Electronic Triage Tool Improves ED Patient Care
By HospiMedica International staff writers Posted on 03 Oct 2017 |
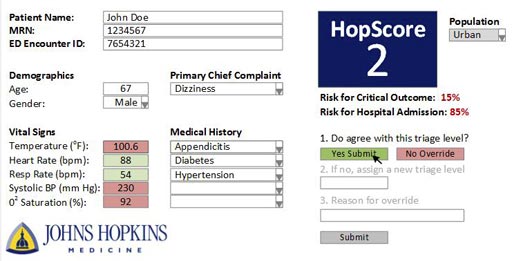
Image: The Johns Hopkins electronic triage tool interface (Photo courtesy of JHU).
Electronic triage (e-triage) systems classify emergency department (ED) patient’s injury severity more accurately and supports triage decision-making, claims a new study.
Researchers at Johns Hopkins University (JHU, Baltimore, MD, USA), StoCastic (Baltimore, MD, USA), and other institutions conducted a multi-site, retrospective, cross-sectional study of 172,726 visits to urban and community EDs in order to evaluate if an e-triage system composed of a random forest model of triage data--vital signs, chief complaint, and active medical history--could predict the need for critical care, an emergency procedure, and inpatient hospitalization in parallel, and translate the risk to triage level designations.
The predicted outcomes and secondary outcomes of the study were elevated levels of troponin and lactate, which were evaluated and compared with the standard Emergency Severity Index (ESI). The results showed significant differences in patient priority levels between e-triage and ESI evaluations. For example, out of the more than 65% of ED visits triaged to ESI Level 3, e-triage identified about 10% of patients who may have benefitted from being up-triaged to a more critical priority level, such as level 1 or 2.
The up-triaged ESI level 3 patients were at least five times more likely to experience a critical outcome--such as death, admission to the ICU, or emergency surgery--and twice as likely to be admitted to the hospital. The e-triage tool was also able to increase the number of patients down-triaged to a lower priority level, such as Level 4 or 5, helping to minimize low-acuity patients from waiting and overusing scarce resources. The study was published on September 6, 2017, in Annals of Emergency Medicine.
“The ultimate objective is that patients should be waiting less in the emergency department,” said lead author Scott Levin, PhD, of JHU. “For patients at risk of having a critical care need, this technology is designed to detect them better and make sure they are seen quicker. For patients who are less sick, e-triage should detect those patients and put them on an expedited track, so they don’t need to wait as long.”
“Machine-based learning takes full advantage of electronic health records and allows a precision of outcomes not previously realizable,” concluded senior author Professor Gabor Kelen, MD, director of the JHU department of emergency medicine. “It is the wave of future health care, although some providers may be hesitant. Decision aids that take advantage of machine-learning are also highly customizable to meet the needs of an emergency department's patient population and local health care delivery systems.”
Random forests are an ensemble learning method for classification, regression, and other tasks that operate by constructing a multitude of decision trees at training time and outputting the class that is the mode of the classes (classification) or mean prediction (regression) of the individual trees.
Related Links:
Johns Hopkins University
StoCastic
Researchers at Johns Hopkins University (JHU, Baltimore, MD, USA), StoCastic (Baltimore, MD, USA), and other institutions conducted a multi-site, retrospective, cross-sectional study of 172,726 visits to urban and community EDs in order to evaluate if an e-triage system composed of a random forest model of triage data--vital signs, chief complaint, and active medical history--could predict the need for critical care, an emergency procedure, and inpatient hospitalization in parallel, and translate the risk to triage level designations.
The predicted outcomes and secondary outcomes of the study were elevated levels of troponin and lactate, which were evaluated and compared with the standard Emergency Severity Index (ESI). The results showed significant differences in patient priority levels between e-triage and ESI evaluations. For example, out of the more than 65% of ED visits triaged to ESI Level 3, e-triage identified about 10% of patients who may have benefitted from being up-triaged to a more critical priority level, such as level 1 or 2.
The up-triaged ESI level 3 patients were at least five times more likely to experience a critical outcome--such as death, admission to the ICU, or emergency surgery--and twice as likely to be admitted to the hospital. The e-triage tool was also able to increase the number of patients down-triaged to a lower priority level, such as Level 4 or 5, helping to minimize low-acuity patients from waiting and overusing scarce resources. The study was published on September 6, 2017, in Annals of Emergency Medicine.
“The ultimate objective is that patients should be waiting less in the emergency department,” said lead author Scott Levin, PhD, of JHU. “For patients at risk of having a critical care need, this technology is designed to detect them better and make sure they are seen quicker. For patients who are less sick, e-triage should detect those patients and put them on an expedited track, so they don’t need to wait as long.”
“Machine-based learning takes full advantage of electronic health records and allows a precision of outcomes not previously realizable,” concluded senior author Professor Gabor Kelen, MD, director of the JHU department of emergency medicine. “It is the wave of future health care, although some providers may be hesitant. Decision aids that take advantage of machine-learning are also highly customizable to meet the needs of an emergency department's patient population and local health care delivery systems.”
Random forests are an ensemble learning method for classification, regression, and other tasks that operate by constructing a multitude of decision trees at training time and outputting the class that is the mode of the classes (classification) or mean prediction (regression) of the individual trees.
Related Links:
Johns Hopkins University
StoCastic
Latest Critical Care News
- AI Can Prioritize Emergecny Department Patients Requiring Urgent Treatment
- AI to Improve Diagnosis of Atrial Fibrillation
- Stretchable Microneedles to Help In Accurate Tracking of Abnormalities and Identifying Rapid Treatment
- Machine Learning Tool Identifies Rare, Undiagnosed Immune Disorders from Patient EHRs
- On-Skin Wearable Bioelectronic Device Paves Way for Intelligent Implants
- First-Of-Its-Kind Dissolvable Stent to Improve Outcomes for Patients with Severe PAD
- AI Brain-Age Estimation Technology Uses EEG Scans to Screen for Degenerative Diseases
- Wheeze-Counting Wearable Device Monitors Patient's Breathing In Real Time
- Wearable Multiplex Biosensors Could Revolutionize COPD Management
- New Low-Energy Defibrillation Method Controls Cardiac Arrhythmias
- New Machine Learning Models Help Predict Heart Disease Risk in Women
- Deep-Learning Model Predicts Arrhythmia 30 Minutes before Onset
- Breakthrough Technology Combines Detection and Treatment of Nerve-Related Disorders in Single Procedure
- Plasma Irradiation Promotes Faster Bone Healing
- New Device Treats Acute Kidney Injury from Sepsis
- Study Confirms Safety of DCB-Only Strategy for Treating De Novo Left Main Coronary Artery Disease
Channels
Artificial Intelligence
view channel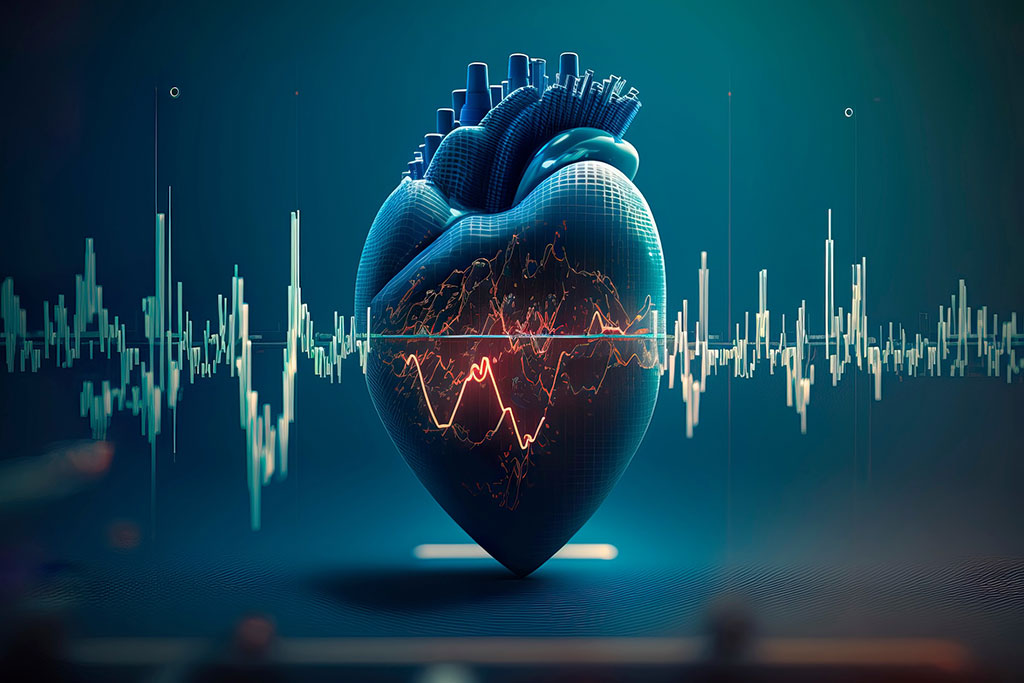
AI-Powered Algorithm to Revolutionize Detection of Atrial Fibrillation
Atrial fibrillation (AFib), a condition characterized by an irregular and often rapid heart rate, is linked to increased risks of stroke and heart failure. This is because the irregular heartbeat in AFib... Read more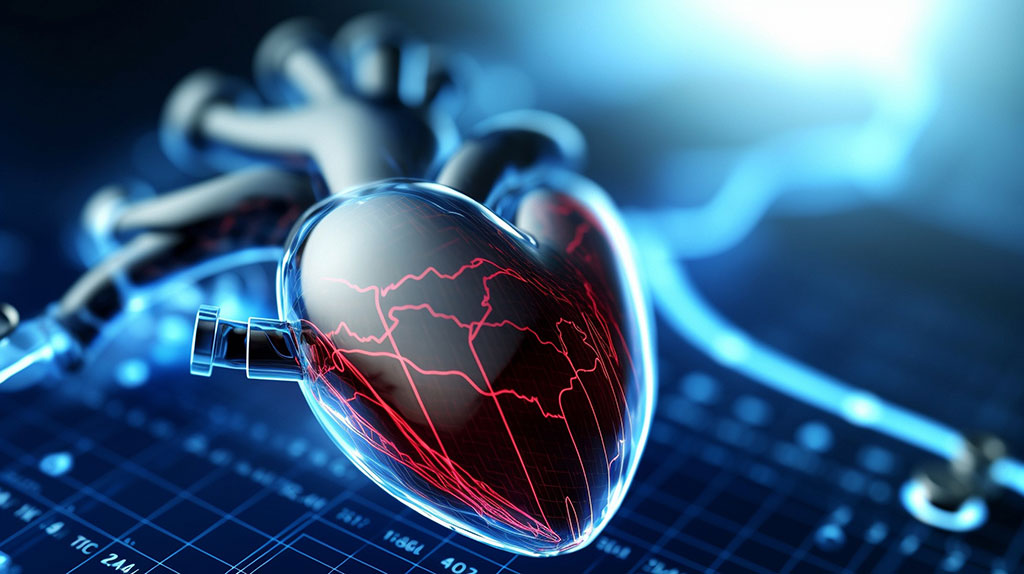
AI Diagnostic Tool Accurately Detects Valvular Disorders Often Missed by Doctors
Doctors generally use stethoscopes to listen for the characteristic lub-dub sounds made by heart valves opening and closing. They also listen for less prominent sounds that indicate problems with these valves.... Read moreSurgical Techniques
view channel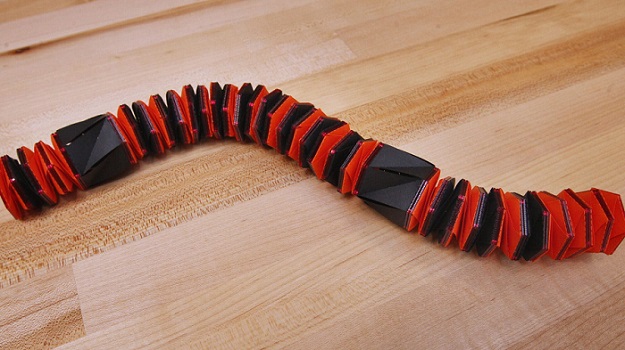
Caterpillar Robot with Built-In Steering System Crawls Easily Through Loops and Bends
Soft robots often face challenges in being guided effectively because adding steering mechanisms typically reduces their flexibility by increasing rigidity. Now, a team of engineers has combined ancient... Read more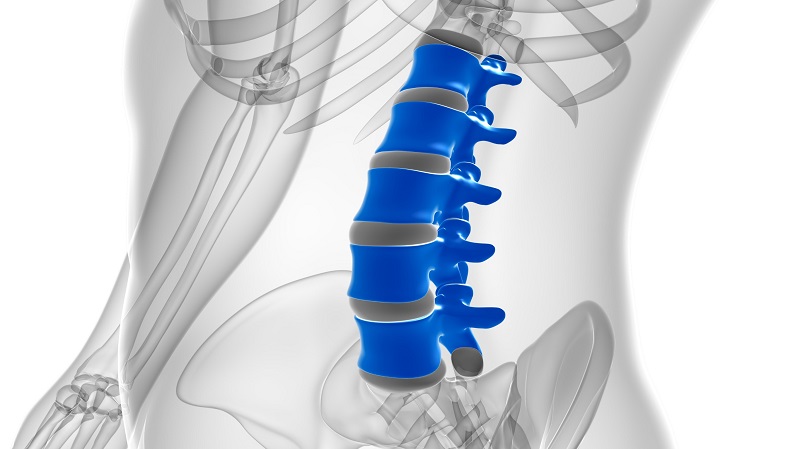
Tiny Wraparound Electronic Implants to Revolutionize Treatment of Spinal Cord Injuries
The spinal cord functions as a vital conduit, transmitting nerve impulses to and from the brain, much like a highway. When the spinal cord is damaged, this flow of information is disrupted, leading to... Read more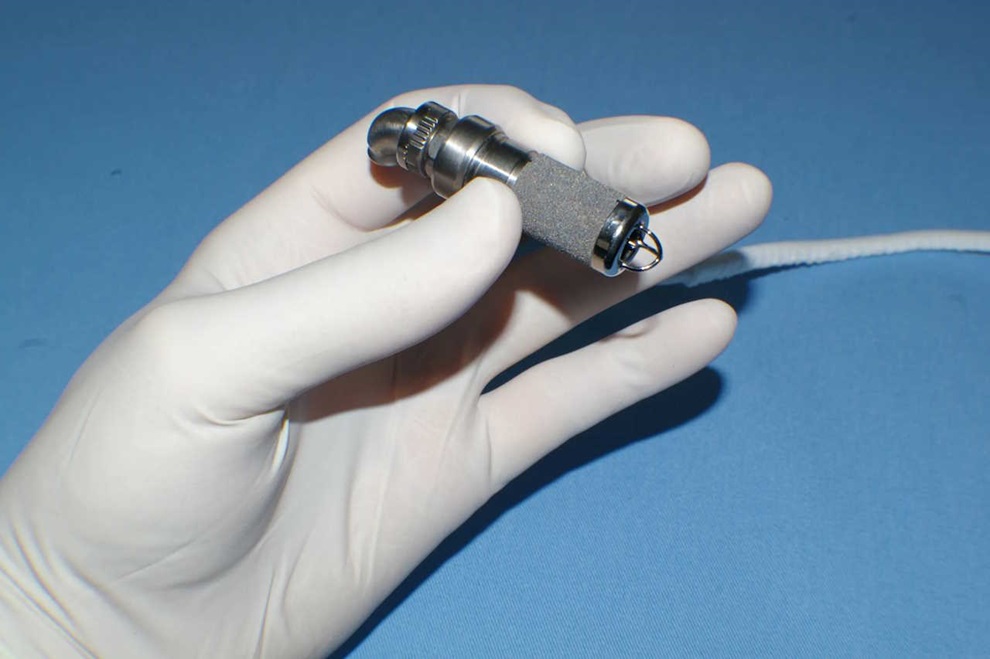
Small, Implantable Cardiac Pump to Help Children Awaiting Heart Transplant
Implantable ventricular assist devices, available for adults for over 40 years, fit inside the chest and are generally safer and easier to use than external devices. These devices enable patients to live... Read more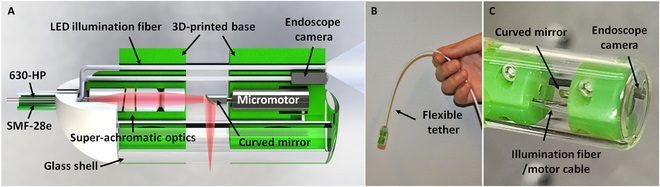
Gastrointestinal Imaging Capsule a Game-Changer in Esophagus Surveillance and Treatment
A newly-developed gastrointestinal imaging capsule is poised to be a game-changer in esophagus surveillance and interventions. The Multifunctional Ablative Gastrointestinal Imaging Capsule (MAGIC) developed... Read morePatient Care
view channel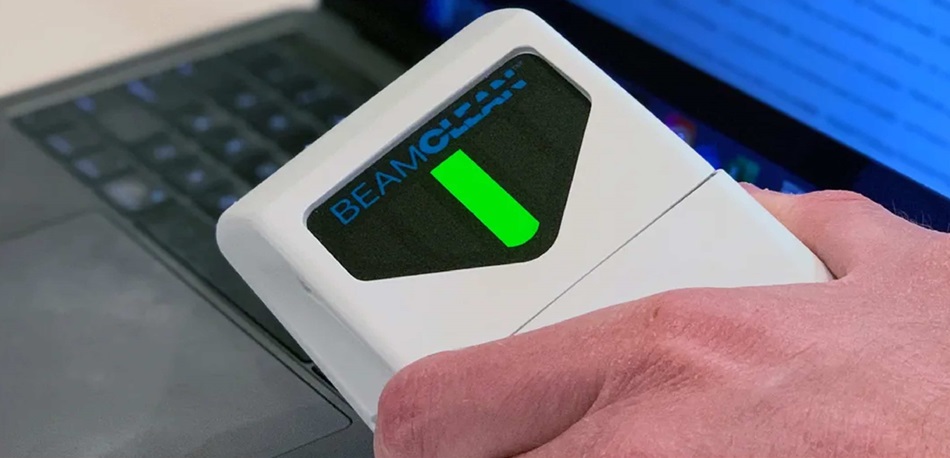
First-Of-Its-Kind Portable Germicidal Light Technology Disinfects High-Touch Clinical Surfaces in Seconds
Reducing healthcare-acquired infections (HAIs) remains a pressing issue within global healthcare systems. In the United States alone, 1.7 million patients contract HAIs annually, leading to approximately... Read more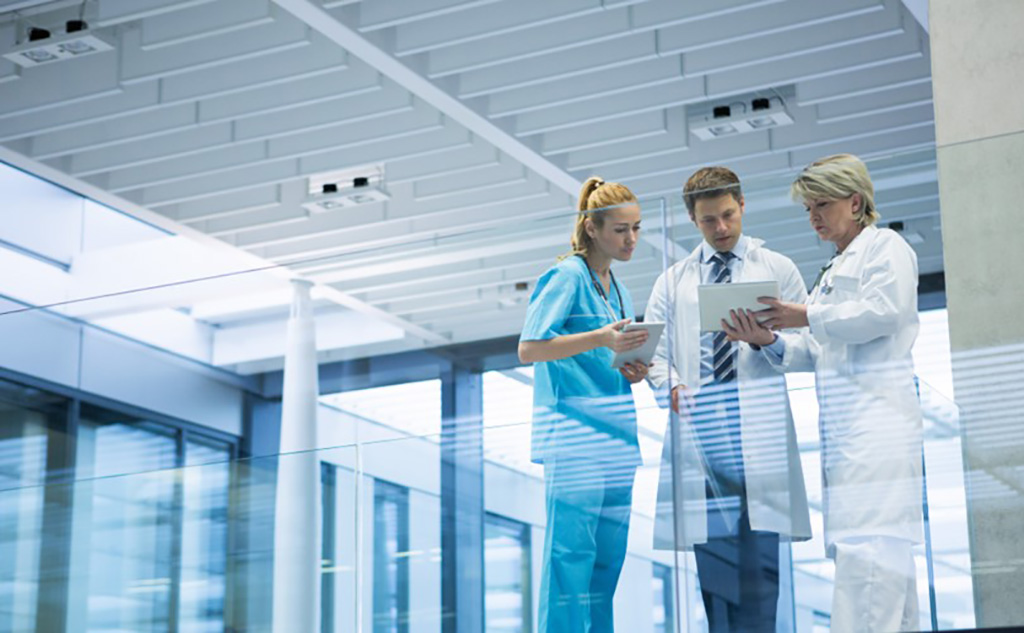
Surgical Capacity Optimization Solution Helps Hospitals Boost OR Utilization
An innovative solution has the capability to transform surgical capacity utilization by targeting the root cause of surgical block time inefficiencies. Fujitsu Limited’s (Tokyo, Japan) Surgical Capacity... Read more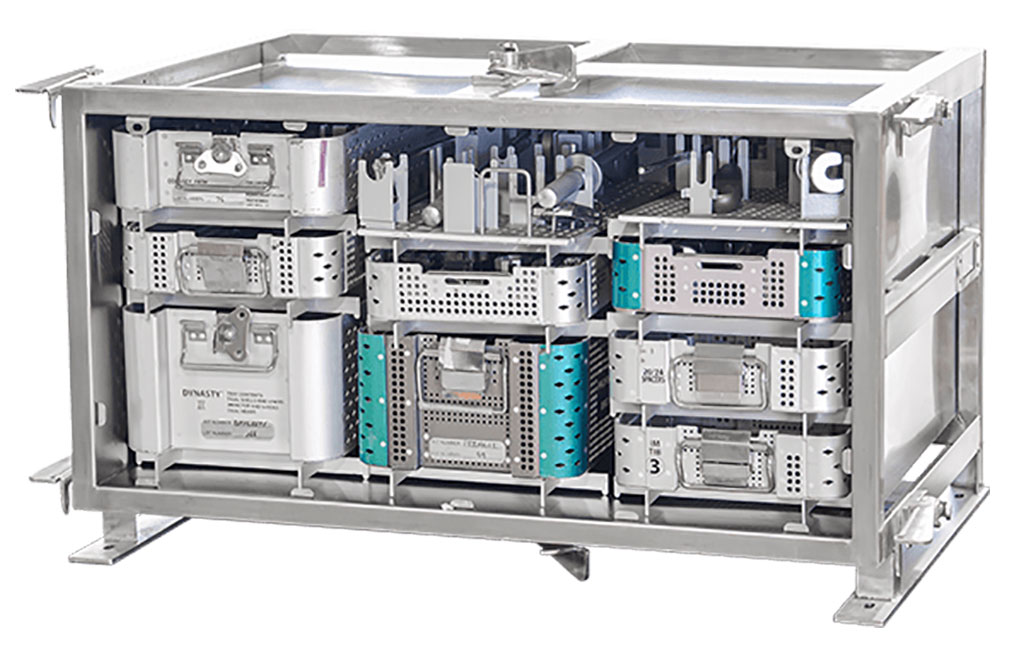
Game-Changing Innovation in Surgical Instrument Sterilization Significantly Improves OR Throughput
A groundbreaking innovation enables hospitals to significantly improve instrument processing time and throughput in operating rooms (ORs) and sterile processing departments. Turbett Surgical, Inc.... Read moreHealth IT
view channel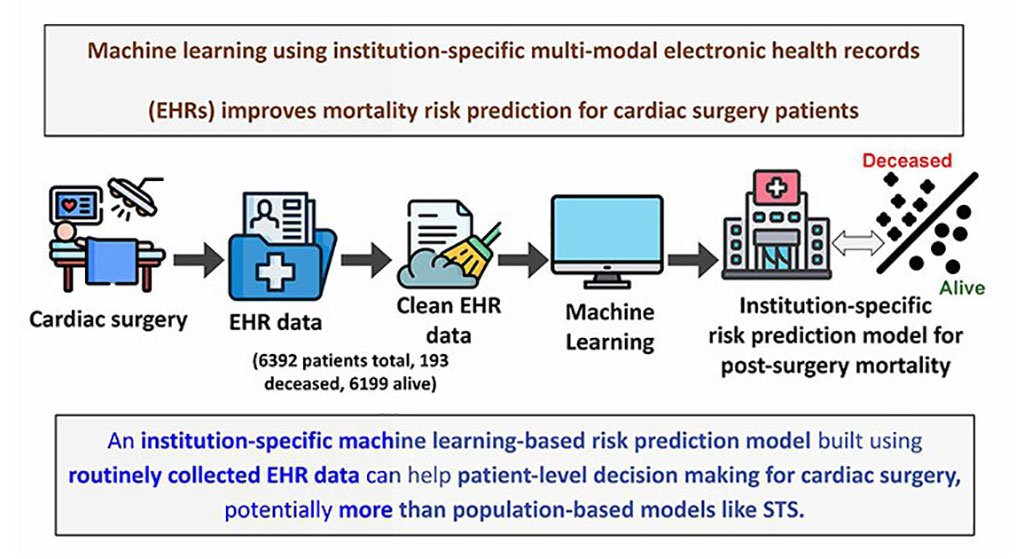
Machine Learning Model Improves Mortality Risk Prediction for Cardiac Surgery Patients
Machine learning algorithms have been deployed to create predictive models in various medical fields, with some demonstrating improved outcomes compared to their standard-of-care counterparts.... Read more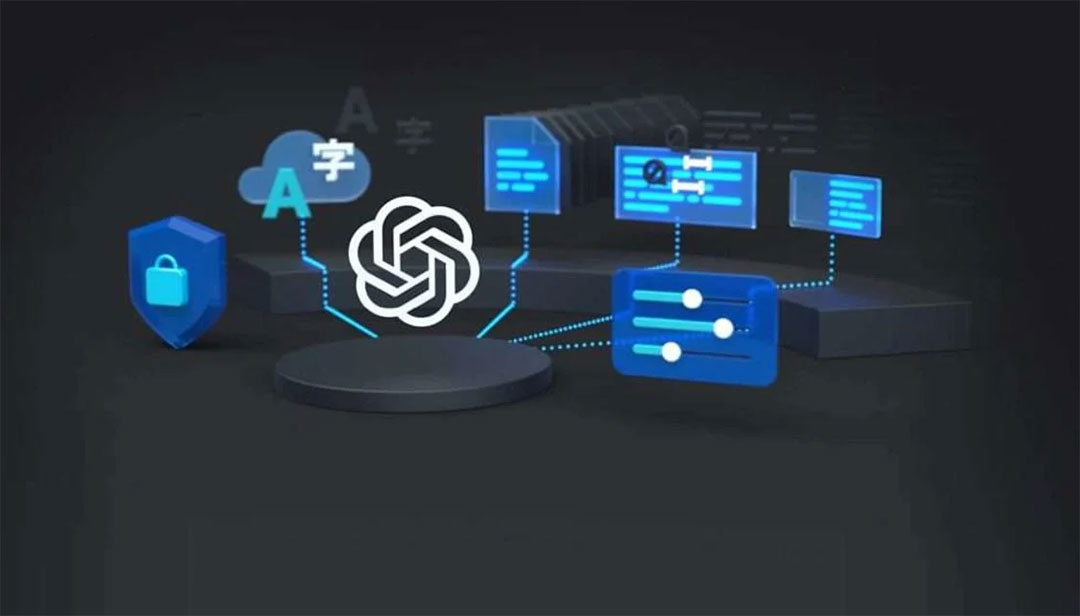
Strategic Collaboration to Develop and Integrate Generative AI into Healthcare
Top industry experts have underscored the immediate requirement for healthcare systems and hospitals to respond to severe cost and margin pressures. Close to half of U.S. hospitals ended 2022 in the red... Read more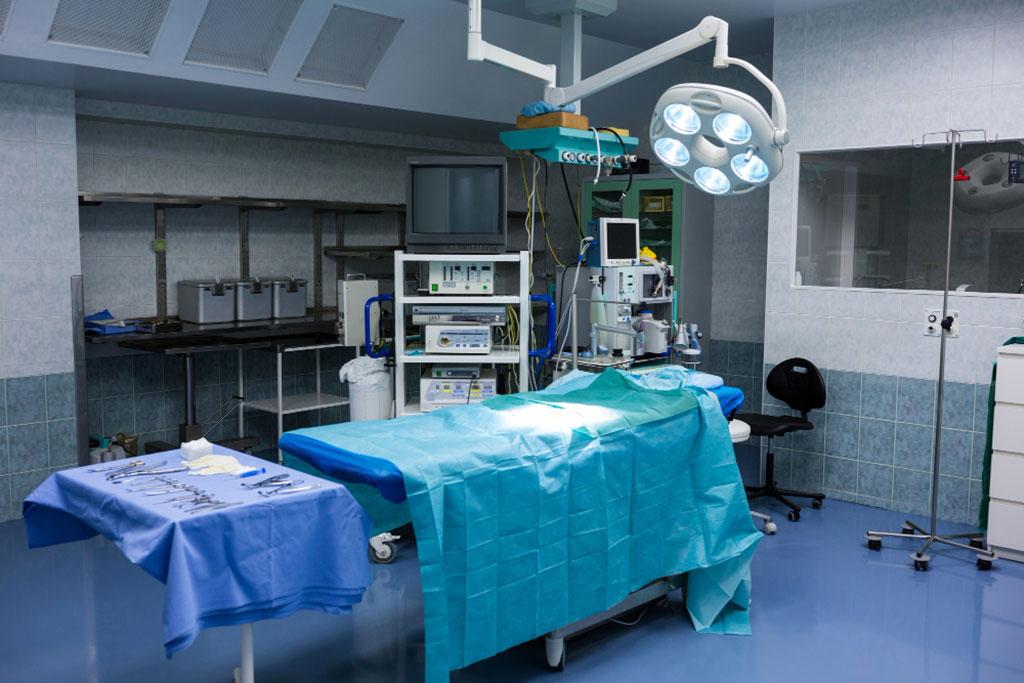
AI-Enabled Operating Rooms Solution Helps Hospitals Maximize Utilization and Unlock Capacity
For healthcare organizations, optimizing operating room (OR) utilization during prime time hours is a complex challenge. Surgeons and clinics face difficulties in finding available slots for booking cases,... Read more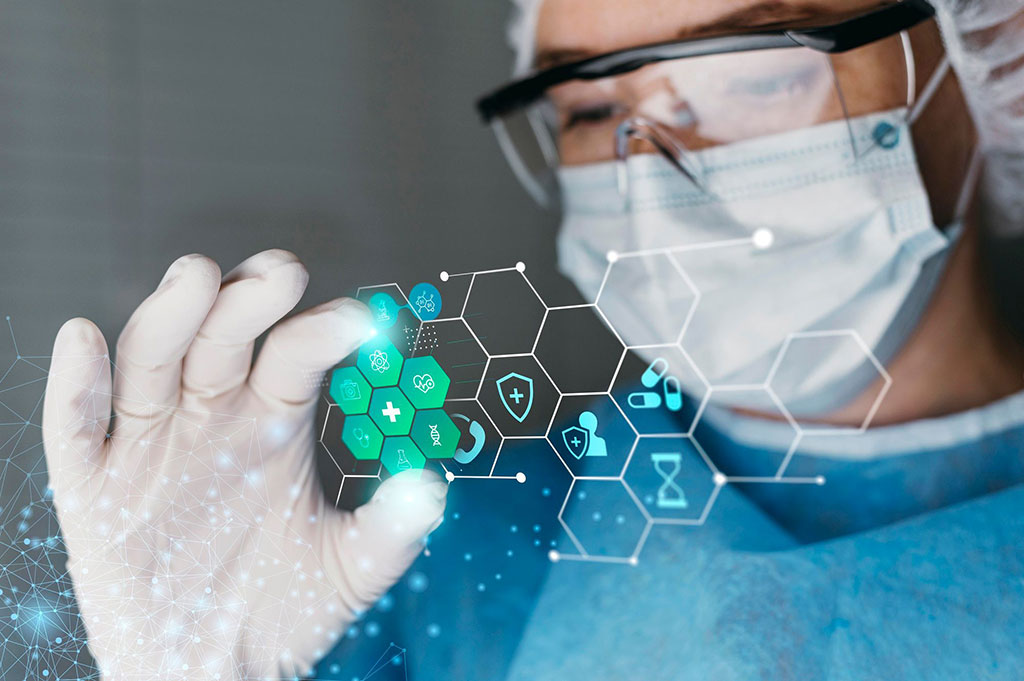
AI Predicts Pancreatic Cancer Three Years before Diagnosis from Patients’ Medical Records
Screening for common cancers like breast, cervix, and prostate cancer relies on relatively simple and highly effective techniques, such as mammograms, Pap smears, and blood tests. These methods have revolutionized... Read morePoint of Care
view channel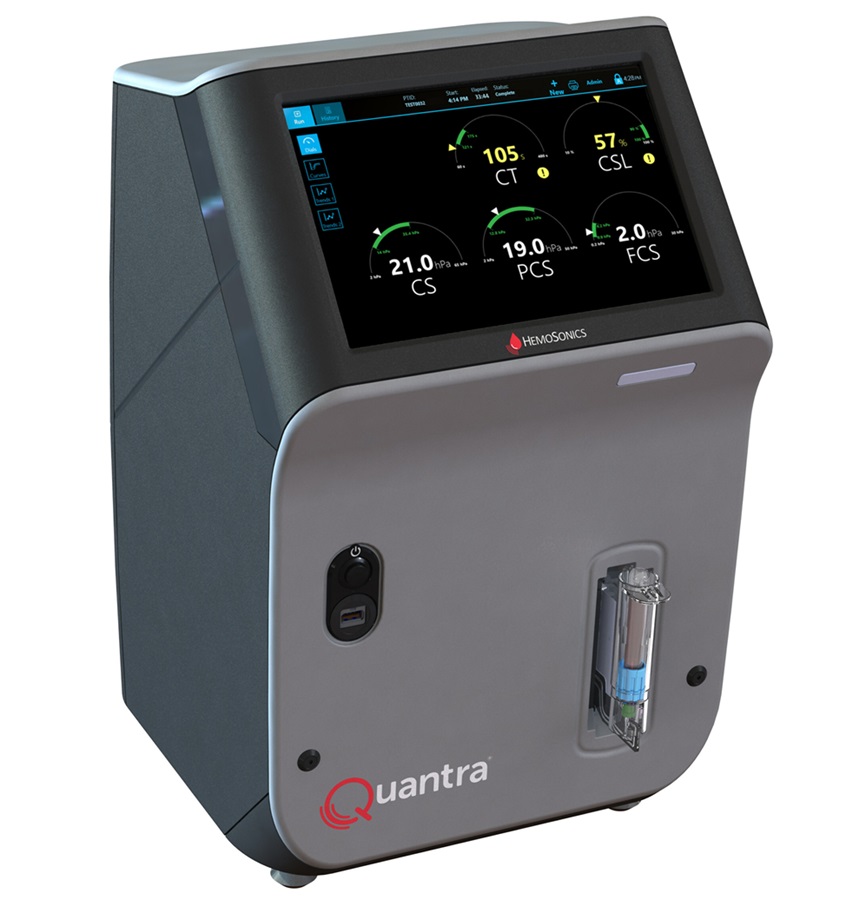
Critical Bleeding Management System to Help Hospitals Further Standardize Viscoelastic Testing
Surgical procedures are often accompanied by significant blood loss and the subsequent high likelihood of the need for allogeneic blood transfusions. These transfusions, while critical, are linked to various... Read more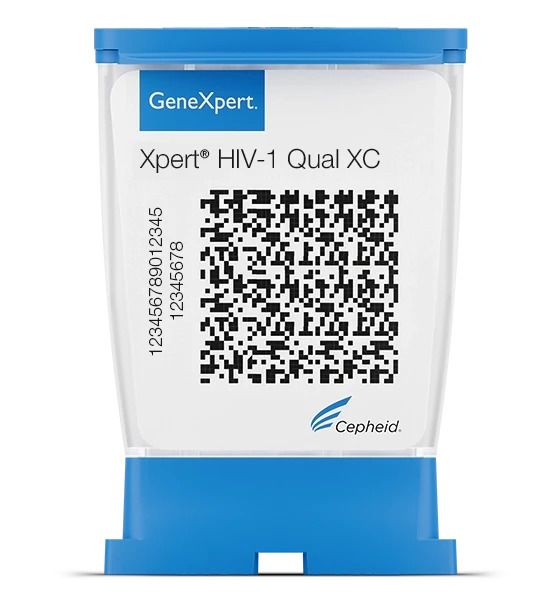
Point of Care HIV Test Enables Early Infection Diagnosis for Infants
Early diagnosis and initiation of treatment are crucial for the survival of infants infected with HIV (human immunodeficiency virus). Without treatment, approximately 50% of infants who acquire HIV during... Read more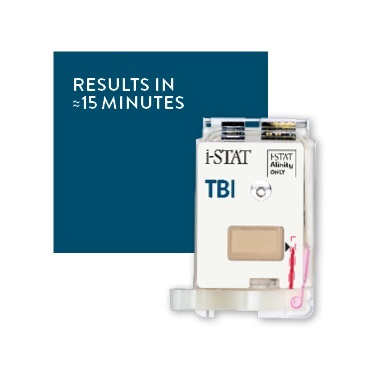
Whole Blood Rapid Test Aids Assessment of Concussion at Patient's Bedside
In the United States annually, approximately five million individuals seek emergency department care for traumatic brain injuries (TBIs), yet over half of those suspecting a concussion may never get it checked.... Read more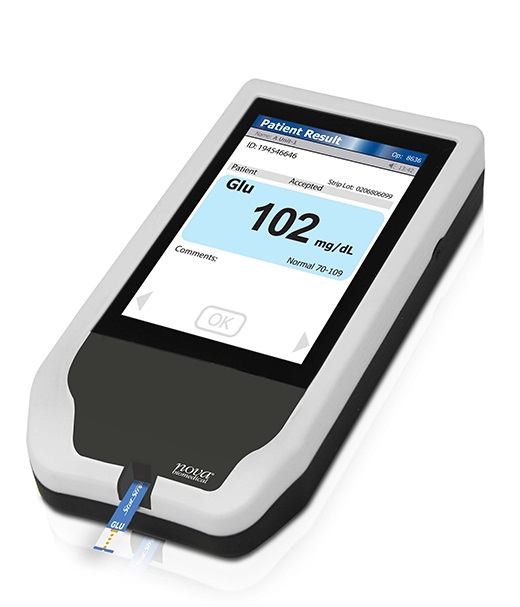
New Generation Glucose Hospital Meter System Ensures Accurate, Interference-Free and Safe Use
A new generation glucose hospital meter system now comes with several features that make hospital glucose testing easier and more secure while continuing to offer accuracy, freedom from interference, and... Read moreBusiness
view channel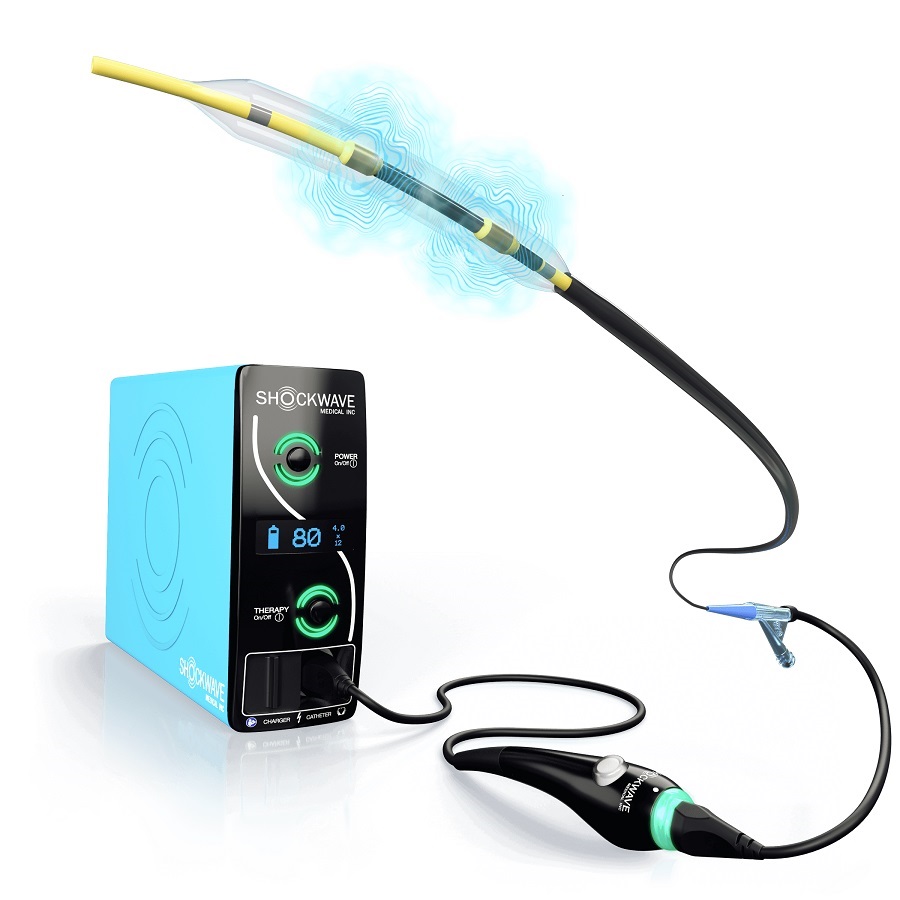
Johnson & Johnson Acquires Cardiovascular Medical Device Company Shockwave Medical
Johnson & Johnson (New Brunswick, N.J., USA) and Shockwave Medical (Santa Clara, CA, USA) have entered into a definitive agreement under which Johnson & Johnson will acquire all of Shockwave’s... Read more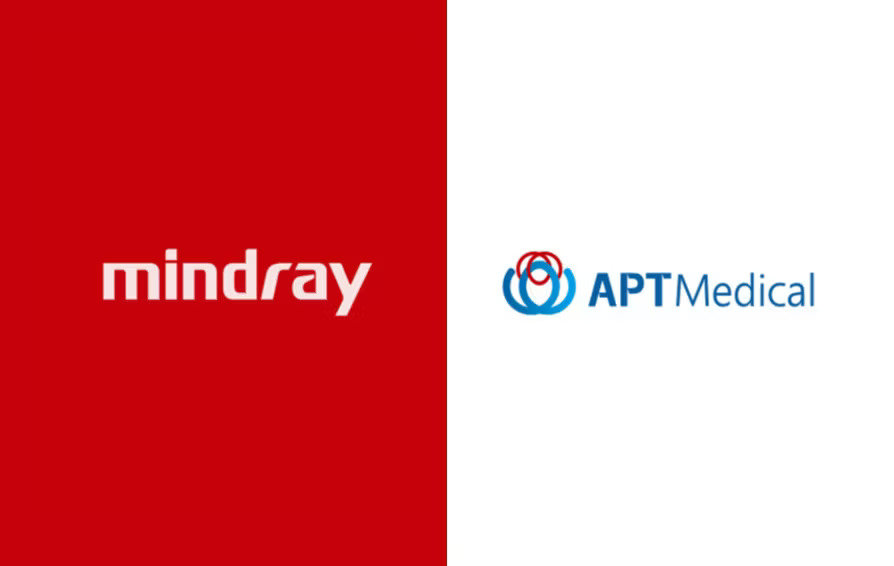