New Machine Learning Tool Accurately Predicts Prostate Cancer
By HospiMedica International staff writers Posted on 01 Mar 2019 |
Researchers from the Icahn School of Medicine at Mount Sinai (New York, NY, USA) and Keck School of Medicine at the University of Southern California (Los Angeles, CA, USA) have developed a machine-learning framework that can distinguish between low- and high-risk prostate cancer with greater precision than ever before. The framework is expected intended to help physicians, particularly radiologists, in identifying treatment options more accurately for prostate cancer patients, thereby reducing the need for unnecessary clinical intervention.
The standard methods currently being used to assess prostate cancer risk are multi-parametric magnetic resonance imaging (mpMRI), which detects prostate lesions, and the Prostate Imaging Reporting and Data System, version 2 (PI-RADS v2), a five-point scoring system that classifies lesions found on the mpMRI. These tools are intended to soundly predict the likelihood of clinically significant prostate cancer. However, PI-RADS v2 scoring is subjective and does not distinguish clearly between intermediate and malignant cancer levels (scores 3, 4, and 5), resulting in differing interpretations among clinicians most of the time.
In order to remedy this drawback, it has been proposed to combine machine learning with radiomics—a branch of medicine that uses algorithms to extract large amounts of quantitative characteristics from medical images. While other studies have only tested a limited number of machine learning methods to address this limitation, the Mount Sinai and USC researchers have developed a predictive framework that rigorously and systematically assessed many such methods to identify the best-performing one. The framework also leverages larger training and validation data sets than previous studies did, allowing the researchers to classify the patients’ prostate cancer with high sensitivity and an even higher predictive value.
“By rigorously and systematically combining machine learning with radiomics, our goal is to provide radiologists and clinical personnel with a sound prediction tool that can eventually translate to more effective and personalized patient care,” said Gaurav Pandey, PhD, Assistant Professor of Genetics and Genomic Sciences at the Icahn School of Medicine at Mount Sinai and senior corresponding author of the publication alongside co-corresponding author Bino Varghese, PhD, Assistant Professor of Research Radiology at the Keck School of Medicine at USC. “The pathway to predicting prostate cancer progression with high accuracy is ever improving, and we believe our objective framework is a much-needed advancement.”
Related Links:
Icahn School of Medicine at Mount Sinai
Keck School of Medicine at the University of Southern California
The standard methods currently being used to assess prostate cancer risk are multi-parametric magnetic resonance imaging (mpMRI), which detects prostate lesions, and the Prostate Imaging Reporting and Data System, version 2 (PI-RADS v2), a five-point scoring system that classifies lesions found on the mpMRI. These tools are intended to soundly predict the likelihood of clinically significant prostate cancer. However, PI-RADS v2 scoring is subjective and does not distinguish clearly between intermediate and malignant cancer levels (scores 3, 4, and 5), resulting in differing interpretations among clinicians most of the time.
In order to remedy this drawback, it has been proposed to combine machine learning with radiomics—a branch of medicine that uses algorithms to extract large amounts of quantitative characteristics from medical images. While other studies have only tested a limited number of machine learning methods to address this limitation, the Mount Sinai and USC researchers have developed a predictive framework that rigorously and systematically assessed many such methods to identify the best-performing one. The framework also leverages larger training and validation data sets than previous studies did, allowing the researchers to classify the patients’ prostate cancer with high sensitivity and an even higher predictive value.
“By rigorously and systematically combining machine learning with radiomics, our goal is to provide radiologists and clinical personnel with a sound prediction tool that can eventually translate to more effective and personalized patient care,” said Gaurav Pandey, PhD, Assistant Professor of Genetics and Genomic Sciences at the Icahn School of Medicine at Mount Sinai and senior corresponding author of the publication alongside co-corresponding author Bino Varghese, PhD, Assistant Professor of Research Radiology at the Keck School of Medicine at USC. “The pathway to predicting prostate cancer progression with high accuracy is ever improving, and we believe our objective framework is a much-needed advancement.”
Related Links:
Icahn School of Medicine at Mount Sinai
Keck School of Medicine at the University of Southern California
Channels
Artificial Intelligence
view channel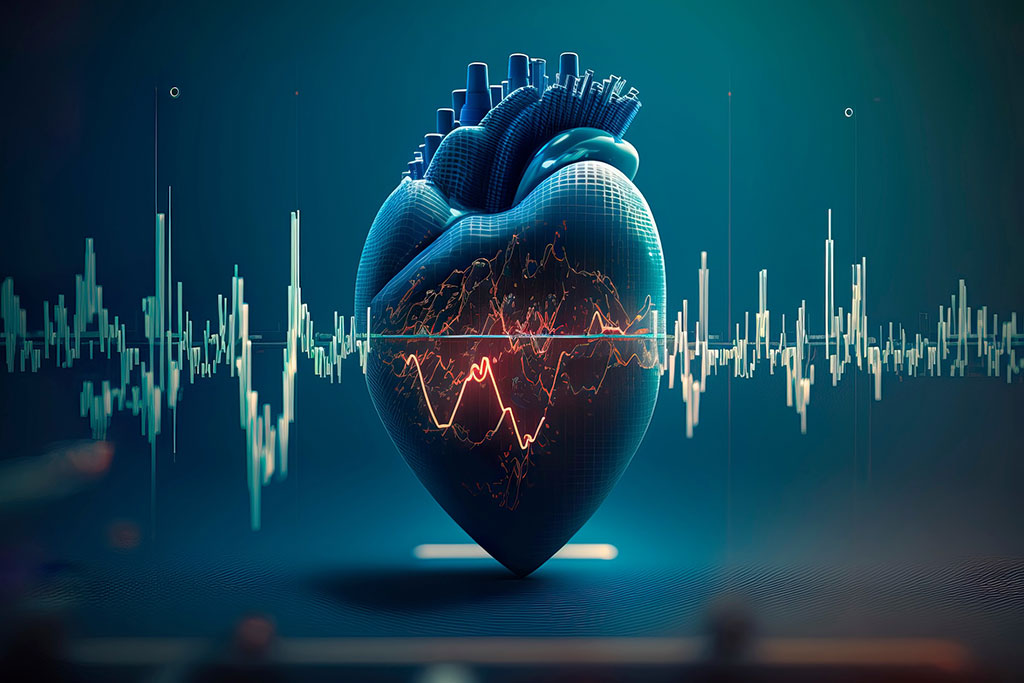
AI-Powered Algorithm to Revolutionize Detection of Atrial Fibrillation
Atrial fibrillation (AFib), a condition characterized by an irregular and often rapid heart rate, is linked to increased risks of stroke and heart failure. This is because the irregular heartbeat in AFib... Read more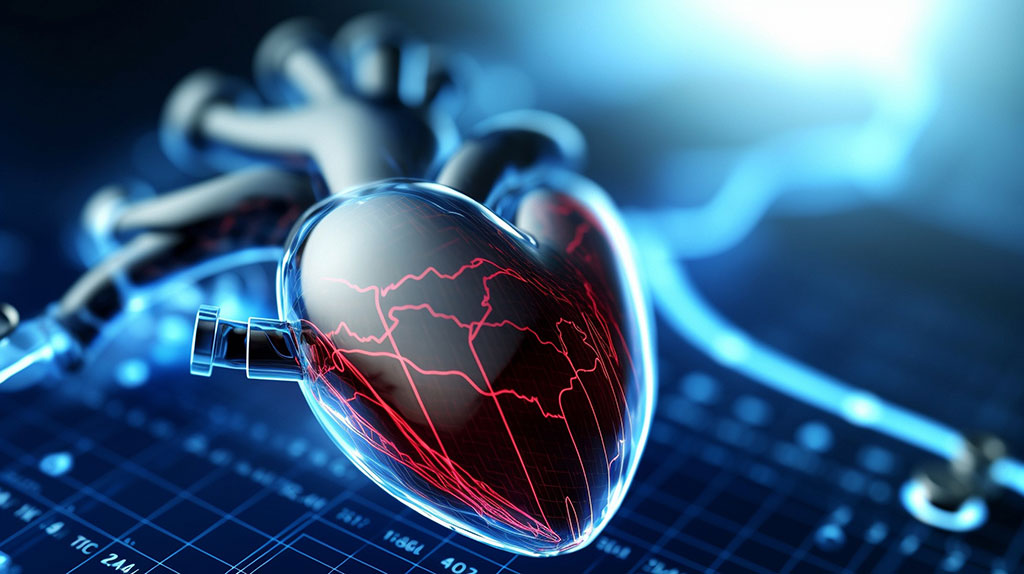
AI Diagnostic Tool Accurately Detects Valvular Disorders Often Missed by Doctors
Doctors generally use stethoscopes to listen for the characteristic lub-dub sounds made by heart valves opening and closing. They also listen for less prominent sounds that indicate problems with these valves.... Read moreCritical Care
view channel.jpeg)
Transcatheter Valve Replacement Outcomes Similar To Surgery, Finds Study
A new study has shown that a minimally invasive procedure for replacing the aortic valve in the heart—known as transcatheter aortic valve replacement (TAVR)—is on par with the more traditional surgical... Read more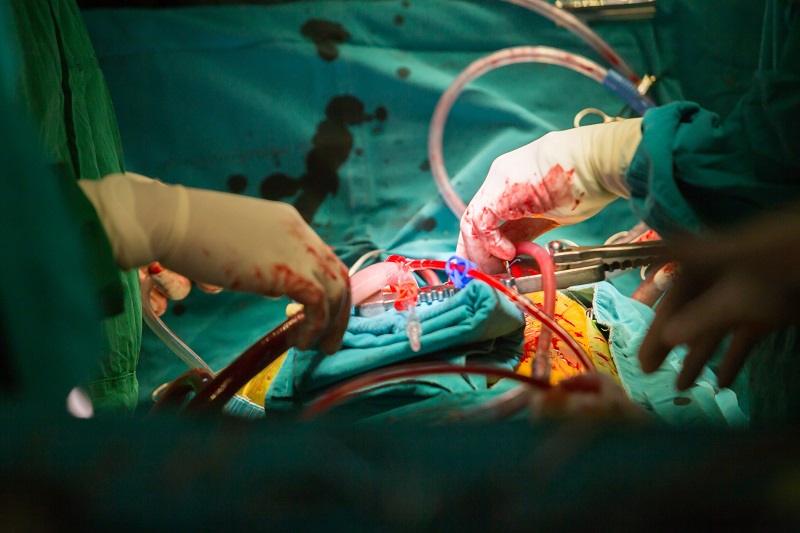
Revascularization Improves Life Quality in Chronic Limb-Threatening Ischemia, Finds Study
Researchers have undertaken a detailed study to evaluate the effects of revascularization strategies on the health-related quality of life (HRQoL) of patients with chronic limb-threatening ischemia.... Read moreSurgical Techniques
view channel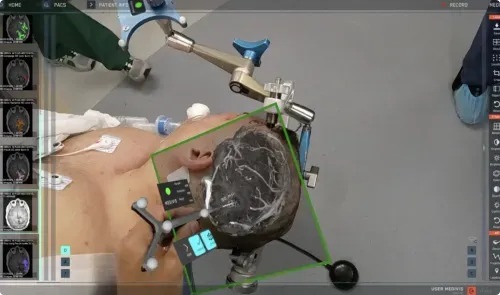
AR Surgical Technology Translates Complex 2D Medical Imaging to Enhance Accuracy
Surgeons often have to switch their focus between a patient’s data displayed on a screen or clipboard and the patient themselves during procedures. But that is about to change. Surgeons can now utilize... Read more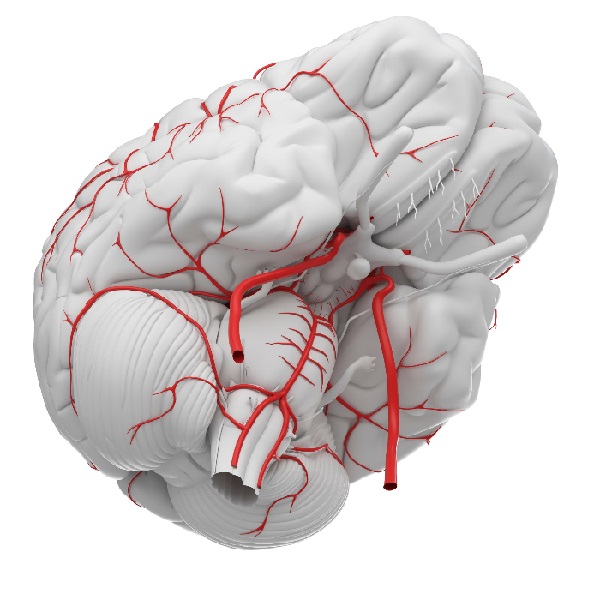
Miniaturized Snake-Like Probe Images Cerebral Arteries From Within
Endovascular interventions are being increasingly favored for treating strokes and cerebral artery diseases, but rely heavily on angiographical imaging that often struggles with limited contrast and spatial... Read morePatient Care
view channel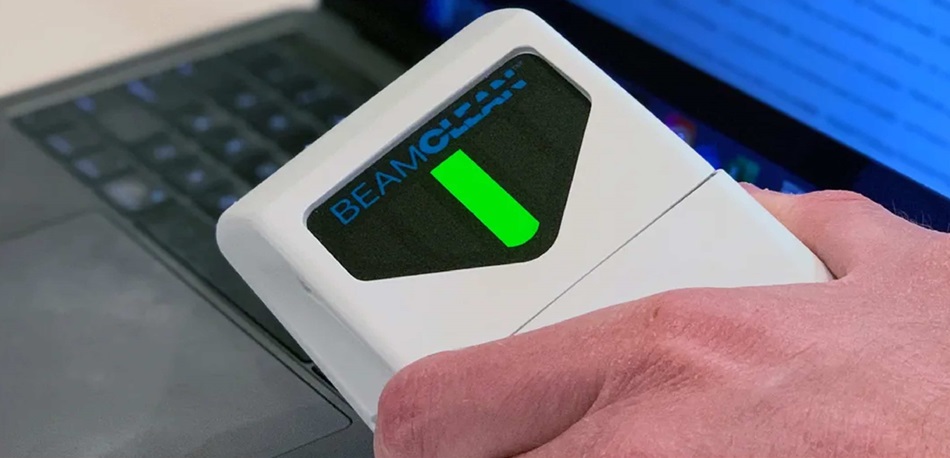
First-Of-Its-Kind Portable Germicidal Light Technology Disinfects High-Touch Clinical Surfaces in Seconds
Reducing healthcare-acquired infections (HAIs) remains a pressing issue within global healthcare systems. In the United States alone, 1.7 million patients contract HAIs annually, leading to approximately... Read more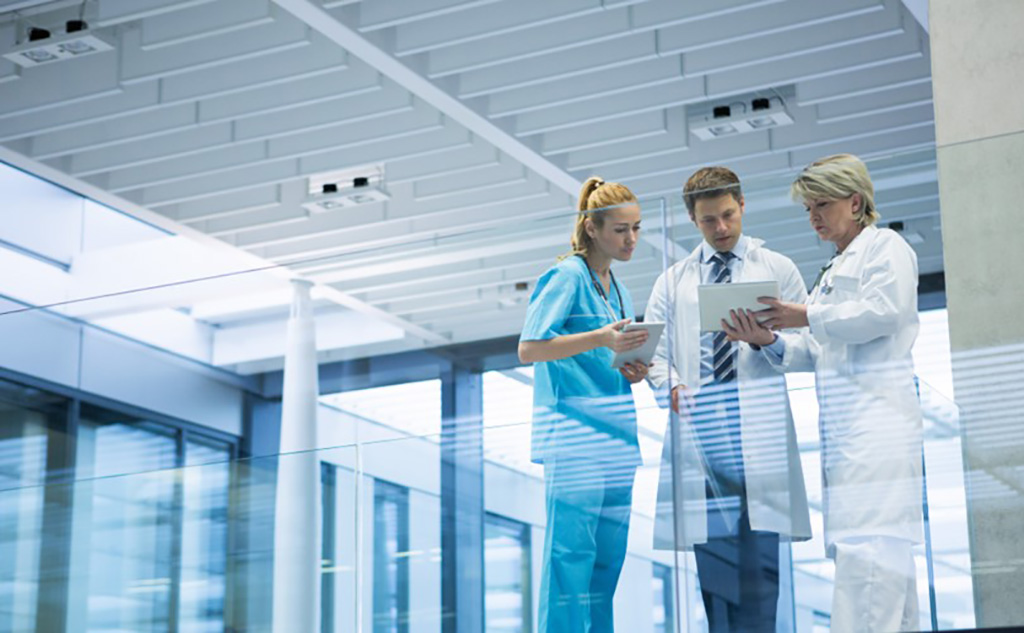
Surgical Capacity Optimization Solution Helps Hospitals Boost OR Utilization
An innovative solution has the capability to transform surgical capacity utilization by targeting the root cause of surgical block time inefficiencies. Fujitsu Limited’s (Tokyo, Japan) Surgical Capacity... Read more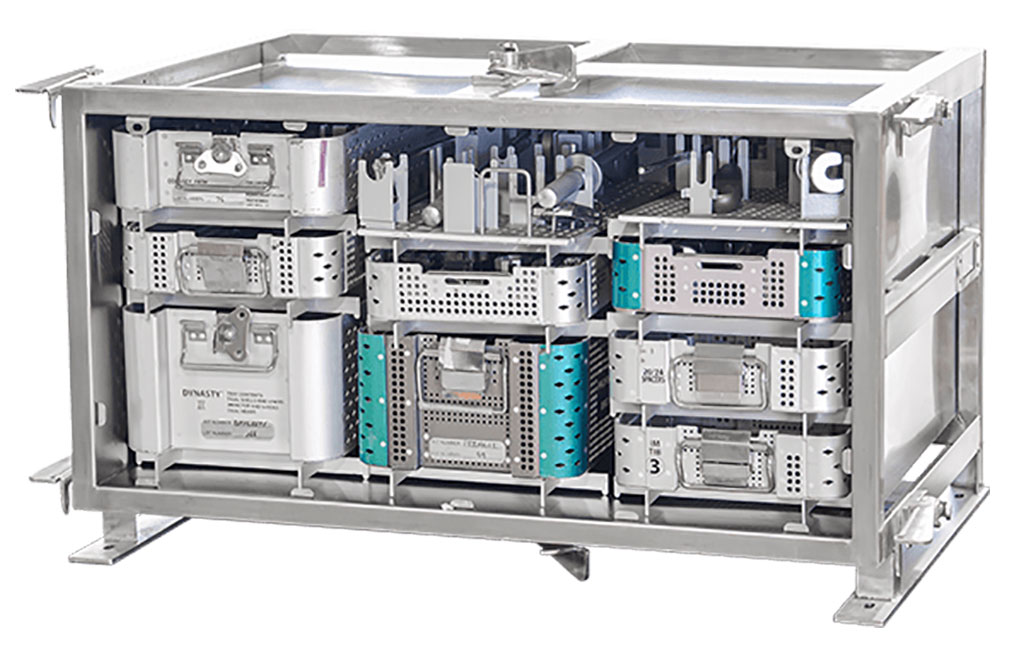
Game-Changing Innovation in Surgical Instrument Sterilization Significantly Improves OR Throughput
A groundbreaking innovation enables hospitals to significantly improve instrument processing time and throughput in operating rooms (ORs) and sterile processing departments. Turbett Surgical, Inc.... Read moreHealth IT
view channel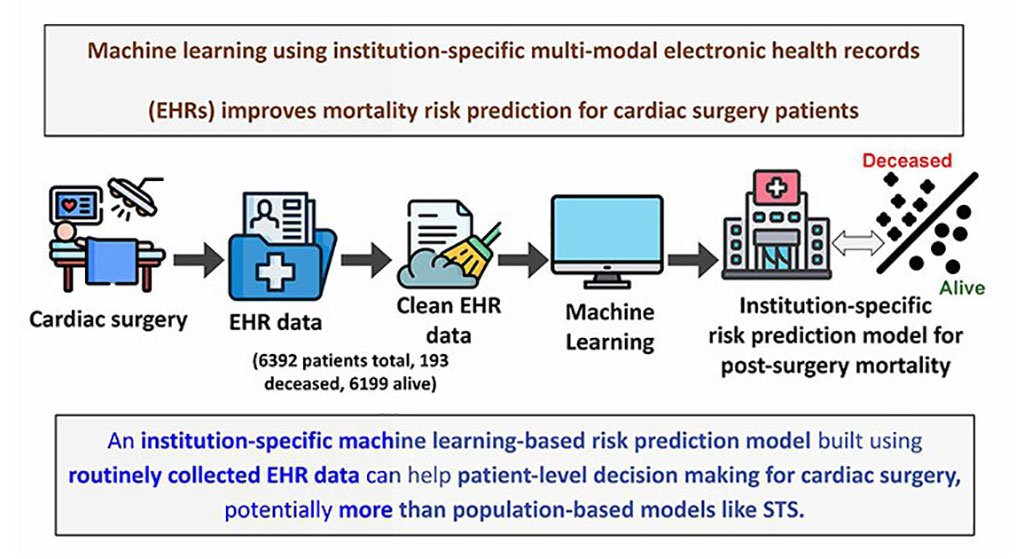
Machine Learning Model Improves Mortality Risk Prediction for Cardiac Surgery Patients
Machine learning algorithms have been deployed to create predictive models in various medical fields, with some demonstrating improved outcomes compared to their standard-of-care counterparts.... Read more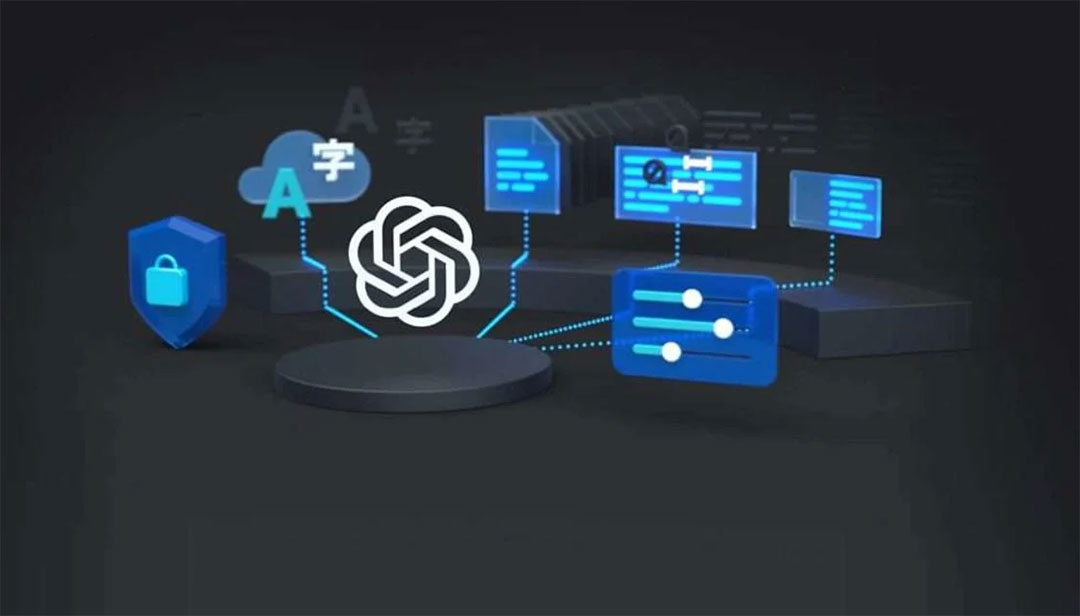
Strategic Collaboration to Develop and Integrate Generative AI into Healthcare
Top industry experts have underscored the immediate requirement for healthcare systems and hospitals to respond to severe cost and margin pressures. Close to half of U.S. hospitals ended 2022 in the red... Read more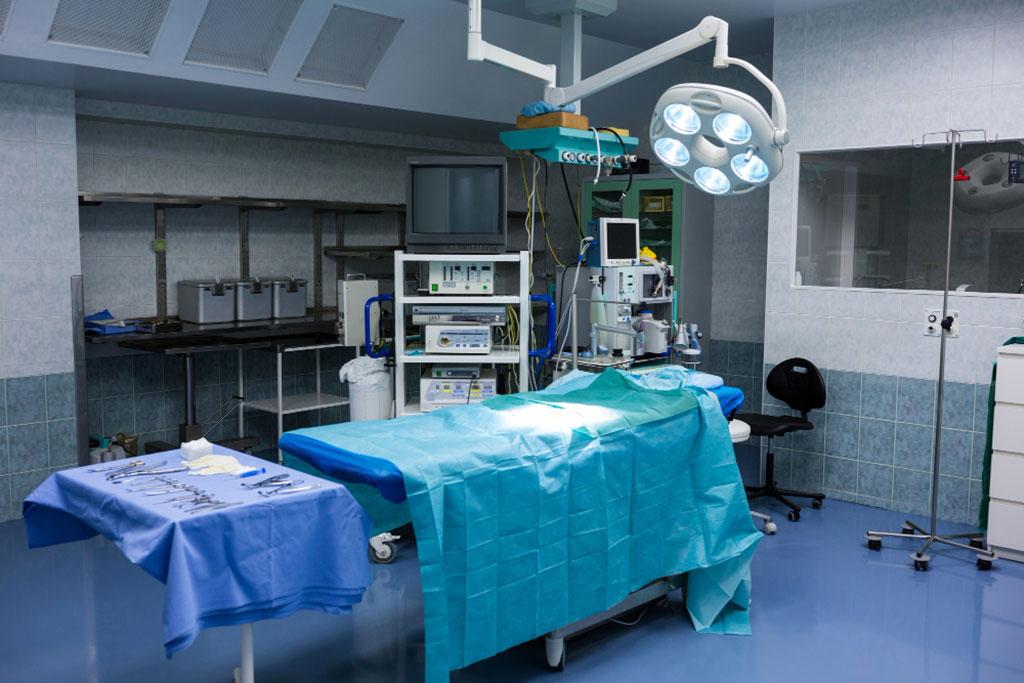
AI-Enabled Operating Rooms Solution Helps Hospitals Maximize Utilization and Unlock Capacity
For healthcare organizations, optimizing operating room (OR) utilization during prime time hours is a complex challenge. Surgeons and clinics face difficulties in finding available slots for booking cases,... Read more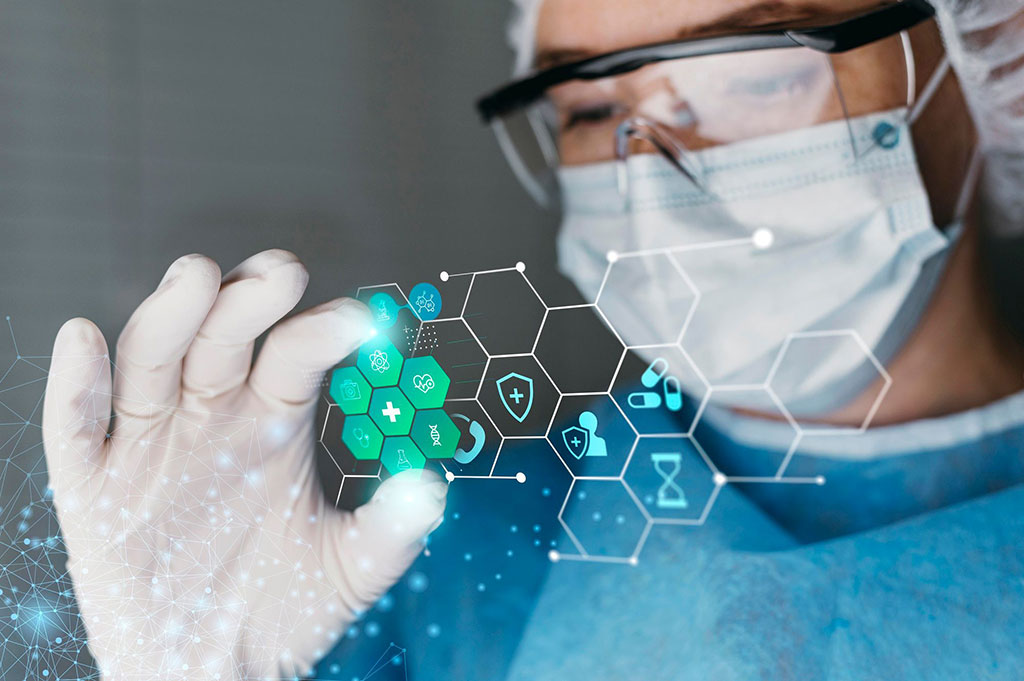
AI Predicts Pancreatic Cancer Three Years before Diagnosis from Patients’ Medical Records
Screening for common cancers like breast, cervix, and prostate cancer relies on relatively simple and highly effective techniques, such as mammograms, Pap smears, and blood tests. These methods have revolutionized... Read morePoint of Care
view channel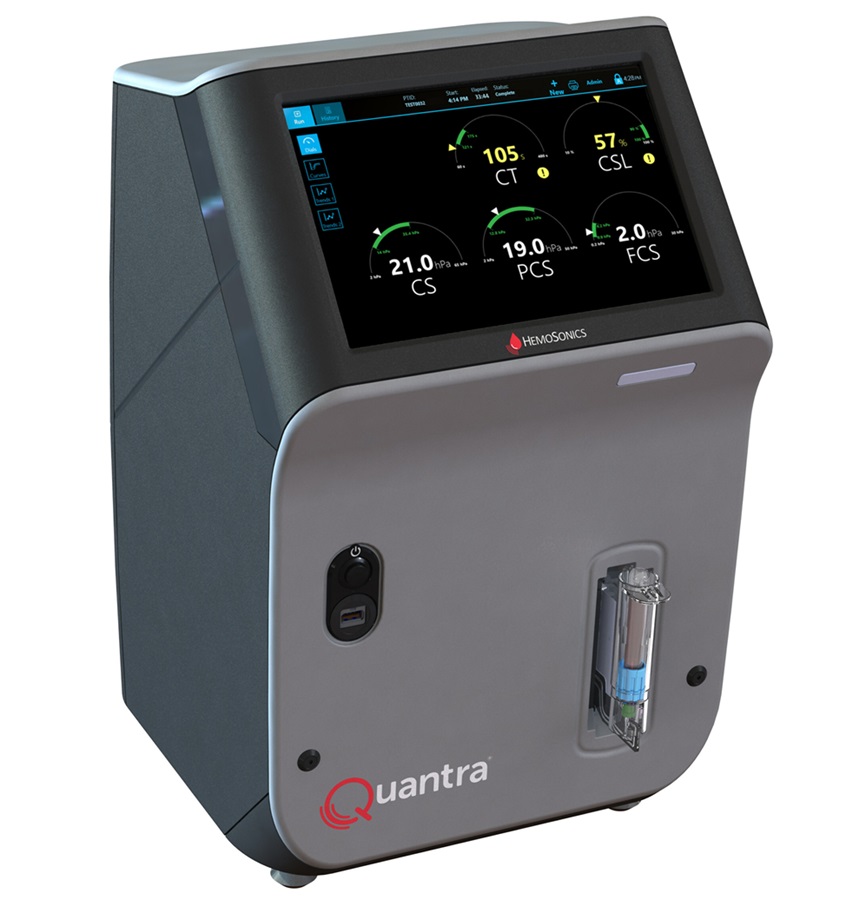
Critical Bleeding Management System to Help Hospitals Further Standardize Viscoelastic Testing
Surgical procedures are often accompanied by significant blood loss and the subsequent high likelihood of the need for allogeneic blood transfusions. These transfusions, while critical, are linked to various... Read more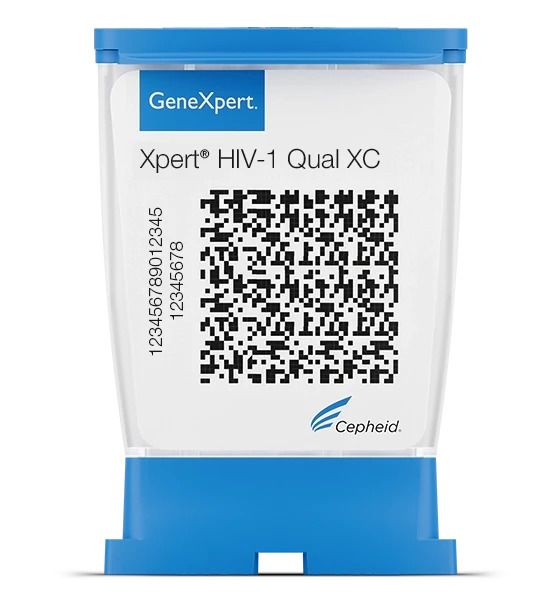
Point of Care HIV Test Enables Early Infection Diagnosis for Infants
Early diagnosis and initiation of treatment are crucial for the survival of infants infected with HIV (human immunodeficiency virus). Without treatment, approximately 50% of infants who acquire HIV during... Read more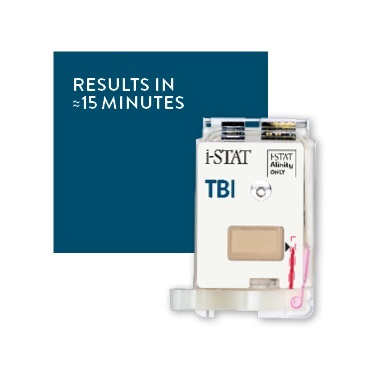
Whole Blood Rapid Test Aids Assessment of Concussion at Patient's Bedside
In the United States annually, approximately five million individuals seek emergency department care for traumatic brain injuries (TBIs), yet over half of those suspecting a concussion may never get it checked.... Read more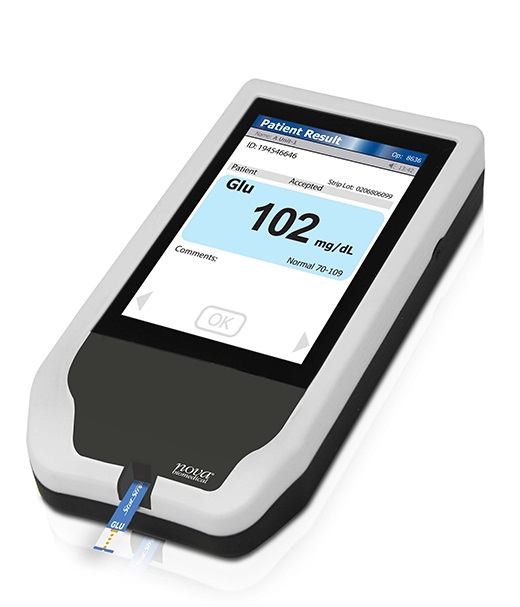
New Generation Glucose Hospital Meter System Ensures Accurate, Interference-Free and Safe Use
A new generation glucose hospital meter system now comes with several features that make hospital glucose testing easier and more secure while continuing to offer accuracy, freedom from interference, and... Read moreBusiness
view channel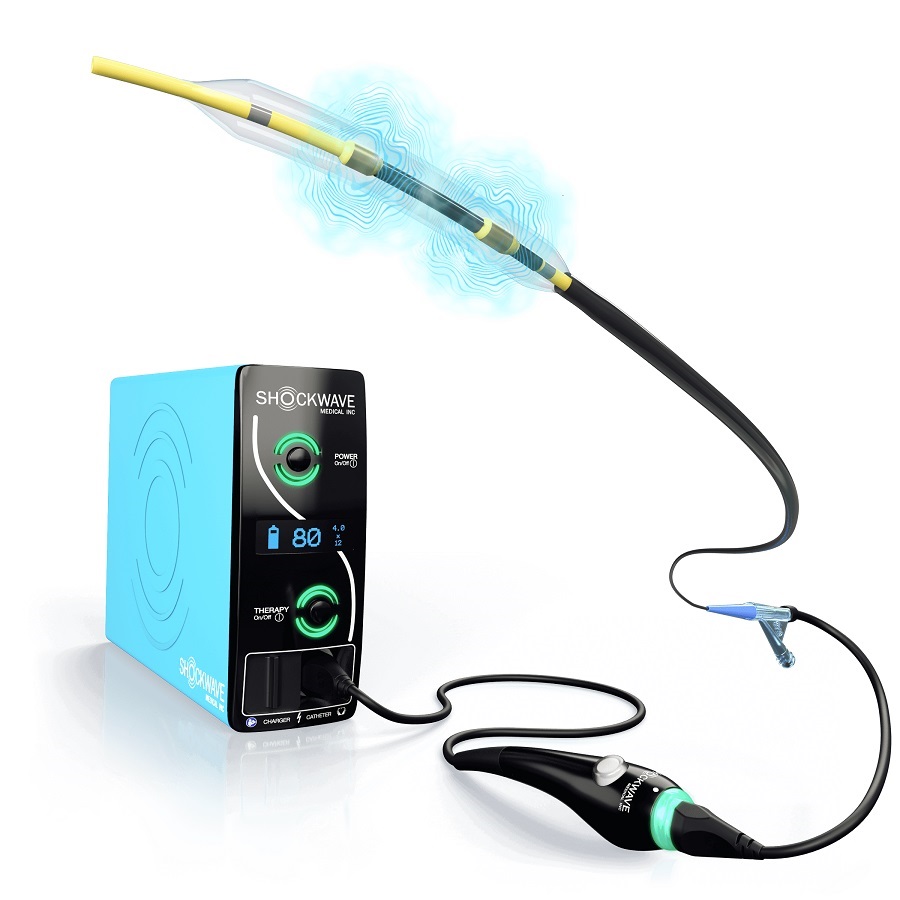
Johnson & Johnson Acquires Cardiovascular Medical Device Company Shockwave Medical
Johnson & Johnson (New Brunswick, N.J., USA) and Shockwave Medical (Santa Clara, CA, USA) have entered into a definitive agreement under which Johnson & Johnson will acquire all of Shockwave’s... Read more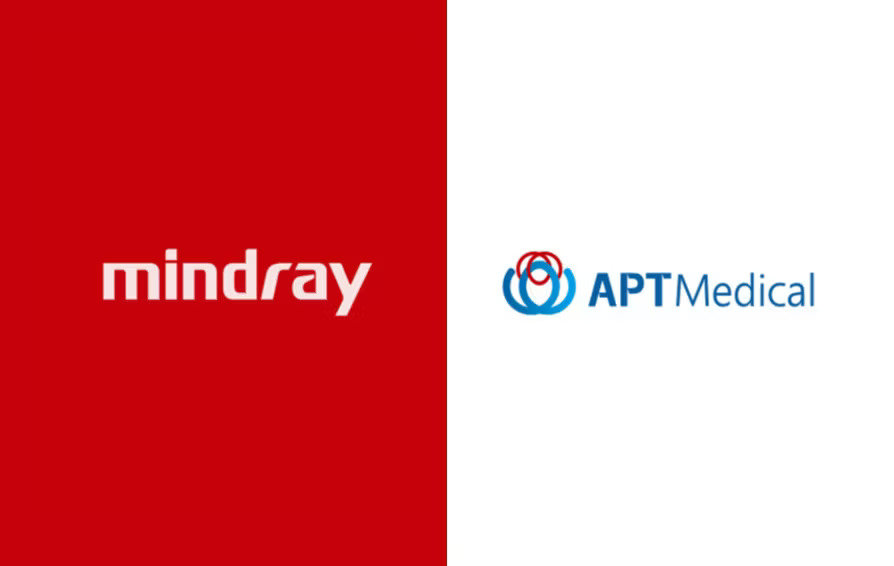