New AI Method Predicts Breast Cancer Five Years in Advance
By HospiMedica International staff writers Posted on 18 May 2019 |
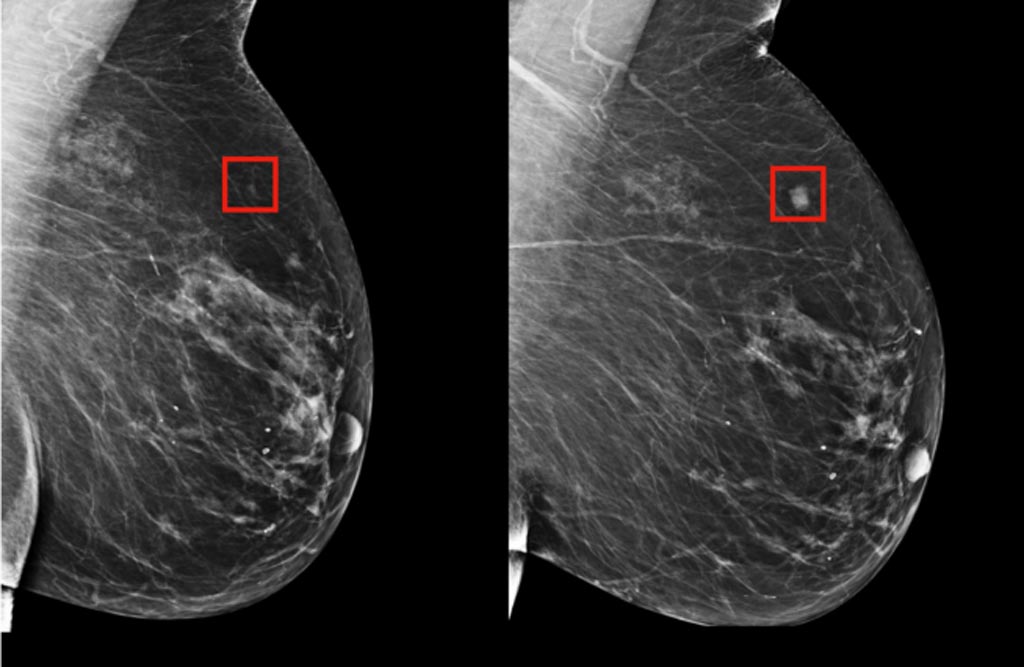
Image: A new AI method for detecting breast cancer is expected to surpass existing methods that fall short in their predictions (Photo courtesy of MIT).
Researchers from two major institutions have developed a new tool with advanced artificial intelligence (AI) methods to predict a woman’s future risk of breast cancer. The currently available models that use factors such as family history and genetics fall far short in predicting an individual woman’s likelihood of being diagnosed with breast cancer.
In some models, breast density—the amount of dense tissue compared to the amount of fatty tissue in the breast on a mammogram— has been added to improve risk assessment as it is an independent risk factor for breast cancer. Since it is based on subjective assessment that can vary across radiologists, deep learning, a subset of AI in which computers learn by example, has been studied as a way to standardize and automate these measurements.
Adam Yala, a Ph.D. candidate at the Massachusetts Institute of Technology (MIT), in collaboration with Regina Barzilay, Ph.D., an AI expert and professor at MIT, and Constance Lehman, M.D, Ph.D., chief of breast imaging at Massachusetts General Hospital and professor of radiology at Harvard Medical School, recently compared three different risk assessment approaches.
The first model relied on traditional risk factors, the second on deep learning that used the mammogram alone, and the third on a hybrid approach that incorporated both the mammogram and traditional risk factors into the deep learning model. The researchers used nearly 90,000 full-resolution screening mammograms from about 40,000 women to train, validate and test the deep learning model. They were able to obtain cancer outcomes through linkage to a regional tumor registry.
The deep learning models yielded substantially improved risk discrimination over the Tyrer-Cuzick model, a current clinical standard that uses breast density in factoring risk. When comparing the hybrid deep learning model against breast density, the researchers found that patients with non-dense breasts and model-assessed high risk had 3.9 times the cancer incidence of patients with dense breasts and model-assessed low risk. The advantages held across different subgroups of women.
“There’s much more information in a mammogram than just the four categories of breast density. By using the deep learning model, we learn subtle cues that are indicative of future cancer,” said Yala. “There’s a very large amount of information in a full-resolution mammogram that breast cancer risk models have not been able to use until recently. Using deep learning, we can learn to leverage that information directly from the data and create models that are significantly more accurate across diverse populations.”
“Unlike traditional models, our deep learning model performs equally well across diverse races, ages and family histories,” Dr. Barzilay said. “Until now, African-American women were at a distinct disadvantage in having accurate risk assessment of future breast cancer. Our AI model has changed that.”
“A missing element to support more effective, more personalized screening programs has been risk-assessment tools that are easy to implement and that work across the full diversity of women whom we serve,” Dr. Lehman said. “We are thrilled with our results and eager to work closely with our health care systems, our providers and, most importantly, our patients to incorporate this discovery into improved outcomes for all women.”
Related Links:
MIT
In some models, breast density—the amount of dense tissue compared to the amount of fatty tissue in the breast on a mammogram— has been added to improve risk assessment as it is an independent risk factor for breast cancer. Since it is based on subjective assessment that can vary across radiologists, deep learning, a subset of AI in which computers learn by example, has been studied as a way to standardize and automate these measurements.
Adam Yala, a Ph.D. candidate at the Massachusetts Institute of Technology (MIT), in collaboration with Regina Barzilay, Ph.D., an AI expert and professor at MIT, and Constance Lehman, M.D, Ph.D., chief of breast imaging at Massachusetts General Hospital and professor of radiology at Harvard Medical School, recently compared three different risk assessment approaches.
The first model relied on traditional risk factors, the second on deep learning that used the mammogram alone, and the third on a hybrid approach that incorporated both the mammogram and traditional risk factors into the deep learning model. The researchers used nearly 90,000 full-resolution screening mammograms from about 40,000 women to train, validate and test the deep learning model. They were able to obtain cancer outcomes through linkage to a regional tumor registry.
The deep learning models yielded substantially improved risk discrimination over the Tyrer-Cuzick model, a current clinical standard that uses breast density in factoring risk. When comparing the hybrid deep learning model against breast density, the researchers found that patients with non-dense breasts and model-assessed high risk had 3.9 times the cancer incidence of patients with dense breasts and model-assessed low risk. The advantages held across different subgroups of women.
“There’s much more information in a mammogram than just the four categories of breast density. By using the deep learning model, we learn subtle cues that are indicative of future cancer,” said Yala. “There’s a very large amount of information in a full-resolution mammogram that breast cancer risk models have not been able to use until recently. Using deep learning, we can learn to leverage that information directly from the data and create models that are significantly more accurate across diverse populations.”
“Unlike traditional models, our deep learning model performs equally well across diverse races, ages and family histories,” Dr. Barzilay said. “Until now, African-American women were at a distinct disadvantage in having accurate risk assessment of future breast cancer. Our AI model has changed that.”
“A missing element to support more effective, more personalized screening programs has been risk-assessment tools that are easy to implement and that work across the full diversity of women whom we serve,” Dr. Lehman said. “We are thrilled with our results and eager to work closely with our health care systems, our providers and, most importantly, our patients to incorporate this discovery into improved outcomes for all women.”
Related Links:
MIT
Channels
Artificial Intelligence
view channel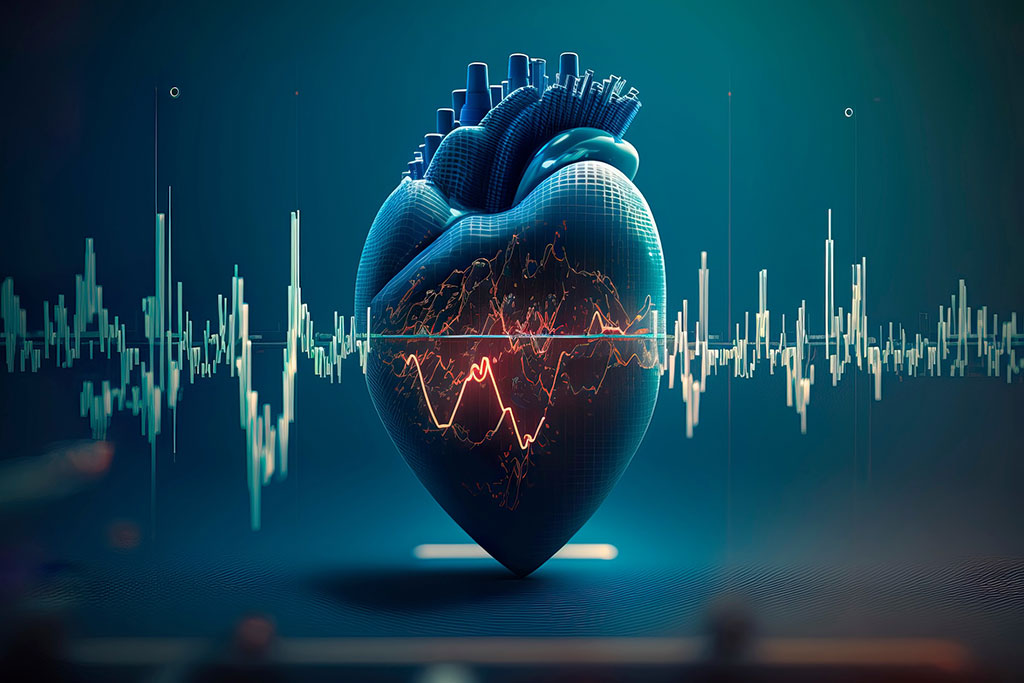
AI-Powered Algorithm to Revolutionize Detection of Atrial Fibrillation
Atrial fibrillation (AFib), a condition characterized by an irregular and often rapid heart rate, is linked to increased risks of stroke and heart failure. This is because the irregular heartbeat in AFib... Read more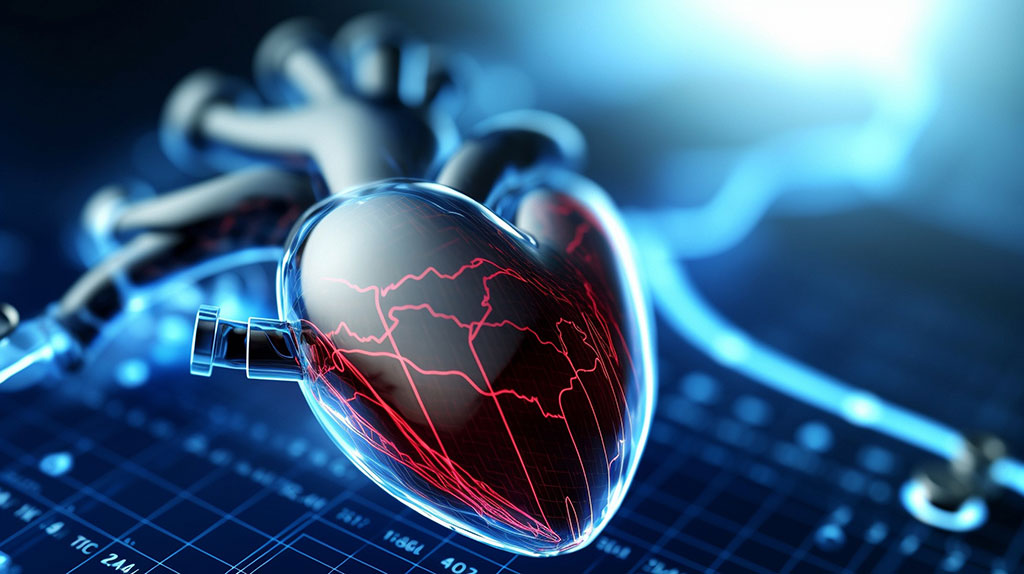
AI Diagnostic Tool Accurately Detects Valvular Disorders Often Missed by Doctors
Doctors generally use stethoscopes to listen for the characteristic lub-dub sounds made by heart valves opening and closing. They also listen for less prominent sounds that indicate problems with these valves.... Read moreCritical Care
view channel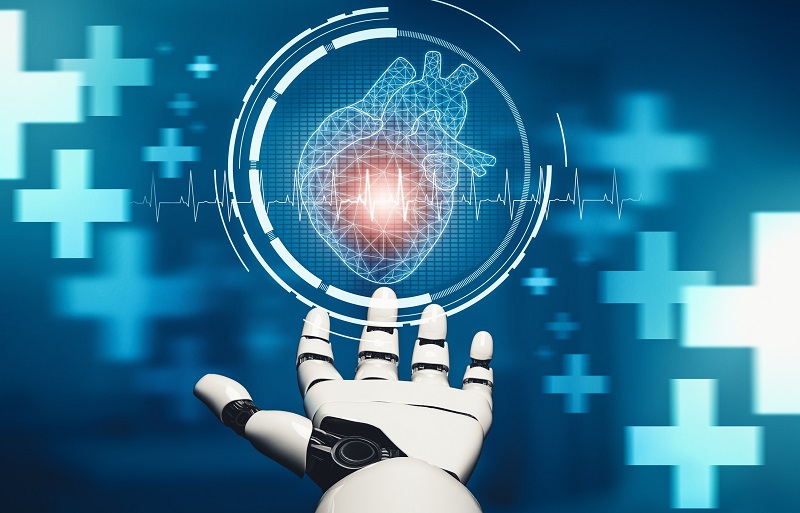
Powerful AI Risk Assessment Tool Predicts Outcomes in Heart Failure Patients
Heart failure is a serious condition where the heart cannot pump sufficient blood to meet the body's needs, leading to symptoms like fatigue, weakness, and swelling in the legs and feet, and it can ultimately... Read more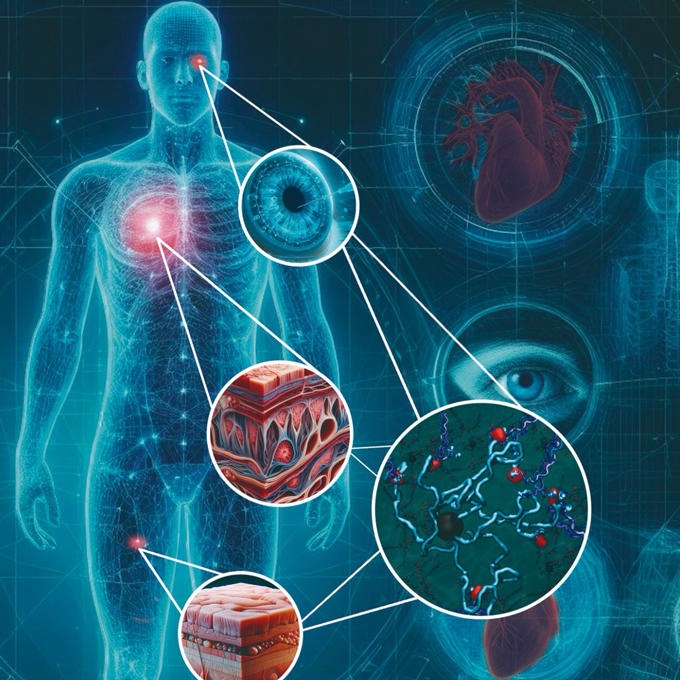
Peptide-Based Hydrogels Repair Damaged Organs and Tissues On-The-Spot
Scientists have ingeniously combined biomedical expertise with nature-inspired engineering to develop a jelly-like material that holds significant promise for immediate repairs to a wide variety of damaged... Read more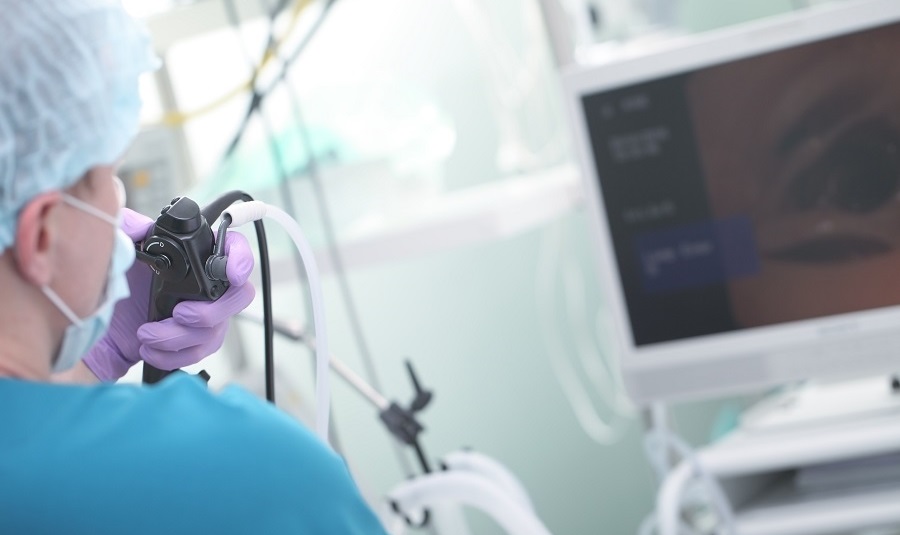
One-Hour Endoscopic Procedure Could Eliminate Need for Insulin for Type 2 Diabetes
Over 37 million Americans are diagnosed with diabetes, and more than 90% of these cases are Type 2 diabetes. This form of diabetes is most commonly seen in individuals over 45, though an increasing number... Read moreSurgical Techniques
view channel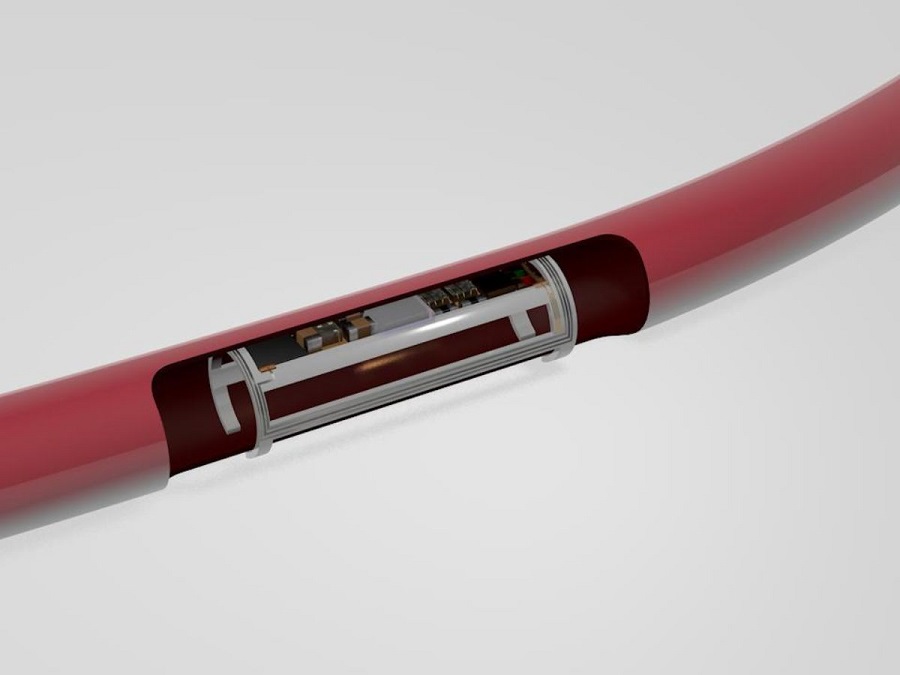
Miniaturized Implantable Multi-Sensors Device to Monitor Vessels Health
Researchers have embarked on a project to develop a multi-sensing device that can be implanted into blood vessels like peripheral veins or arteries to monitor a range of bodily parameters and overall health status.... Read more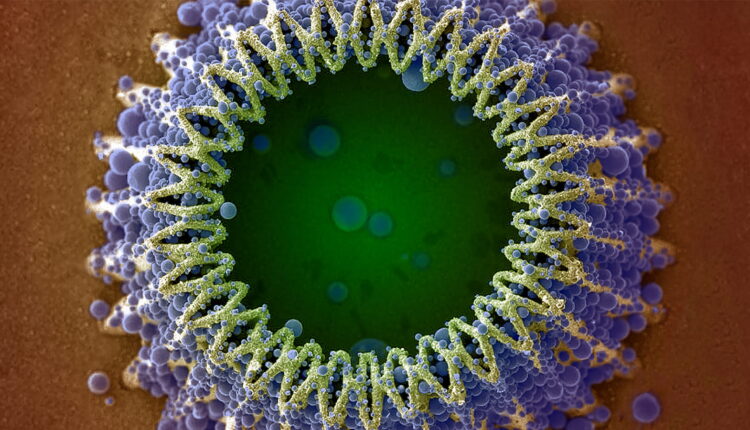
Tiny Robots Made Out Of Carbon Could Conduct Colonoscopy, Pelvic Exam or Blood Test
Researchers at the University of Alberta (Edmonton, AB, Canada) are developing cutting-edge robots so tiny that they are invisible to the naked eye but are capable of traveling through the human body to... Read more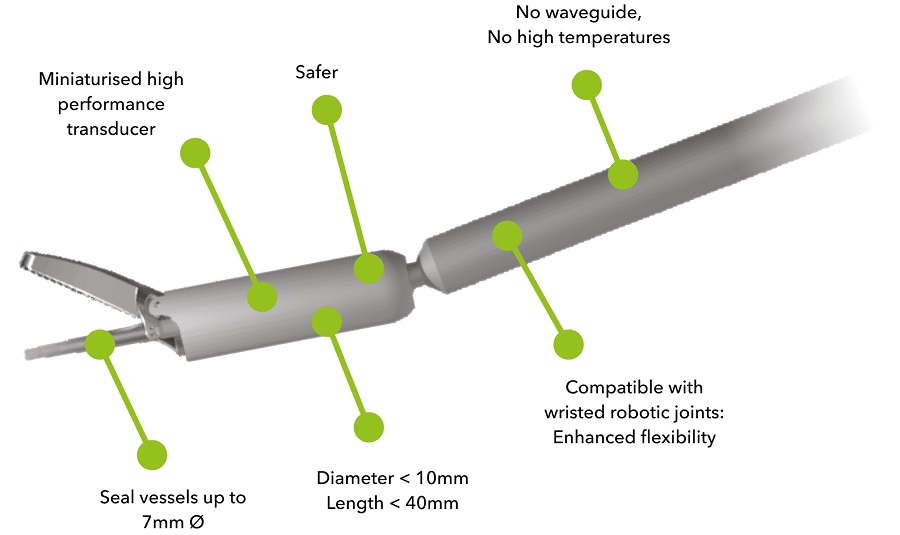
Miniaturized Ultrasonic Scalpel Enables Faster and Safer Robotic-Assisted Surgery
Robot-assisted surgery (RAS) has gained significant popularity in recent years and is now extensively used across various surgical fields such as urology, gynecology, and cardiology. These surgeries, performed... Read morePatient Care
view channel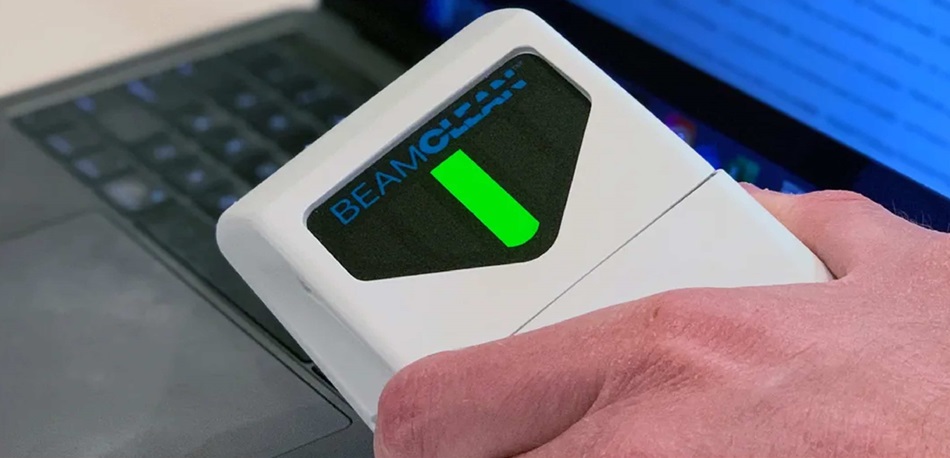
First-Of-Its-Kind Portable Germicidal Light Technology Disinfects High-Touch Clinical Surfaces in Seconds
Reducing healthcare-acquired infections (HAIs) remains a pressing issue within global healthcare systems. In the United States alone, 1.7 million patients contract HAIs annually, leading to approximately... Read more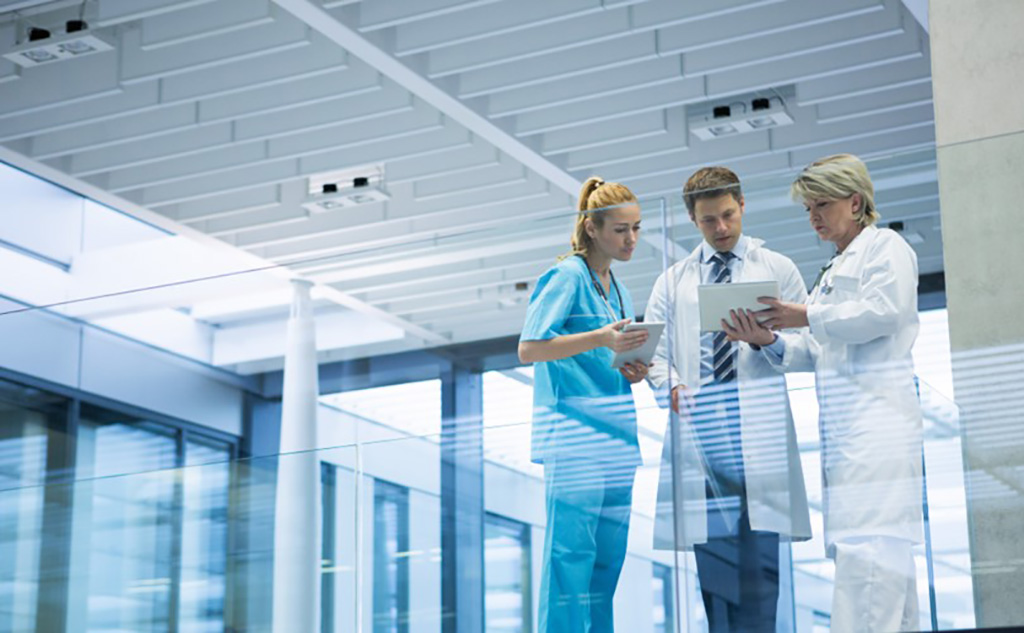
Surgical Capacity Optimization Solution Helps Hospitals Boost OR Utilization
An innovative solution has the capability to transform surgical capacity utilization by targeting the root cause of surgical block time inefficiencies. Fujitsu Limited’s (Tokyo, Japan) Surgical Capacity... Read more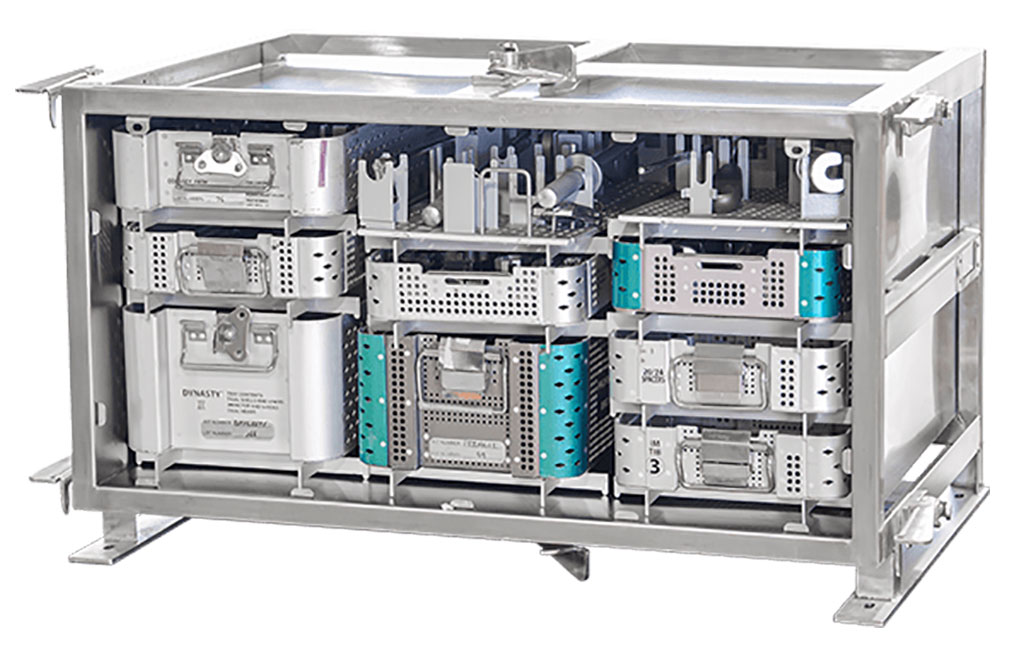
Game-Changing Innovation in Surgical Instrument Sterilization Significantly Improves OR Throughput
A groundbreaking innovation enables hospitals to significantly improve instrument processing time and throughput in operating rooms (ORs) and sterile processing departments. Turbett Surgical, Inc.... Read moreHealth IT
view channel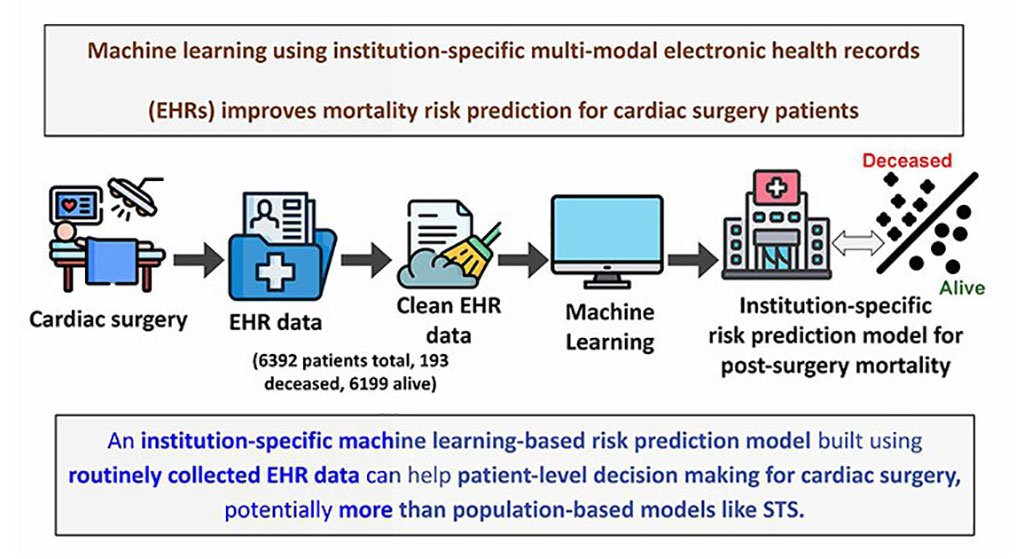
Machine Learning Model Improves Mortality Risk Prediction for Cardiac Surgery Patients
Machine learning algorithms have been deployed to create predictive models in various medical fields, with some demonstrating improved outcomes compared to their standard-of-care counterparts.... Read more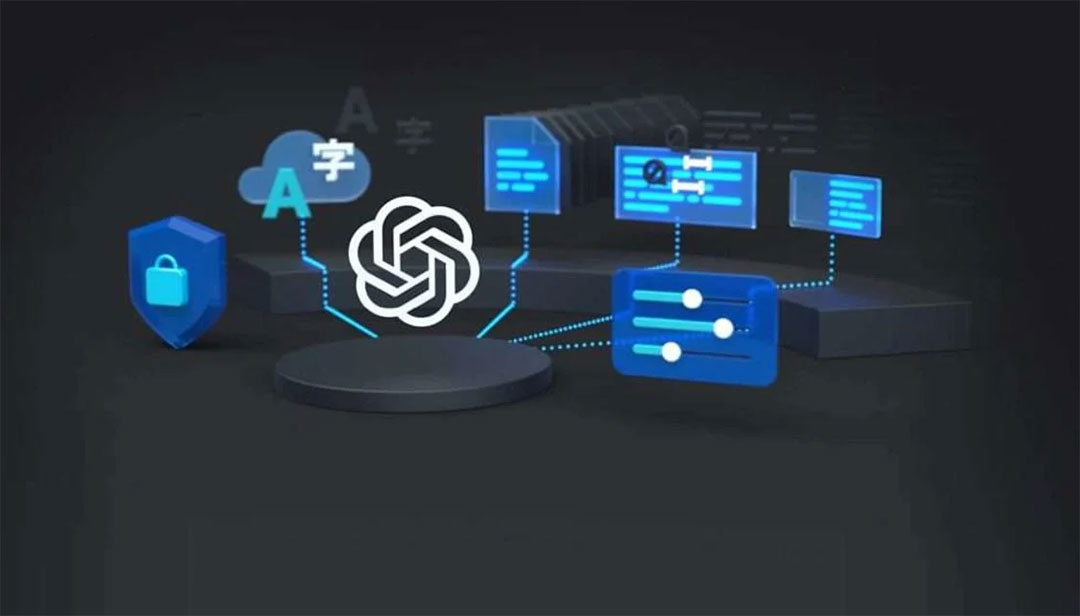
Strategic Collaboration to Develop and Integrate Generative AI into Healthcare
Top industry experts have underscored the immediate requirement for healthcare systems and hospitals to respond to severe cost and margin pressures. Close to half of U.S. hospitals ended 2022 in the red... Read more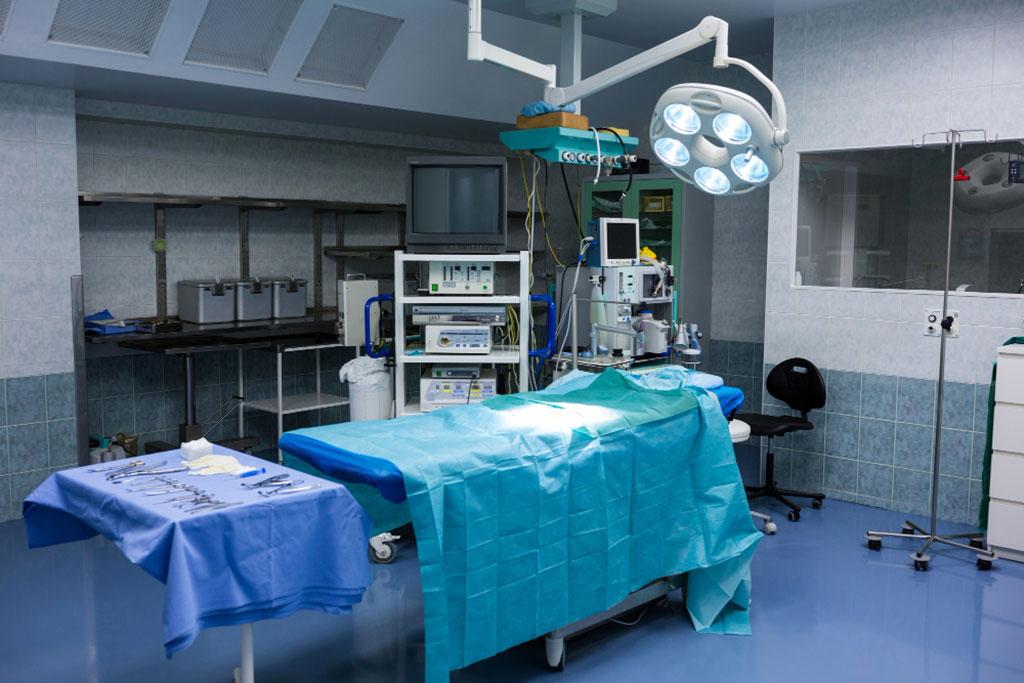
AI-Enabled Operating Rooms Solution Helps Hospitals Maximize Utilization and Unlock Capacity
For healthcare organizations, optimizing operating room (OR) utilization during prime time hours is a complex challenge. Surgeons and clinics face difficulties in finding available slots for booking cases,... Read more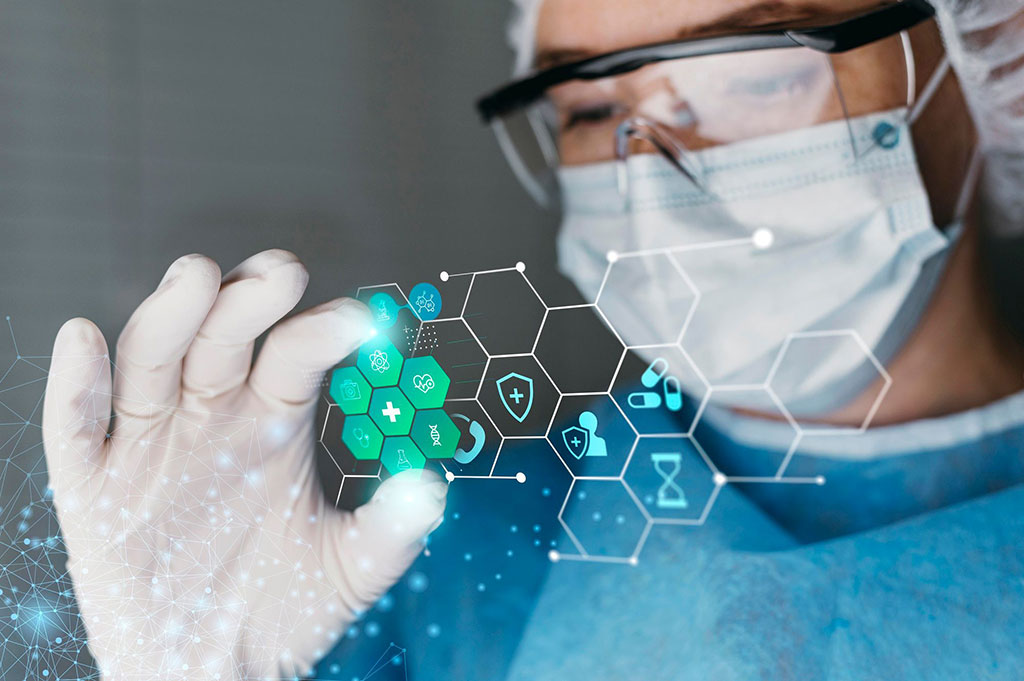
AI Predicts Pancreatic Cancer Three Years before Diagnosis from Patients’ Medical Records
Screening for common cancers like breast, cervix, and prostate cancer relies on relatively simple and highly effective techniques, such as mammograms, Pap smears, and blood tests. These methods have revolutionized... Read morePoint of Care
view channel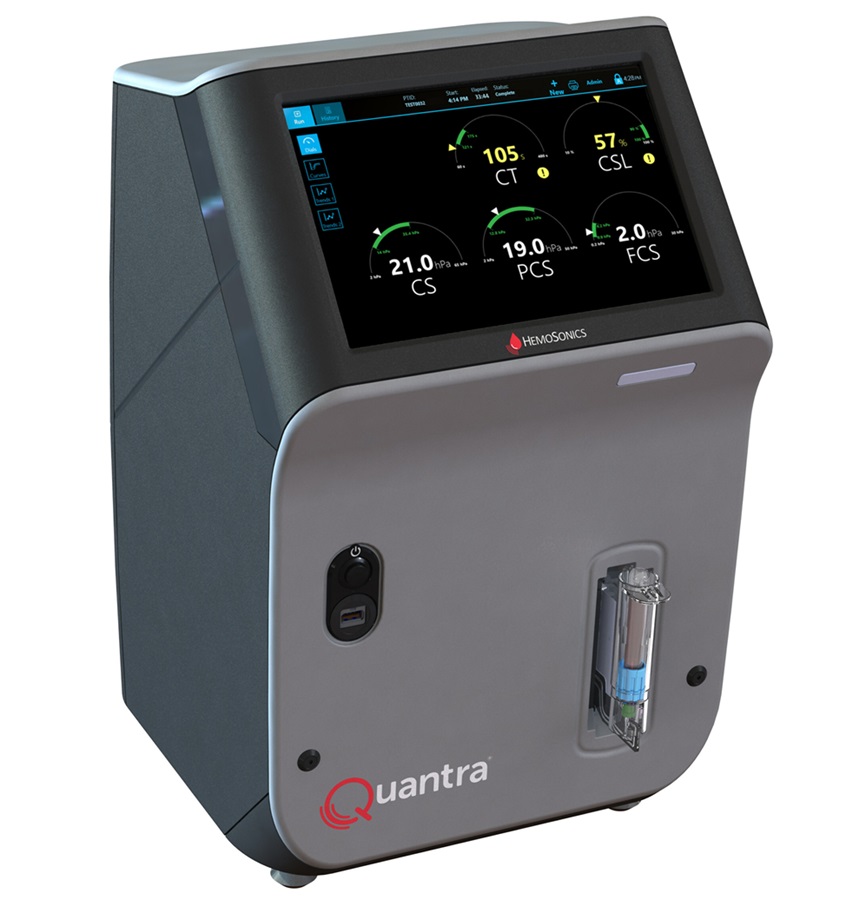
Critical Bleeding Management System to Help Hospitals Further Standardize Viscoelastic Testing
Surgical procedures are often accompanied by significant blood loss and the subsequent high likelihood of the need for allogeneic blood transfusions. These transfusions, while critical, are linked to various... Read more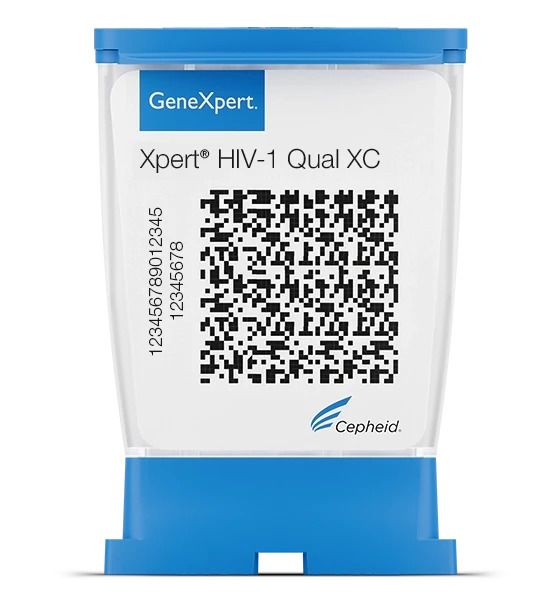
Point of Care HIV Test Enables Early Infection Diagnosis for Infants
Early diagnosis and initiation of treatment are crucial for the survival of infants infected with HIV (human immunodeficiency virus). Without treatment, approximately 50% of infants who acquire HIV during... Read more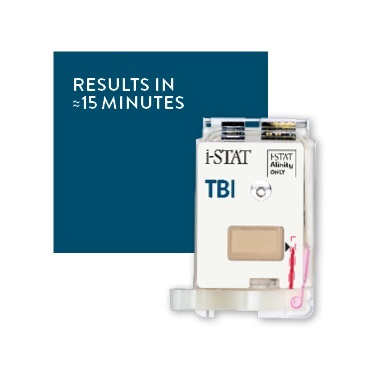
Whole Blood Rapid Test Aids Assessment of Concussion at Patient's Bedside
In the United States annually, approximately five million individuals seek emergency department care for traumatic brain injuries (TBIs), yet over half of those suspecting a concussion may never get it checked.... Read more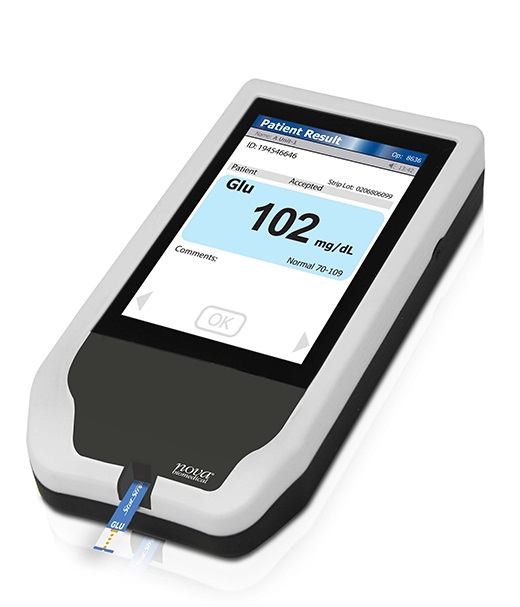
New Generation Glucose Hospital Meter System Ensures Accurate, Interference-Free and Safe Use
A new generation glucose hospital meter system now comes with several features that make hospital glucose testing easier and more secure while continuing to offer accuracy, freedom from interference, and... Read moreBusiness
view channel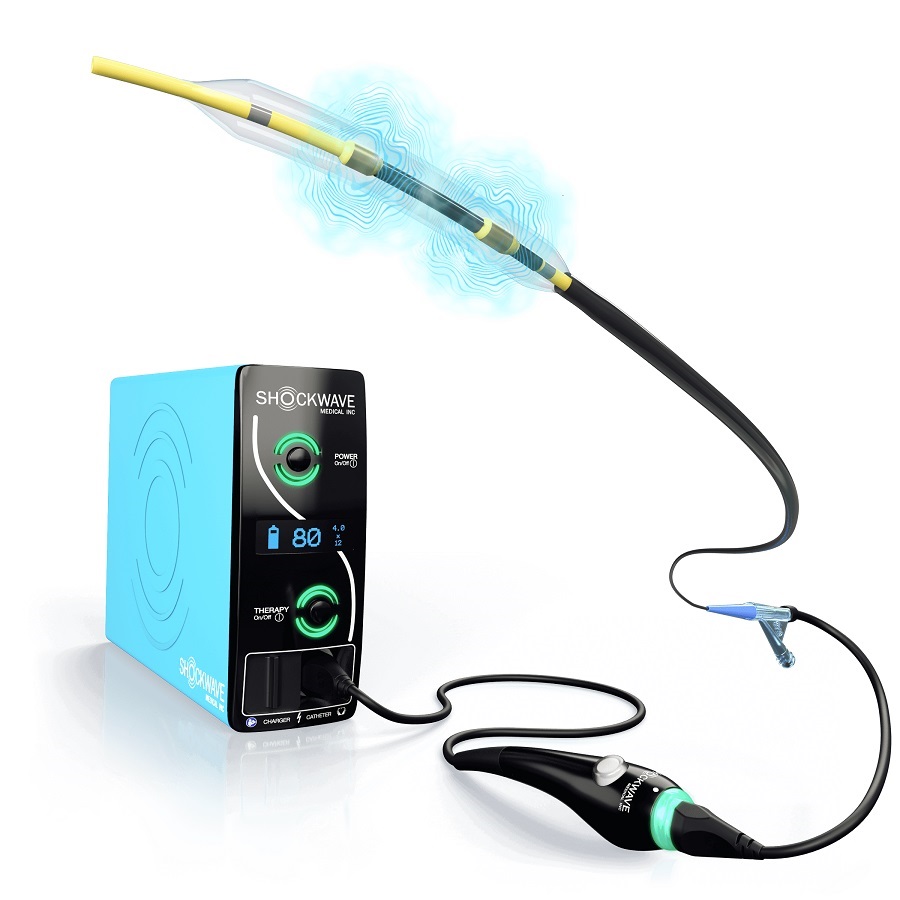
Johnson & Johnson Acquires Cardiovascular Medical Device Company Shockwave Medical
Johnson & Johnson (New Brunswick, N.J., USA) and Shockwave Medical (Santa Clara, CA, USA) have entered into a definitive agreement under which Johnson & Johnson will acquire all of Shockwave’s... Read more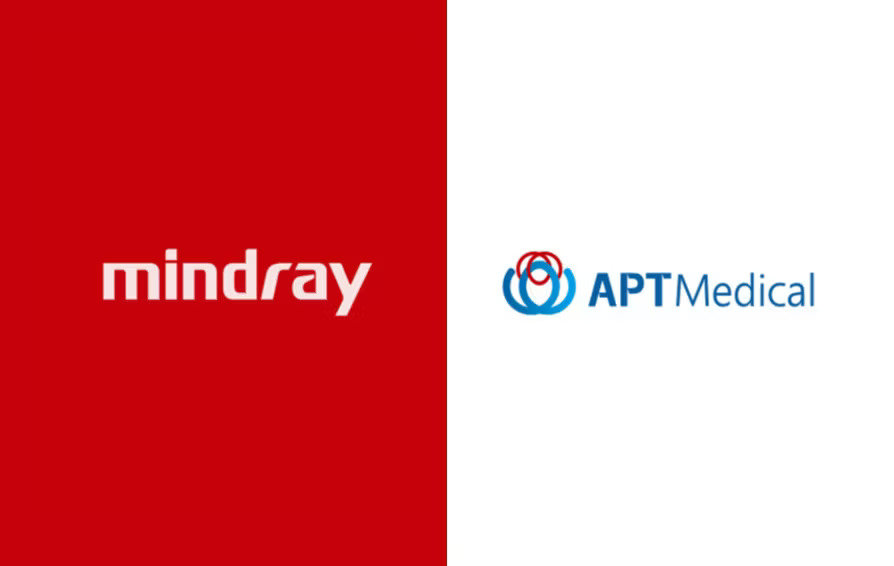