Sophisticated Machine-Learning Approach Uses Patient EHRs to Predict Pneumonia Outcomes
By HospiMedica International staff writers Posted on 30 Oct 2024 |
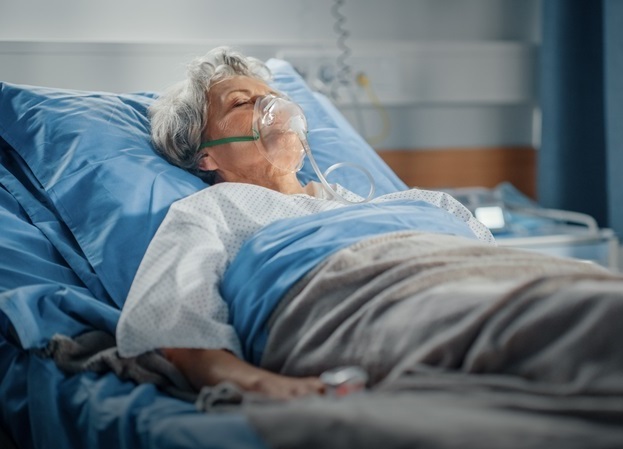
Pneumonia, an infection that results in difficulty breathing due to fluid accumulation in the lungs, is one of the leading causes of death worldwide. This condition is particularly challenging to treat because of the various ways it can manifest and be contracted, along with the risk of antibiotic overuse. Two patients suffering from pneumonia can present very differently and may experience contrasting outcomes. Traditionally, physicians have classified pneumonia patients in intensive care units based on the cause of the infection into three categories: community-acquired (which may follow a prior bacterial or viral infection), hospital-acquired, and ventilator-associated (developing after mechanical ventilation). However, this classification often provides minimal insight into a patient’s likelihood of recovery, making it difficult for doctors to accurately predict prognoses and determine the best treatment strategies. Now, a novel approach could assist clinicians in making more informed treatment decisions for critically ill patients and may have broader applications.
Researchers at Northwestern University (Evanston, IL, USA) have employed a sophisticated machine-learning method on electronic health records (EHRs) from pneumonia patients to identify five distinct clinical states. Three of these states are closely linked to patient outcomes, while the other two aid physicians in determining the cause of the disease. One identified state correlates with a 7.5% chance of mortality within 24 hours. Understanding individual survival probabilities can help prepare family members for the potential loss and guide physicians in avoiding unnecessary treatments. The research team faced multiple challenges while developing a suite of machine-learning tools to cluster patient conditions from two EHR data sources: one from Northwestern’s SCRIPT project and another from a standard clinical dataset.
First, they had to integrate various data types that were collected at different frequencies. Additionally, they needed to devise a new test to evaluate the reliability of their approach. Finally, they had to assess whether the information from these physiological variables could be condensed into fewer combinations. This analysis allowed the researchers to identify five distinct clusters—equating to different clinical states—that significantly outperformed current methods in predicting patient mortality. These five states incorporate a range of data (such as body temperature, respiratory rate, glucose levels, and oxygenation) to reveal relationships between different measures.
The study, published in the journal Proceedings of the National Academy of Sciences (PNAS), shows that linear combinations of variables reflecting motor response, renal function, heart rate, systolic blood pressure, and respiratory rate provided the most insight into patient status. Notably, one of the identified clusters primarily consisted of patients whose pneumonia was linked to COVID-19 infections. The technical advancements achieved during this research may have applications in other areas. In fact, the team is currently applying these methodologies to experimental data from a mouse model of sepsis. They have yet to explore why certain patients transition between states, a topic they are now investigating. Future research on pneumonia and other diseases may ultimately lead to more effective and predictable treatment options.
Latest Health IT News
- Printable Molecule-Selective Nanoparticles Enable Mass Production of Wearable Biosensors
- Smartwatches Could Detect Congestive Heart Failure
- Versatile Smart Patch Combines Health Monitoring and Drug Delivery
- Machine Learning Model Improves Mortality Risk Prediction for Cardiac Surgery Patients
- Strategic Collaboration to Develop and Integrate Generative AI into Healthcare
- AI-Enabled Operating Rooms Solution Helps Hospitals Maximize Utilization and Unlock Capacity
- AI Predicts Pancreatic Cancer Three Years before Diagnosis from Patients’ Medical Records
- First Fully Autonomous Generative AI Personalized Medical Authorizations System Reduces Care Delay
- Electronic Health Records May Be Key to Improving Patient Care, Study Finds
- AI Trained for Specific Vocal Biomarkers Could Accurately Predict Coronary Artery Disease
Channels
Surgical Techniques
view channel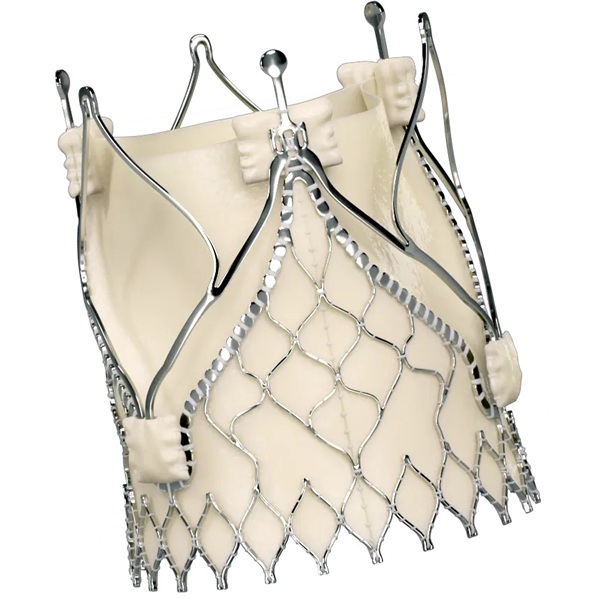
New Transcatheter Valve Found Safe and Effective for Treating Aortic Regurgitation
Aortic regurgitation is a condition in which the aortic valve does not close properly, allowing blood to flow backward into the left ventricle. This results in decreased blood flow from the heart to the... Read more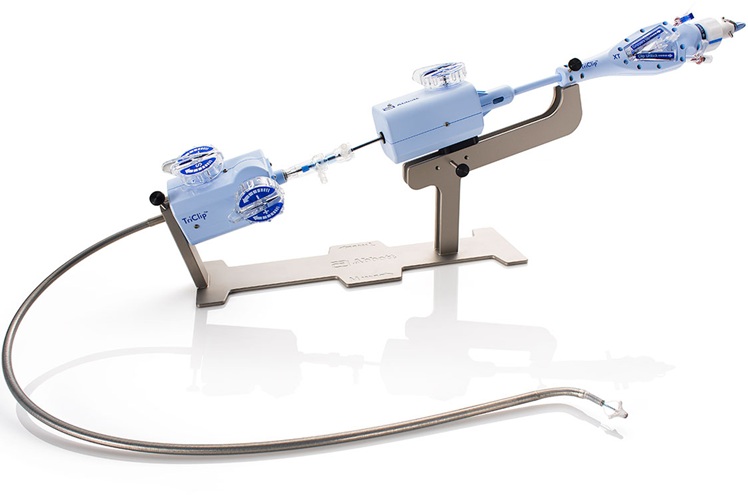
Minimally Invasive Valve Repair Reduces Hospitalizations in Severe Tricuspid Regurgitation Patients
The tricuspid valve is one of the four heart valves, responsible for regulating blood flow from the right atrium (the heart's upper-right chamber) to the right ventricle (the lower-right chamber).... Read morePatient Care
view channel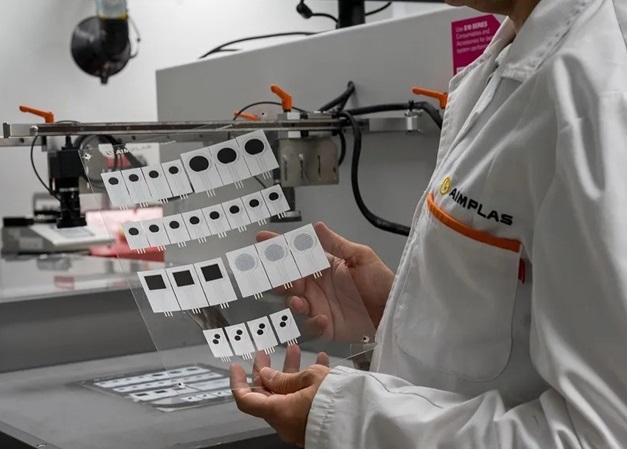
Portable Biosensor Platform to Reduce Hospital-Acquired Infections
Approximately 4 million patients in the European Union acquire healthcare-associated infections (HAIs) or nosocomial infections each year, with around 37,000 deaths directly resulting from these infections,... Read more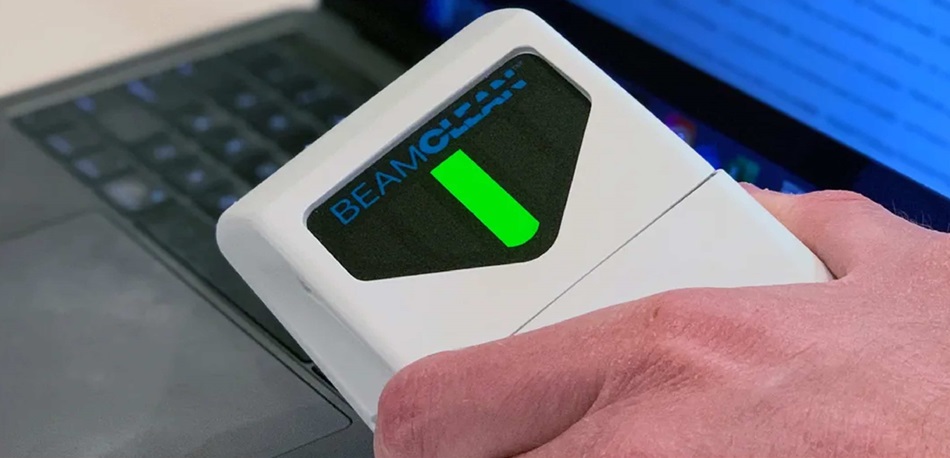
First-Of-Its-Kind Portable Germicidal Light Technology Disinfects High-Touch Clinical Surfaces in Seconds
Reducing healthcare-acquired infections (HAIs) remains a pressing issue within global healthcare systems. In the United States alone, 1.7 million patients contract HAIs annually, leading to approximately... Read more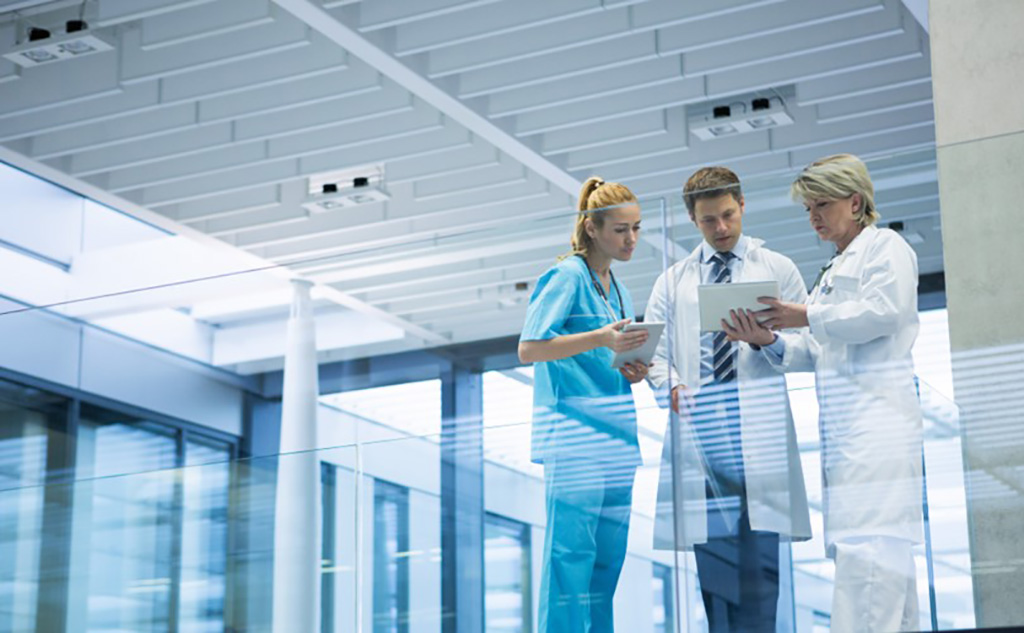
Surgical Capacity Optimization Solution Helps Hospitals Boost OR Utilization
An innovative solution has the capability to transform surgical capacity utilization by targeting the root cause of surgical block time inefficiencies. Fujitsu Limited’s (Tokyo, Japan) Surgical Capacity... Read more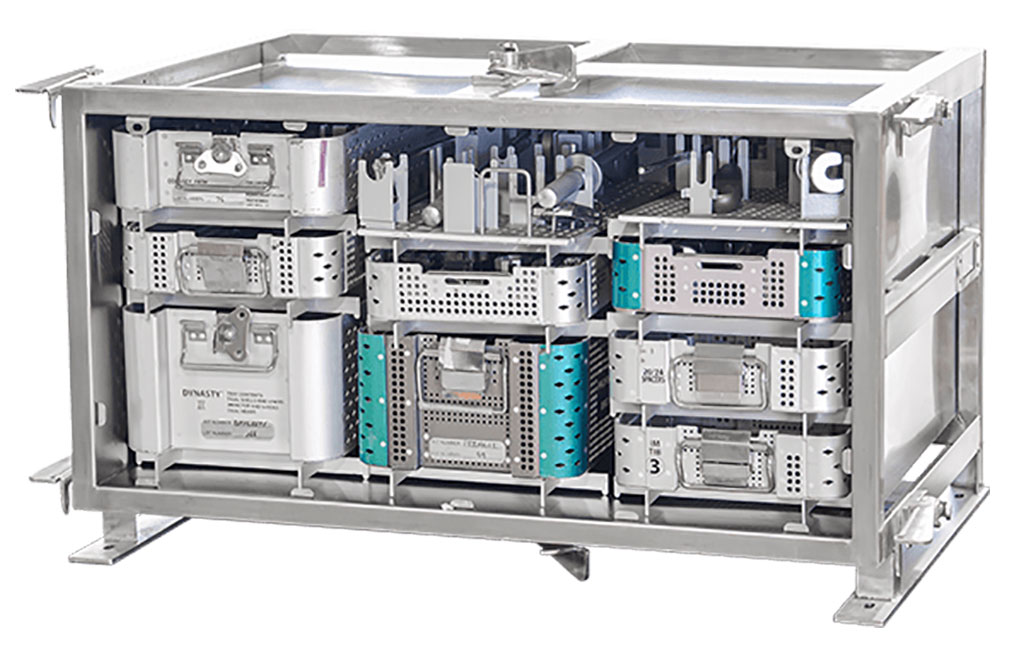
Game-Changing Innovation in Surgical Instrument Sterilization Significantly Improves OR Throughput
A groundbreaking innovation enables hospitals to significantly improve instrument processing time and throughput in operating rooms (ORs) and sterile processing departments. Turbett Surgical, Inc.... Read moreHealth IT
view channel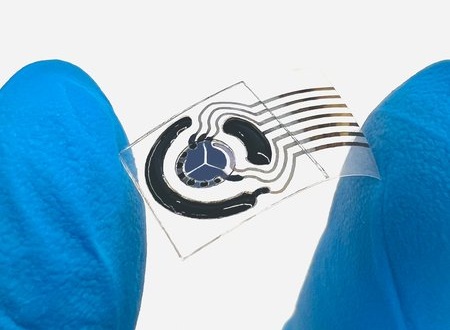
Printable Molecule-Selective Nanoparticles Enable Mass Production of Wearable Biosensors
The future of medicine is likely to focus on the personalization of healthcare—understanding exactly what an individual requires and delivering the appropriate combination of nutrients, metabolites, and... Read more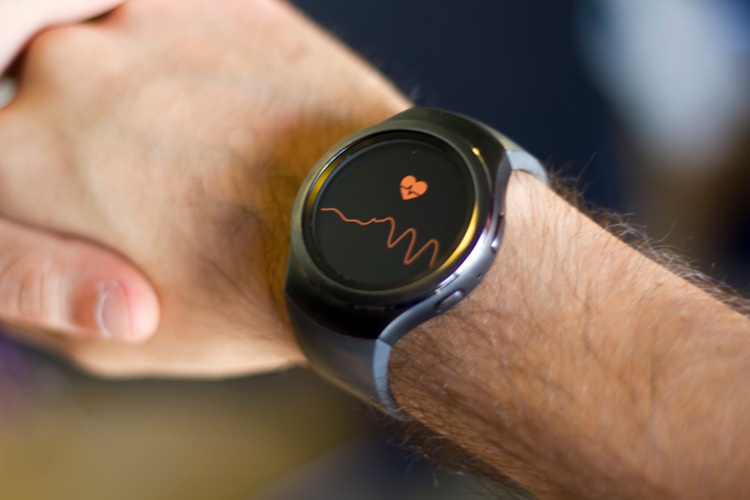
Smartwatches Could Detect Congestive Heart Failure
Diagnosing congestive heart failure (CHF) typically requires expensive and time-consuming imaging techniques like echocardiography, also known as cardiac ultrasound. Previously, detecting CHF by analyzing... Read morePoint of Care
view channel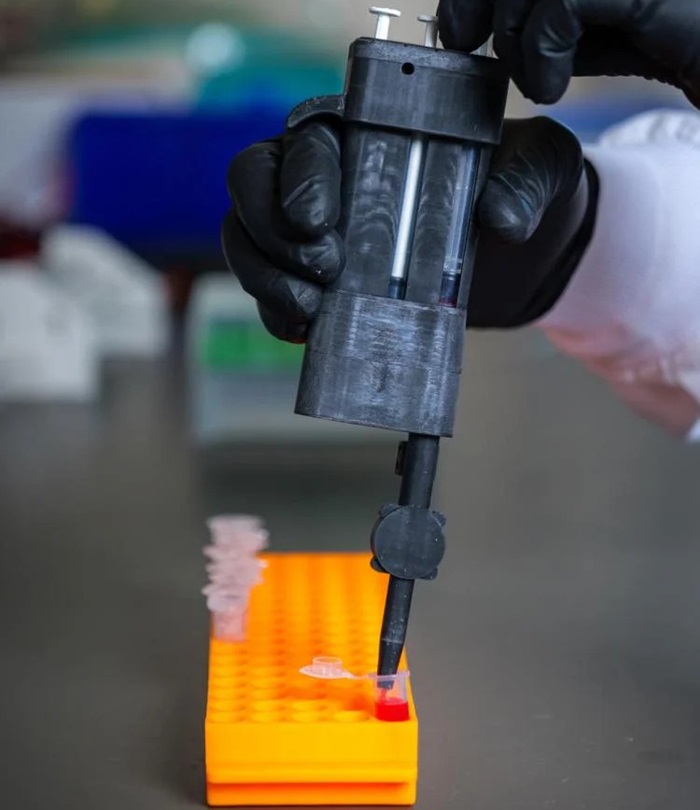
Handheld, Sound-Based Diagnostic System Delivers Bedside Blood Test Results in An Hour
Patients who go to a doctor for a blood test often have to contend with a needle and syringe, followed by a long wait—sometimes hours or even days—for lab results. Scientists have been working hard to... Read more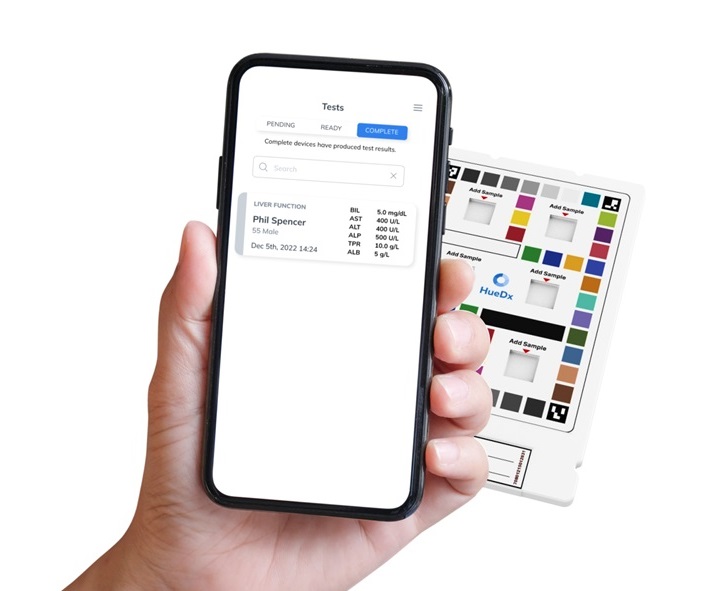
Smartphone-Enabled, Paper-Based Quantitative Diagnostic Platform Transforms POC Testing
Point-of-care diagnostics are crucial for public health, offering rapid, on-site testing that enables prompt diagnosis and treatment. This is especially valuable in remote or underserved regions where... Read moreBusiness
view channel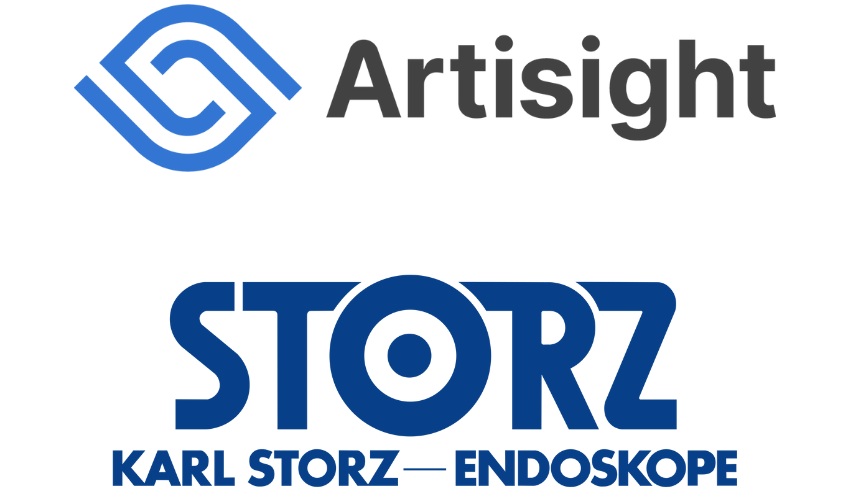
Expanded Collaboration to Transform OR Technology Through AI and Automation
The expansion of an existing collaboration between three leading companies aims to develop artificial intelligence (AI)-driven solutions for smart operating rooms with sophisticated monitoring and automation.... Read more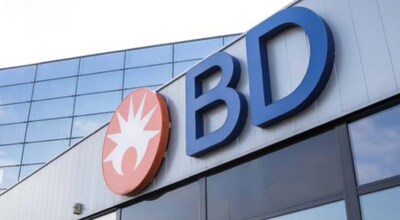