Scientists Use Machine Learning Algorithm to Identify Six Types of COVID-19 with Distinctive Symptoms
By HospiMedica International staff writers Posted on 20 Jul 2020 |
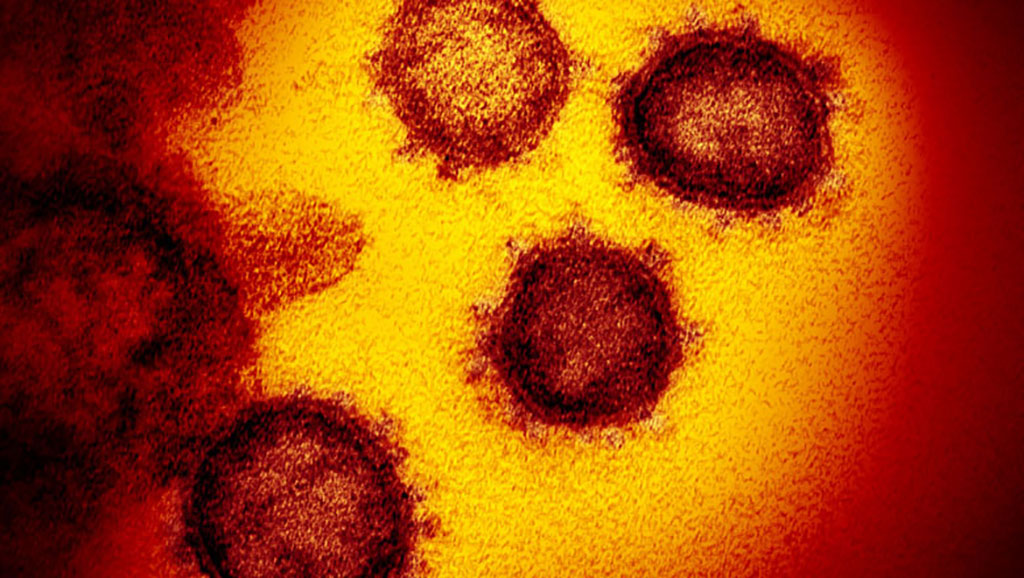
Image: SARS-CoV-2 (Photo courtesy of NIAID)
An analysis of data from the COVID Symptom Study app has revealed that there are six distinct ‘types’ of COVID-19, each distinguished by a particular cluster of symptoms and differing in the severity of the disease as well as need for respiratory support during hospitalization.
The findings have major implications for clinical management of COVID-19, and could help doctors predict who is most at risk and likely to need hospital care in a second wave of coronavirus infections. Although continuous cough, fever and loss of smell (anosmia) are usually highlighted as the three key symptoms of COVID-19, data gathered from app users shows that people can experience a wide range of different symptoms including headaches, muscle pains, fatigue, diarrhea, confusion, loss of appetite, shortness of breath and more. The progression and outcomes also vary significantly between people, ranging from mild flu-like symptoms or a simple rash to severe or fatal disease.
To find out whether particular symptoms tend to appear together and how this related to the progression of the disease, the research team at King’s College London (London, UK) used a machine learning algorithm to analyze data from a subset of around 1,600 users in the UK and US with confirmed COVID-19 who had regularly logged their symptoms using the app in March and April. The analysis revealed six specific groupings of symptoms emerging at characteristic timepoints in the progression of the illness, representing six distinct ‘types’ of COVID-19. The algorithm was then tested by running it on a second independent dataset of 1,000 users in the UK, US and Sweden, who had logged their symptoms during May. All people reporting symptoms experienced headache and loss of smell, with varying combinations of additional symptoms at various times. Some of these, such as confusion, abdominal pain and shortness of breath, are not widely known as COVID-19 symptoms, yet are hallmarks of the most severe forms of the disease.
The team also discovered that people experiencing particular symptom clusters were more likely to require breathing support in the form of ventilation or additional oxygen. The researchers then developed a model combining information about age, sex, BMI and pre-existing conditions together with symptoms gathered over just five days from the onset of the illness. This was able to predict which cluster a patient falls into and their risk of requiring hospitalization and breathing support with a higher likelihood of being correct than an existing risk model based purely on age, sex, BMI and pre-existing conditions alone. Given that most people who require breathing support come to hospital around 13 days after their first symptoms, this extra eight days represents a significant ‘early warning’ as to who is most likely to need more intensive care.
“These findings have important implications for care and monitoring of people who are most vulnerable to severe COVID-19,” said Dr Claire Steves from King’s College London. “If you can predict who these people are at day five, you have time to give them support and early interventions such as monitoring blood oxygen and sugar levels, and ensuring they are properly hydrated - simple care that could be given at home, preventing hospitalizations and saving lives.”
“Being able to gather big datasets through the app and apply machine learning to them is having a profound impact on our understanding of the extent and impact of COVID-19, and human health more widely,” said Sebastien Ourselin, professor of healthcare engineering at King’s College London and senior author of the study.
Related Links:
King’s College London
The findings have major implications for clinical management of COVID-19, and could help doctors predict who is most at risk and likely to need hospital care in a second wave of coronavirus infections. Although continuous cough, fever and loss of smell (anosmia) are usually highlighted as the three key symptoms of COVID-19, data gathered from app users shows that people can experience a wide range of different symptoms including headaches, muscle pains, fatigue, diarrhea, confusion, loss of appetite, shortness of breath and more. The progression and outcomes also vary significantly between people, ranging from mild flu-like symptoms or a simple rash to severe or fatal disease.
To find out whether particular symptoms tend to appear together and how this related to the progression of the disease, the research team at King’s College London (London, UK) used a machine learning algorithm to analyze data from a subset of around 1,600 users in the UK and US with confirmed COVID-19 who had regularly logged their symptoms using the app in March and April. The analysis revealed six specific groupings of symptoms emerging at characteristic timepoints in the progression of the illness, representing six distinct ‘types’ of COVID-19. The algorithm was then tested by running it on a second independent dataset of 1,000 users in the UK, US and Sweden, who had logged their symptoms during May. All people reporting symptoms experienced headache and loss of smell, with varying combinations of additional symptoms at various times. Some of these, such as confusion, abdominal pain and shortness of breath, are not widely known as COVID-19 symptoms, yet are hallmarks of the most severe forms of the disease.
The team also discovered that people experiencing particular symptom clusters were more likely to require breathing support in the form of ventilation or additional oxygen. The researchers then developed a model combining information about age, sex, BMI and pre-existing conditions together with symptoms gathered over just five days from the onset of the illness. This was able to predict which cluster a patient falls into and their risk of requiring hospitalization and breathing support with a higher likelihood of being correct than an existing risk model based purely on age, sex, BMI and pre-existing conditions alone. Given that most people who require breathing support come to hospital around 13 days after their first symptoms, this extra eight days represents a significant ‘early warning’ as to who is most likely to need more intensive care.
“These findings have important implications for care and monitoring of people who are most vulnerable to severe COVID-19,” said Dr Claire Steves from King’s College London. “If you can predict who these people are at day five, you have time to give them support and early interventions such as monitoring blood oxygen and sugar levels, and ensuring they are properly hydrated - simple care that could be given at home, preventing hospitalizations and saving lives.”
“Being able to gather big datasets through the app and apply machine learning to them is having a profound impact on our understanding of the extent and impact of COVID-19, and human health more widely,” said Sebastien Ourselin, professor of healthcare engineering at King’s College London and senior author of the study.
Related Links:
King’s College London
Latest COVID-19 News
- Low-Cost System Detects SARS-CoV-2 Virus in Hospital Air Using High-Tech Bubbles
- World's First Inhalable COVID-19 Vaccine Approved in China
- COVID-19 Vaccine Patch Fights SARS-CoV-2 Variants Better than Needles
- Blood Viscosity Testing Can Predict Risk of Death in Hospitalized COVID-19 Patients
- ‘Covid Computer’ Uses AI to Detect COVID-19 from Chest CT Scans
- MRI Lung-Imaging Technique Shows Cause of Long-COVID Symptoms
- Chest CT Scans of COVID-19 Patients Could Help Distinguish Between SARS-CoV-2 Variants
- Specialized MRI Detects Lung Abnormalities in Non-Hospitalized Long COVID Patients
- AI Algorithm Identifies Hospitalized Patients at Highest Risk of Dying From COVID-19
- Sweat Sensor Detects Key Biomarkers That Provide Early Warning of COVID-19 and Flu
- Study Assesses Impact of COVID-19 on Ventilation/Perfusion Scintigraphy
- CT Imaging Study Finds Vaccination Reduces Risk of COVID-19 Associated Pulmonary Embolism
- Third Day in Hospital a ‘Tipping Point’ in Severity of COVID-19 Pneumonia
- Longer Interval Between COVID-19 Vaccines Generates Up to Nine Times as Many Antibodies
- AI Model for Monitoring COVID-19 Predicts Mortality Within First 30 Days of Admission
- AI Predicts COVID Prognosis at Near-Expert Level Based Off CT Scans
Channels
Critical Care
view channel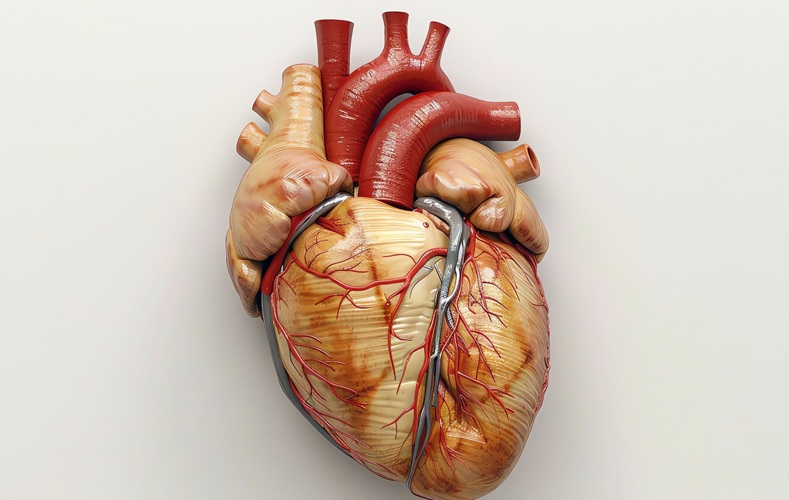
Mechanosensing-Based Approach Offers Promising Strategy to Treat Cardiovascular Fibrosis
Cardiac fibrosis, which involves the stiffening and scarring of heart tissue, is a fundamental feature of nearly every type of heart disease, from acute ischemic injuries to genetic cardiomyopathies.... Read more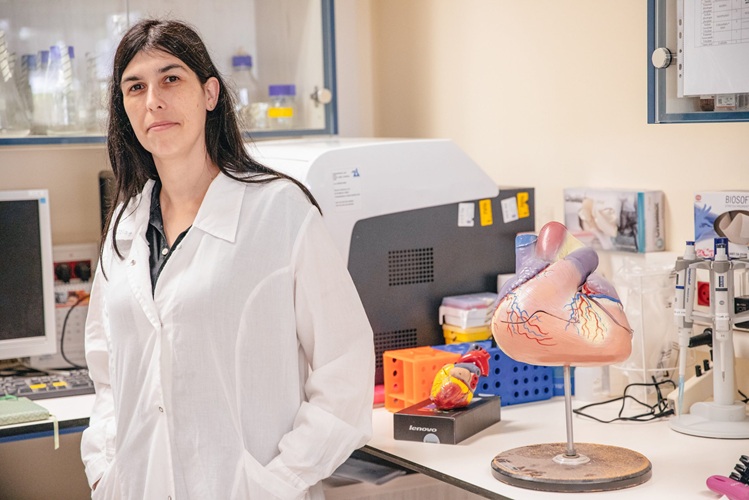
AI Interpretability Tool for Photographed ECG Images Offers Pixel-Level Precision
The electrocardiogram (ECG) is a crucial diagnostic tool in modern medicine, used to detect heart conditions such as arrhythmias and structural abnormalities. Every year, millions of ECGs are performed... Read moreSurgical Techniques
view channel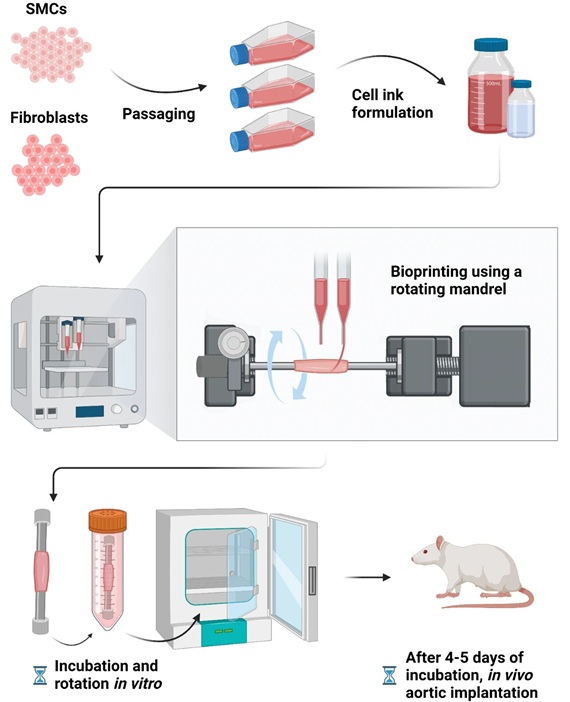
Bioprinted Aortas Offer New Hope for Vascular Repair
Current treatment options for severe cardiovascular diseases include using grafts made from a patient's own tissue (autologous) or synthetic materials. However, autologous grafts require invasive surgery... Read more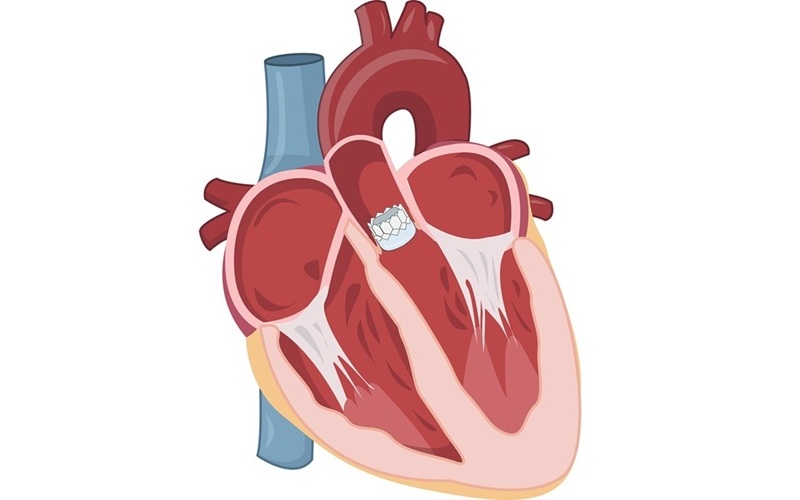
Early TAVR Intervention Reduces Cardiovascular Events in Asymptomatic Aortic Stenosis Patients
Each year, approximately 300,000 Americans are diagnosed with aortic stenosis (AS), a serious condition that results from the narrowing or blockage of the aortic valve in the heart. Two common treatments... Read more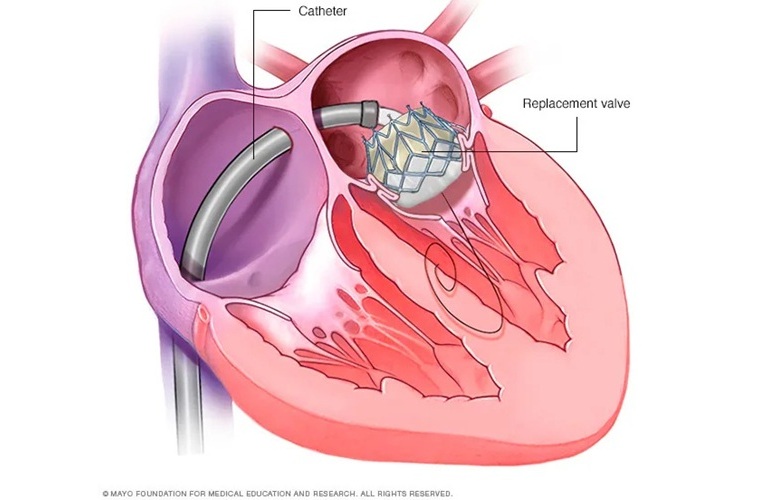
New Procedure Found Safe and Effective for Patients Undergoing Transcatheter Mitral Valve Replacement
In the United States, approximately four million people suffer from mitral valve regurgitation, the most common type of heart valve disease. As an alternative to open-heart surgery, transcatheter mitral... Read morePatient Care
view channel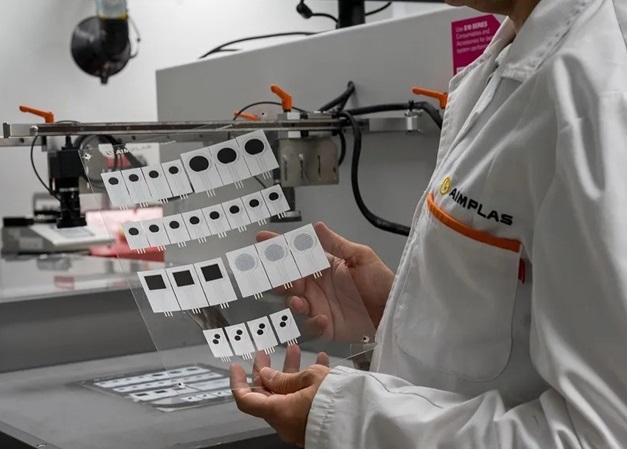
Portable Biosensor Platform to Reduce Hospital-Acquired Infections
Approximately 4 million patients in the European Union acquire healthcare-associated infections (HAIs) or nosocomial infections each year, with around 37,000 deaths directly resulting from these infections,... Read more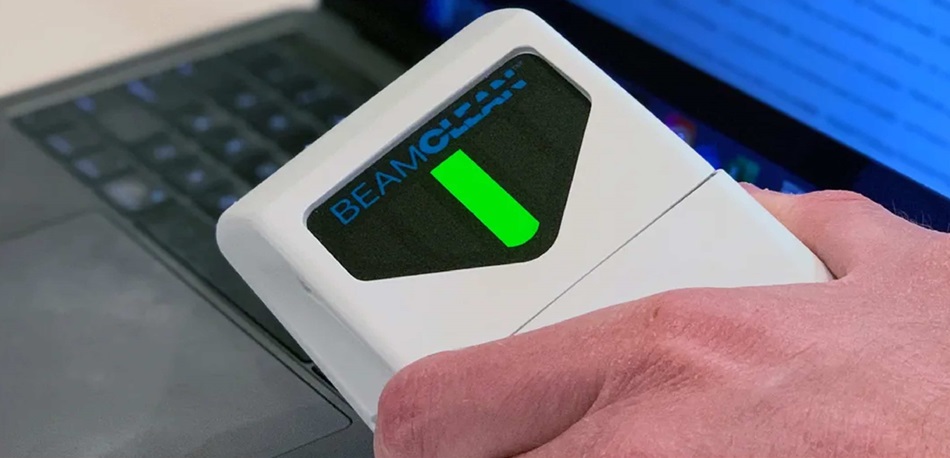
First-Of-Its-Kind Portable Germicidal Light Technology Disinfects High-Touch Clinical Surfaces in Seconds
Reducing healthcare-acquired infections (HAIs) remains a pressing issue within global healthcare systems. In the United States alone, 1.7 million patients contract HAIs annually, leading to approximately... Read more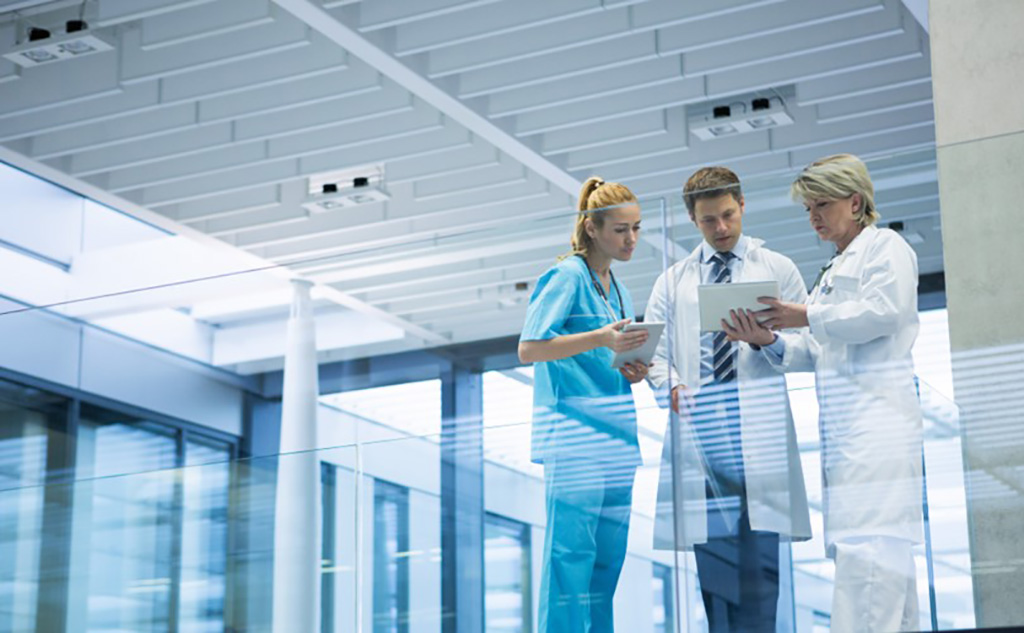
Surgical Capacity Optimization Solution Helps Hospitals Boost OR Utilization
An innovative solution has the capability to transform surgical capacity utilization by targeting the root cause of surgical block time inefficiencies. Fujitsu Limited’s (Tokyo, Japan) Surgical Capacity... Read more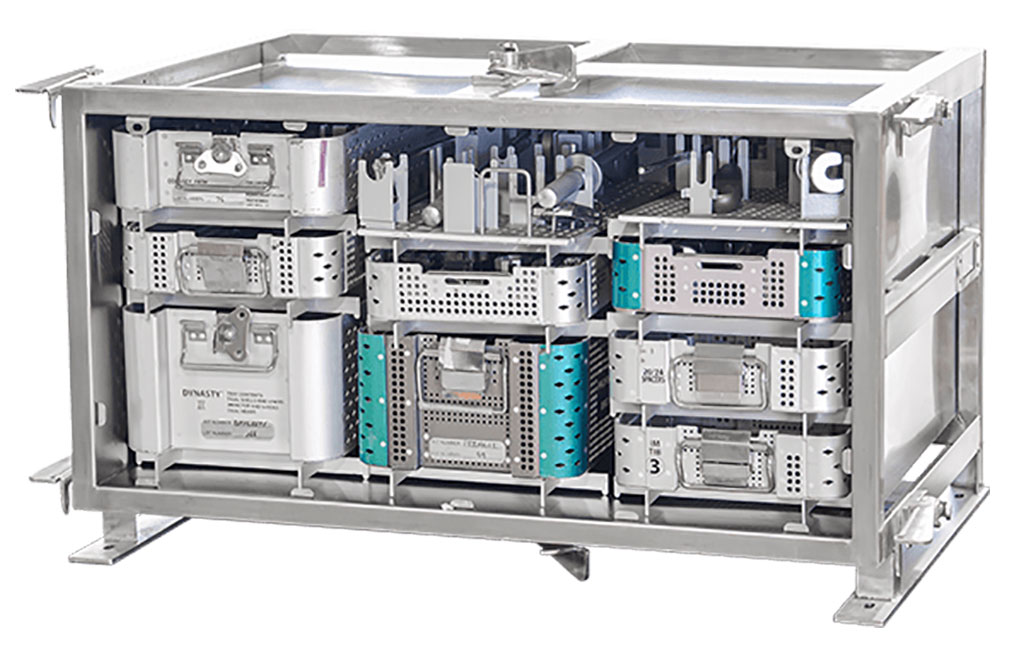
Game-Changing Innovation in Surgical Instrument Sterilization Significantly Improves OR Throughput
A groundbreaking innovation enables hospitals to significantly improve instrument processing time and throughput in operating rooms (ORs) and sterile processing departments. Turbett Surgical, Inc.... Read moreHealth IT
view channel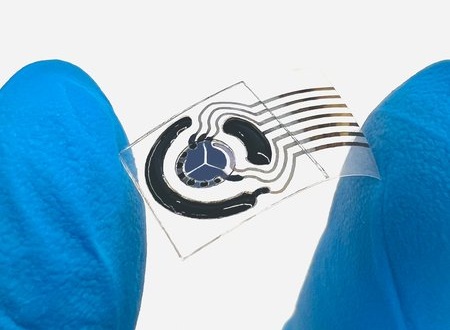
Printable Molecule-Selective Nanoparticles Enable Mass Production of Wearable Biosensors
The future of medicine is likely to focus on the personalization of healthcare—understanding exactly what an individual requires and delivering the appropriate combination of nutrients, metabolites, and... Read more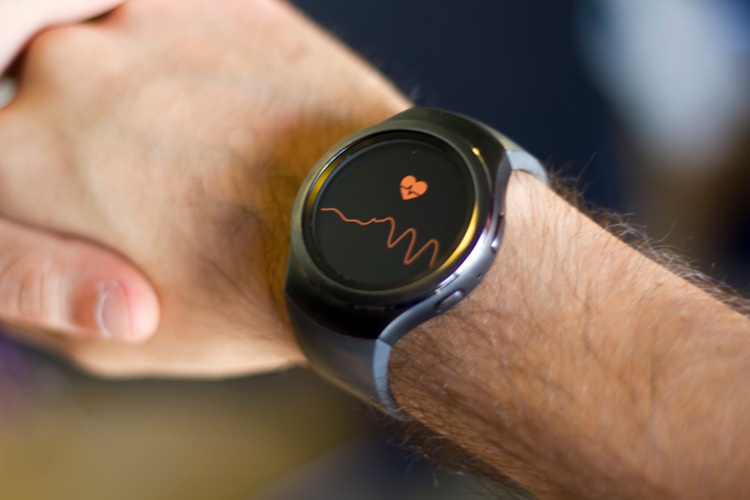
Smartwatches Could Detect Congestive Heart Failure
Diagnosing congestive heart failure (CHF) typically requires expensive and time-consuming imaging techniques like echocardiography, also known as cardiac ultrasound. Previously, detecting CHF by analyzing... Read moreBusiness
view channel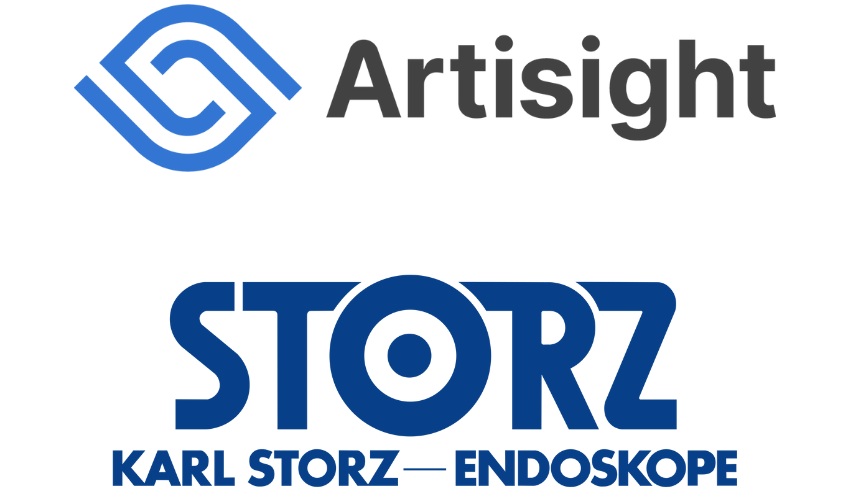
Expanded Collaboration to Transform OR Technology Through AI and Automation
The expansion of an existing collaboration between three leading companies aims to develop artificial intelligence (AI)-driven solutions for smart operating rooms with sophisticated monitoring and automation.... Read more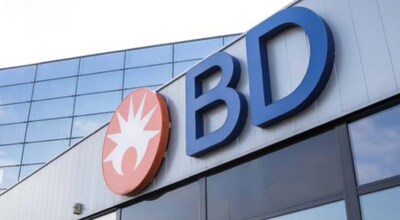