Deep Learning-Based System Detects and Classifies Mammogram Masses
By HospiMedica International staff writers Posted on 16 Aug 2018 |
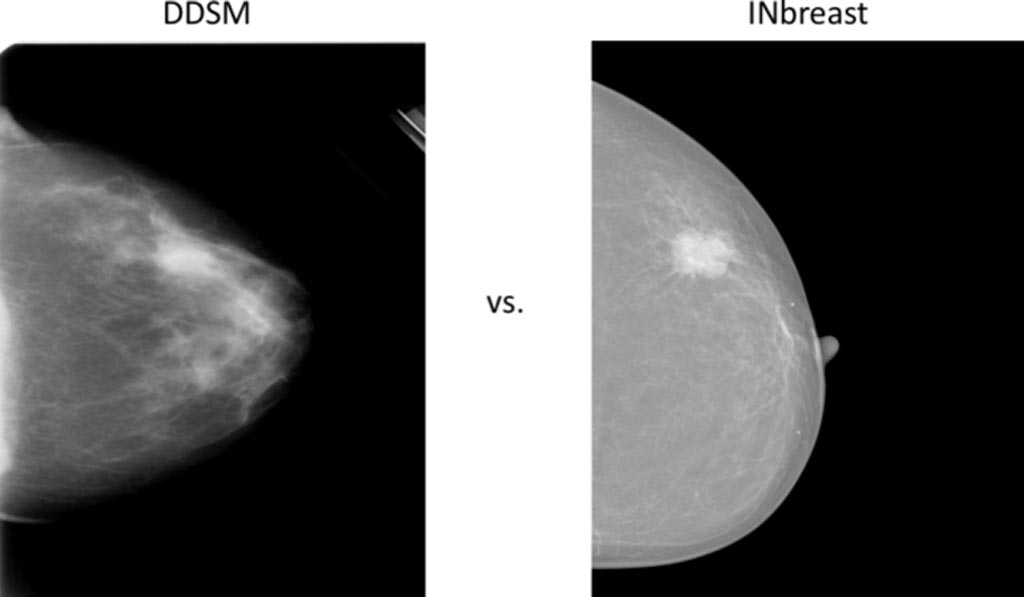
Image: Comparison of two example mammograms from DDSM and INbreast (Photo courtesy of ResearchGate).
Researchers from the Kyung Hee University (Yongin, South Korea) have developed a fully integrated computer-aided diagnosis (CAD) system that uses deep learning and a deep convolutional neural network (CNN) to detect, segment and classify masses from mammograms. In a new study published by the International Journal of Medical Informatics, the researchers have described the use of their regional deep learning model, You-Only-Look-Once (YOLO), to detect breast mass from entire mammograms. The researchers then went on to use a new deep network model based on a full resolution convolutional network (FrCN), to segment the mass lesions pixel-to-pixel. Finally, a deep CNN was used to recognize the mass and classify it as either benign or malignant.
The researchers used the publicly available and annotated INbreast database to evaluate the integrated CAD system’s accuracy in detection, segmentation, and classification. According to the researchers, the evaluation results of the proposed CAD system via four-fold cross-validation tests showed a mass detection accuracy of 98.96%, Matthews correlation coefficient (MCC) of 97.62%, and F1-score of 99.24% with the INbreast dataset. The mass segmentation results via FrCN demonstrated an overall accuracy of 92.97%, MCC of 85.93%, and Dice (F1-score) of 92.69% and Jaccard similarity coefficient metrics of 86.37%, respectively. The detected and segmented masses classified via CNN achieved an overall accuracy of 95.64%, AUC of 94.78%, MCC of 89.91%, and F1-score of 96.84%, respectively.
The researchers concluded that that the CAD system outperformed the latest conventional deep learning methodologies and will assist radiologists in all the stages of detection, segmentation, and classification.
Related Links:
Kyung Hee University
The researchers used the publicly available and annotated INbreast database to evaluate the integrated CAD system’s accuracy in detection, segmentation, and classification. According to the researchers, the evaluation results of the proposed CAD system via four-fold cross-validation tests showed a mass detection accuracy of 98.96%, Matthews correlation coefficient (MCC) of 97.62%, and F1-score of 99.24% with the INbreast dataset. The mass segmentation results via FrCN demonstrated an overall accuracy of 92.97%, MCC of 85.93%, and Dice (F1-score) of 92.69% and Jaccard similarity coefficient metrics of 86.37%, respectively. The detected and segmented masses classified via CNN achieved an overall accuracy of 95.64%, AUC of 94.78%, MCC of 89.91%, and F1-score of 96.84%, respectively.
The researchers concluded that that the CAD system outperformed the latest conventional deep learning methodologies and will assist radiologists in all the stages of detection, segmentation, and classification.
Related Links:
Kyung Hee University
Channels
Artificial Intelligence
view channel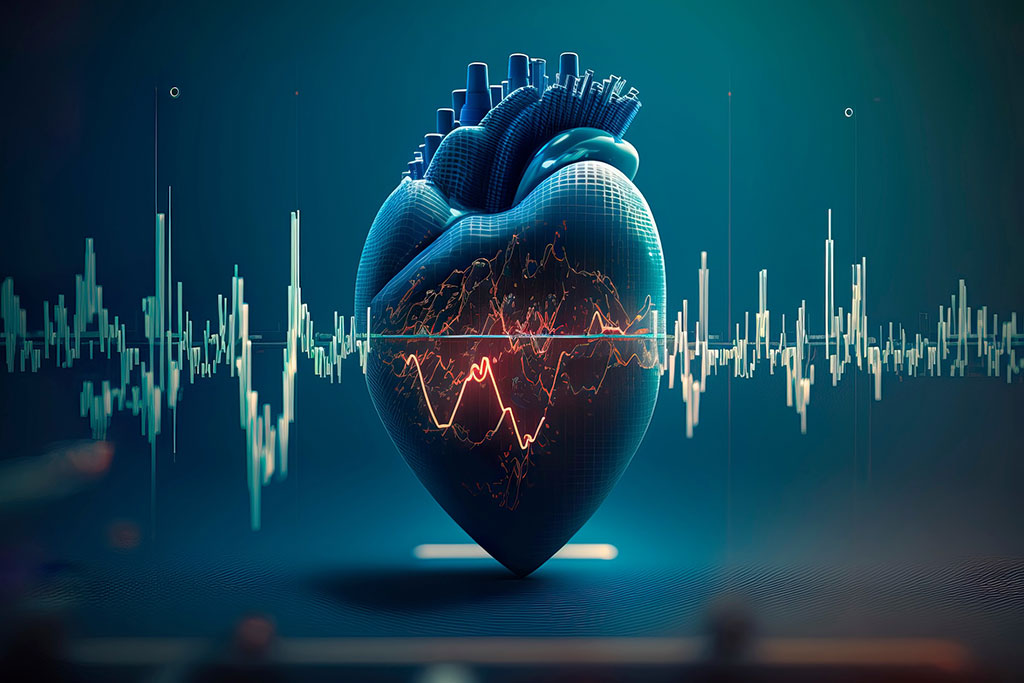
AI-Powered Algorithm to Revolutionize Detection of Atrial Fibrillation
Atrial fibrillation (AFib), a condition characterized by an irregular and often rapid heart rate, is linked to increased risks of stroke and heart failure. This is because the irregular heartbeat in AFib... Read more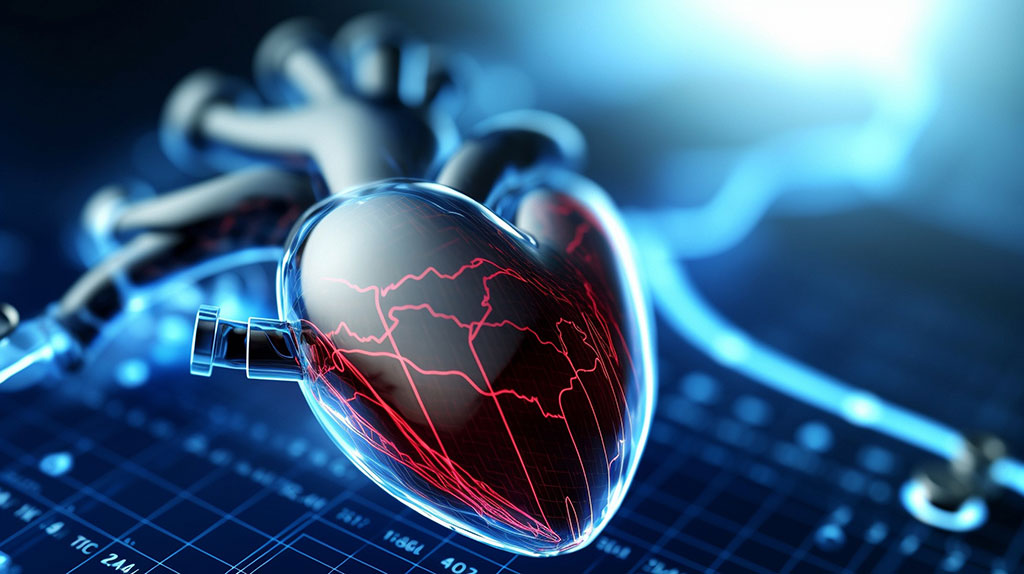
AI Diagnostic Tool Accurately Detects Valvular Disorders Often Missed by Doctors
Doctors generally use stethoscopes to listen for the characteristic lub-dub sounds made by heart valves opening and closing. They also listen for less prominent sounds that indicate problems with these valves.... Read moreCritical Care
view channel.jpeg)
Transcatheter Valve Replacement Outcomes Similar To Surgery, Finds Study
A new study has shown that a minimally invasive procedure for replacing the aortic valve in the heart—known as transcatheter aortic valve replacement (TAVR)—is on par with the more traditional surgical... Read more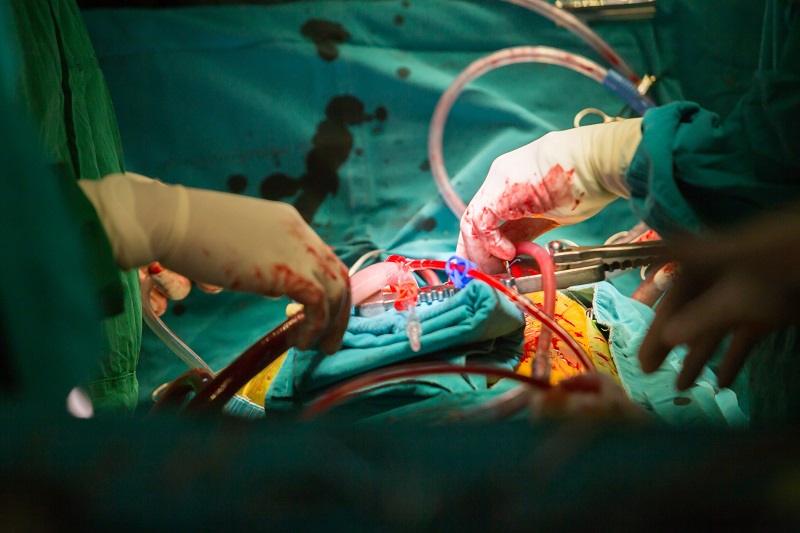
Revascularization Improves Life Quality in Chronic Limb-Threatening Ischemia, Finds Study
Researchers have undertaken a detailed study to evaluate the effects of revascularization strategies on the health-related quality of life (HRQoL) of patients with chronic limb-threatening ischemia.... Read moreSurgical Techniques
view channel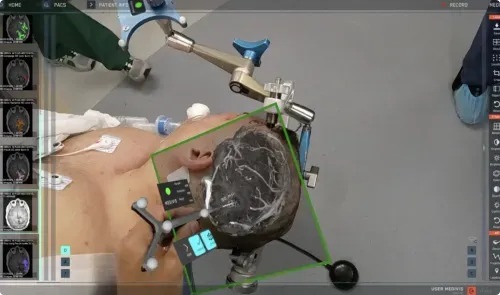
AR Surgical Technology Translates Complex 2D Medical Imaging to Enhance Accuracy
Surgeons often have to switch their focus between a patient’s data displayed on a screen or clipboard and the patient themselves during procedures. But that is about to change. Surgeons can now utilize... Read more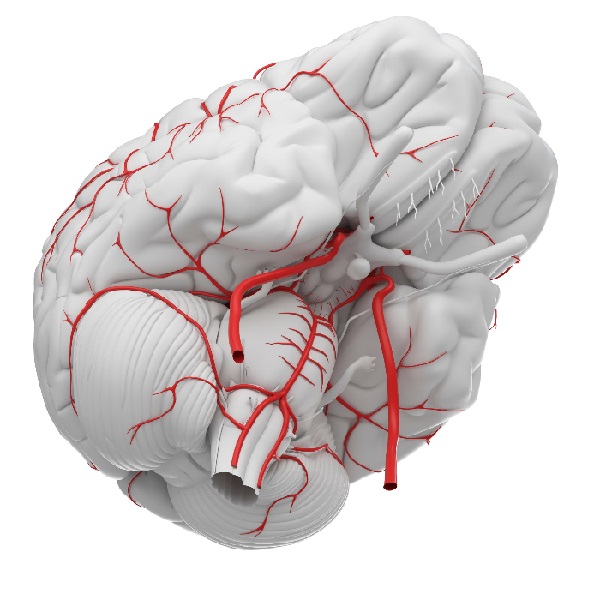
Miniaturized Snake-Like Probe Images Cerebral Arteries From Within
Endovascular interventions are being increasingly favored for treating strokes and cerebral artery diseases, but rely heavily on angiographical imaging that often struggles with limited contrast and spatial... Read morePatient Care
view channel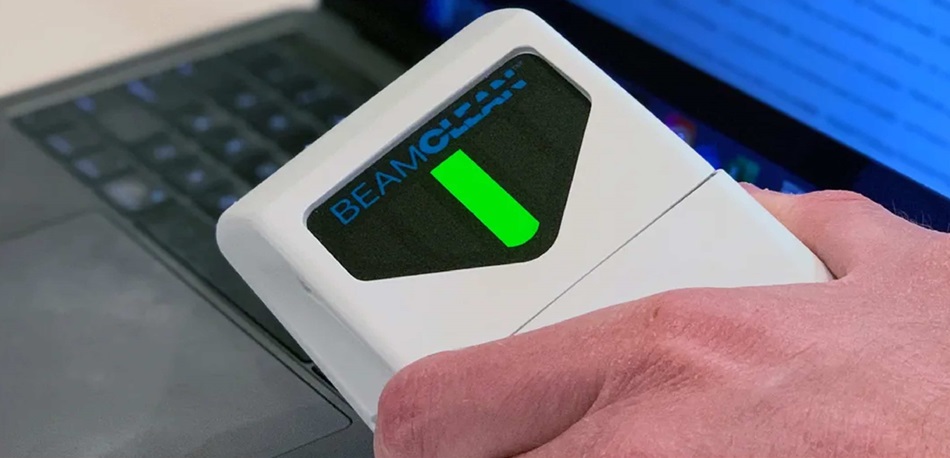
First-Of-Its-Kind Portable Germicidal Light Technology Disinfects High-Touch Clinical Surfaces in Seconds
Reducing healthcare-acquired infections (HAIs) remains a pressing issue within global healthcare systems. In the United States alone, 1.7 million patients contract HAIs annually, leading to approximately... Read more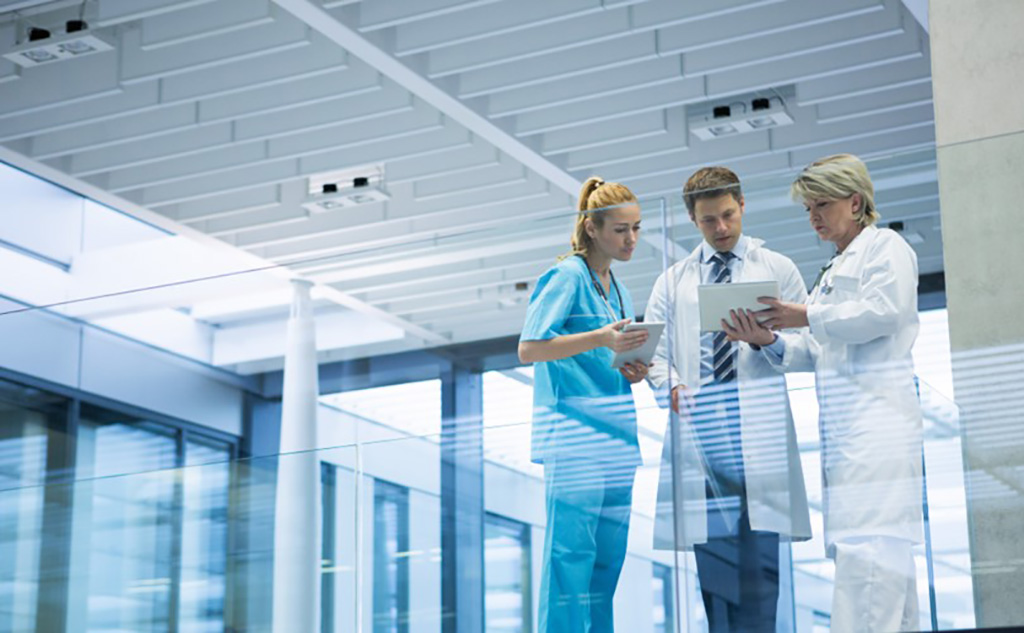
Surgical Capacity Optimization Solution Helps Hospitals Boost OR Utilization
An innovative solution has the capability to transform surgical capacity utilization by targeting the root cause of surgical block time inefficiencies. Fujitsu Limited’s (Tokyo, Japan) Surgical Capacity... Read more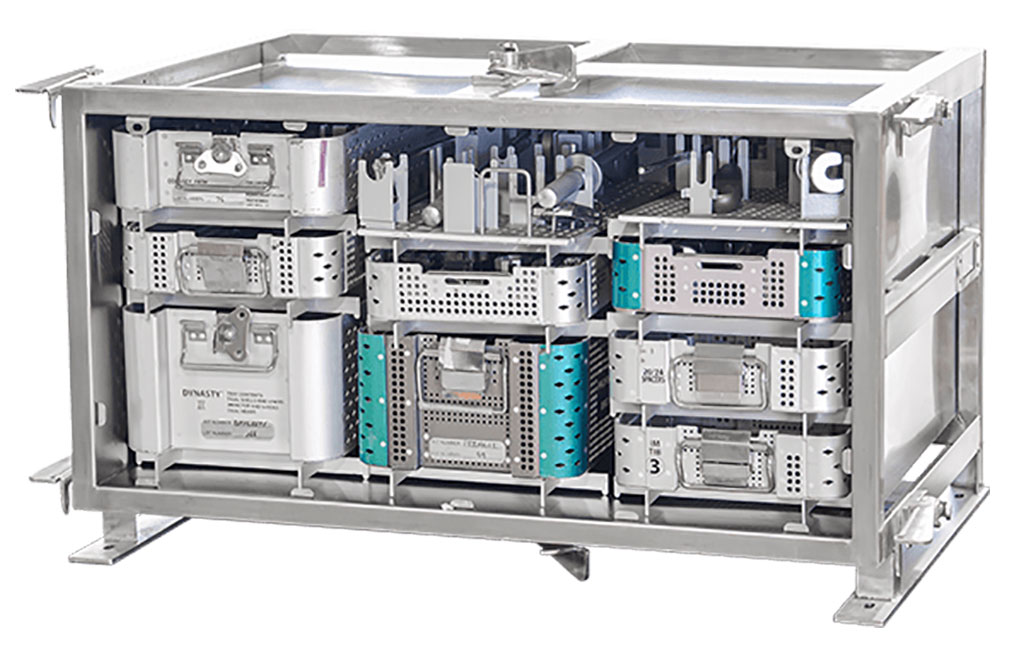
Game-Changing Innovation in Surgical Instrument Sterilization Significantly Improves OR Throughput
A groundbreaking innovation enables hospitals to significantly improve instrument processing time and throughput in operating rooms (ORs) and sterile processing departments. Turbett Surgical, Inc.... Read moreHealth IT
view channel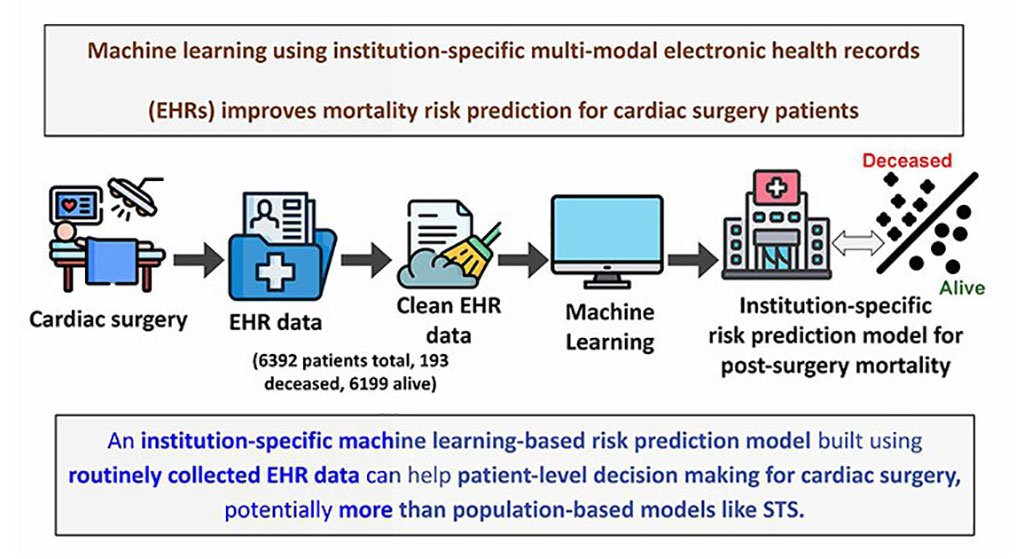
Machine Learning Model Improves Mortality Risk Prediction for Cardiac Surgery Patients
Machine learning algorithms have been deployed to create predictive models in various medical fields, with some demonstrating improved outcomes compared to their standard-of-care counterparts.... Read more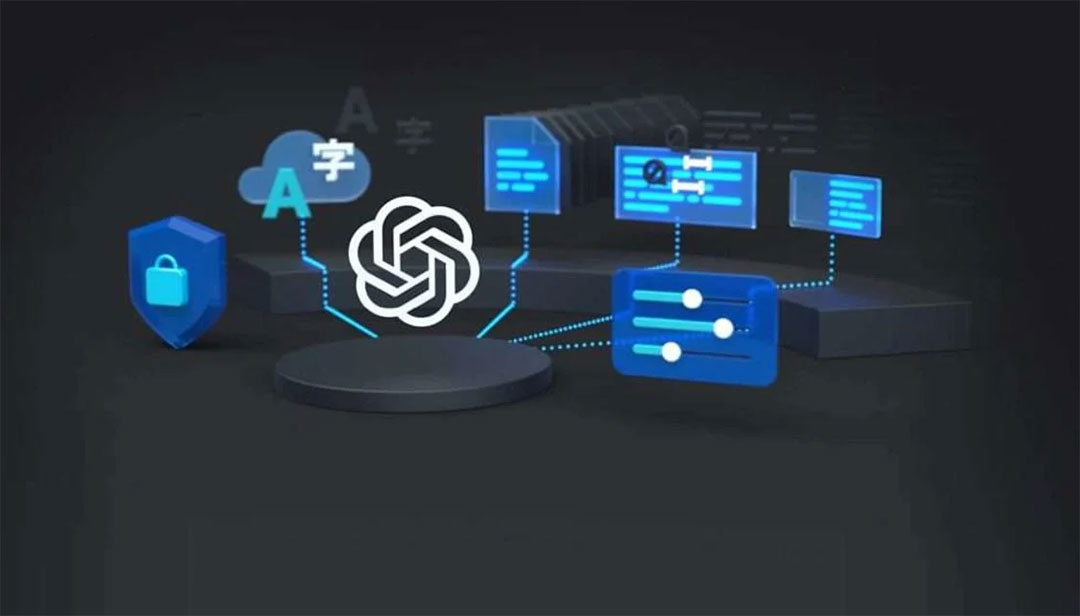
Strategic Collaboration to Develop and Integrate Generative AI into Healthcare
Top industry experts have underscored the immediate requirement for healthcare systems and hospitals to respond to severe cost and margin pressures. Close to half of U.S. hospitals ended 2022 in the red... Read more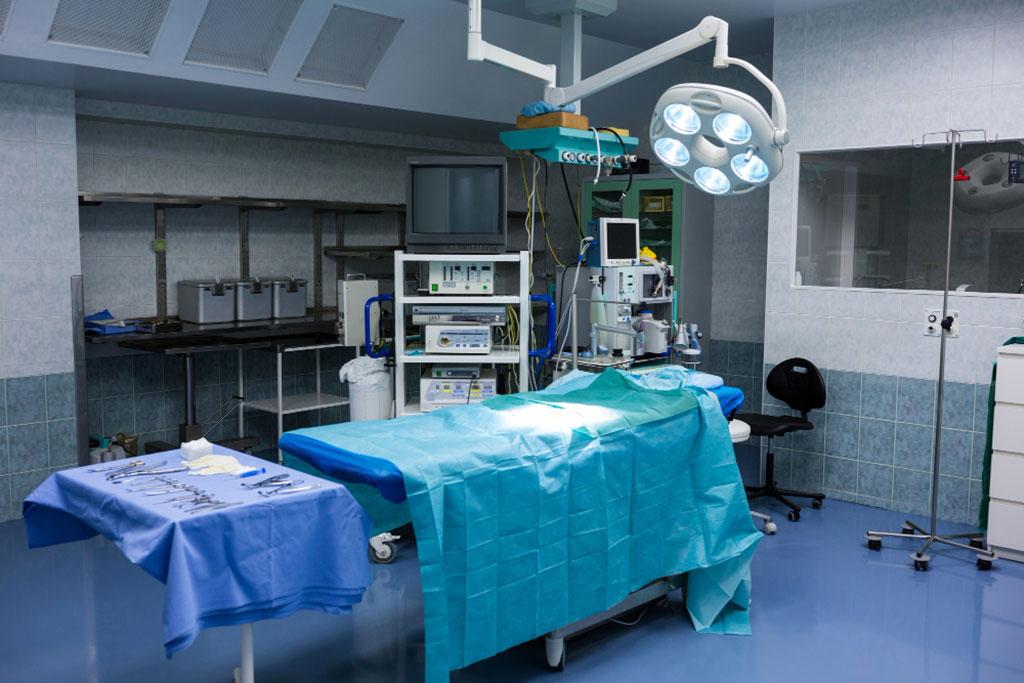
AI-Enabled Operating Rooms Solution Helps Hospitals Maximize Utilization and Unlock Capacity
For healthcare organizations, optimizing operating room (OR) utilization during prime time hours is a complex challenge. Surgeons and clinics face difficulties in finding available slots for booking cases,... Read more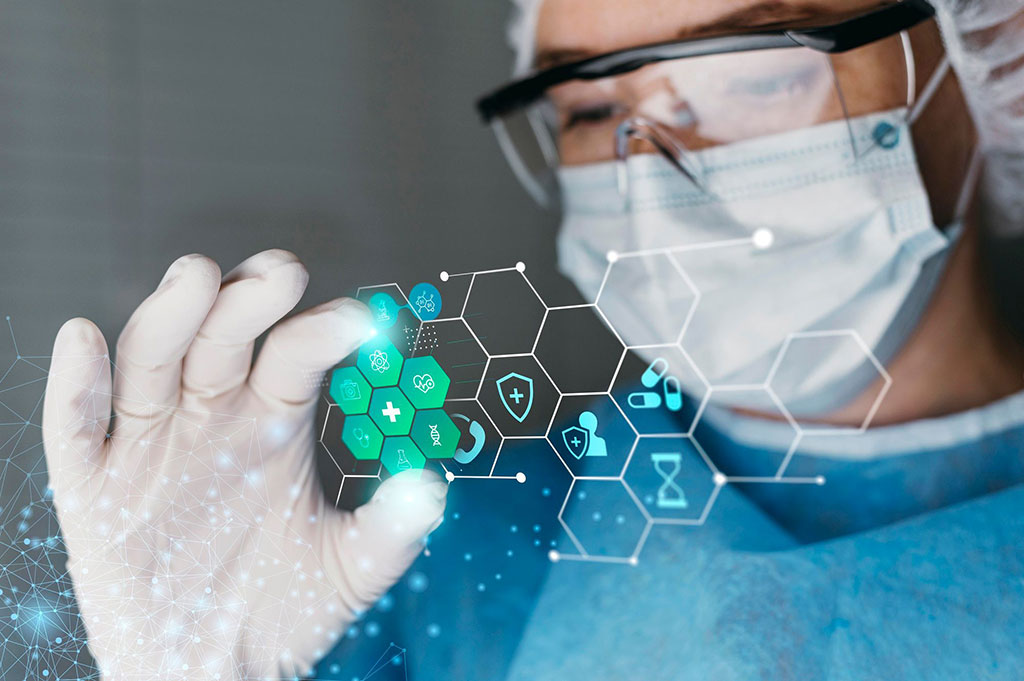
AI Predicts Pancreatic Cancer Three Years before Diagnosis from Patients’ Medical Records
Screening for common cancers like breast, cervix, and prostate cancer relies on relatively simple and highly effective techniques, such as mammograms, Pap smears, and blood tests. These methods have revolutionized... Read morePoint of Care
view channel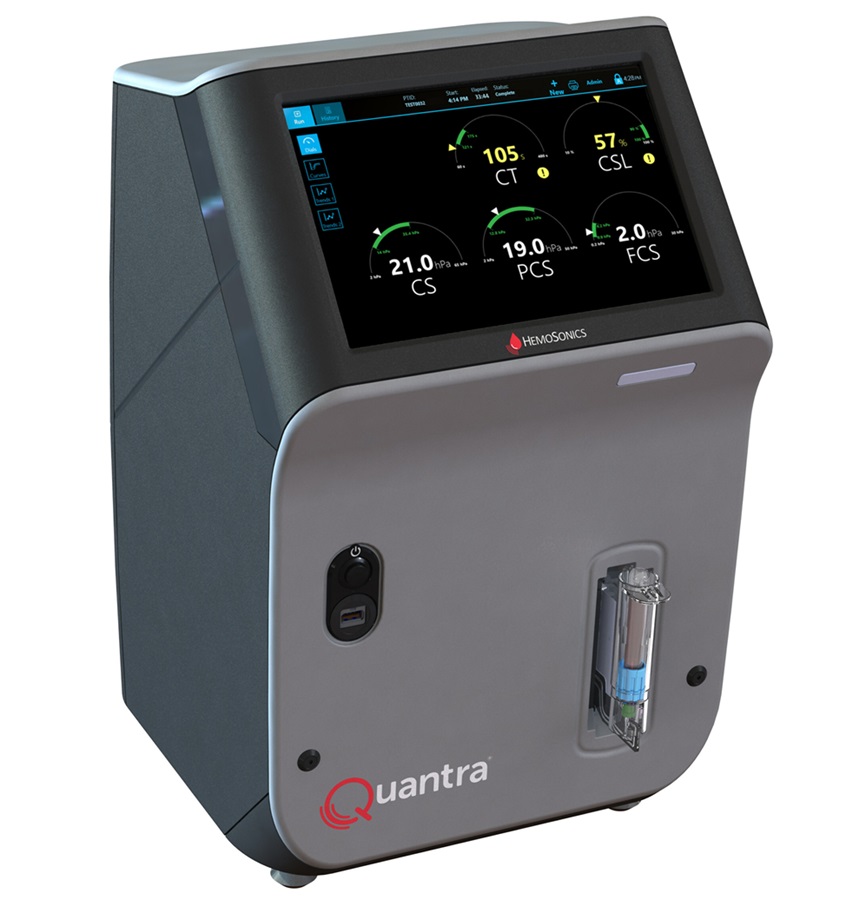
Critical Bleeding Management System to Help Hospitals Further Standardize Viscoelastic Testing
Surgical procedures are often accompanied by significant blood loss and the subsequent high likelihood of the need for allogeneic blood transfusions. These transfusions, while critical, are linked to various... Read more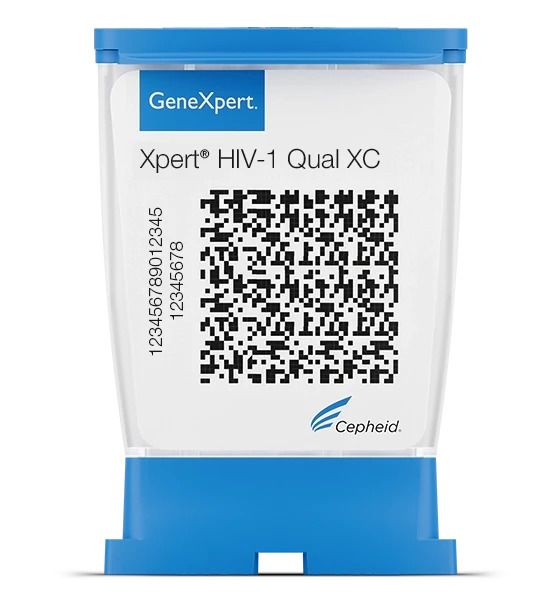
Point of Care HIV Test Enables Early Infection Diagnosis for Infants
Early diagnosis and initiation of treatment are crucial for the survival of infants infected with HIV (human immunodeficiency virus). Without treatment, approximately 50% of infants who acquire HIV during... Read more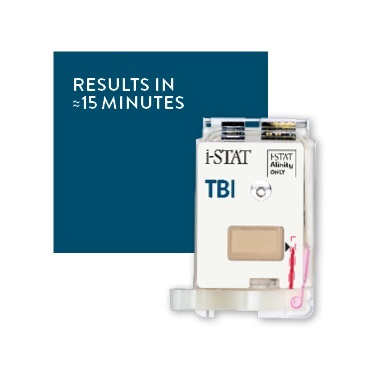
Whole Blood Rapid Test Aids Assessment of Concussion at Patient's Bedside
In the United States annually, approximately five million individuals seek emergency department care for traumatic brain injuries (TBIs), yet over half of those suspecting a concussion may never get it checked.... Read more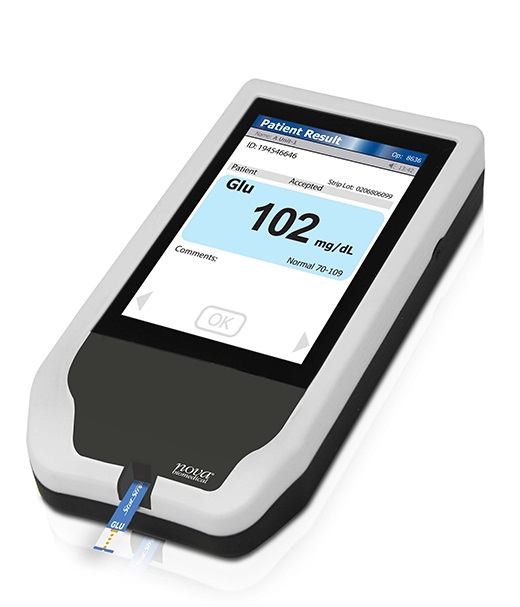
New Generation Glucose Hospital Meter System Ensures Accurate, Interference-Free and Safe Use
A new generation glucose hospital meter system now comes with several features that make hospital glucose testing easier and more secure while continuing to offer accuracy, freedom from interference, and... Read moreBusiness
view channel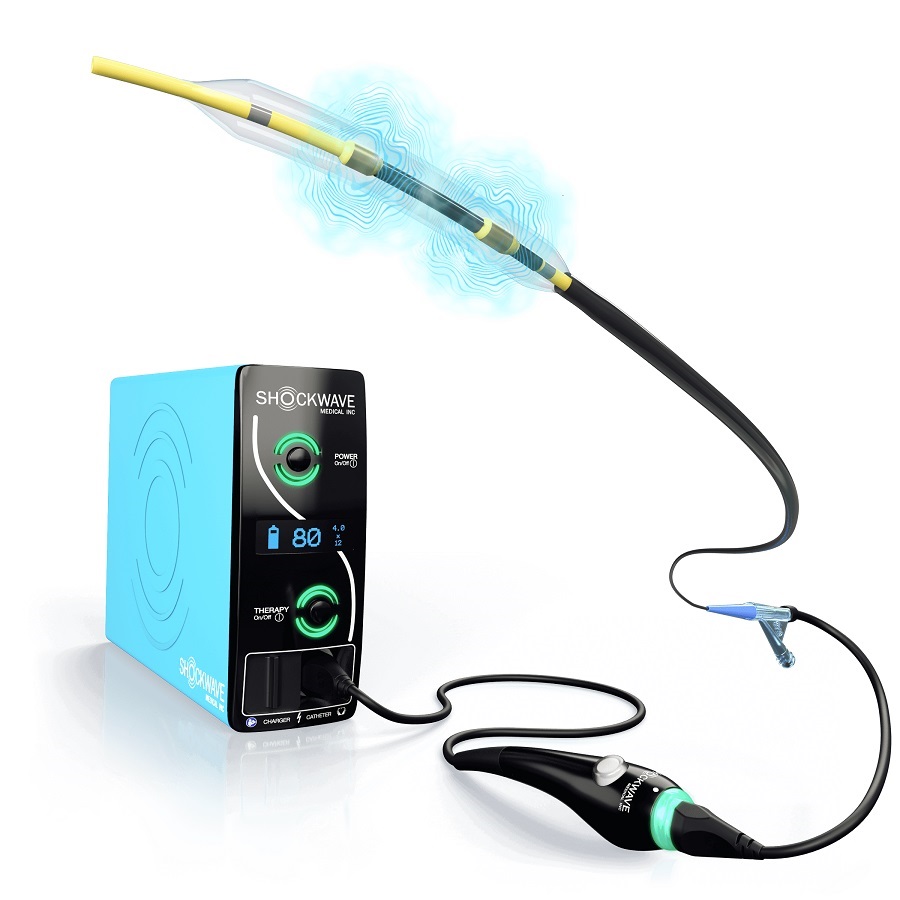
Johnson & Johnson Acquires Cardiovascular Medical Device Company Shockwave Medical
Johnson & Johnson (New Brunswick, N.J., USA) and Shockwave Medical (Santa Clara, CA, USA) have entered into a definitive agreement under which Johnson & Johnson will acquire all of Shockwave’s... Read more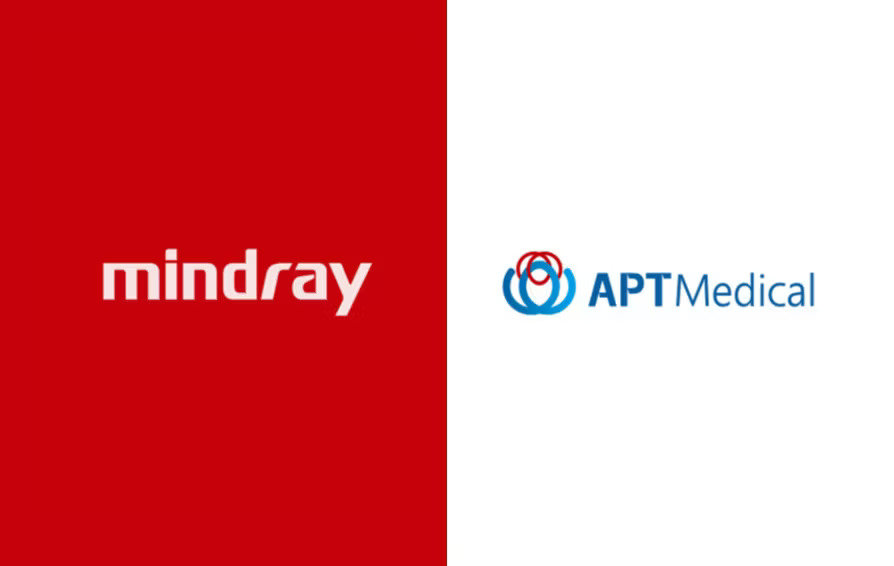