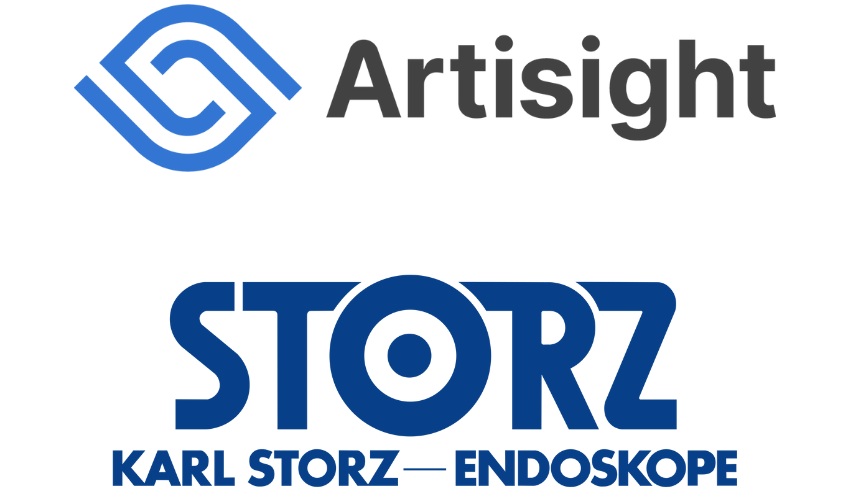
Expanded Collaboration to Transform OR Technology Through AI and Automation
The expansion of an existing collaboration between three leading companies aims to develop artificial intelligence (AI)-driven solutions for smart operating rooms with sophisticated monitoring and automation.... Read more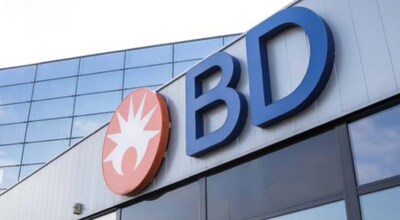