Unsupervised AI Model Accurately Predicts COVID-19 Patient's Survival Based on Chest CT Exams
By HospiMedica International staff writers Posted on 08 Aug 2021 |
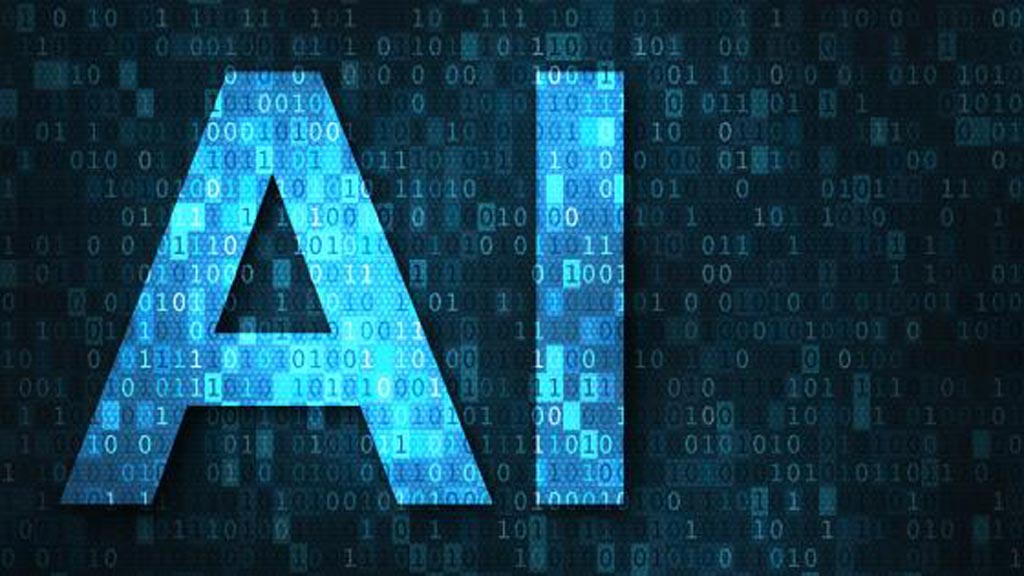
Illustration
An "unsupervised" artificial intelligence (AI) model, or one trained without image annotations, can accurately predict the survival of COVID-19 patients on the basis of their chest computed tomography (CT) exams.
Researchers from Massachusetts General Hospital (Boston, MA, USA) have shown that the performance of their pix2surv algorithm based on CT images significantly outperformed those of existing laboratory tests and image-based visual and quantitative predictors in estimating the disease progression and mortality of COVID-19 patients. Thus, pix2surv offers a promising approach for performing image-based prognostic predictions.
Because of the rapid spread and wide range of the clinical manifestations of the coronavirus disease 2019 (COVID-19), fast and accurate estimation of the disease progression and mortality is vital for the management of the patients. Currently available image-based prognostic predictors for patients with COVID-19 are largely limited to semi-automated schemes with manually designed features and supervised learning, and the survival analysis is largely limited to logistic regression. To resolve this problem, the researchers developed a weakly unsupervised conditional generative adversarial network, called pix2surv, which can be trained to estimate the time-to-event information for survival analysis directly from the chest CT images of a patient.
pix2surv enables the estimation of the distribution of the survival time directly from the chest CT images of patients. The model avoids the technical limitations of the previous image-based COVID-19 predictors, because the use of a fully automated conditional GAN makes it possible to train a complete image-based end-to-end survival analysis model for producing the time-to-event distribution directly from input chest CT images without an explicit segmentation or feature extraction efforts. Also, because of the use of weakly unsupervised learning, the annotation effort is reduced to the pairing of input training CT images with the corresponding observed survival time of the patient.
In their study the researchers showed that the prognostic performance of pix2surv based on chest CT images compares favorably with those of currently available laboratory tests and existing image-based visual and quantitative predictors in the estimation of the disease progression and mortality of COVID-19 patients. They also showed that the time-to-event information calculated by pix2surv based on chest CT images enables stratification of the patients into low- and high-risk groups by a wider margin than those of the other predictors. Thus, pix2surv offers a promising approach for performing image-based prognostic prediction for the management of COVID-19 patients.
Related Links:
Researchers from Massachusetts General Hospital (Boston, MA, USA) have shown that the performance of their pix2surv algorithm based on CT images significantly outperformed those of existing laboratory tests and image-based visual and quantitative predictors in estimating the disease progression and mortality of COVID-19 patients. Thus, pix2surv offers a promising approach for performing image-based prognostic predictions.
Because of the rapid spread and wide range of the clinical manifestations of the coronavirus disease 2019 (COVID-19), fast and accurate estimation of the disease progression and mortality is vital for the management of the patients. Currently available image-based prognostic predictors for patients with COVID-19 are largely limited to semi-automated schemes with manually designed features and supervised learning, and the survival analysis is largely limited to logistic regression. To resolve this problem, the researchers developed a weakly unsupervised conditional generative adversarial network, called pix2surv, which can be trained to estimate the time-to-event information for survival analysis directly from the chest CT images of a patient.
pix2surv enables the estimation of the distribution of the survival time directly from the chest CT images of patients. The model avoids the technical limitations of the previous image-based COVID-19 predictors, because the use of a fully automated conditional GAN makes it possible to train a complete image-based end-to-end survival analysis model for producing the time-to-event distribution directly from input chest CT images without an explicit segmentation or feature extraction efforts. Also, because of the use of weakly unsupervised learning, the annotation effort is reduced to the pairing of input training CT images with the corresponding observed survival time of the patient.
In their study the researchers showed that the prognostic performance of pix2surv based on chest CT images compares favorably with those of currently available laboratory tests and existing image-based visual and quantitative predictors in the estimation of the disease progression and mortality of COVID-19 patients. They also showed that the time-to-event information calculated by pix2surv based on chest CT images enables stratification of the patients into low- and high-risk groups by a wider margin than those of the other predictors. Thus, pix2surv offers a promising approach for performing image-based prognostic prediction for the management of COVID-19 patients.
Related Links:
Latest COVID-19 News
- Low-Cost System Detects SARS-CoV-2 Virus in Hospital Air Using High-Tech Bubbles
- World's First Inhalable COVID-19 Vaccine Approved in China
- COVID-19 Vaccine Patch Fights SARS-CoV-2 Variants Better than Needles
- Blood Viscosity Testing Can Predict Risk of Death in Hospitalized COVID-19 Patients
- ‘Covid Computer’ Uses AI to Detect COVID-19 from Chest CT Scans
- MRI Lung-Imaging Technique Shows Cause of Long-COVID Symptoms
- Chest CT Scans of COVID-19 Patients Could Help Distinguish Between SARS-CoV-2 Variants
- Specialized MRI Detects Lung Abnormalities in Non-Hospitalized Long COVID Patients
- AI Algorithm Identifies Hospitalized Patients at Highest Risk of Dying From COVID-19
- Sweat Sensor Detects Key Biomarkers That Provide Early Warning of COVID-19 and Flu
- Study Assesses Impact of COVID-19 on Ventilation/Perfusion Scintigraphy
- CT Imaging Study Finds Vaccination Reduces Risk of COVID-19 Associated Pulmonary Embolism
- Third Day in Hospital a ‘Tipping Point’ in Severity of COVID-19 Pneumonia
- Longer Interval Between COVID-19 Vaccines Generates Up to Nine Times as Many Antibodies
- AI Model for Monitoring COVID-19 Predicts Mortality Within First 30 Days of Admission
- AI Predicts COVID Prognosis at Near-Expert Level Based Off CT Scans
Channels
Artificial Intelligence
view channel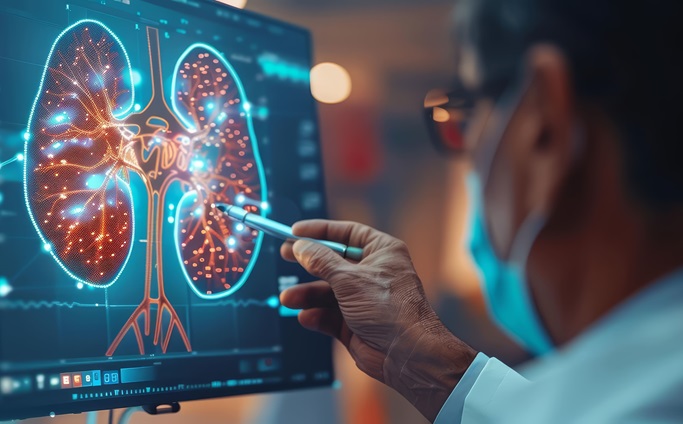
Innovative Risk Score Predicts Heart Attack or Stroke in Kidney Transplant Candidates
Heart researchers have utilized an innovative risk assessment score to accurately predict whether patients being evaluated for kidney transplants are at risk for future major cardiac events, such as a... Read more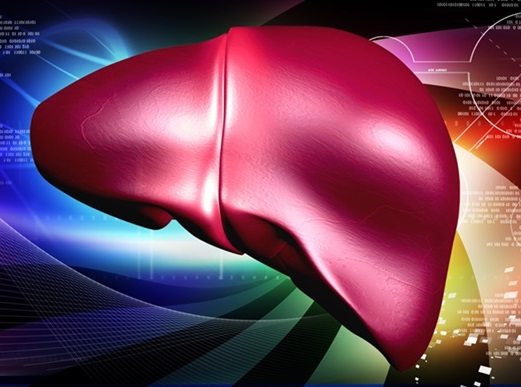
AI Algorithm Detects Early-Stage Metabolic-Associated Steatotic Liver Disease Using EHRs
Liver disease, which is treatable when detected early, often goes unnoticed until it reaches advanced stages. Metabolic-associated steatotic liver disease (MASLD), the most prevalent form of liver disease,... Read moreCritical Care
view channel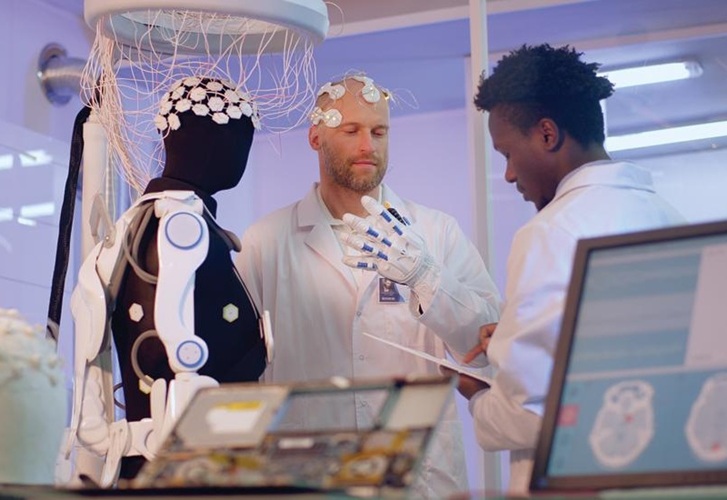
AI-Powered, Internet-Connected Medical Devices to Revolutionize Healthcare, Finds Study
A new study suggests that artificial intelligence (AI)-powered, internet-connected medical devices have the potential to transform healthcare by enabling earlier detection of diseases, real-time patient... Read more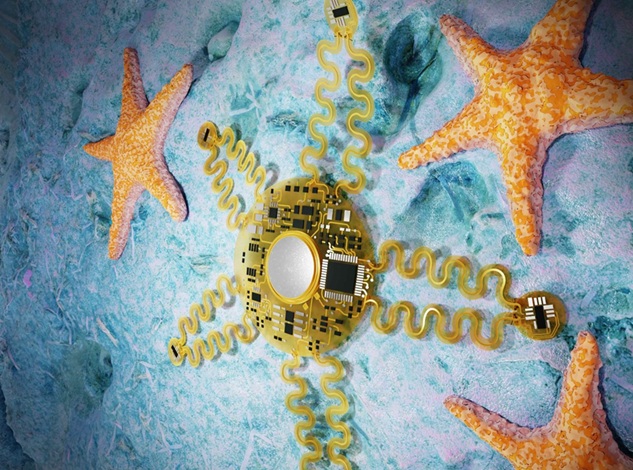
Starfish-Inspired Wearable Tech Enables Smarter Heart Monitoring
Physical movement can make it challenging for current wearable devices to accurately track heart activity. Now, a starfish’s five-arm shape has helped resolve this issue. Inspired by the starfish's ability... Read moreSurgical Techniques
view channel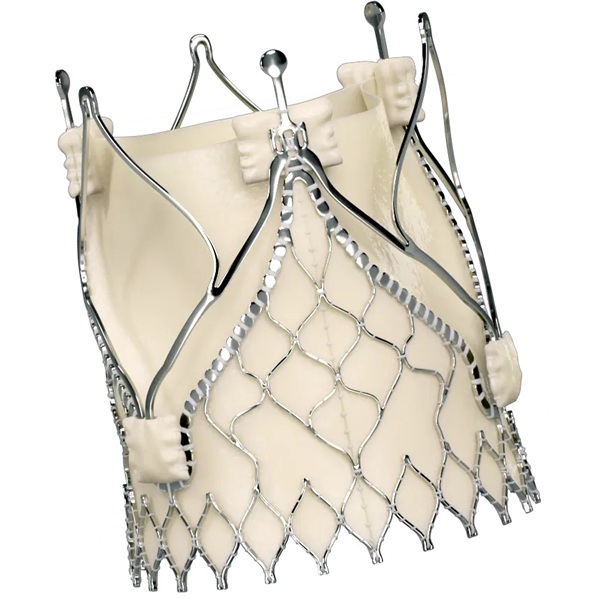
New Transcatheter Valve Found Safe and Effective for Treating Aortic Regurgitation
Aortic regurgitation is a condition in which the aortic valve does not close properly, allowing blood to flow backward into the left ventricle. This results in decreased blood flow from the heart to the... Read more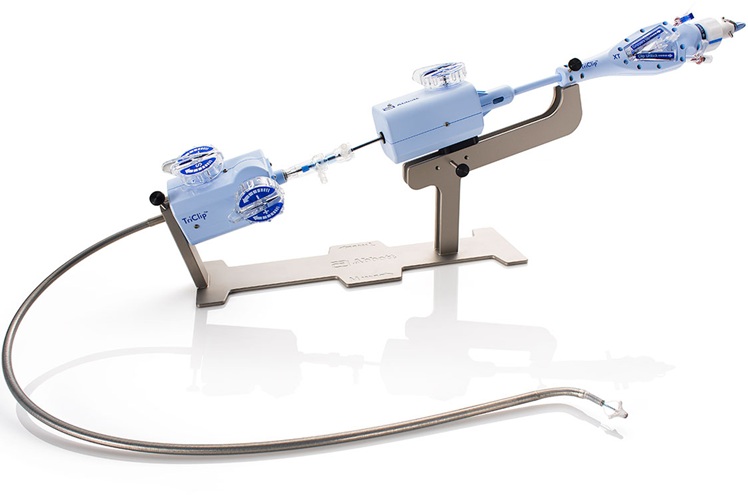
Minimally Invasive Valve Repair Reduces Hospitalizations in Severe Tricuspid Regurgitation Patients
The tricuspid valve is one of the four heart valves, responsible for regulating blood flow from the right atrium (the heart's upper-right chamber) to the right ventricle (the lower-right chamber).... Read morePatient Care
view channel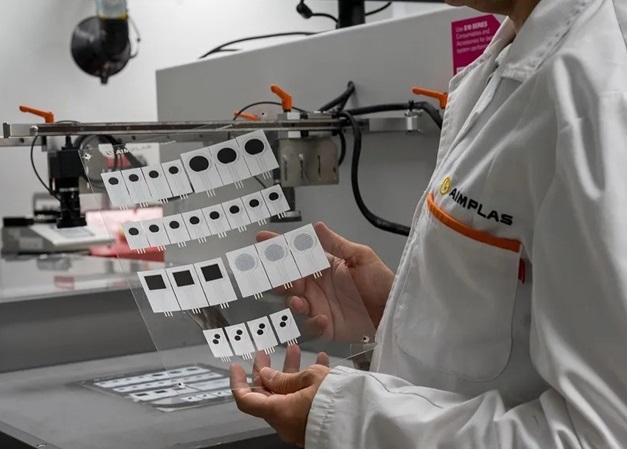
Portable Biosensor Platform to Reduce Hospital-Acquired Infections
Approximately 4 million patients in the European Union acquire healthcare-associated infections (HAIs) or nosocomial infections each year, with around 37,000 deaths directly resulting from these infections,... Read more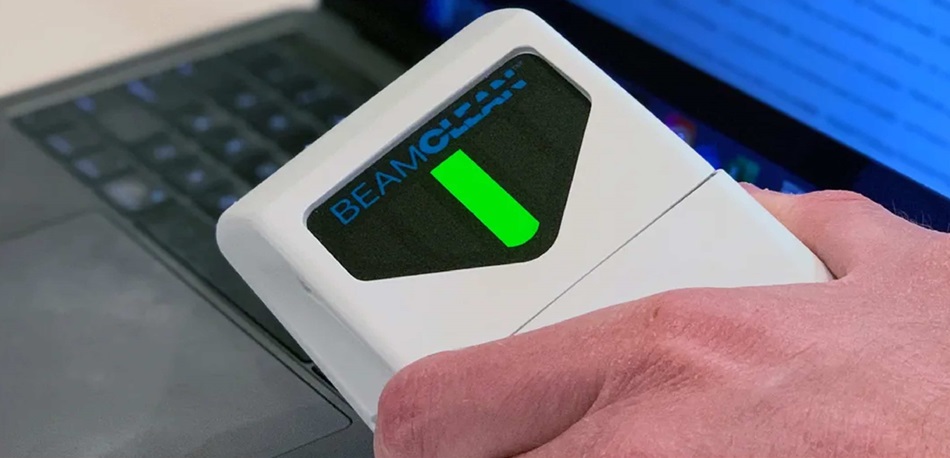
First-Of-Its-Kind Portable Germicidal Light Technology Disinfects High-Touch Clinical Surfaces in Seconds
Reducing healthcare-acquired infections (HAIs) remains a pressing issue within global healthcare systems. In the United States alone, 1.7 million patients contract HAIs annually, leading to approximately... Read more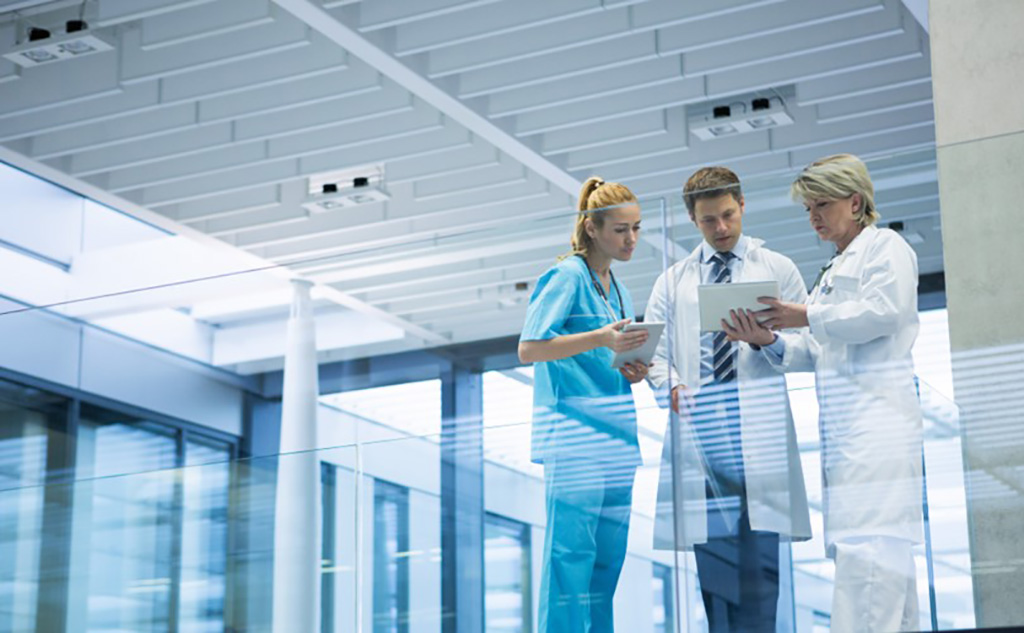
Surgical Capacity Optimization Solution Helps Hospitals Boost OR Utilization
An innovative solution has the capability to transform surgical capacity utilization by targeting the root cause of surgical block time inefficiencies. Fujitsu Limited’s (Tokyo, Japan) Surgical Capacity... Read more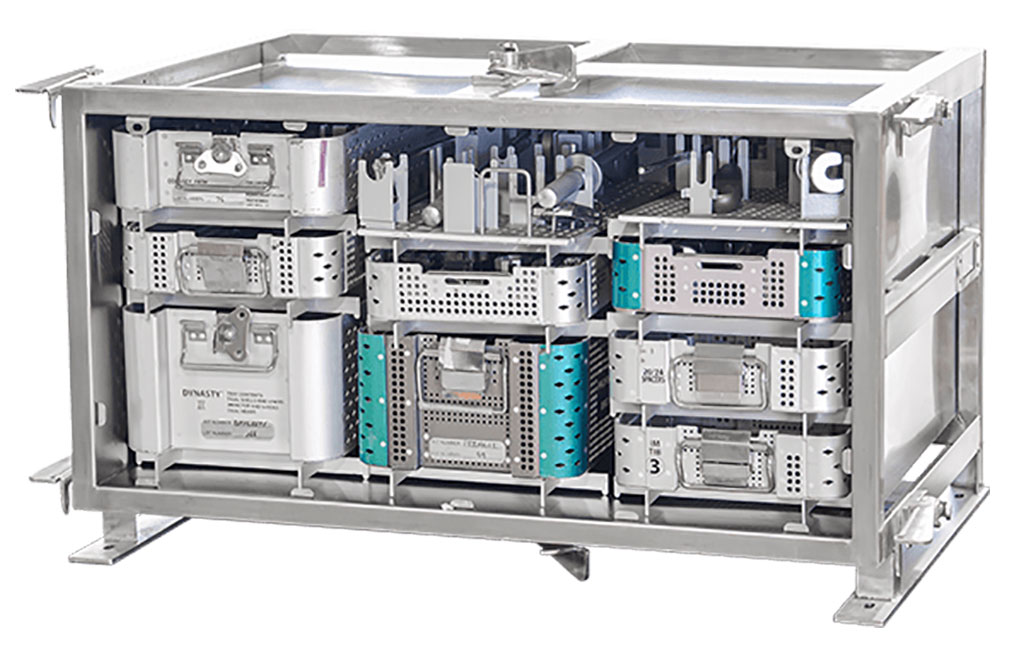
Game-Changing Innovation in Surgical Instrument Sterilization Significantly Improves OR Throughput
A groundbreaking innovation enables hospitals to significantly improve instrument processing time and throughput in operating rooms (ORs) and sterile processing departments. Turbett Surgical, Inc.... Read moreHealth IT
view channel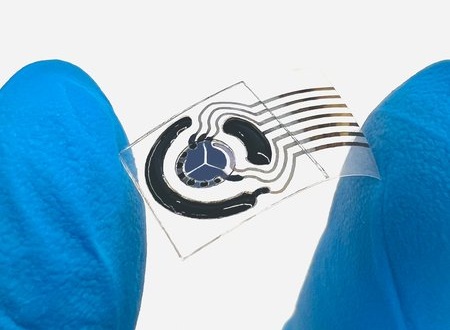
Printable Molecule-Selective Nanoparticles Enable Mass Production of Wearable Biosensors
The future of medicine is likely to focus on the personalization of healthcare—understanding exactly what an individual requires and delivering the appropriate combination of nutrients, metabolites, and... Read more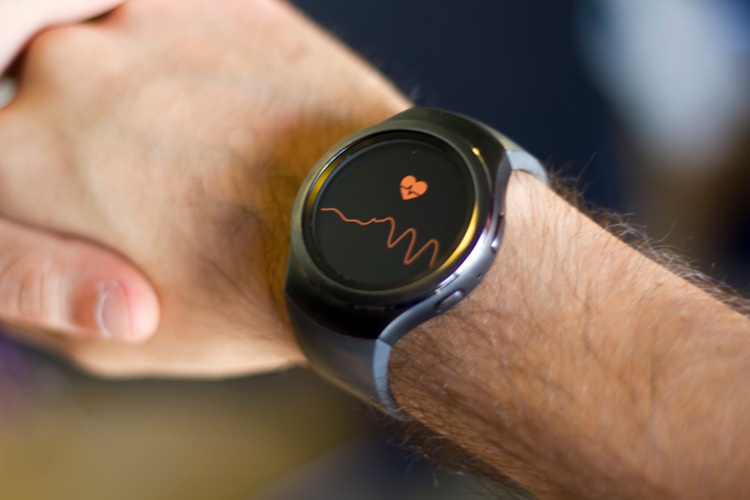
Smartwatches Could Detect Congestive Heart Failure
Diagnosing congestive heart failure (CHF) typically requires expensive and time-consuming imaging techniques like echocardiography, also known as cardiac ultrasound. Previously, detecting CHF by analyzing... Read morePoint of Care
view channel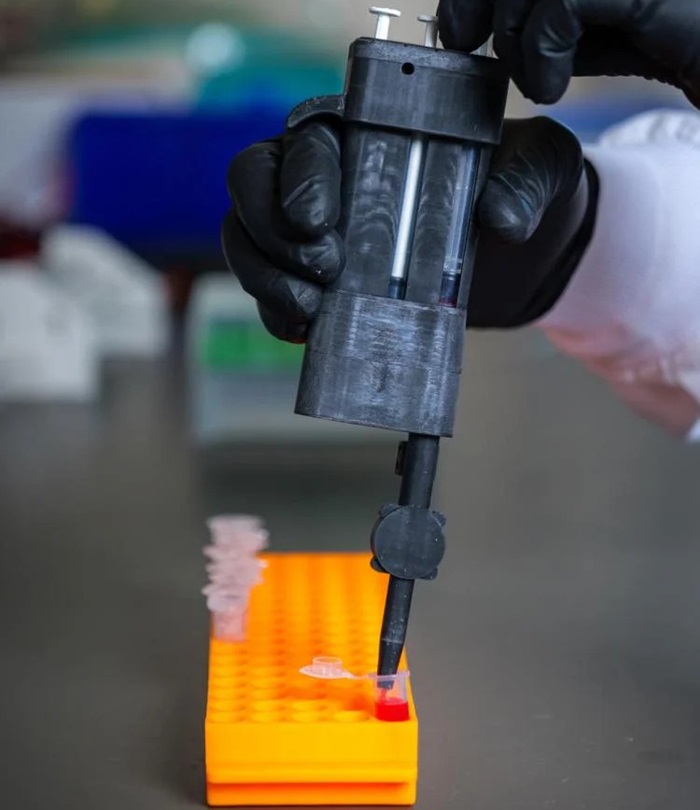
Handheld, Sound-Based Diagnostic System Delivers Bedside Blood Test Results in An Hour
Patients who go to a doctor for a blood test often have to contend with a needle and syringe, followed by a long wait—sometimes hours or even days—for lab results. Scientists have been working hard to... Read more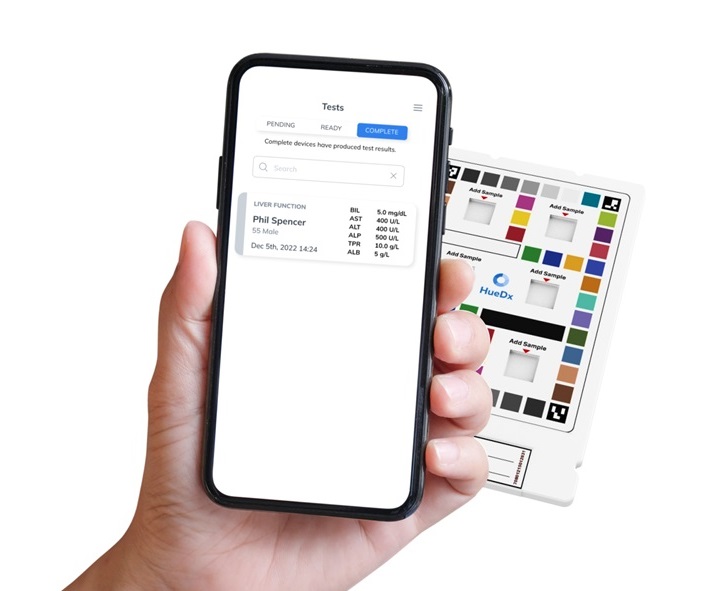
Smartphone-Enabled, Paper-Based Quantitative Diagnostic Platform Transforms POC Testing
Point-of-care diagnostics are crucial for public health, offering rapid, on-site testing that enables prompt diagnosis and treatment. This is especially valuable in remote or underserved regions where... Read moreBusiness
view channel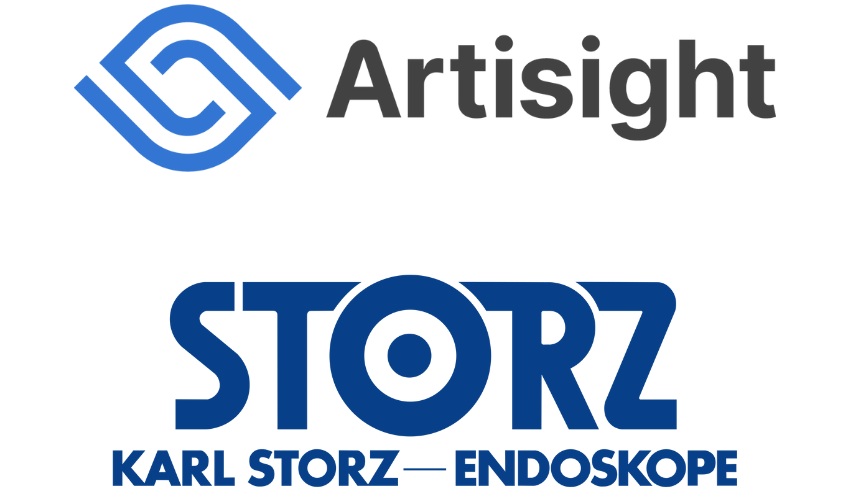
Expanded Collaboration to Transform OR Technology Through AI and Automation
The expansion of an existing collaboration between three leading companies aims to develop artificial intelligence (AI)-driven solutions for smart operating rooms with sophisticated monitoring and automation.... Read more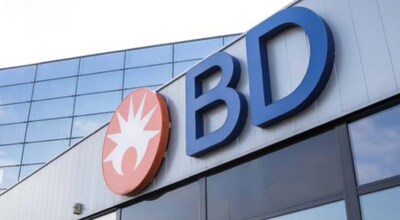