New Artificial Intelligence Method Helps Design Better COVID-19 Antibody Drugs
By HospiMedica International staff writers Posted on 20 Apr 2021 |
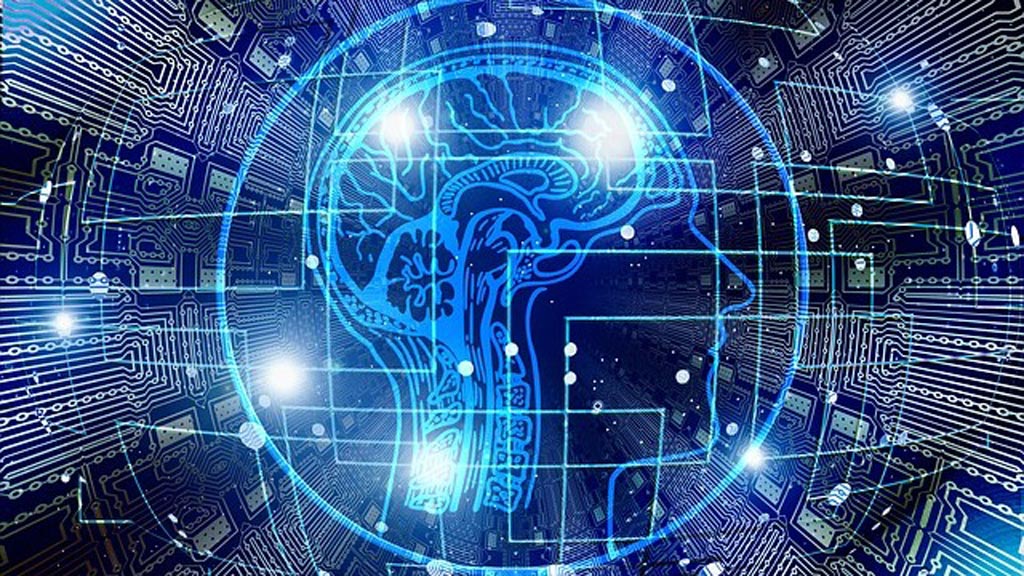
Illustration
Machine learning methods can help to optimize the development of COVID-19 antibody drugs, leading to active substances with improved properties, also with regard to tolerability in the body, according to researchers.
Scientists at ETH Zürich (Zürich, Switzerland) have developed a machine learning method that supports the optimization phase, helping to develop more effective antibody drugs. Antibodies are not only produced by our immune cells to fight viruses and other pathogens in the body. For a few decades now, medicine has also been using antibodies produced by biotechnology as drugs. This is because antibodies are extremely good at binding specifically to molecular structures according to the lock-and-key principle. Their use ranges from oncology to the treatment of autoimmune diseases and neurodegenerative conditions.
However, developing such antibody drugs is anything but simple. The basic requirement is for an antibody to bind to its target molecule in an optimal way. At the same time, an antibody drug must fulfill a host of additional criteria. For example, it should not trigger an immune response in the body, it should be efficient to produce using biotechnology, and it should remain stable over a long period of time. Once scientists have found an antibody that binds to the desired molecular target structure, the development process is far from over. Rather, this marks the start of a phase in which researchers use bioengineering to try to improve the antibody’s properties.
When researchers optimize an entire antibody molecule in its therapeutic form (i.e. not just a fragment of an antibody), it used to start with an antibody lead candidate that binds reasonably well to the desired target structure. Then researchers randomly mutate the gene that carries the blueprint for the antibody in order to produce a few thousand related antibody candidates in the lab. The next step is to search among them to find the ones that bind best to the target structure. The ETH researchers are now using machine learning to increase the initial set of antibodies to be tested to several million.
The researchers provided the proof of concept for their new method using Roche’s antibody cancer drug Herceptin, which has been on the market for 20 years. Starting out from the DNA sequence of the Herceptin antibody, the ETH researchers created about 40,000 related antibodies using a CRISPR mutation method they developed a few years ago. Experiments showed that 10,000 of them bound well to the target protein in question, a specific cell surface protein. The scientists used the DNA sequences of these 40,000 antibodies to train a machine learning algorithm. They then applied the trained algorithm to search a database of 70 million potential antibody DNA sequences. For these 70 million candidates, the algorithm predicted how well the corresponding antibodies would bind to the target protein, resulting in a list of millions of sequences expected to bind.
Using further computer models, the scientists predicted how well these millions of sequences would meet the additional criteria for drug development (tolerance, production, physical properties). This reduced the number of candidate sequences to 8,000. From the list of optimized candidate sequences on their computer, the scientists selected 55 sequences from which to produce antibodies in the lab and characterize their properties. Subsequent experiments showed that several of them bound even better to the target protein than Herceptin itself, as well as being easier to produce and more stable than Herceptin. The ETH scientists are now applying their AI method to optimize antibody drugs that are in clinical development.
“With automated processes, you can test a few thousand therapeutic candidates in a lab. But it is not really feasible to screen any more than that,” said Sai Reddy, a professor at the Department of Biosystems Science and Engineering at ETH Zurich who led the study. “Typically, the best dozen antibodies from this screening move on to the next step and are tested for how well they meet additional criteria. “Ultimately, this approach lets you identify the best antibody from a group of a few thousand.”
Related Links:
ETH Zürich
Scientists at ETH Zürich (Zürich, Switzerland) have developed a machine learning method that supports the optimization phase, helping to develop more effective antibody drugs. Antibodies are not only produced by our immune cells to fight viruses and other pathogens in the body. For a few decades now, medicine has also been using antibodies produced by biotechnology as drugs. This is because antibodies are extremely good at binding specifically to molecular structures according to the lock-and-key principle. Their use ranges from oncology to the treatment of autoimmune diseases and neurodegenerative conditions.
However, developing such antibody drugs is anything but simple. The basic requirement is for an antibody to bind to its target molecule in an optimal way. At the same time, an antibody drug must fulfill a host of additional criteria. For example, it should not trigger an immune response in the body, it should be efficient to produce using biotechnology, and it should remain stable over a long period of time. Once scientists have found an antibody that binds to the desired molecular target structure, the development process is far from over. Rather, this marks the start of a phase in which researchers use bioengineering to try to improve the antibody’s properties.
When researchers optimize an entire antibody molecule in its therapeutic form (i.e. not just a fragment of an antibody), it used to start with an antibody lead candidate that binds reasonably well to the desired target structure. Then researchers randomly mutate the gene that carries the blueprint for the antibody in order to produce a few thousand related antibody candidates in the lab. The next step is to search among them to find the ones that bind best to the target structure. The ETH researchers are now using machine learning to increase the initial set of antibodies to be tested to several million.
The researchers provided the proof of concept for their new method using Roche’s antibody cancer drug Herceptin, which has been on the market for 20 years. Starting out from the DNA sequence of the Herceptin antibody, the ETH researchers created about 40,000 related antibodies using a CRISPR mutation method they developed a few years ago. Experiments showed that 10,000 of them bound well to the target protein in question, a specific cell surface protein. The scientists used the DNA sequences of these 40,000 antibodies to train a machine learning algorithm. They then applied the trained algorithm to search a database of 70 million potential antibody DNA sequences. For these 70 million candidates, the algorithm predicted how well the corresponding antibodies would bind to the target protein, resulting in a list of millions of sequences expected to bind.
Using further computer models, the scientists predicted how well these millions of sequences would meet the additional criteria for drug development (tolerance, production, physical properties). This reduced the number of candidate sequences to 8,000. From the list of optimized candidate sequences on their computer, the scientists selected 55 sequences from which to produce antibodies in the lab and characterize their properties. Subsequent experiments showed that several of them bound even better to the target protein than Herceptin itself, as well as being easier to produce and more stable than Herceptin. The ETH scientists are now applying their AI method to optimize antibody drugs that are in clinical development.
“With automated processes, you can test a few thousand therapeutic candidates in a lab. But it is not really feasible to screen any more than that,” said Sai Reddy, a professor at the Department of Biosystems Science and Engineering at ETH Zurich who led the study. “Typically, the best dozen antibodies from this screening move on to the next step and are tested for how well they meet additional criteria. “Ultimately, this approach lets you identify the best antibody from a group of a few thousand.”
Related Links:
ETH Zürich
Latest COVID-19 News
- Low-Cost System Detects SARS-CoV-2 Virus in Hospital Air Using High-Tech Bubbles
- World's First Inhalable COVID-19 Vaccine Approved in China
- COVID-19 Vaccine Patch Fights SARS-CoV-2 Variants Better than Needles
- Blood Viscosity Testing Can Predict Risk of Death in Hospitalized COVID-19 Patients
- ‘Covid Computer’ Uses AI to Detect COVID-19 from Chest CT Scans
- MRI Lung-Imaging Technique Shows Cause of Long-COVID Symptoms
- Chest CT Scans of COVID-19 Patients Could Help Distinguish Between SARS-CoV-2 Variants
- Specialized MRI Detects Lung Abnormalities in Non-Hospitalized Long COVID Patients
- AI Algorithm Identifies Hospitalized Patients at Highest Risk of Dying From COVID-19
- Sweat Sensor Detects Key Biomarkers That Provide Early Warning of COVID-19 and Flu
- Study Assesses Impact of COVID-19 on Ventilation/Perfusion Scintigraphy
- CT Imaging Study Finds Vaccination Reduces Risk of COVID-19 Associated Pulmonary Embolism
- Third Day in Hospital a ‘Tipping Point’ in Severity of COVID-19 Pneumonia
- Longer Interval Between COVID-19 Vaccines Generates Up to Nine Times as Many Antibodies
- AI Model for Monitoring COVID-19 Predicts Mortality Within First 30 Days of Admission
- AI Predicts COVID Prognosis at Near-Expert Level Based Off CT Scans
Channels
Critical Care
view channel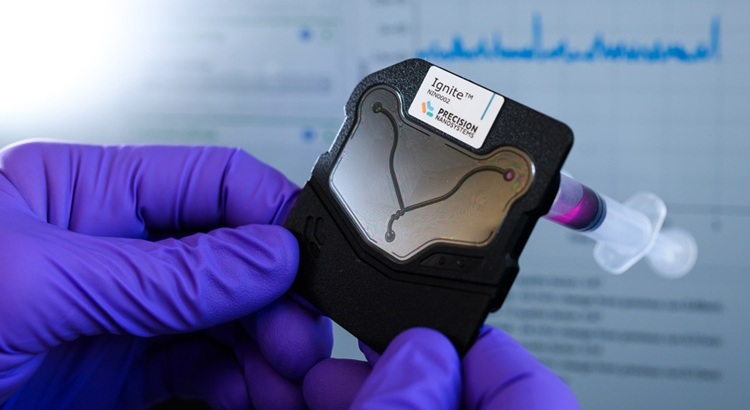
Mass Manufactured Nanoparticles to Deliver Cancer Drugs Directly to Tumors
Polymer-coated nanoparticles loaded with therapeutic drugs hold significant potential for treating cancers, including ovarian cancer. These particles can be precisely directed to tumors, delivering their... Read more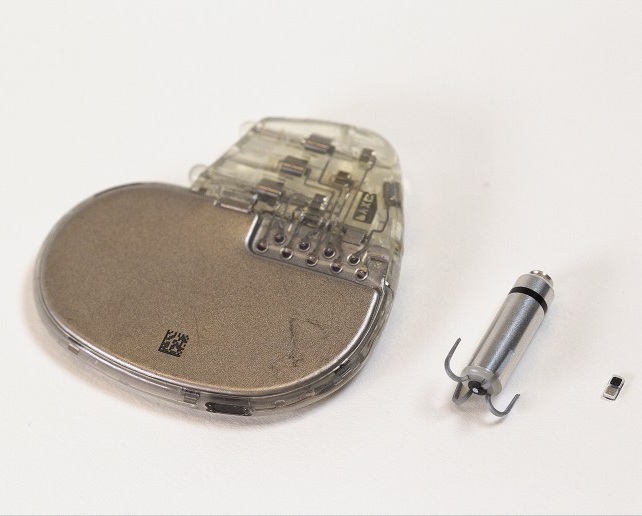
World’s Smallest Pacemaker Fits Inside Syringe Tip
After heart surgery, many patients require temporary pacemakers either to regulate the heart rate while waiting for a permanent pacemaker or to support normal heart rhythm during recovery.... Read more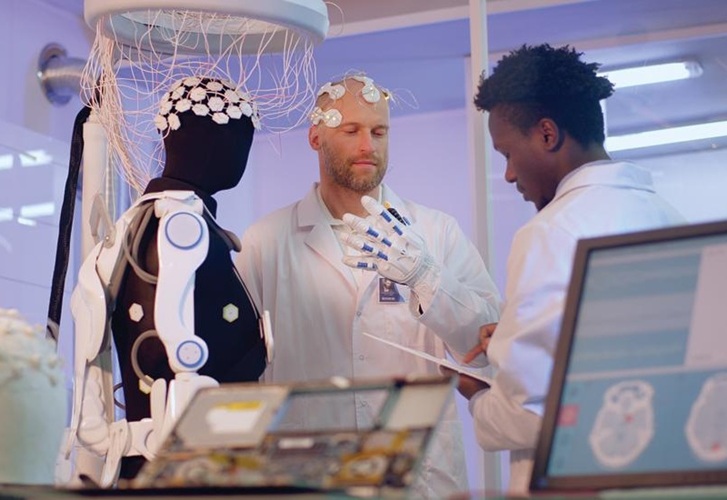
AI-Powered, Internet-Connected Medical Devices to Revolutionize Healthcare, Finds Study
A new study suggests that artificial intelligence (AI)-powered, internet-connected medical devices have the potential to transform healthcare by enabling earlier detection of diseases, real-time patient... Read moreSurgical Techniques
view channel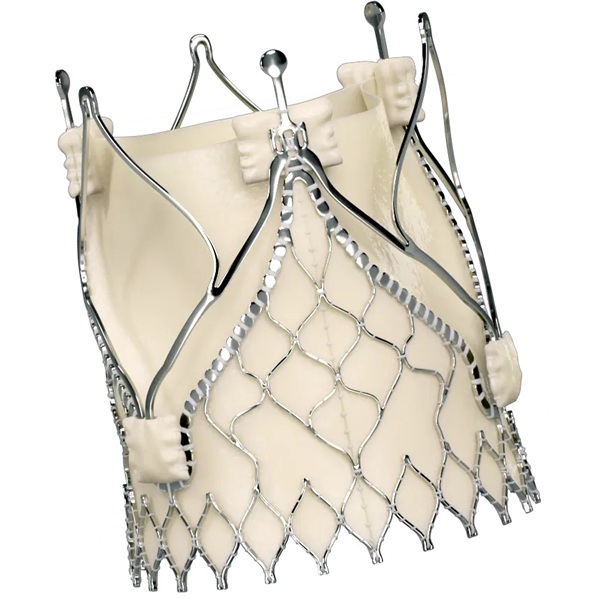
New Transcatheter Valve Found Safe and Effective for Treating Aortic Regurgitation
Aortic regurgitation is a condition in which the aortic valve does not close properly, allowing blood to flow backward into the left ventricle. This results in decreased blood flow from the heart to the... Read more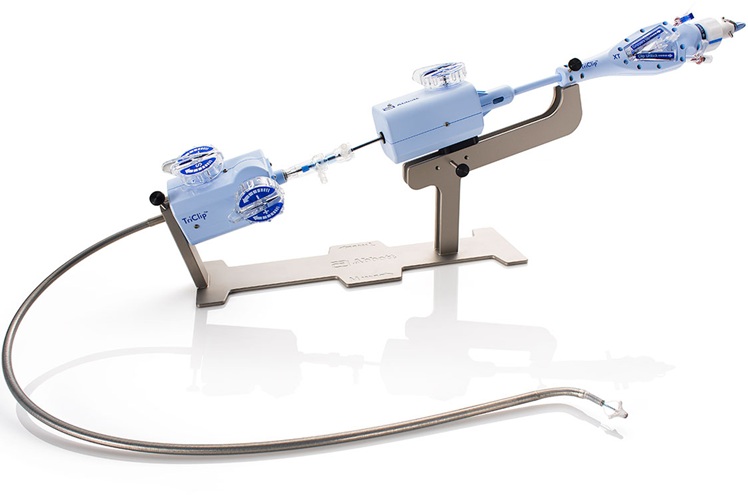
Minimally Invasive Valve Repair Reduces Hospitalizations in Severe Tricuspid Regurgitation Patients
The tricuspid valve is one of the four heart valves, responsible for regulating blood flow from the right atrium (the heart's upper-right chamber) to the right ventricle (the lower-right chamber).... Read morePatient Care
view channel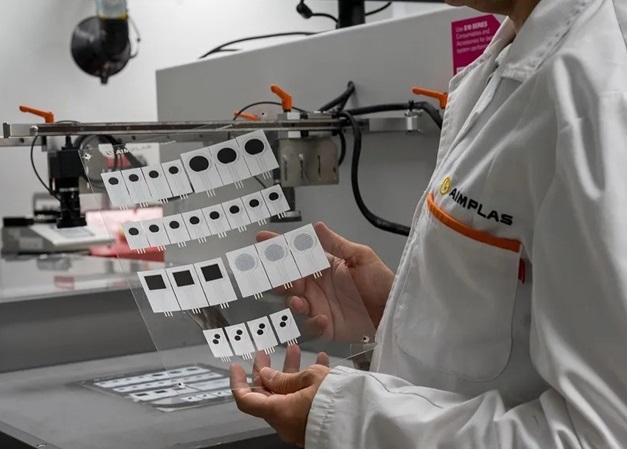
Portable Biosensor Platform to Reduce Hospital-Acquired Infections
Approximately 4 million patients in the European Union acquire healthcare-associated infections (HAIs) or nosocomial infections each year, with around 37,000 deaths directly resulting from these infections,... Read more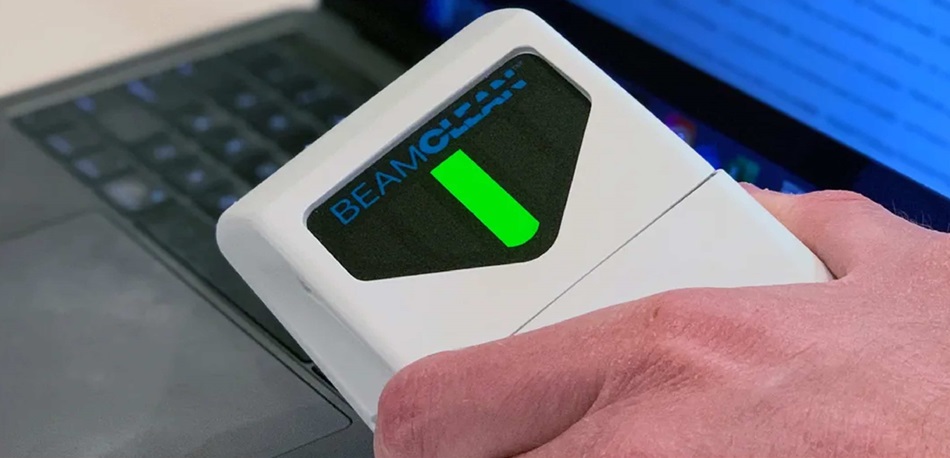
First-Of-Its-Kind Portable Germicidal Light Technology Disinfects High-Touch Clinical Surfaces in Seconds
Reducing healthcare-acquired infections (HAIs) remains a pressing issue within global healthcare systems. In the United States alone, 1.7 million patients contract HAIs annually, leading to approximately... Read more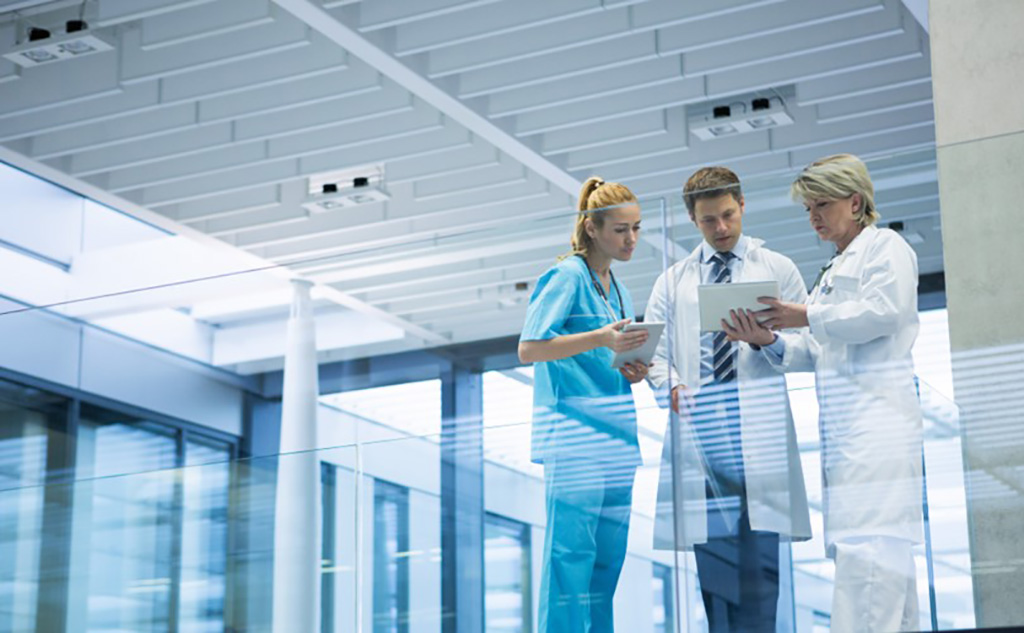
Surgical Capacity Optimization Solution Helps Hospitals Boost OR Utilization
An innovative solution has the capability to transform surgical capacity utilization by targeting the root cause of surgical block time inefficiencies. Fujitsu Limited’s (Tokyo, Japan) Surgical Capacity... Read more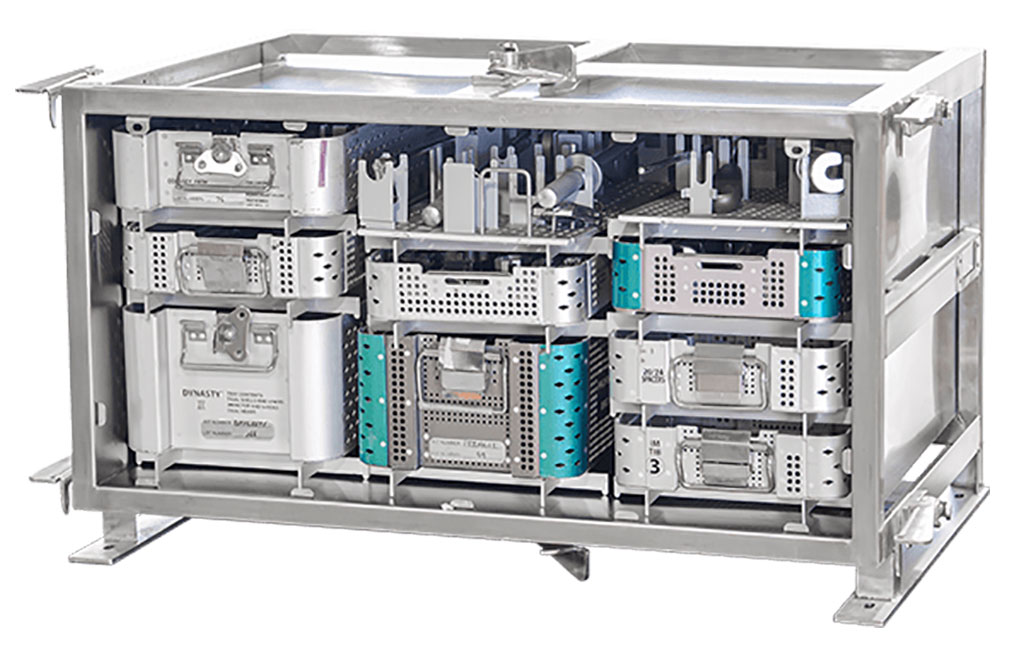
Game-Changing Innovation in Surgical Instrument Sterilization Significantly Improves OR Throughput
A groundbreaking innovation enables hospitals to significantly improve instrument processing time and throughput in operating rooms (ORs) and sterile processing departments. Turbett Surgical, Inc.... Read moreHealth IT
view channel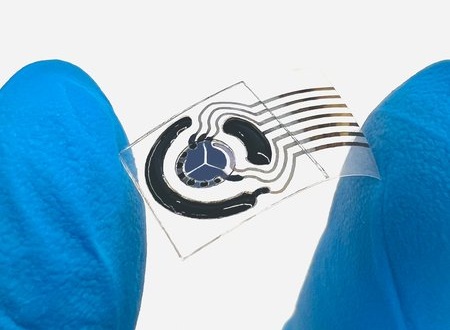
Printable Molecule-Selective Nanoparticles Enable Mass Production of Wearable Biosensors
The future of medicine is likely to focus on the personalization of healthcare—understanding exactly what an individual requires and delivering the appropriate combination of nutrients, metabolites, and... Read more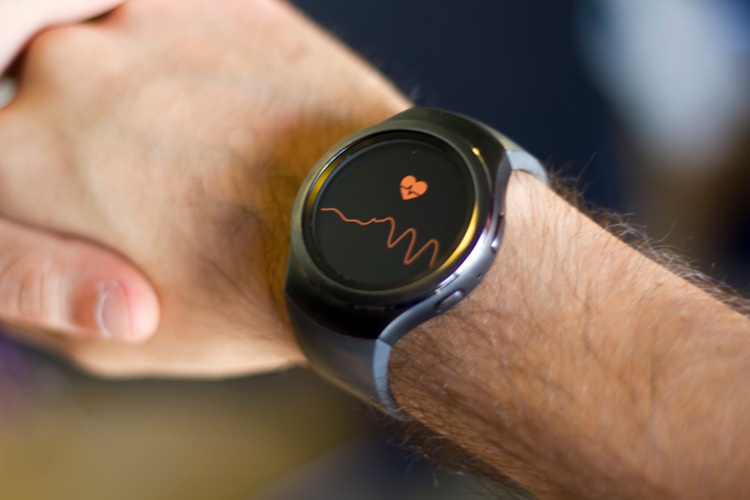
Smartwatches Could Detect Congestive Heart Failure
Diagnosing congestive heart failure (CHF) typically requires expensive and time-consuming imaging techniques like echocardiography, also known as cardiac ultrasound. Previously, detecting CHF by analyzing... Read morePoint of Care
view channel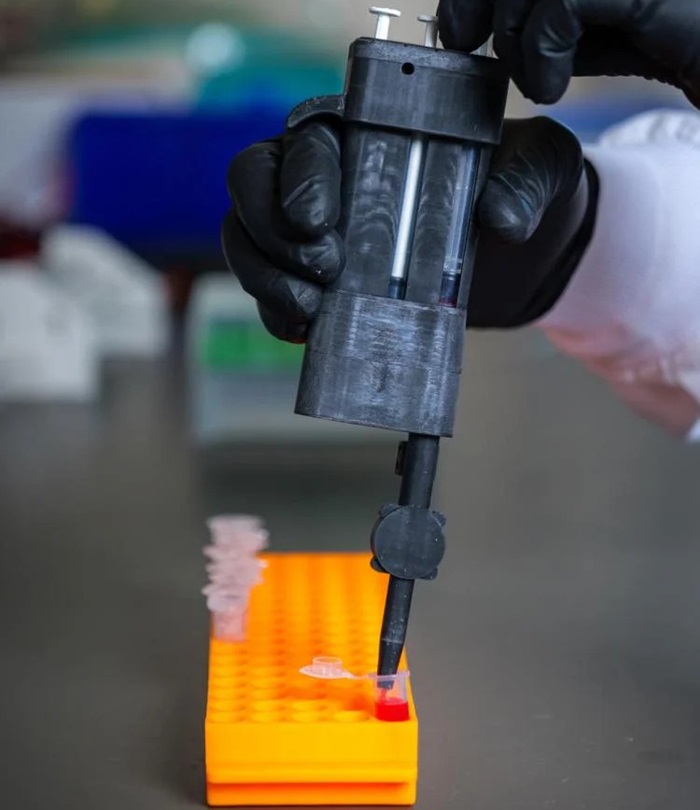
Handheld, Sound-Based Diagnostic System Delivers Bedside Blood Test Results in An Hour
Patients who go to a doctor for a blood test often have to contend with a needle and syringe, followed by a long wait—sometimes hours or even days—for lab results. Scientists have been working hard to... Read moreBusiness
view channel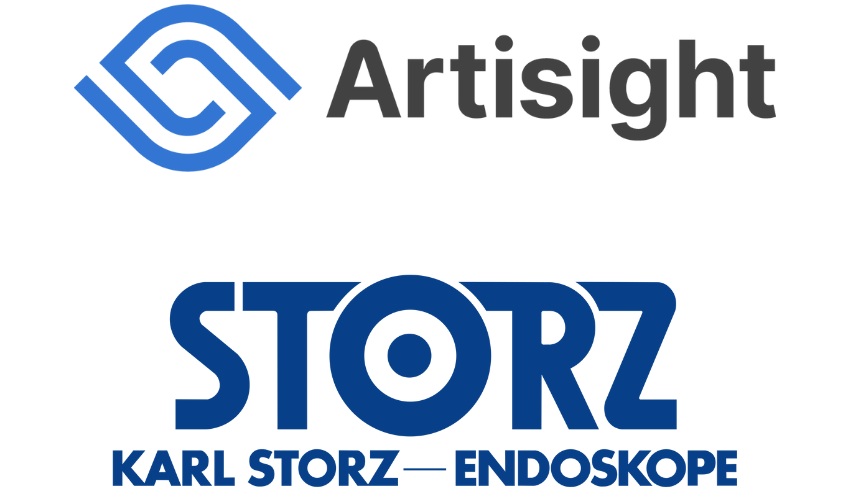
Expanded Collaboration to Transform OR Technology Through AI and Automation
The expansion of an existing collaboration between three leading companies aims to develop artificial intelligence (AI)-driven solutions for smart operating rooms with sophisticated monitoring and automation.... Read more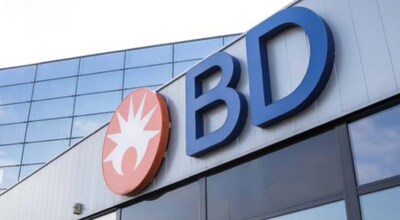