Deep-Learning Model that Can Predict How Human Genes and Medicines Will Interact Identifies Promising COVID-19 Treatments
By HospiMedica International staff writers Posted on 03 Feb 2021 |
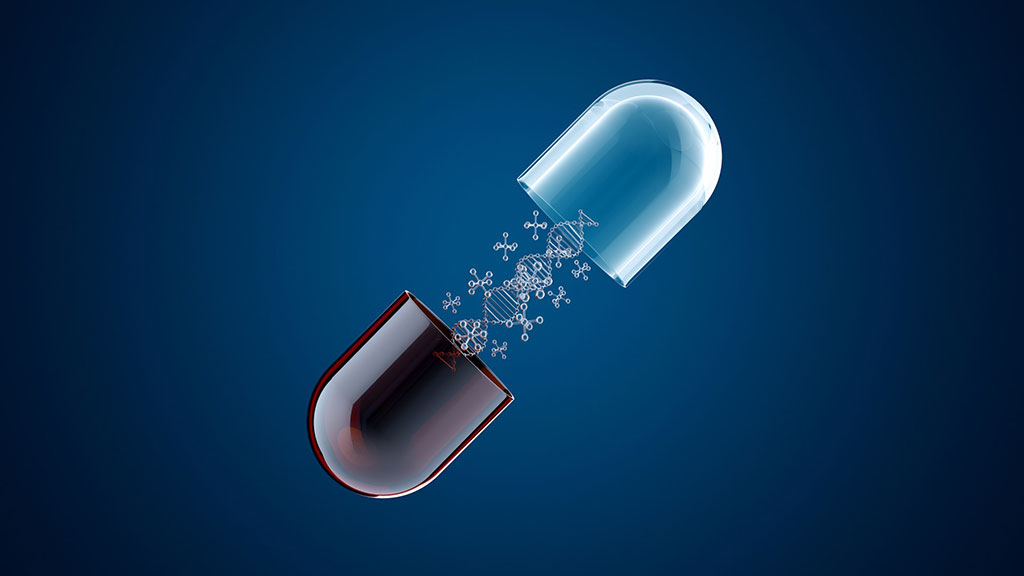
Image: The computer model can predict how human genes and medicines will interact (Photo courtesy of Getty Images)
A new deep-learning model that can predict how human genes and medicines will interact has identified at least 10 compounds that may hold promise as treatments for COVID-19.
The computer model, which the researchers call “DeepCE” - pronounced “Deep Sea” was created by computer scientists at The Ohio State University (Columbus, OH, USA) and helps find drug repurposing candidates for new diseases. All but two of the drugs identified as potential COVID-19 treatments by the deep-learning model are still considered investigational and are being tested for effectiveness against hepatitis C, fungal disease, cancer and heart disease. The list also includes the approved drugs cyclosporine, an immunosuppressant that prevents transplant organ rejection, and anidulafungin, an antifungal agent.
Much more work needs to be done before any of these medications would be confirmed as safe and effective treatments for people infected with SARS-CoV-2. But by using artificial intelligence to arrive at these options, the scientists have saved pharmaceutical and clinical researchers the time and money it would take to search for potential COVID-19 drugs on a piecemeal basis. The researchers have noted that a few of the repurposing candidates the model generated have already been studied for their potential use in COVID-19 patients.
To make predictions about how genes and medicines will interact and yield drug repurposing candidates, DeepCE relies on two primary sources of publicly available data: L1000, a National Institutes of Health-funded repository of human cell-line data showing how gene expression changes in response to drugs, and DrugBank, which contains information on the chemical structures and other details on about 11,000 approved and investigational drugs.
L1000 displays side-by-side cell-line comparisons of standard gene expression activity with gene expression changes produced by interactions with specific drugs. The cell lines represent diseases, such as melanoma, and organs, like kidneys and lungs. It is an ongoing project, with data being added as experiments in animals or humans supplement the gene expression profiles produced in cell-line experiments.
The Ohio State researchers trained the DeepCE model by running all of the L1000 data through an algorithm against specific chemical compounds and their dosages. To fill in data gaps, the model converts chemical compound descriptions into figures, allowing for automatic consideration of their separate components’ effects on genes. And for genes not represented in L1000, the team used a deep learning approach called an “attention mechanism” to increase the model’s “learned” sample of gene-chemical compound interactions, which improves the framework’s performance.
The team applied DeepCE’s gene expression prediction matrix - focusing on data from lung and airway cell lines and the entire DrugBank catalog of compounds - to the genetic information provided from the early COVID-19 papers and additional government data. The COVID-19 data demonstrated how human gene expression had responded to being infected with SARS-CoV-2, creating a “disease signature.”
“When no one has any information on a new disease, this model shows how artificial intelligence can help solve the problem of how to consider a potential treatment,” said senior author Ping Zhang, assistant professor of computer science and engineering and biomedical informatics at The Ohio State University. “Great minds think alike - some lead compounds identified by machine intelligence coincide with later discoveries by human intelligence.”
Related Links:
The Ohio State University
The computer model, which the researchers call “DeepCE” - pronounced “Deep Sea” was created by computer scientists at The Ohio State University (Columbus, OH, USA) and helps find drug repurposing candidates for new diseases. All but two of the drugs identified as potential COVID-19 treatments by the deep-learning model are still considered investigational and are being tested for effectiveness against hepatitis C, fungal disease, cancer and heart disease. The list also includes the approved drugs cyclosporine, an immunosuppressant that prevents transplant organ rejection, and anidulafungin, an antifungal agent.
Much more work needs to be done before any of these medications would be confirmed as safe and effective treatments for people infected with SARS-CoV-2. But by using artificial intelligence to arrive at these options, the scientists have saved pharmaceutical and clinical researchers the time and money it would take to search for potential COVID-19 drugs on a piecemeal basis. The researchers have noted that a few of the repurposing candidates the model generated have already been studied for their potential use in COVID-19 patients.
To make predictions about how genes and medicines will interact and yield drug repurposing candidates, DeepCE relies on two primary sources of publicly available data: L1000, a National Institutes of Health-funded repository of human cell-line data showing how gene expression changes in response to drugs, and DrugBank, which contains information on the chemical structures and other details on about 11,000 approved and investigational drugs.
L1000 displays side-by-side cell-line comparisons of standard gene expression activity with gene expression changes produced by interactions with specific drugs. The cell lines represent diseases, such as melanoma, and organs, like kidneys and lungs. It is an ongoing project, with data being added as experiments in animals or humans supplement the gene expression profiles produced in cell-line experiments.
The Ohio State researchers trained the DeepCE model by running all of the L1000 data through an algorithm against specific chemical compounds and their dosages. To fill in data gaps, the model converts chemical compound descriptions into figures, allowing for automatic consideration of their separate components’ effects on genes. And for genes not represented in L1000, the team used a deep learning approach called an “attention mechanism” to increase the model’s “learned” sample of gene-chemical compound interactions, which improves the framework’s performance.
The team applied DeepCE’s gene expression prediction matrix - focusing on data from lung and airway cell lines and the entire DrugBank catalog of compounds - to the genetic information provided from the early COVID-19 papers and additional government data. The COVID-19 data demonstrated how human gene expression had responded to being infected with SARS-CoV-2, creating a “disease signature.”
“When no one has any information on a new disease, this model shows how artificial intelligence can help solve the problem of how to consider a potential treatment,” said senior author Ping Zhang, assistant professor of computer science and engineering and biomedical informatics at The Ohio State University. “Great minds think alike - some lead compounds identified by machine intelligence coincide with later discoveries by human intelligence.”
Related Links:
The Ohio State University
Latest COVID-19 News
- Low-Cost System Detects SARS-CoV-2 Virus in Hospital Air Using High-Tech Bubbles
- World's First Inhalable COVID-19 Vaccine Approved in China
- COVID-19 Vaccine Patch Fights SARS-CoV-2 Variants Better than Needles
- Blood Viscosity Testing Can Predict Risk of Death in Hospitalized COVID-19 Patients
- ‘Covid Computer’ Uses AI to Detect COVID-19 from Chest CT Scans
- MRI Lung-Imaging Technique Shows Cause of Long-COVID Symptoms
- Chest CT Scans of COVID-19 Patients Could Help Distinguish Between SARS-CoV-2 Variants
- Specialized MRI Detects Lung Abnormalities in Non-Hospitalized Long COVID Patients
- AI Algorithm Identifies Hospitalized Patients at Highest Risk of Dying From COVID-19
- Sweat Sensor Detects Key Biomarkers That Provide Early Warning of COVID-19 and Flu
- Study Assesses Impact of COVID-19 on Ventilation/Perfusion Scintigraphy
- CT Imaging Study Finds Vaccination Reduces Risk of COVID-19 Associated Pulmonary Embolism
- Third Day in Hospital a ‘Tipping Point’ in Severity of COVID-19 Pneumonia
- Longer Interval Between COVID-19 Vaccines Generates Up to Nine Times as Many Antibodies
- AI Model for Monitoring COVID-19 Predicts Mortality Within First 30 Days of Admission
- AI Predicts COVID Prognosis at Near-Expert Level Based Off CT Scans
Channels
Critical Care
view channel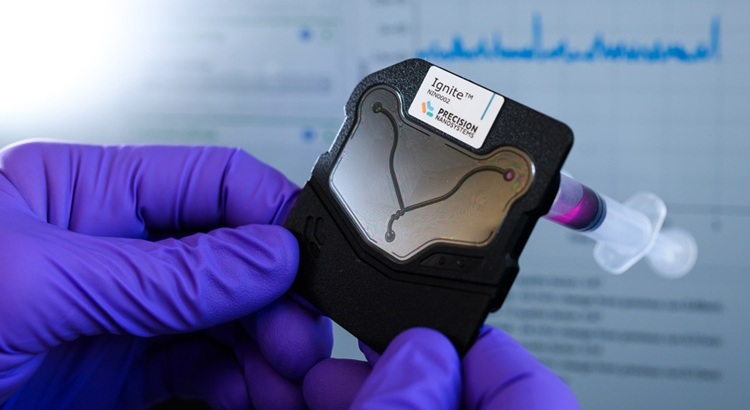
Mass Manufactured Nanoparticles to Deliver Cancer Drugs Directly to Tumors
Polymer-coated nanoparticles loaded with therapeutic drugs hold significant potential for treating cancers, including ovarian cancer. These particles can be precisely directed to tumors, delivering their... Read more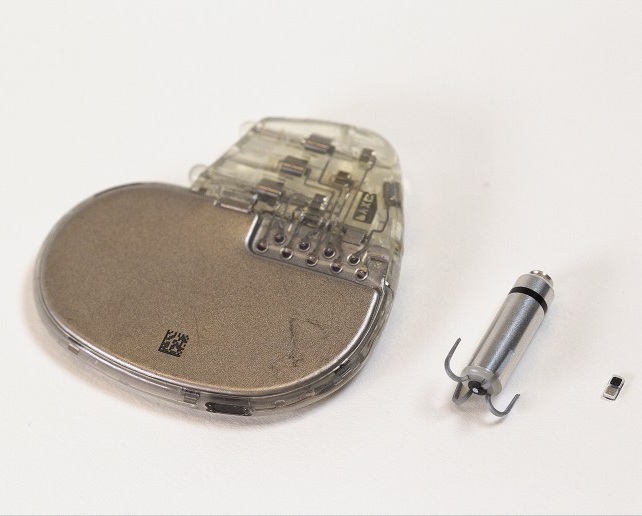
World’s Smallest Pacemaker Fits Inside Syringe Tip
After heart surgery, many patients require temporary pacemakers either to regulate the heart rate while waiting for a permanent pacemaker or to support normal heart rhythm during recovery.... Read more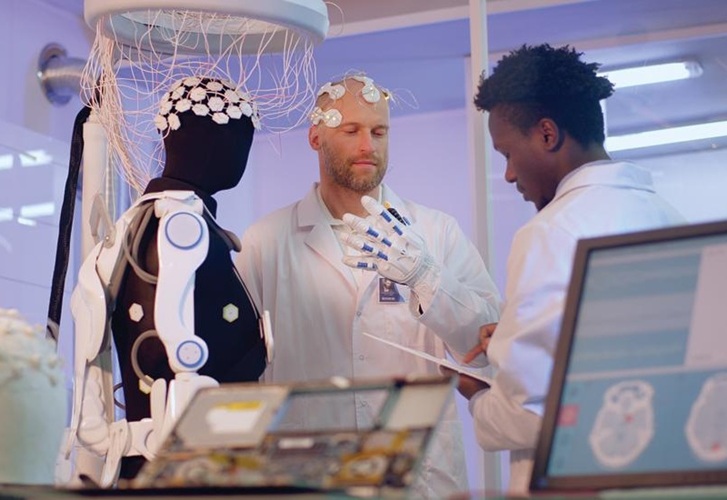
AI-Powered, Internet-Connected Medical Devices to Revolutionize Healthcare, Finds Study
A new study suggests that artificial intelligence (AI)-powered, internet-connected medical devices have the potential to transform healthcare by enabling earlier detection of diseases, real-time patient... Read moreSurgical Techniques
view channel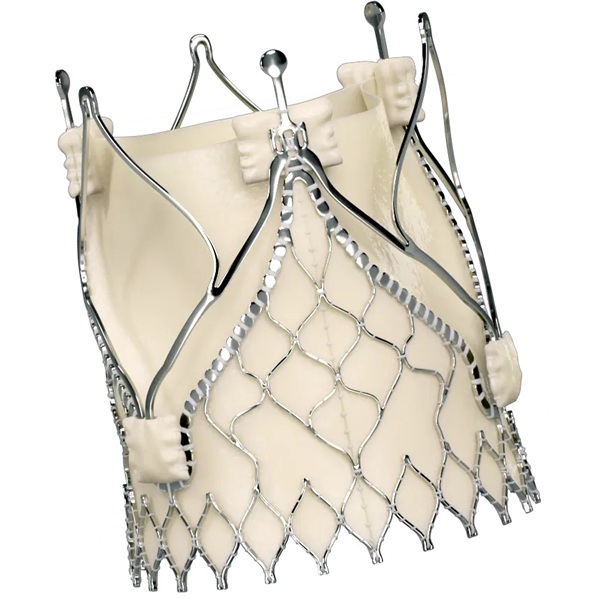
New Transcatheter Valve Found Safe and Effective for Treating Aortic Regurgitation
Aortic regurgitation is a condition in which the aortic valve does not close properly, allowing blood to flow backward into the left ventricle. This results in decreased blood flow from the heart to the... Read more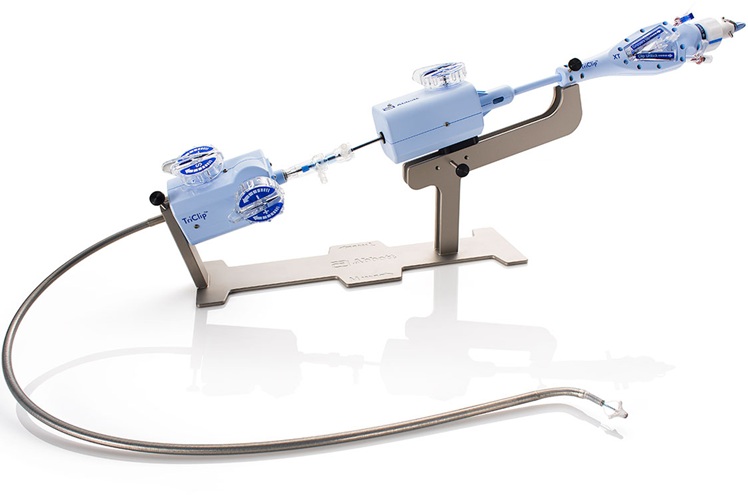
Minimally Invasive Valve Repair Reduces Hospitalizations in Severe Tricuspid Regurgitation Patients
The tricuspid valve is one of the four heart valves, responsible for regulating blood flow from the right atrium (the heart's upper-right chamber) to the right ventricle (the lower-right chamber).... Read morePatient Care
view channel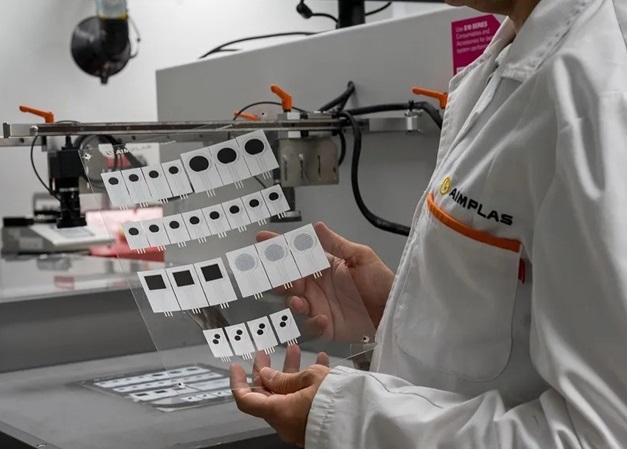
Portable Biosensor Platform to Reduce Hospital-Acquired Infections
Approximately 4 million patients in the European Union acquire healthcare-associated infections (HAIs) or nosocomial infections each year, with around 37,000 deaths directly resulting from these infections,... Read more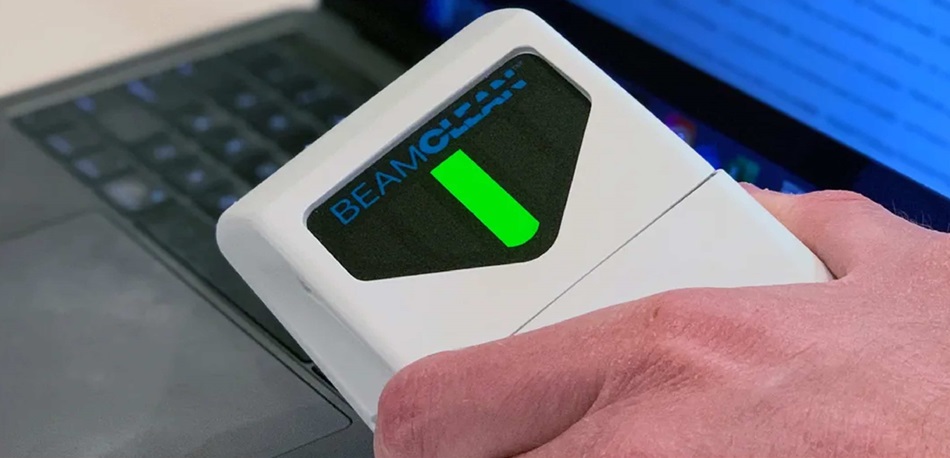
First-Of-Its-Kind Portable Germicidal Light Technology Disinfects High-Touch Clinical Surfaces in Seconds
Reducing healthcare-acquired infections (HAIs) remains a pressing issue within global healthcare systems. In the United States alone, 1.7 million patients contract HAIs annually, leading to approximately... Read more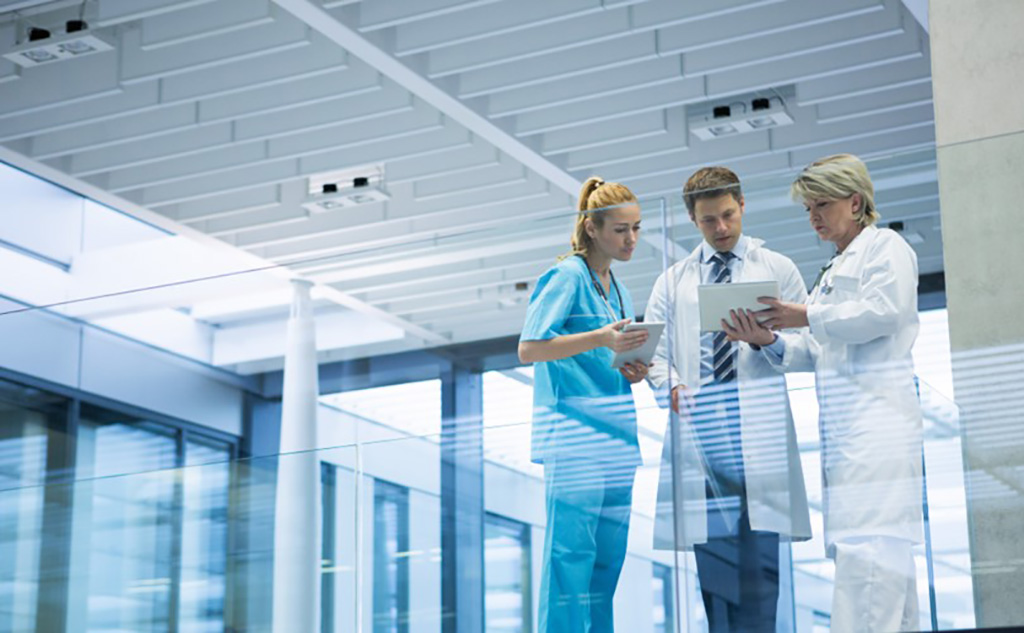
Surgical Capacity Optimization Solution Helps Hospitals Boost OR Utilization
An innovative solution has the capability to transform surgical capacity utilization by targeting the root cause of surgical block time inefficiencies. Fujitsu Limited’s (Tokyo, Japan) Surgical Capacity... Read more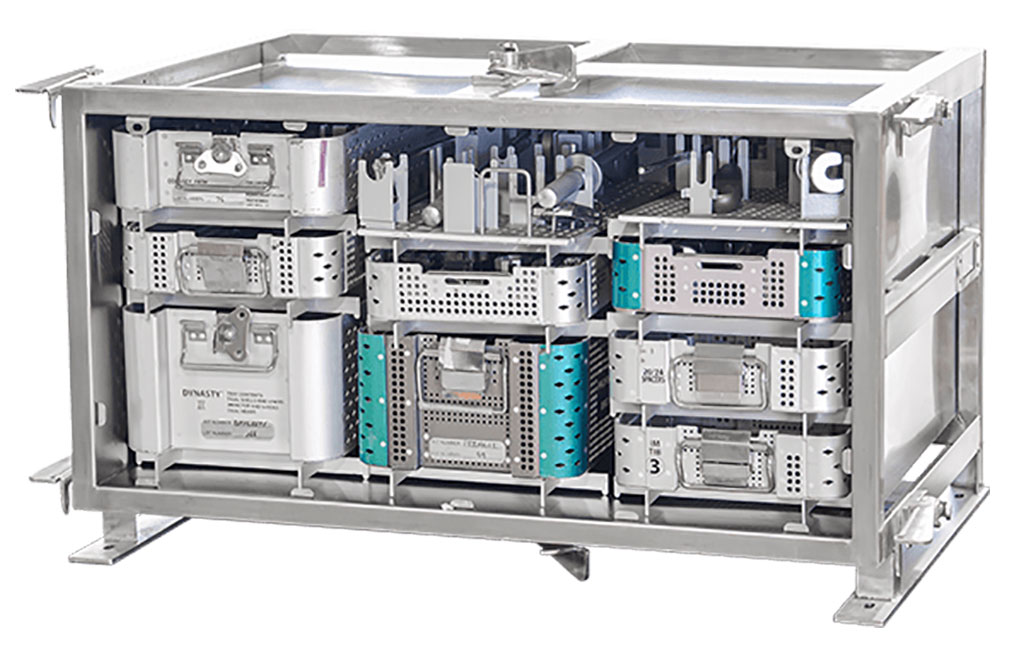
Game-Changing Innovation in Surgical Instrument Sterilization Significantly Improves OR Throughput
A groundbreaking innovation enables hospitals to significantly improve instrument processing time and throughput in operating rooms (ORs) and sterile processing departments. Turbett Surgical, Inc.... Read moreHealth IT
view channel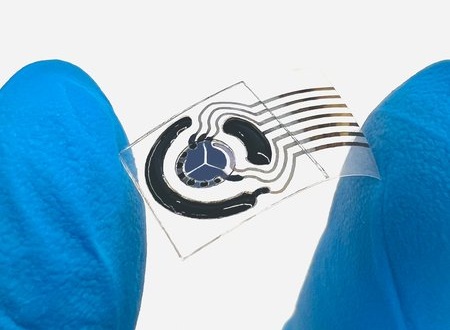
Printable Molecule-Selective Nanoparticles Enable Mass Production of Wearable Biosensors
The future of medicine is likely to focus on the personalization of healthcare—understanding exactly what an individual requires and delivering the appropriate combination of nutrients, metabolites, and... Read more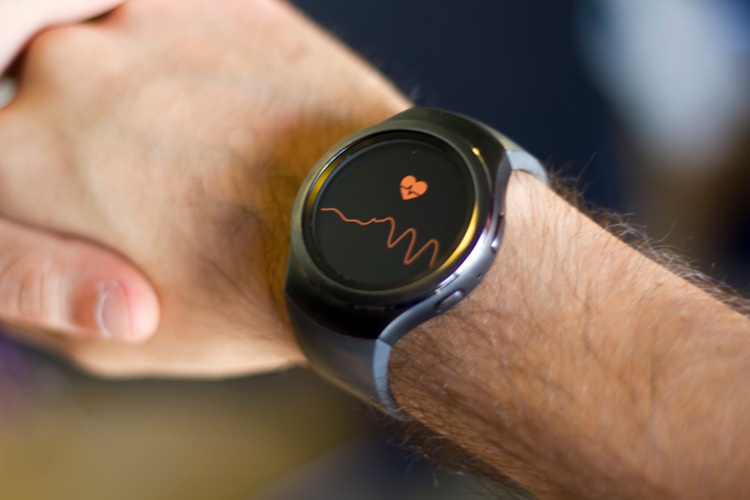
Smartwatches Could Detect Congestive Heart Failure
Diagnosing congestive heart failure (CHF) typically requires expensive and time-consuming imaging techniques like echocardiography, also known as cardiac ultrasound. Previously, detecting CHF by analyzing... Read morePoint of Care
view channel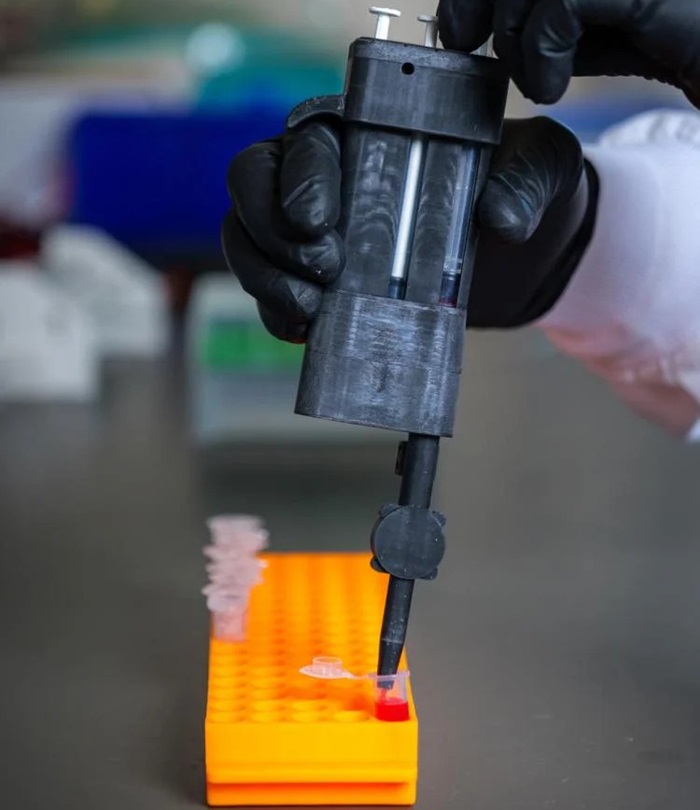
Handheld, Sound-Based Diagnostic System Delivers Bedside Blood Test Results in An Hour
Patients who go to a doctor for a blood test often have to contend with a needle and syringe, followed by a long wait—sometimes hours or even days—for lab results. Scientists have been working hard to... Read moreBusiness
view channel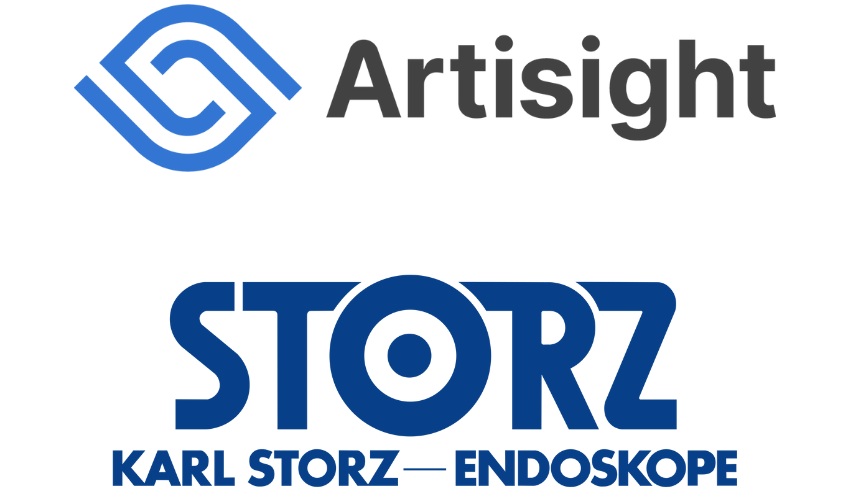
Expanded Collaboration to Transform OR Technology Through AI and Automation
The expansion of an existing collaboration between three leading companies aims to develop artificial intelligence (AI)-driven solutions for smart operating rooms with sophisticated monitoring and automation.... Read more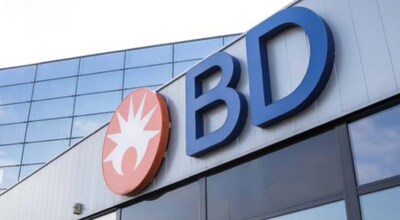