New AI Research to Help Predict COVID-19 Resource Needs from Series of X-Rays
By HospiMedica International staff writers Posted on 18 Jan 2021 |
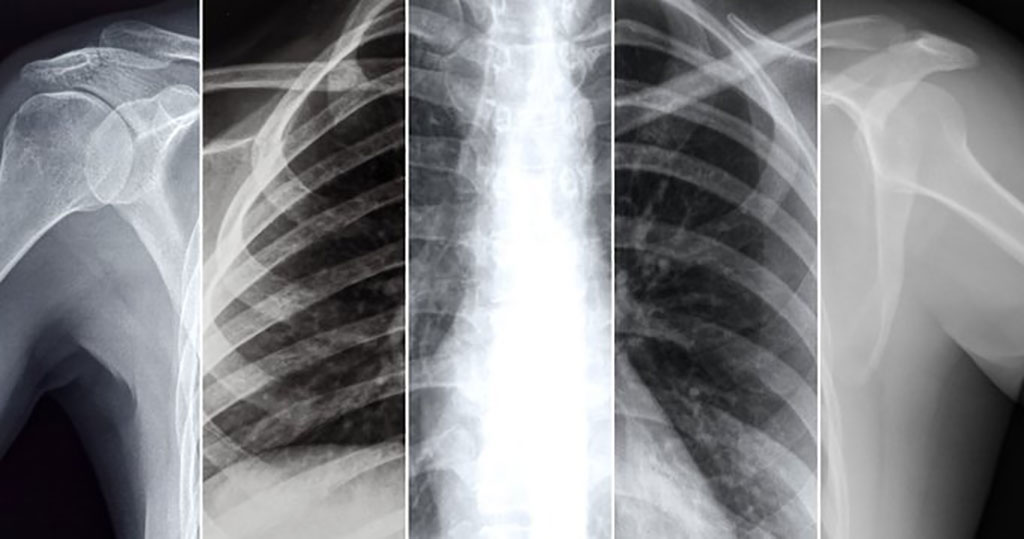
Illustration
Scientists have developed machine learning (ML) models that could help doctors predict how a COVID-19 patient’s condition may develop, in order to help hospitals ensure they have sufficient resources to care for patients.
Facebook AI (Menlo Park, CA, USA), in collaboration with NYU Langone Health’s Predictive Analytics Unit and Department of Radiology (Brooklyn, NY, USA) has developed three ML models that can help hospitals know whether COVID-19 patients are likely to need escalated treatment and plan accordingly. The first ML model can predict patient deterioration based on a single X-ray and the second model can predict patient deterioration based on a sequence of X-rays, while the third model can predict how much supplemental oxygen (if any) a patient might need based on a single X-ray. Their model using sequential chest X-rays can predict up to four days (96 hours) in advance if a patient may need more intensive care solutions, generally outperforming predictions by human experts. These predictions could help doctors avoid sending at-risk patients home too soon, and help hospitals better predict demand for supplemental oxygen and other limited resources.
Previous approaches to this problem have relied on supervised training and used single timeframe images. While progress has been made with supervised training methods, labeling data is extremely time-intensive and thus limiting. The researchers chose instead to pretrain their ML system on two large, public chest X-ray data sets, MIMIC-CXR-JPG and CheXpert, using a self-supervised learning technique called Momentum Contrast (MoCo). This allowed them to use large amounts of non-COVID chest X-ray data to train a neural network that could extract information from chest X-ray images. Then the team fine-tuned the MoCo model using an extended version of the NYU COVID-19 data set.
MoCo relies on unsupervised learning using a contrastive loss function, mapping images to a latent space wherein similar images are mapped to vectors that are close together and dissimilar images to vectors that are further apart. These vectors can be used as feature representations, allowing one to train classifiers using a small number of labeled examples. Recent research shows that self-supervised learning using contrastive loss functions is effective in a variety of classification tasks. After pretraining the MoCo model on MIMIC-CXR-JPG and CheXpert, the researchers then used the pretrained model to build classifiers that could predict whether a COVID-19 patient’s condition is likely to deteriorate. The team used the NYU COVID chest X-ray data set for fine-tuning, as it contained 26,838 X-ray images taken from 4,914 patients. This smaller data set was labeled with whether the patient’s condition worsened within 24, 48, 72, or 96 hours of the scan in question.
The researchers built two kinds of classifiers to predict patient deterioration. The first model predicts patient deterioration based on a single X-ray in a fashion similar to a previous study. The second model predicts patient deterioration based on a sequence of X-rays by aggregating the image features via a Transformer model. Using self-supervised learning without having to rely on labeled data sets is crucial, as few research groups have enough COVID chest X-rays to train AI models. Building AI models that can use a sequence of X-rays for prediction purposes is particularly valuable because this method mirrors how human radiologists work, as using a sequence of X-rays is more accurate for long-term predictions. Importantly, this method also accounts for the evolution of COVID infections over time.
Based on reader studies conducted by Facebook AI researchers with radiologists at NYU Langone, their models that used sequences of X-ray images outperformed human experts at predicting ICU needs and mortality predictions, and overall adverse event predictions in the longer term (up to 96 hours). Being able to predict whether a patient will need oxygen resources would also be a first, and could help hospitals as they decide how to allocate resources in the weeks and months to come. With COVID-19 cases rising again across the world, hospitals need tools to predict and prepare for upcoming surges as they plan their resource allocations. These models could help in fight against COVID-19.
“We have been able to show that with the use of this AI algorithm, serial chest radiographs can predict the need for escalation of care in patients with COVID-19,” said William Moore, MD, a Professor of Radiology at NYU Langone Health. “As COVID-19 continues to be a major public health issue, the ability to predict a patient’s need for elevation of care - for example, ICU admission - will be essential for hospitals.”
Related Links:
Facebook AI
NYU Langone Health
Facebook AI (Menlo Park, CA, USA), in collaboration with NYU Langone Health’s Predictive Analytics Unit and Department of Radiology (Brooklyn, NY, USA) has developed three ML models that can help hospitals know whether COVID-19 patients are likely to need escalated treatment and plan accordingly. The first ML model can predict patient deterioration based on a single X-ray and the second model can predict patient deterioration based on a sequence of X-rays, while the third model can predict how much supplemental oxygen (if any) a patient might need based on a single X-ray. Their model using sequential chest X-rays can predict up to four days (96 hours) in advance if a patient may need more intensive care solutions, generally outperforming predictions by human experts. These predictions could help doctors avoid sending at-risk patients home too soon, and help hospitals better predict demand for supplemental oxygen and other limited resources.
Previous approaches to this problem have relied on supervised training and used single timeframe images. While progress has been made with supervised training methods, labeling data is extremely time-intensive and thus limiting. The researchers chose instead to pretrain their ML system on two large, public chest X-ray data sets, MIMIC-CXR-JPG and CheXpert, using a self-supervised learning technique called Momentum Contrast (MoCo). This allowed them to use large amounts of non-COVID chest X-ray data to train a neural network that could extract information from chest X-ray images. Then the team fine-tuned the MoCo model using an extended version of the NYU COVID-19 data set.
MoCo relies on unsupervised learning using a contrastive loss function, mapping images to a latent space wherein similar images are mapped to vectors that are close together and dissimilar images to vectors that are further apart. These vectors can be used as feature representations, allowing one to train classifiers using a small number of labeled examples. Recent research shows that self-supervised learning using contrastive loss functions is effective in a variety of classification tasks. After pretraining the MoCo model on MIMIC-CXR-JPG and CheXpert, the researchers then used the pretrained model to build classifiers that could predict whether a COVID-19 patient’s condition is likely to deteriorate. The team used the NYU COVID chest X-ray data set for fine-tuning, as it contained 26,838 X-ray images taken from 4,914 patients. This smaller data set was labeled with whether the patient’s condition worsened within 24, 48, 72, or 96 hours of the scan in question.
The researchers built two kinds of classifiers to predict patient deterioration. The first model predicts patient deterioration based on a single X-ray in a fashion similar to a previous study. The second model predicts patient deterioration based on a sequence of X-rays by aggregating the image features via a Transformer model. Using self-supervised learning without having to rely on labeled data sets is crucial, as few research groups have enough COVID chest X-rays to train AI models. Building AI models that can use a sequence of X-rays for prediction purposes is particularly valuable because this method mirrors how human radiologists work, as using a sequence of X-rays is more accurate for long-term predictions. Importantly, this method also accounts for the evolution of COVID infections over time.
Based on reader studies conducted by Facebook AI researchers with radiologists at NYU Langone, their models that used sequences of X-ray images outperformed human experts at predicting ICU needs and mortality predictions, and overall adverse event predictions in the longer term (up to 96 hours). Being able to predict whether a patient will need oxygen resources would also be a first, and could help hospitals as they decide how to allocate resources in the weeks and months to come. With COVID-19 cases rising again across the world, hospitals need tools to predict and prepare for upcoming surges as they plan their resource allocations. These models could help in fight against COVID-19.
“We have been able to show that with the use of this AI algorithm, serial chest radiographs can predict the need for escalation of care in patients with COVID-19,” said William Moore, MD, a Professor of Radiology at NYU Langone Health. “As COVID-19 continues to be a major public health issue, the ability to predict a patient’s need for elevation of care - for example, ICU admission - will be essential for hospitals.”
Related Links:
Facebook AI
NYU Langone Health
Latest COVID-19 News
- Low-Cost System Detects SARS-CoV-2 Virus in Hospital Air Using High-Tech Bubbles
- World's First Inhalable COVID-19 Vaccine Approved in China
- COVID-19 Vaccine Patch Fights SARS-CoV-2 Variants Better than Needles
- Blood Viscosity Testing Can Predict Risk of Death in Hospitalized COVID-19 Patients
- ‘Covid Computer’ Uses AI to Detect COVID-19 from Chest CT Scans
- MRI Lung-Imaging Technique Shows Cause of Long-COVID Symptoms
- Chest CT Scans of COVID-19 Patients Could Help Distinguish Between SARS-CoV-2 Variants
- Specialized MRI Detects Lung Abnormalities in Non-Hospitalized Long COVID Patients
- AI Algorithm Identifies Hospitalized Patients at Highest Risk of Dying From COVID-19
- Sweat Sensor Detects Key Biomarkers That Provide Early Warning of COVID-19 and Flu
- Study Assesses Impact of COVID-19 on Ventilation/Perfusion Scintigraphy
- CT Imaging Study Finds Vaccination Reduces Risk of COVID-19 Associated Pulmonary Embolism
- Third Day in Hospital a ‘Tipping Point’ in Severity of COVID-19 Pneumonia
- Longer Interval Between COVID-19 Vaccines Generates Up to Nine Times as Many Antibodies
- AI Model for Monitoring COVID-19 Predicts Mortality Within First 30 Days of Admission
- AI Predicts COVID Prognosis at Near-Expert Level Based Off CT Scans
Channels
Critical Care
view channel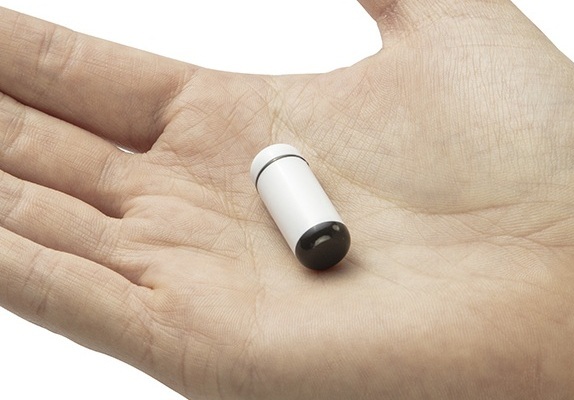
Ingestible Smart Capsule for Chemical Sensing in the Gut Moves Closer to Market
Intestinal gases are associated with several health conditions, including colon cancer, irritable bowel syndrome, and inflammatory bowel disease, and they have the potential to serve as crucial biomarkers... Read more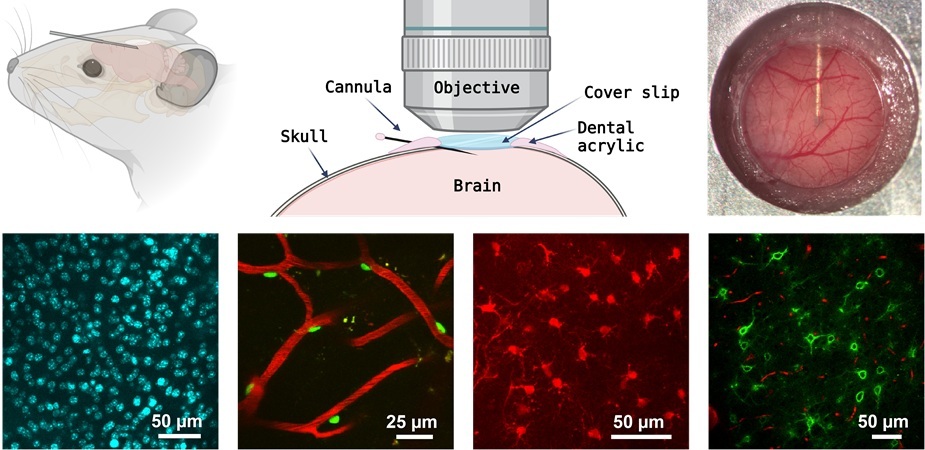
Novel Cannula Delivery System Enables Targeted Delivery of Imaging Agents and Drugs
Multiphoton microscopy has become an invaluable tool in neuroscience, allowing researchers to observe brain activity in real time with high-resolution imaging. A crucial aspect of many multiphoton microscopy... Read more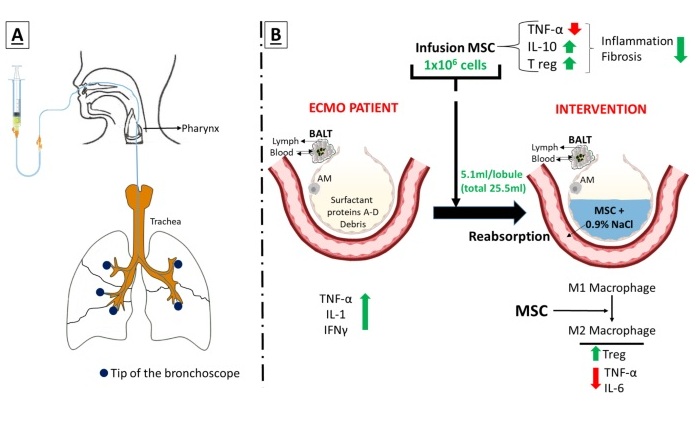
Novel Intrabronchial Method Delivers Cell Therapies in Critically Ill Patients on External Lung Support
Until now, administering cell therapies to patients on extracorporeal membrane oxygenation (ECMO)—a life-support system typically used for severe lung failure—has been nearly impossible.... Read moreSurgical Techniques
view channel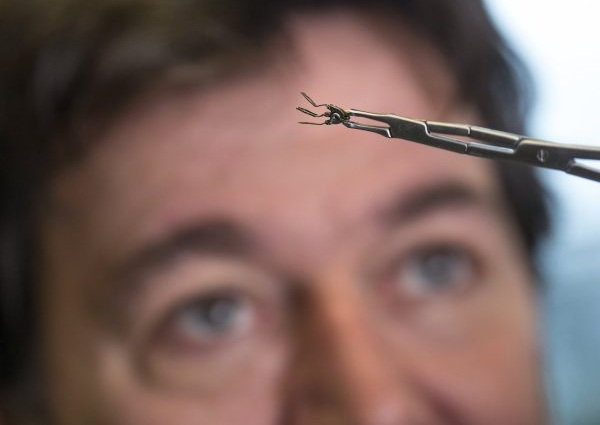
Pioneering Sutureless Coronary Bypass Technology to Eliminate Open-Chest Procedures
In patients with coronary artery disease, certain blood vessels may be narrowed or blocked, requiring a stent or a bypass (also known as diversion) to restore blood flow to the heart. Bypass surgeries... Read more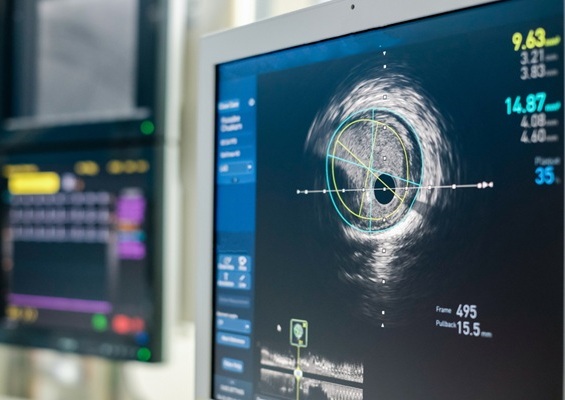
Intravascular Imaging for Guiding Stent Implantation Ensures Safer Stenting Procedures
Patients diagnosed with coronary artery disease, which is caused by plaque accumulation within the arteries leading to chest pain, shortness of breath, and potential heart attacks, frequently undergo percutaneous... Read more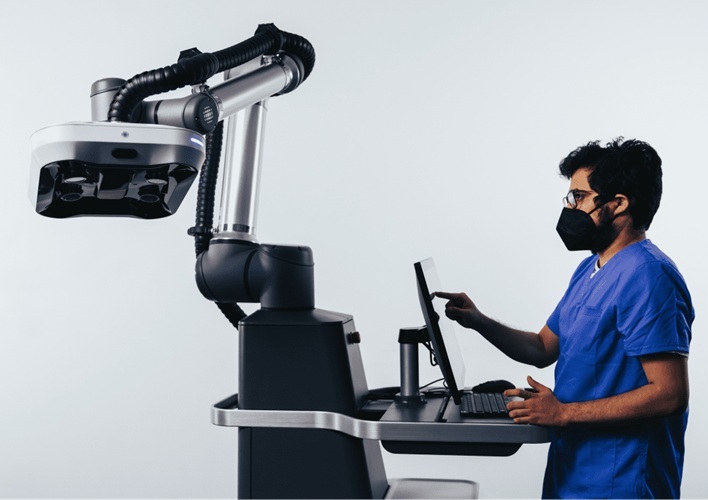
World's First AI Surgical Guidance Platform Allows Surgeons to Measure Success in Real-Time
Surgeons have always faced challenges in measuring their progress toward surgical goals during procedures. Traditionally, obtaining measurements required stepping out of the sterile environment to perform... Read morePatient Care
view channel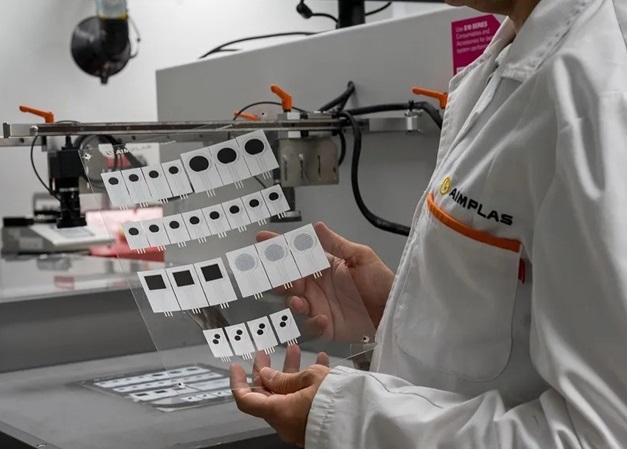
Portable Biosensor Platform to Reduce Hospital-Acquired Infections
Approximately 4 million patients in the European Union acquire healthcare-associated infections (HAIs) or nosocomial infections each year, with around 37,000 deaths directly resulting from these infections,... Read more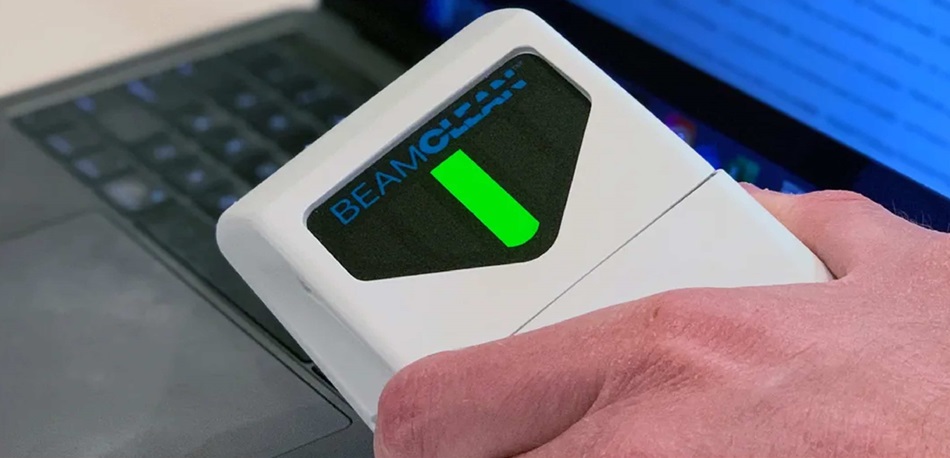
First-Of-Its-Kind Portable Germicidal Light Technology Disinfects High-Touch Clinical Surfaces in Seconds
Reducing healthcare-acquired infections (HAIs) remains a pressing issue within global healthcare systems. In the United States alone, 1.7 million patients contract HAIs annually, leading to approximately... Read more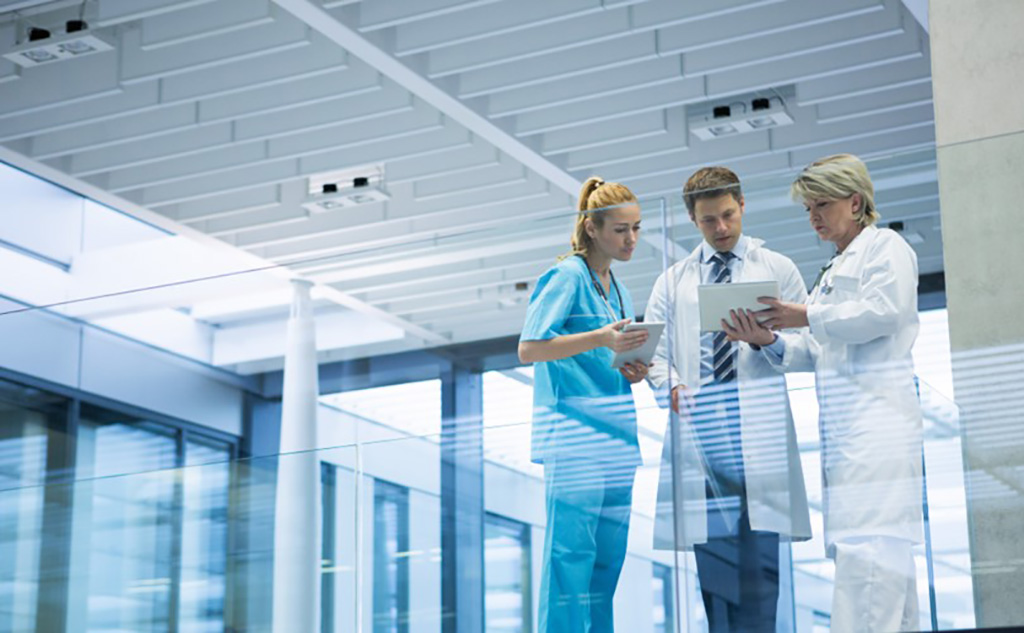
Surgical Capacity Optimization Solution Helps Hospitals Boost OR Utilization
An innovative solution has the capability to transform surgical capacity utilization by targeting the root cause of surgical block time inefficiencies. Fujitsu Limited’s (Tokyo, Japan) Surgical Capacity... Read more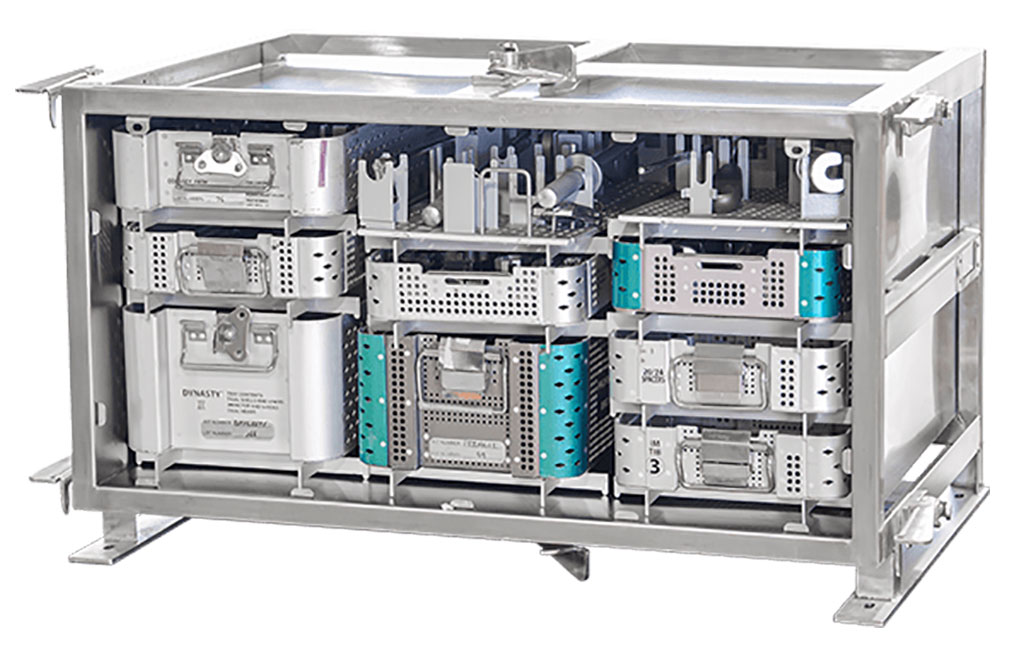
Game-Changing Innovation in Surgical Instrument Sterilization Significantly Improves OR Throughput
A groundbreaking innovation enables hospitals to significantly improve instrument processing time and throughput in operating rooms (ORs) and sterile processing departments. Turbett Surgical, Inc.... Read moreHealth IT
view channel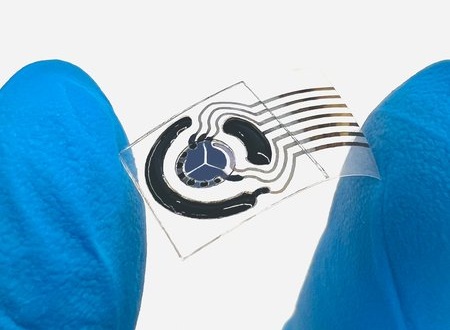
Printable Molecule-Selective Nanoparticles Enable Mass Production of Wearable Biosensors
The future of medicine is likely to focus on the personalization of healthcare—understanding exactly what an individual requires and delivering the appropriate combination of nutrients, metabolites, and... Read more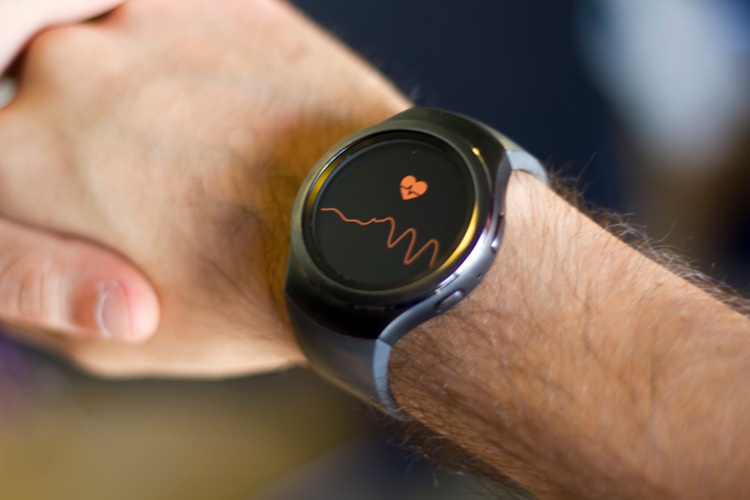
Smartwatches Could Detect Congestive Heart Failure
Diagnosing congestive heart failure (CHF) typically requires expensive and time-consuming imaging techniques like echocardiography, also known as cardiac ultrasound. Previously, detecting CHF by analyzing... Read moreBusiness
view channel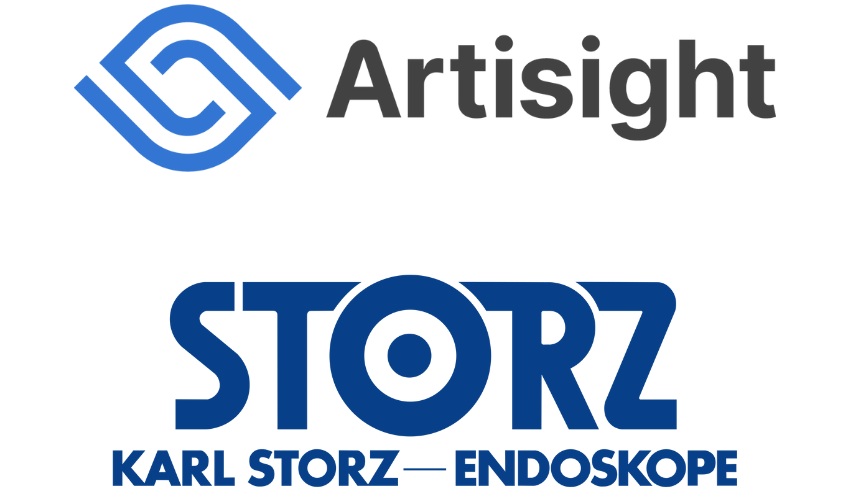
Expanded Collaboration to Transform OR Technology Through AI and Automation
The expansion of an existing collaboration between three leading companies aims to develop artificial intelligence (AI)-driven solutions for smart operating rooms with sophisticated monitoring and automation.... Read more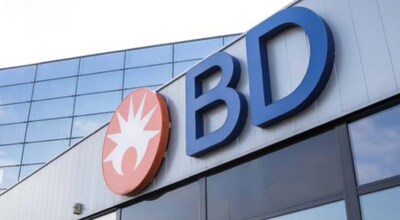