Machine-Learning Scans Accurately Predict Undiagnosed Dementia
By HospiMedica International staff writers Posted on 29 Aug 2018 |
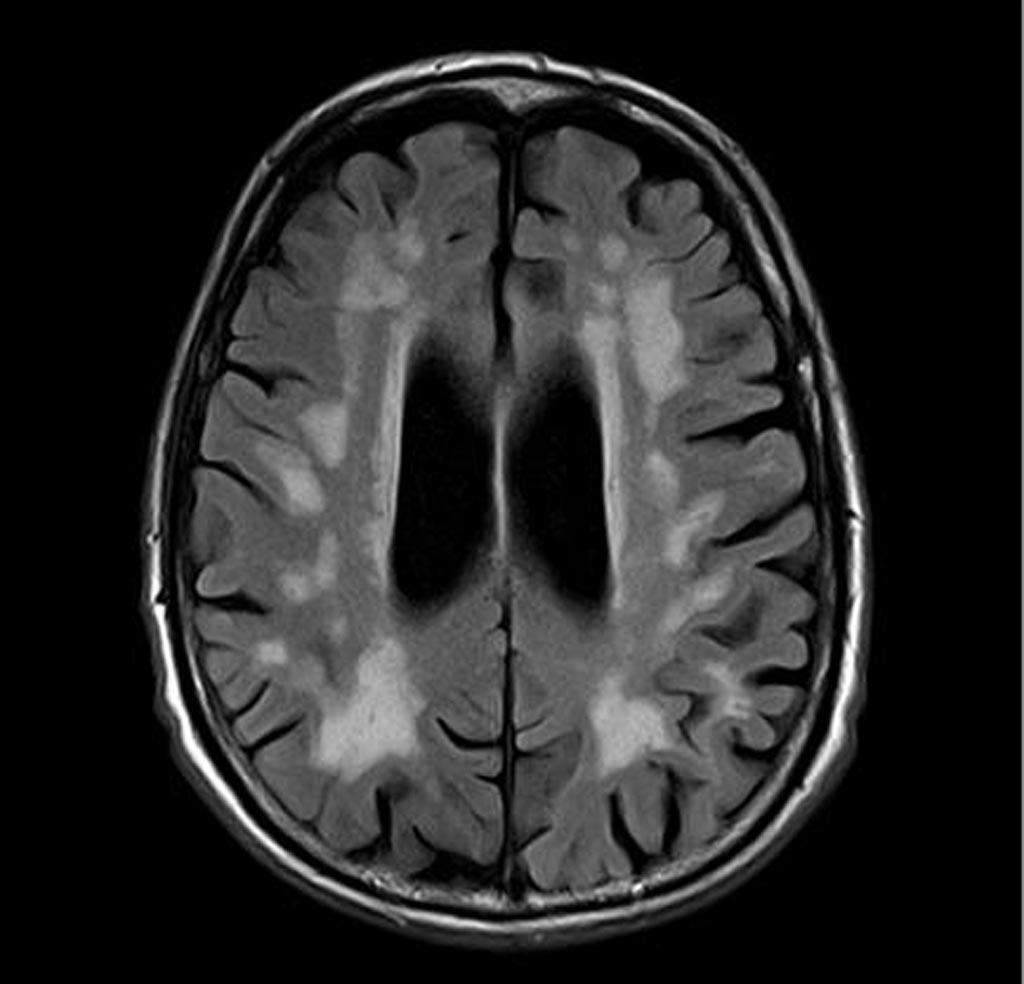
Image: A brain scan showing vascular dementia (Photo courtesy of Radiopaedia).
Researchers from the University of Plymouth (Devon, UK) have developed a new machine-learning model that scanned routinely collected National Health Service {(NHS) England, UK)} data in a study and predicted undiagnosed dementia in primary care with high accuracy.
For the study, the researchers used Read-encoded data from 18 consenting GP surgeries across Devon, UK, for 26,483 patients aged over 65 years. The Read codes is a thesaurus of clinical terms used to summarize clinical and administrative data for UK GPs and were assessed on whether they can contribute to dementia risk, along with other factors such as weight and blood pressure. The researchers used the codes to train the machine-learning classification model to identify patients with possible underlying dementia. The study found that the model can detect people with underlying dementia with an accuracy of 84%, suggesting that it could significantly reduce the number of people living with undiagnosed dementia from the current estimated figure of 50% to 8%.
“Machine learning is an application of artificial intelligence where systems automatically learn and improve from experience without being explicitly programmed,” said Emmanuel Ifeachor, Principal Investigator Professor from the School of Computing Electronics and Mathematics at the University of Plymouth. “It’s already being used for many applications throughout healthcare such as medical imaging, but using it for patient data has not been done in quite this way before. The methodology is promising and, if successfully developed and deployed, may help to increase dementia diagnosis in primary care.”
“Dementia is a disease with so many different contributing factors, and it can be quite difficult to pinpoint or predict,” said Dr. Camille Carroll, Consultant Neurologist at University Hospitals Plymouth NHS Trust and Researcher in the Institute of Translational and Stratified Medicine at the University of Plymouth. “There is strong epidemiological evidence that a number of cardiovascular and lifestyle factors such as hypertension; high cholesterol; diabetes; obesity; stroke; atrial fibrillation; smoking; and reduced cognitive, physical, or social activities can predict the risk of dementia in later life, but no studies have taken place that allow us to see this quickly. So having tools that can take a vast amount of data, and automatically identify patients with possible dementia, to facilitate targeted screening, could potentially be very useful and help improve diagnosis rates.”
Related Links:
University of Plymouth
National Health Service
For the study, the researchers used Read-encoded data from 18 consenting GP surgeries across Devon, UK, for 26,483 patients aged over 65 years. The Read codes is a thesaurus of clinical terms used to summarize clinical and administrative data for UK GPs and were assessed on whether they can contribute to dementia risk, along with other factors such as weight and blood pressure. The researchers used the codes to train the machine-learning classification model to identify patients with possible underlying dementia. The study found that the model can detect people with underlying dementia with an accuracy of 84%, suggesting that it could significantly reduce the number of people living with undiagnosed dementia from the current estimated figure of 50% to 8%.
“Machine learning is an application of artificial intelligence where systems automatically learn and improve from experience without being explicitly programmed,” said Emmanuel Ifeachor, Principal Investigator Professor from the School of Computing Electronics and Mathematics at the University of Plymouth. “It’s already being used for many applications throughout healthcare such as medical imaging, but using it for patient data has not been done in quite this way before. The methodology is promising and, if successfully developed and deployed, may help to increase dementia diagnosis in primary care.”
“Dementia is a disease with so many different contributing factors, and it can be quite difficult to pinpoint or predict,” said Dr. Camille Carroll, Consultant Neurologist at University Hospitals Plymouth NHS Trust and Researcher in the Institute of Translational and Stratified Medicine at the University of Plymouth. “There is strong epidemiological evidence that a number of cardiovascular and lifestyle factors such as hypertension; high cholesterol; diabetes; obesity; stroke; atrial fibrillation; smoking; and reduced cognitive, physical, or social activities can predict the risk of dementia in later life, but no studies have taken place that allow us to see this quickly. So having tools that can take a vast amount of data, and automatically identify patients with possible dementia, to facilitate targeted screening, could potentially be very useful and help improve diagnosis rates.”
Related Links:
University of Plymouth
National Health Service
Channels
Artificial Intelligence
view channel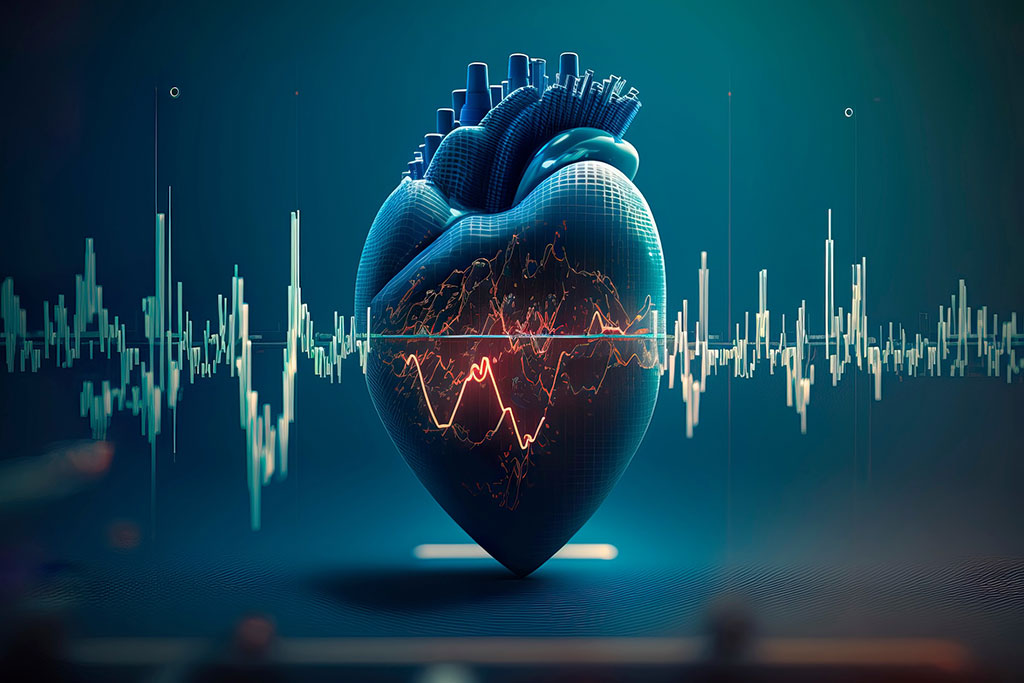
AI-Powered Algorithm to Revolutionize Detection of Atrial Fibrillation
Atrial fibrillation (AFib), a condition characterized by an irregular and often rapid heart rate, is linked to increased risks of stroke and heart failure. This is because the irregular heartbeat in AFib... Read more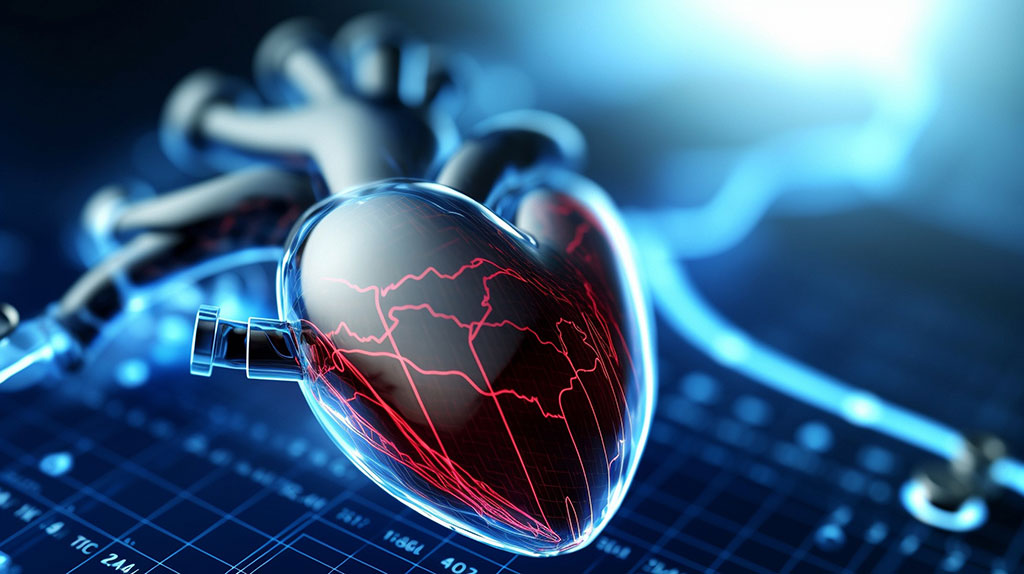
AI Diagnostic Tool Accurately Detects Valvular Disorders Often Missed by Doctors
Doctors generally use stethoscopes to listen for the characteristic lub-dub sounds made by heart valves opening and closing. They also listen for less prominent sounds that indicate problems with these valves.... Read moreCritical Care
view channel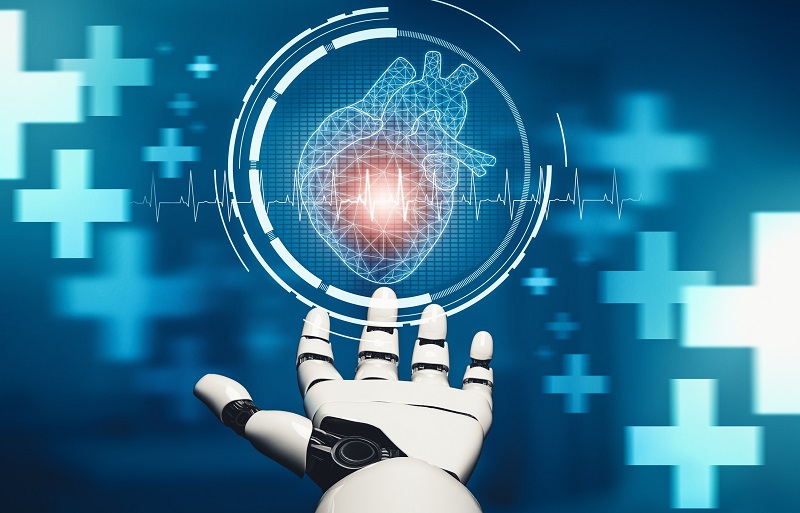
Powerful AI Risk Assessment Tool Predicts Outcomes in Heart Failure Patients
Heart failure is a serious condition where the heart cannot pump sufficient blood to meet the body's needs, leading to symptoms like fatigue, weakness, and swelling in the legs and feet, and it can ultimately... Read more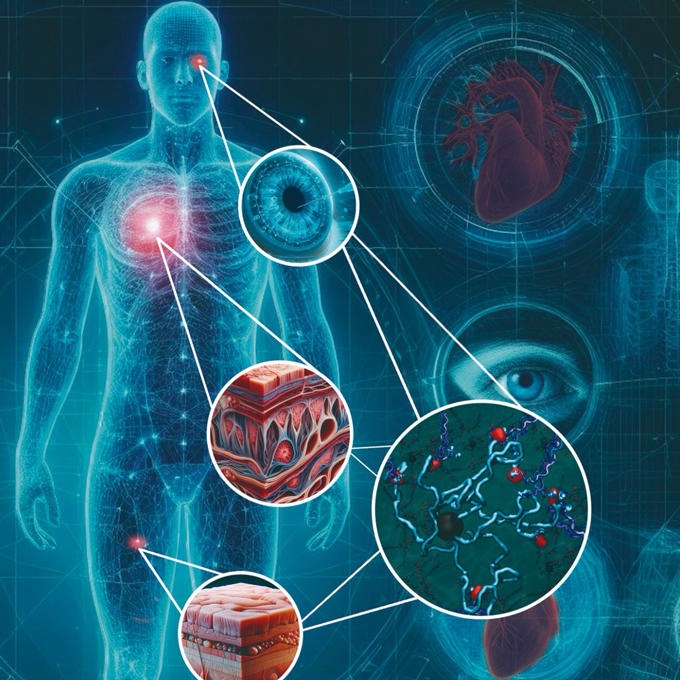
Peptide-Based Hydrogels Repair Damaged Organs and Tissues On-The-Spot
Scientists have ingeniously combined biomedical expertise with nature-inspired engineering to develop a jelly-like material that holds significant promise for immediate repairs to a wide variety of damaged... Read more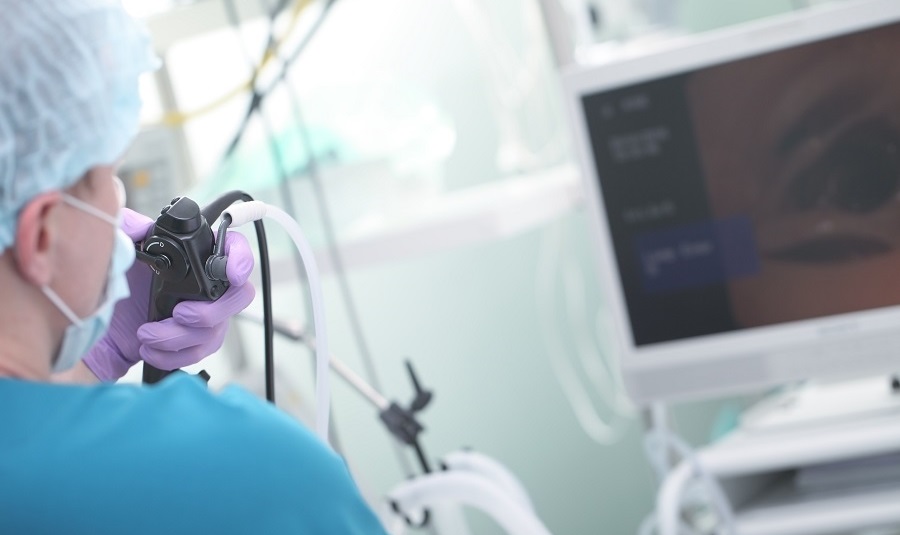
One-Hour Endoscopic Procedure Could Eliminate Need for Insulin for Type 2 Diabetes
Over 37 million Americans are diagnosed with diabetes, and more than 90% of these cases are Type 2 diabetes. This form of diabetes is most commonly seen in individuals over 45, though an increasing number... Read moreSurgical Techniques
view channel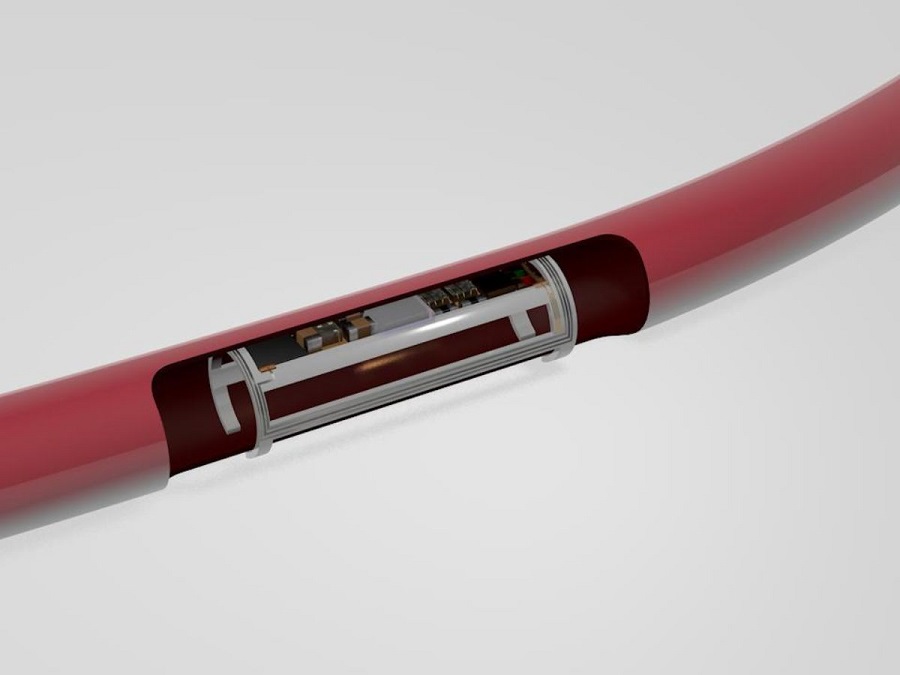
Miniaturized Implantable Multi-Sensors Device to Monitor Vessels Health
Researchers have embarked on a project to develop a multi-sensing device that can be implanted into blood vessels like peripheral veins or arteries to monitor a range of bodily parameters and overall health status.... Read more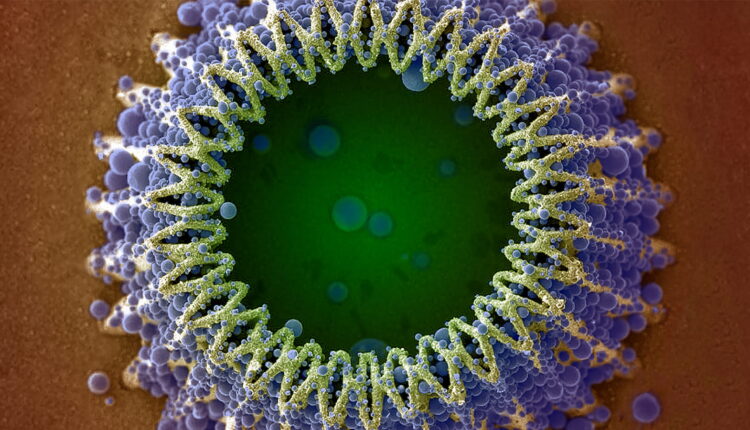
Tiny Robots Made Out Of Carbon Could Conduct Colonoscopy, Pelvic Exam or Blood Test
Researchers at the University of Alberta (Edmonton, AB, Canada) are developing cutting-edge robots so tiny that they are invisible to the naked eye but are capable of traveling through the human body to... Read more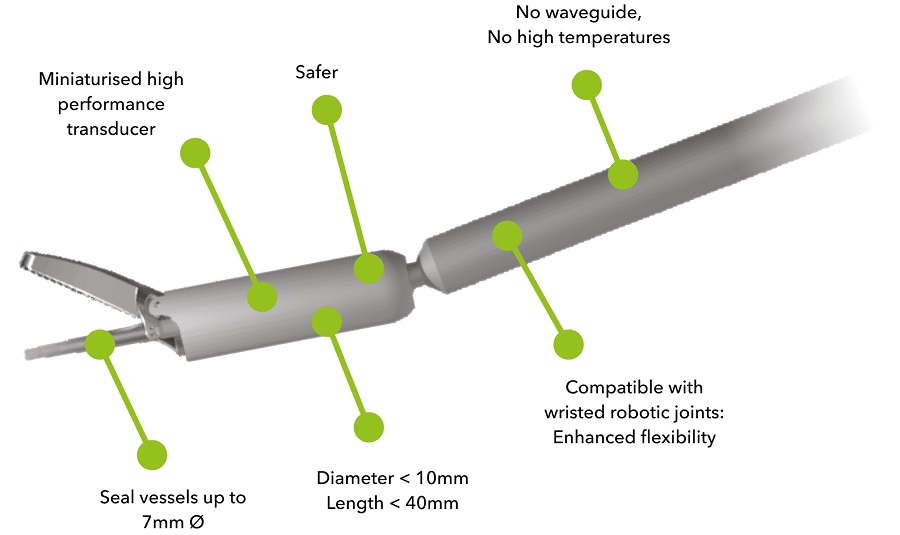
Miniaturized Ultrasonic Scalpel Enables Faster and Safer Robotic-Assisted Surgery
Robot-assisted surgery (RAS) has gained significant popularity in recent years and is now extensively used across various surgical fields such as urology, gynecology, and cardiology. These surgeries, performed... Read morePatient Care
view channel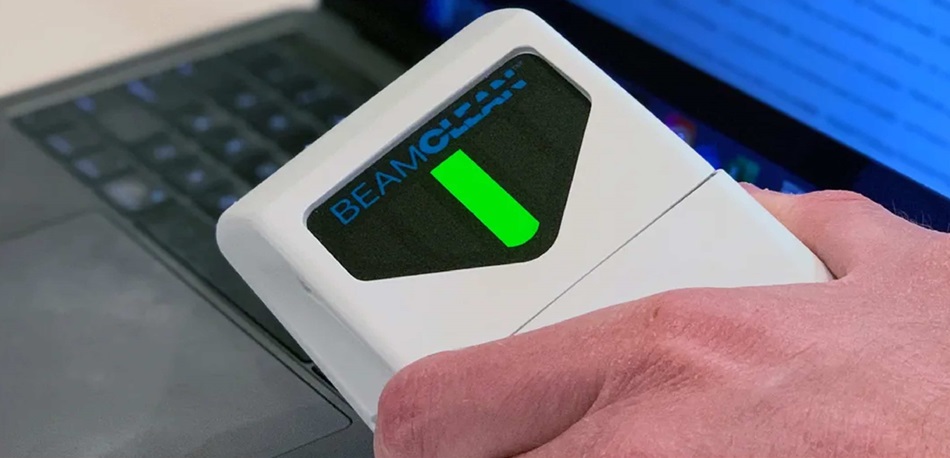
First-Of-Its-Kind Portable Germicidal Light Technology Disinfects High-Touch Clinical Surfaces in Seconds
Reducing healthcare-acquired infections (HAIs) remains a pressing issue within global healthcare systems. In the United States alone, 1.7 million patients contract HAIs annually, leading to approximately... Read more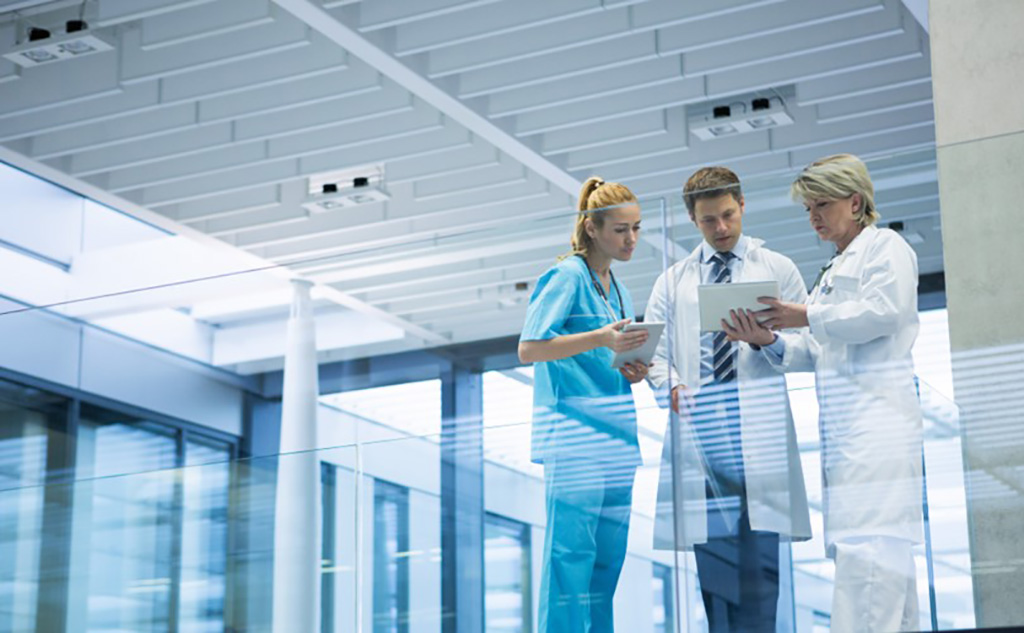
Surgical Capacity Optimization Solution Helps Hospitals Boost OR Utilization
An innovative solution has the capability to transform surgical capacity utilization by targeting the root cause of surgical block time inefficiencies. Fujitsu Limited’s (Tokyo, Japan) Surgical Capacity... Read more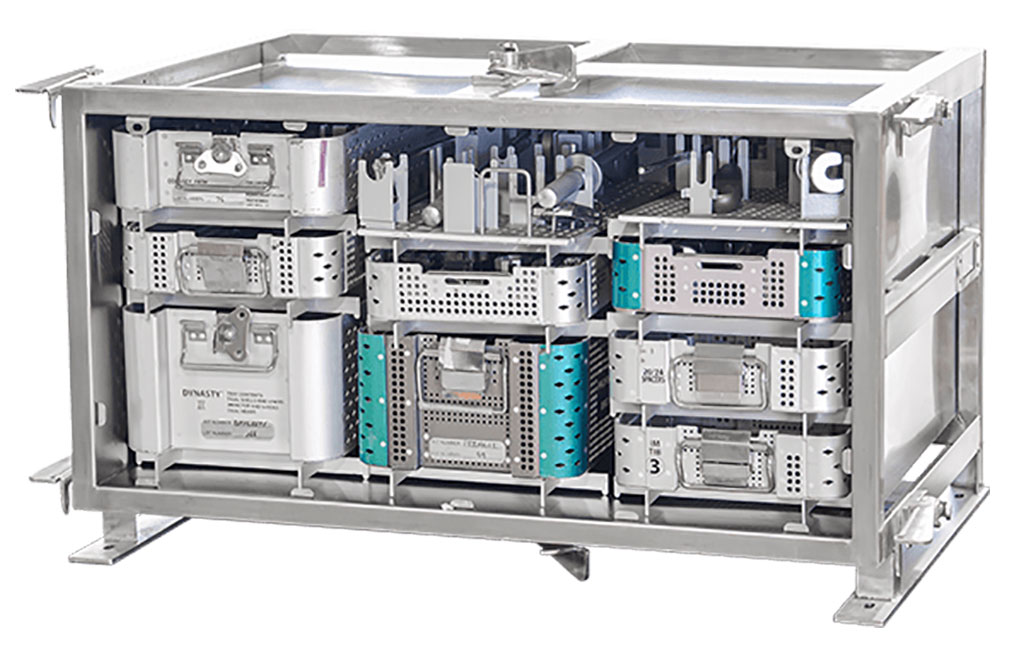
Game-Changing Innovation in Surgical Instrument Sterilization Significantly Improves OR Throughput
A groundbreaking innovation enables hospitals to significantly improve instrument processing time and throughput in operating rooms (ORs) and sterile processing departments. Turbett Surgical, Inc.... Read moreHealth IT
view channel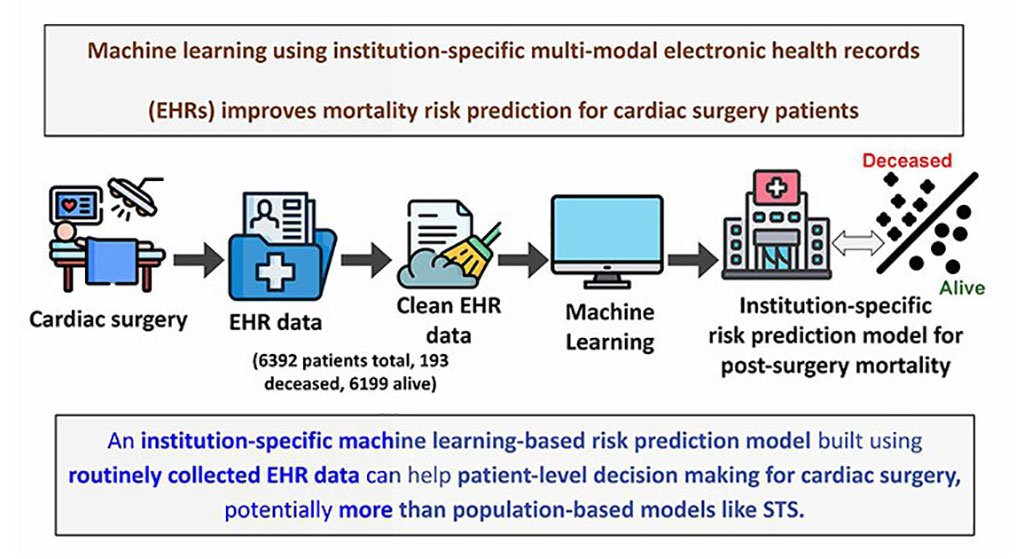
Machine Learning Model Improves Mortality Risk Prediction for Cardiac Surgery Patients
Machine learning algorithms have been deployed to create predictive models in various medical fields, with some demonstrating improved outcomes compared to their standard-of-care counterparts.... Read more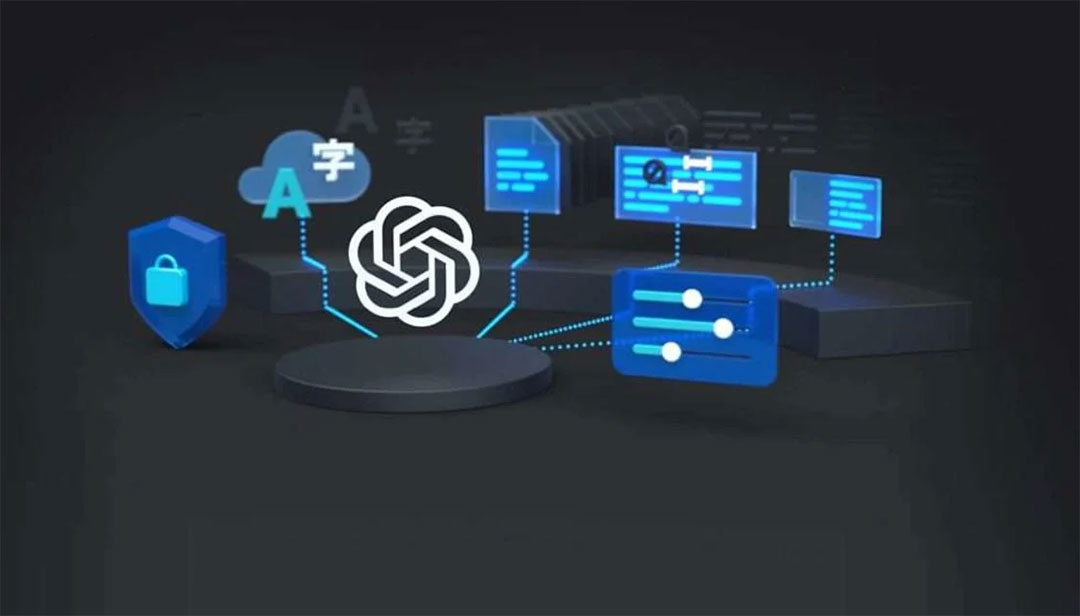
Strategic Collaboration to Develop and Integrate Generative AI into Healthcare
Top industry experts have underscored the immediate requirement for healthcare systems and hospitals to respond to severe cost and margin pressures. Close to half of U.S. hospitals ended 2022 in the red... Read more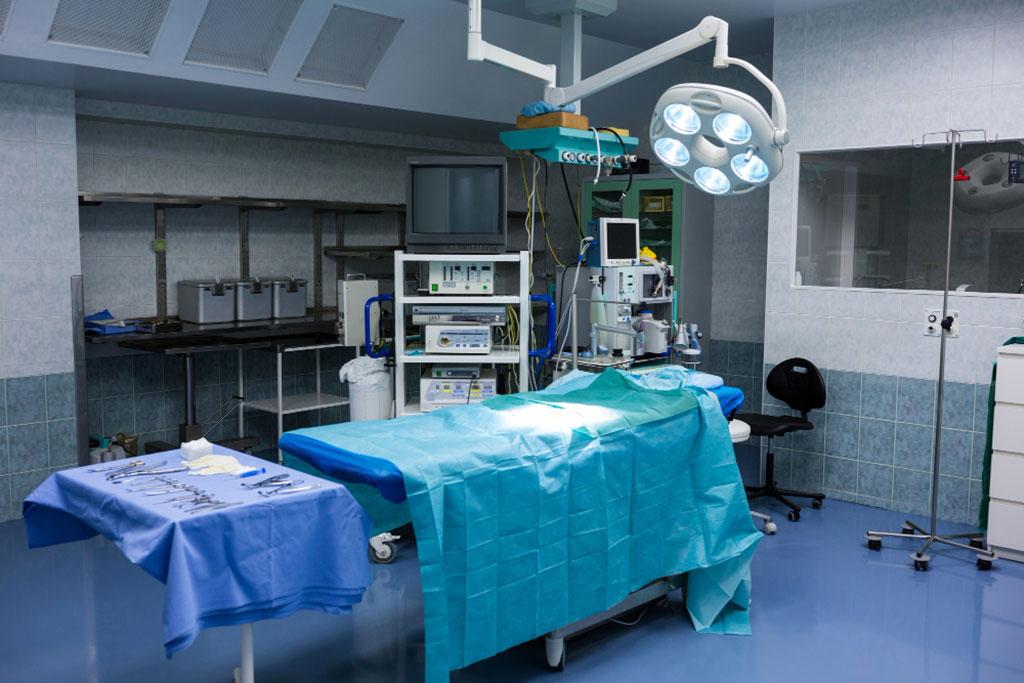
AI-Enabled Operating Rooms Solution Helps Hospitals Maximize Utilization and Unlock Capacity
For healthcare organizations, optimizing operating room (OR) utilization during prime time hours is a complex challenge. Surgeons and clinics face difficulties in finding available slots for booking cases,... Read more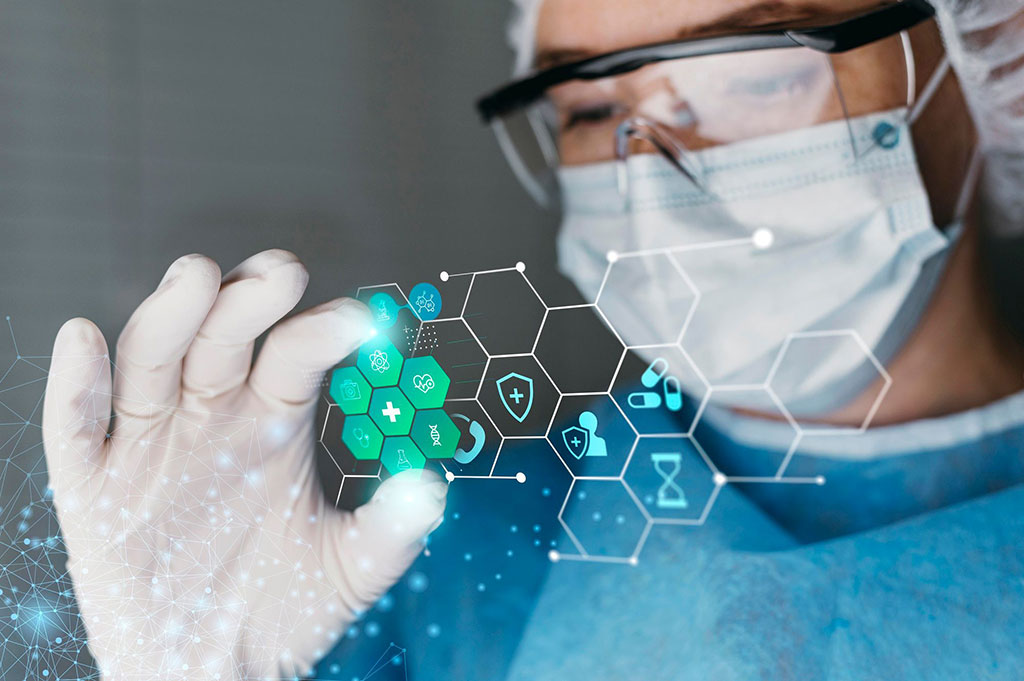
AI Predicts Pancreatic Cancer Three Years before Diagnosis from Patients’ Medical Records
Screening for common cancers like breast, cervix, and prostate cancer relies on relatively simple and highly effective techniques, such as mammograms, Pap smears, and blood tests. These methods have revolutionized... Read morePoint of Care
view channel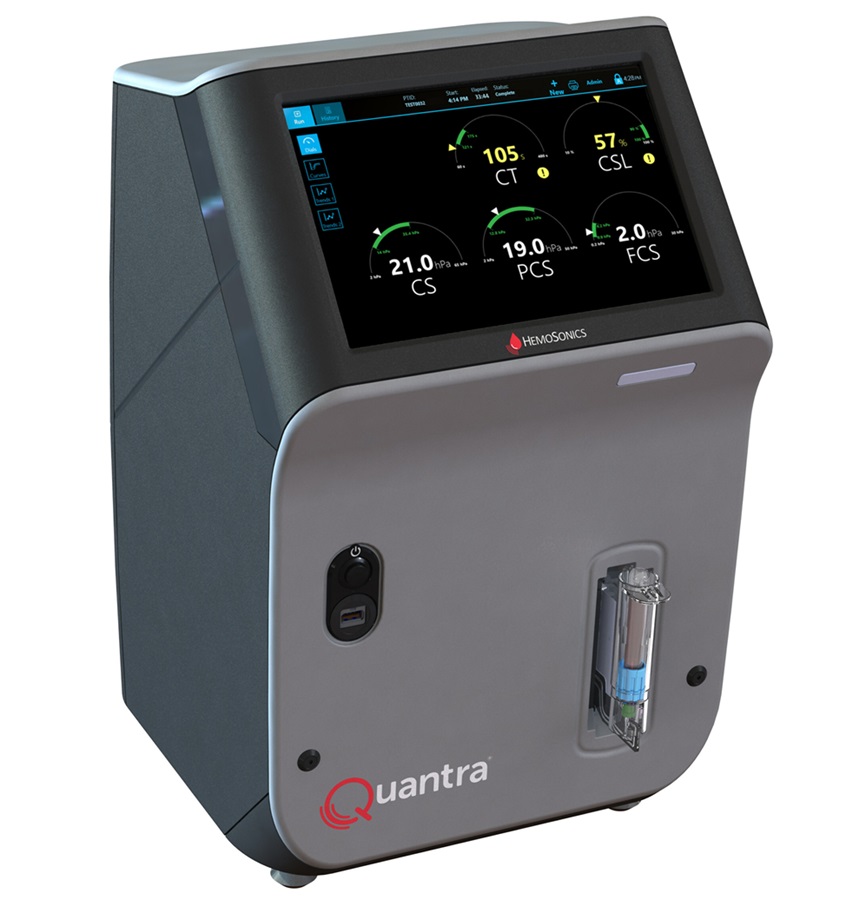
Critical Bleeding Management System to Help Hospitals Further Standardize Viscoelastic Testing
Surgical procedures are often accompanied by significant blood loss and the subsequent high likelihood of the need for allogeneic blood transfusions. These transfusions, while critical, are linked to various... Read more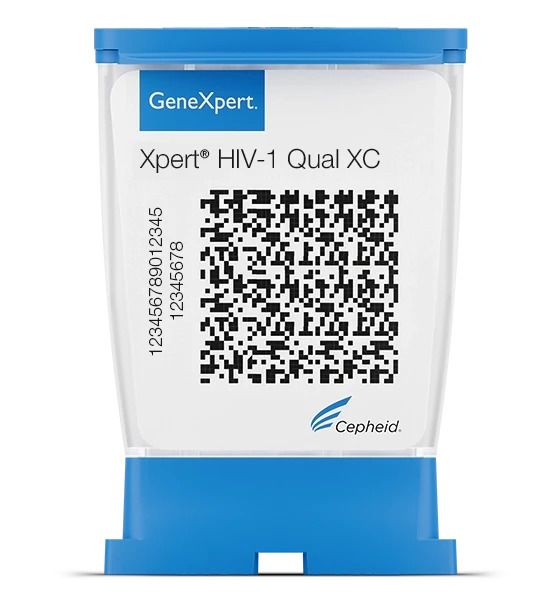
Point of Care HIV Test Enables Early Infection Diagnosis for Infants
Early diagnosis and initiation of treatment are crucial for the survival of infants infected with HIV (human immunodeficiency virus). Without treatment, approximately 50% of infants who acquire HIV during... Read more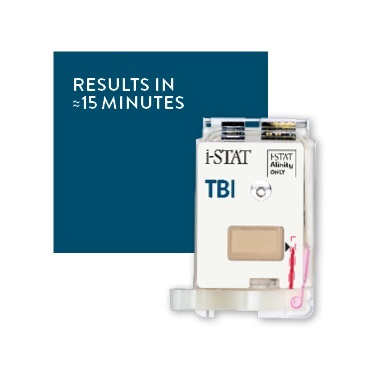
Whole Blood Rapid Test Aids Assessment of Concussion at Patient's Bedside
In the United States annually, approximately five million individuals seek emergency department care for traumatic brain injuries (TBIs), yet over half of those suspecting a concussion may never get it checked.... Read more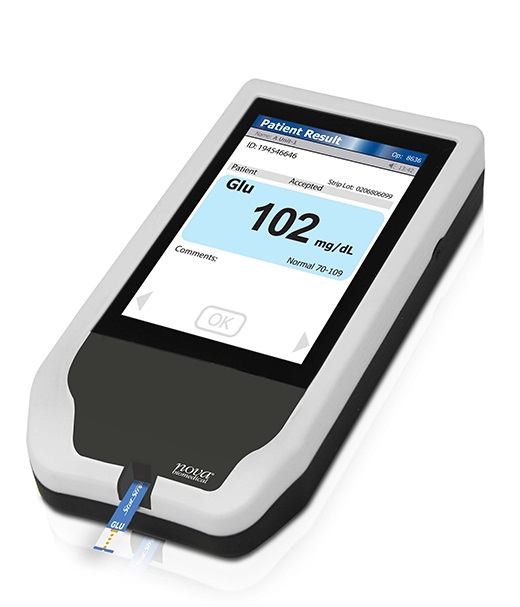
New Generation Glucose Hospital Meter System Ensures Accurate, Interference-Free and Safe Use
A new generation glucose hospital meter system now comes with several features that make hospital glucose testing easier and more secure while continuing to offer accuracy, freedom from interference, and... Read moreBusiness
view channel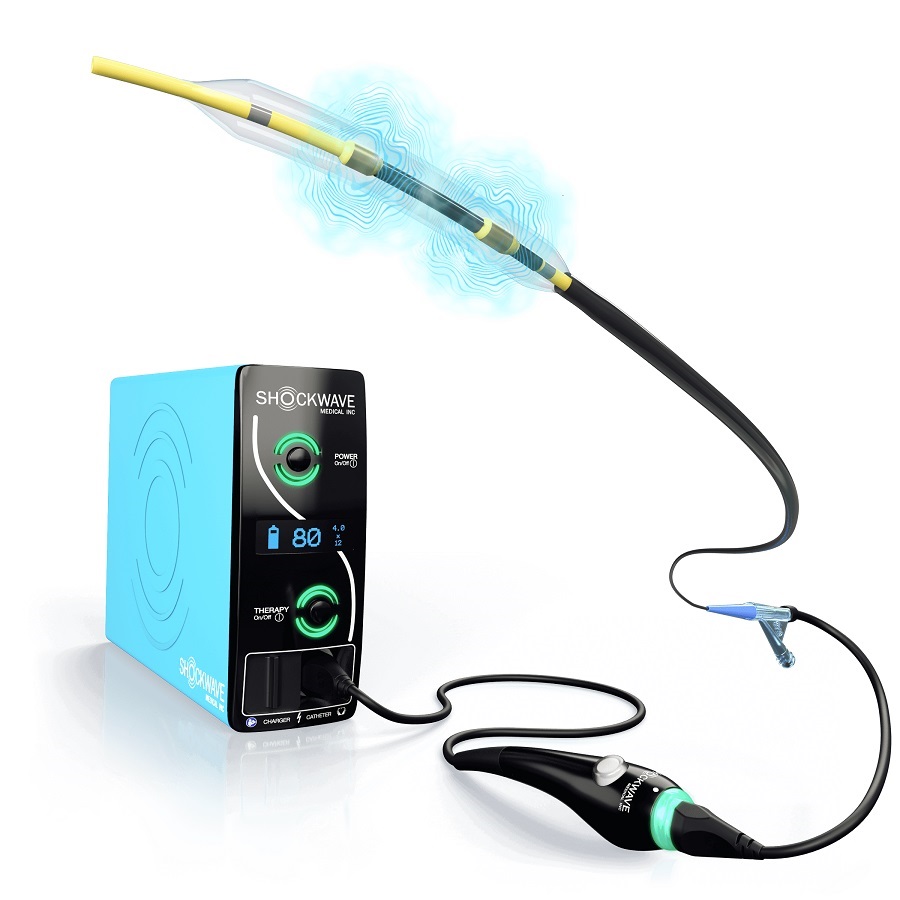
Johnson & Johnson Acquires Cardiovascular Medical Device Company Shockwave Medical
Johnson & Johnson (New Brunswick, N.J., USA) and Shockwave Medical (Santa Clara, CA, USA) have entered into a definitive agreement under which Johnson & Johnson will acquire all of Shockwave’s... Read more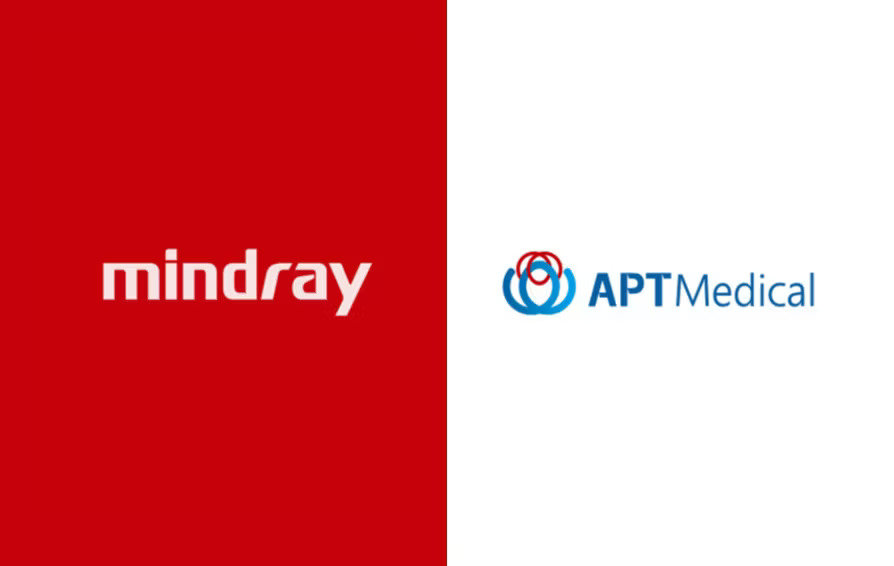