Google Shows AI Can Predict Lung Cancer from CT Scans
By HospiMedica International staff writers Posted on 01 Jun 2019 |
Google LLC (Mountain View, CA, USA) has shared new research demonstrating how artificial intelligence (AI) can predict lung cancer to boost the chances of survival for people at risk across the world.
Since 2017, Google has been exploring how AI can be used to address the challenges in screening people at a high-risk for lung cancer with lower dose CT screening that leads to unclear diagnosis, subsequent unnecessary procedures, and financial costs. Google used advances in 3D volumetric modeling along with datasets from its partners for modeling lung cancer prediction and laying the groundwork for future clinical testing.
Generally, radiologists go through hundreds of 2D images within a single CT scan with cancer being miniscule and hard to spot. Google researchers created a model that can generate the overall lung cancer malignancy prediction (viewed in 3D volume) as well as identify subtle malignant tissue in the lungs (lung nodules). The model can also factor in information from previous scans, which can be useful in predicting lung cancer risk as the growth rate of suspicious lung nodules can be an indicator of malignancy.
The researchers leveraged 45,856 de-identified chest CT screening cases and validated the results with a second dataset and also compared their results against six US board-certified radiologists. They found that when using a single CT scan for diagnosis, their model performed on par or better than the six radiologists and detected 5% more cancer cases while reducing false-positive exams by more than 11% as compared to unassisted radiologists in the study. Google’s approach achieved an AUC (a common metric used in machine learning that provides an aggregate measure for classification performance) of 94.4%.
With only 2-4% of eligible patients in the US being currently screened for lung cancer, Google’s research demonstrates the potential for AI to increase accuracy as well as consistency, which could help accelerate the adoption of lung cancer screening globally. Google now plans to conduct further studies to assess its impact and utility in clinical practice. It is collaborating with Google Cloud Healthcare and Life Sciences team to serve the model through the Cloud Healthcare API and is holding discussions with its partners across the world to continue additional clinical validation research and deployment.
Related Links:
Google
Since 2017, Google has been exploring how AI can be used to address the challenges in screening people at a high-risk for lung cancer with lower dose CT screening that leads to unclear diagnosis, subsequent unnecessary procedures, and financial costs. Google used advances in 3D volumetric modeling along with datasets from its partners for modeling lung cancer prediction and laying the groundwork for future clinical testing.
Generally, radiologists go through hundreds of 2D images within a single CT scan with cancer being miniscule and hard to spot. Google researchers created a model that can generate the overall lung cancer malignancy prediction (viewed in 3D volume) as well as identify subtle malignant tissue in the lungs (lung nodules). The model can also factor in information from previous scans, which can be useful in predicting lung cancer risk as the growth rate of suspicious lung nodules can be an indicator of malignancy.
The researchers leveraged 45,856 de-identified chest CT screening cases and validated the results with a second dataset and also compared their results against six US board-certified radiologists. They found that when using a single CT scan for diagnosis, their model performed on par or better than the six radiologists and detected 5% more cancer cases while reducing false-positive exams by more than 11% as compared to unassisted radiologists in the study. Google’s approach achieved an AUC (a common metric used in machine learning that provides an aggregate measure for classification performance) of 94.4%.
With only 2-4% of eligible patients in the US being currently screened for lung cancer, Google’s research demonstrates the potential for AI to increase accuracy as well as consistency, which could help accelerate the adoption of lung cancer screening globally. Google now plans to conduct further studies to assess its impact and utility in clinical practice. It is collaborating with Google Cloud Healthcare and Life Sciences team to serve the model through the Cloud Healthcare API and is holding discussions with its partners across the world to continue additional clinical validation research and deployment.
Related Links:
Latest AI News
- AI-Powered Algorithm to Revolutionize Detection of Atrial Fibrillation
- AI Diagnostic Tool Accurately Detects Valvular Disorders Often Missed by Doctors
- New Model Predicts 10 Year Breast Cancer Risk
- AI Tool Accurately Predicts Cancer Three Years Prior to Diagnosis
- Ground-Breaking Tool Predicts 10-Year Risk of Esophageal Cancer
- AI Tool Analyzes Capsule Endoscopy Videos for Accurately Predicting Patient Outcomes for Crohn’s Disease
Channels
Artificial Intelligence
view channel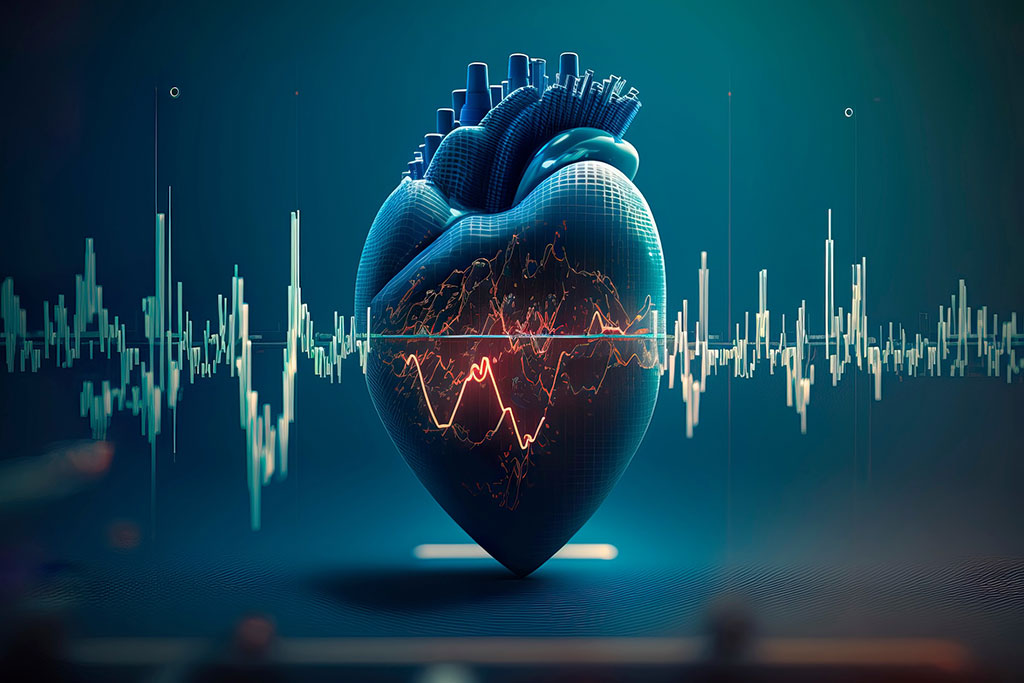
AI-Powered Algorithm to Revolutionize Detection of Atrial Fibrillation
Atrial fibrillation (AFib), a condition characterized by an irregular and often rapid heart rate, is linked to increased risks of stroke and heart failure. This is because the irregular heartbeat in AFib... Read more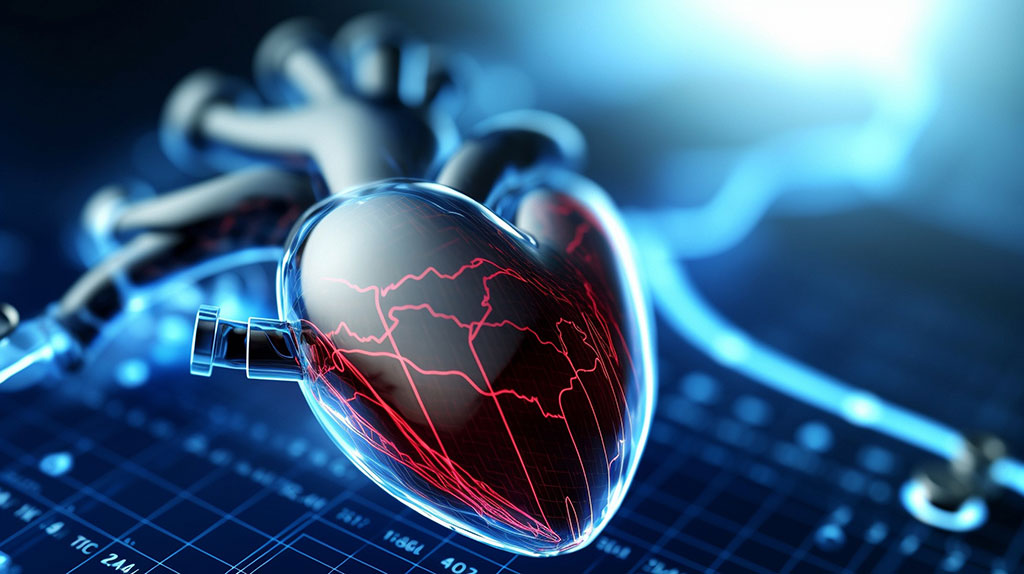
AI Diagnostic Tool Accurately Detects Valvular Disorders Often Missed by Doctors
Doctors generally use stethoscopes to listen for the characteristic lub-dub sounds made by heart valves opening and closing. They also listen for less prominent sounds that indicate problems with these valves.... Read moreCritical Care
view channel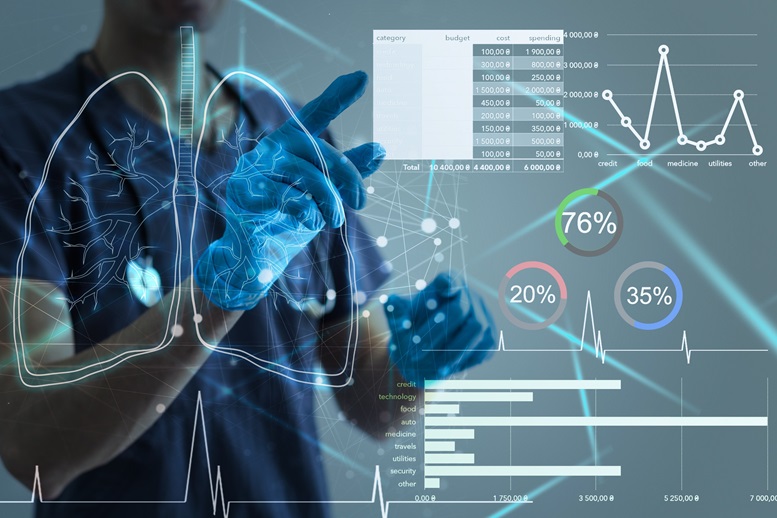
Machine Learning Tool Identifies Rare, Undiagnosed Immune Disorders from Patient EHRs
Patients suffering from rare diseases often endure extensive delays in receiving accurate diagnoses and treatments, which can lead to unnecessary tests, worsening health, psychological strain, and significant... Read more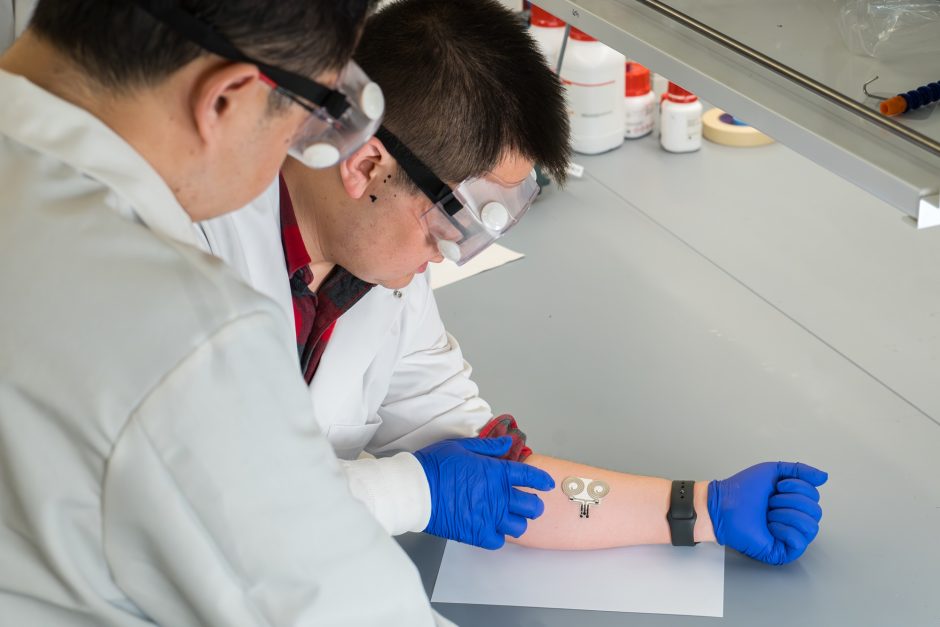
On-Skin Wearable Bioelectronic Device Paves Way for Intelligent Implants
A team of researchers at the University of Missouri (Columbia, MO, USA) has achieved a milestone in developing a state-of-the-art on-skin wearable bioelectronic device. This development comes from a lab... Read more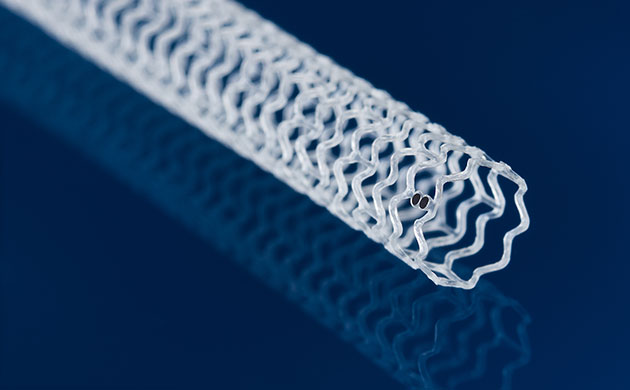
First-Of-Its-Kind Dissolvable Stent to Improve Outcomes for Patients with Severe PAD
Peripheral artery disease (PAD) affects millions and presents serious health risks, particularly its severe form, chronic limb-threatening ischemia (CLTI). CLTI develops when arteries are blocked by plaque,... Read more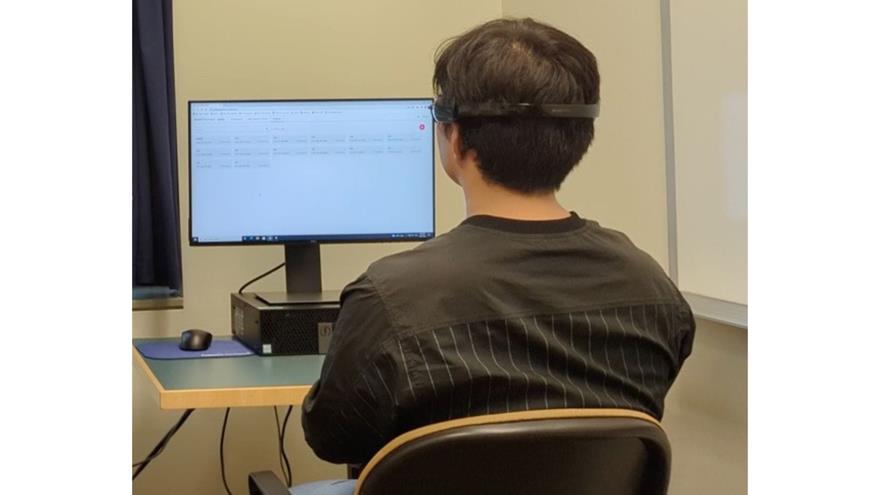
AI Brain-Age Estimation Technology Uses EEG Scans to Screen for Degenerative Diseases
As individuals age, so do their brains. Premature aging of the brain can lead to age-related conditions such as mild cognitive impairment, dementia, or Parkinson's disease. The ability to determine "brain... Read moreSurgical Techniques
view channel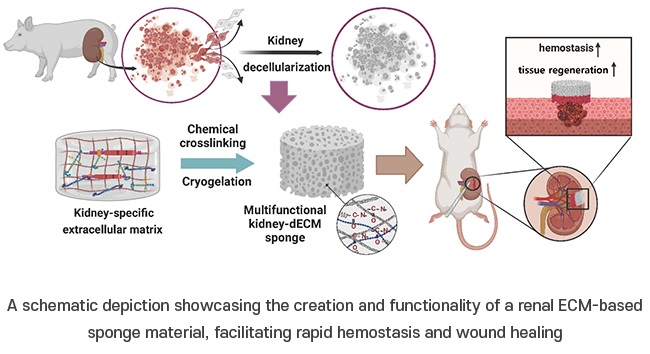
Porous Gel Sponge Facilitates Rapid Hemostasis and Wound Healing
The kidneys are essential organs that handle critical bodily functions, including waste elimination and blood pressure regulation. Often referred to as the silent organ because they typically do not manifest... Read more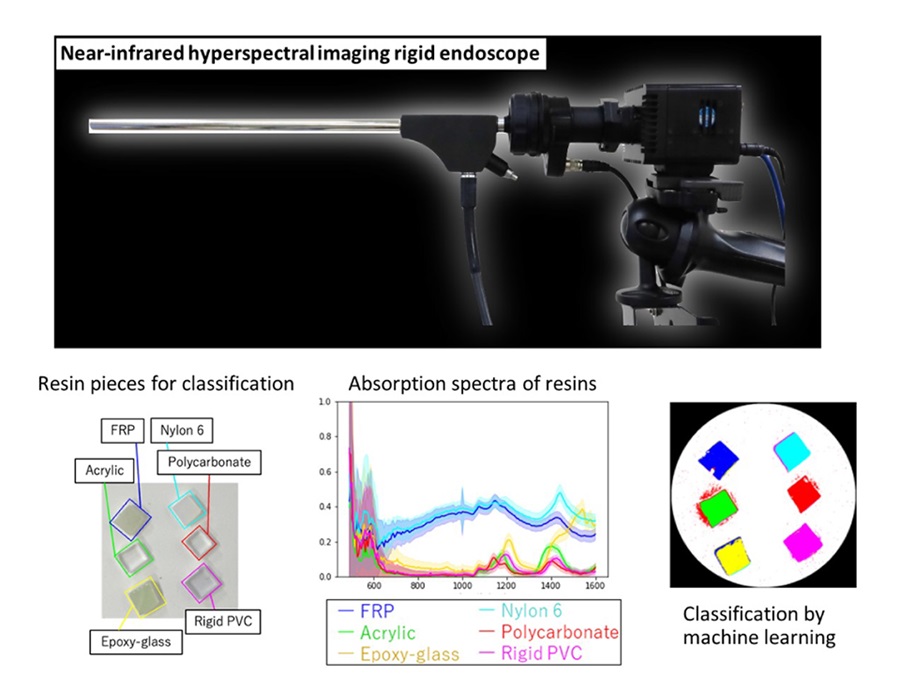
Novel Rigid Endoscope System Enables Deep Tissue Imaging During Surgery
Hyperspectral imaging (HSI) is an advanced technique that captures and processes information across a given electromagnetic spectrum. Near-infrared hyperspectral imaging (NIR-HSI) has particularly gained... Read more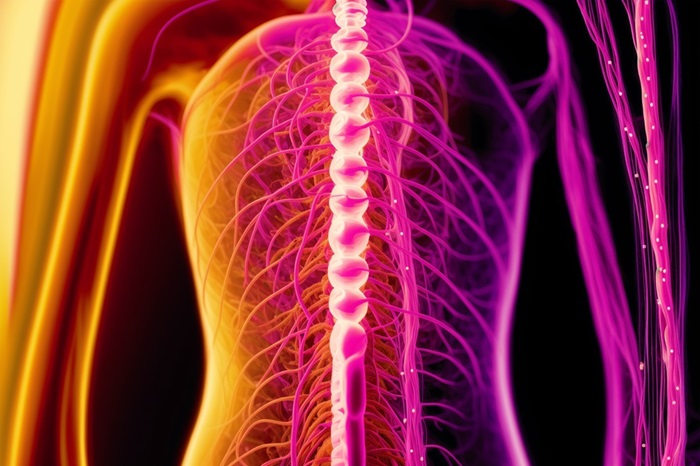
Robotic Nerve ‘Cuffs’ Could Treat Various Neurological Conditions
Electric nerve implants serve dual functions: they can either stimulate or block signals in specific nerves. For example, they may alleviate pain by inhibiting pain signals or restore movement in paralyzed... Read more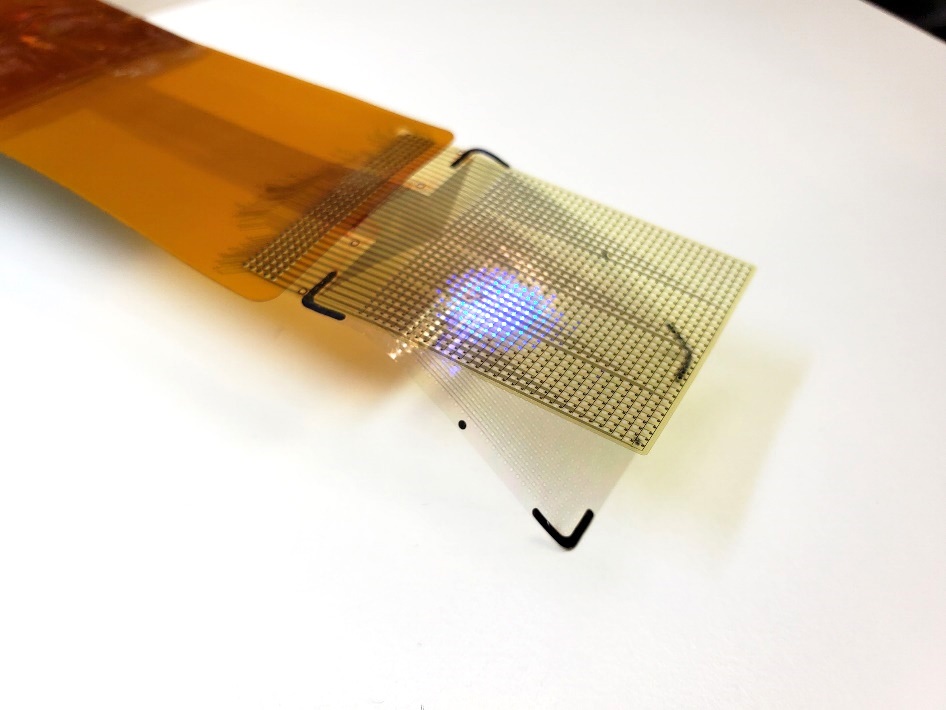
Flexible Microdisplay Visualizes Brain Activity in Real-Time To Guide Neurosurgeons
During brain surgery, neurosurgeons need to identify and preserve regions responsible for critical functions while removing harmful tissue. Traditionally, neurosurgeons rely on a team of electrophysiologists,... Read morePatient Care
view channel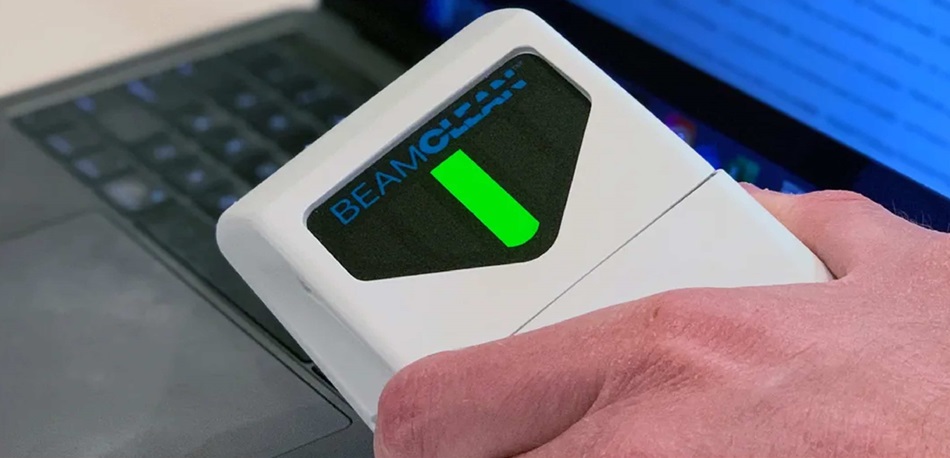
First-Of-Its-Kind Portable Germicidal Light Technology Disinfects High-Touch Clinical Surfaces in Seconds
Reducing healthcare-acquired infections (HAIs) remains a pressing issue within global healthcare systems. In the United States alone, 1.7 million patients contract HAIs annually, leading to approximately... Read more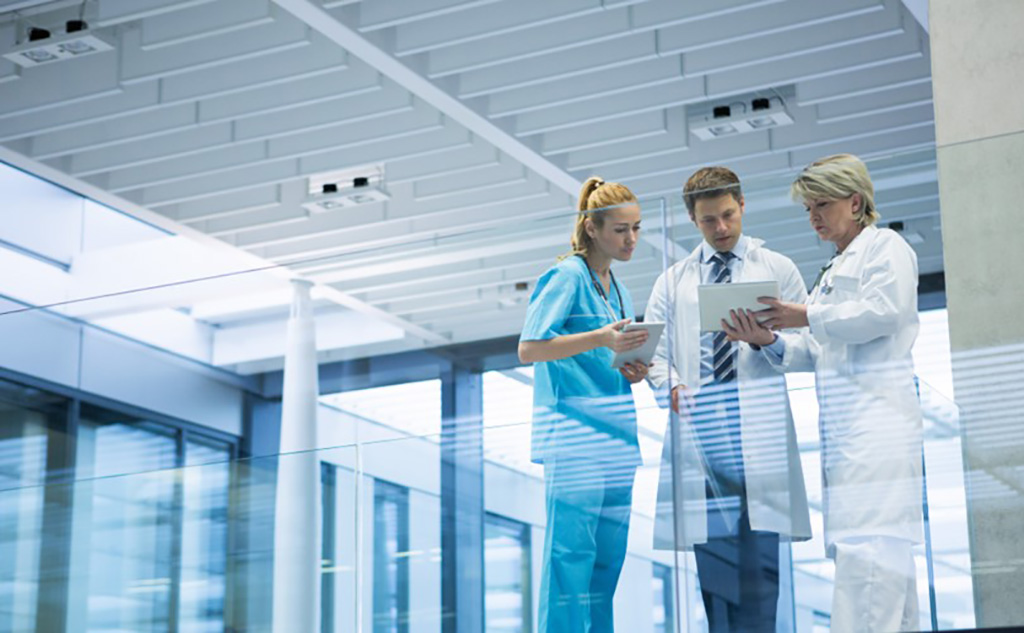
Surgical Capacity Optimization Solution Helps Hospitals Boost OR Utilization
An innovative solution has the capability to transform surgical capacity utilization by targeting the root cause of surgical block time inefficiencies. Fujitsu Limited’s (Tokyo, Japan) Surgical Capacity... Read more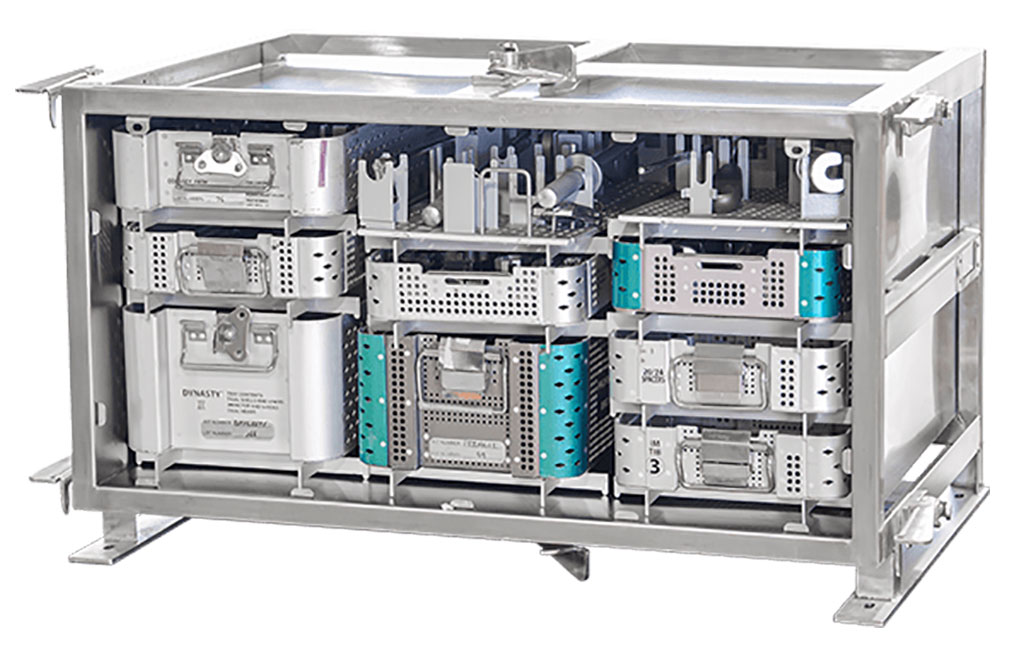
Game-Changing Innovation in Surgical Instrument Sterilization Significantly Improves OR Throughput
A groundbreaking innovation enables hospitals to significantly improve instrument processing time and throughput in operating rooms (ORs) and sterile processing departments. Turbett Surgical, Inc.... Read moreHealth IT
view channel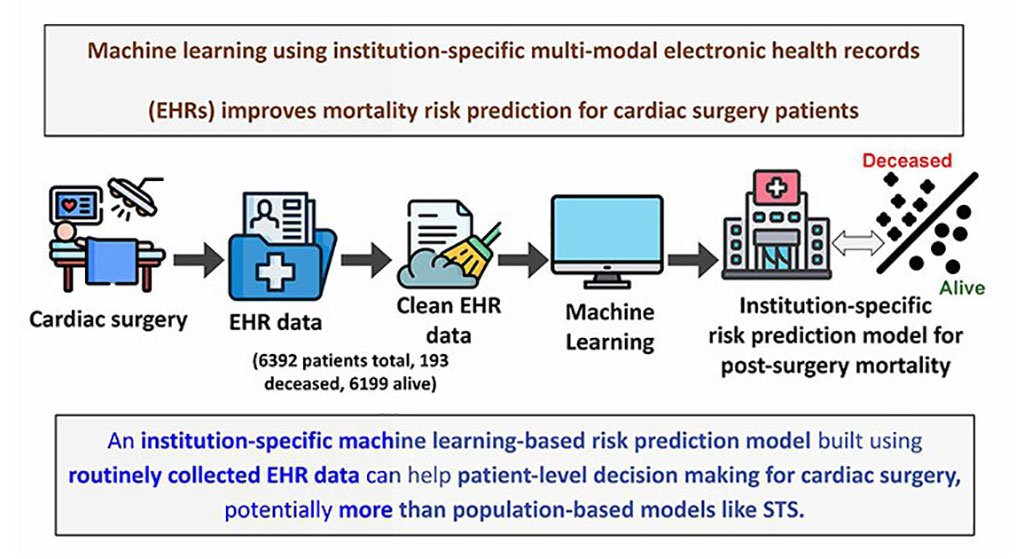
Machine Learning Model Improves Mortality Risk Prediction for Cardiac Surgery Patients
Machine learning algorithms have been deployed to create predictive models in various medical fields, with some demonstrating improved outcomes compared to their standard-of-care counterparts.... Read more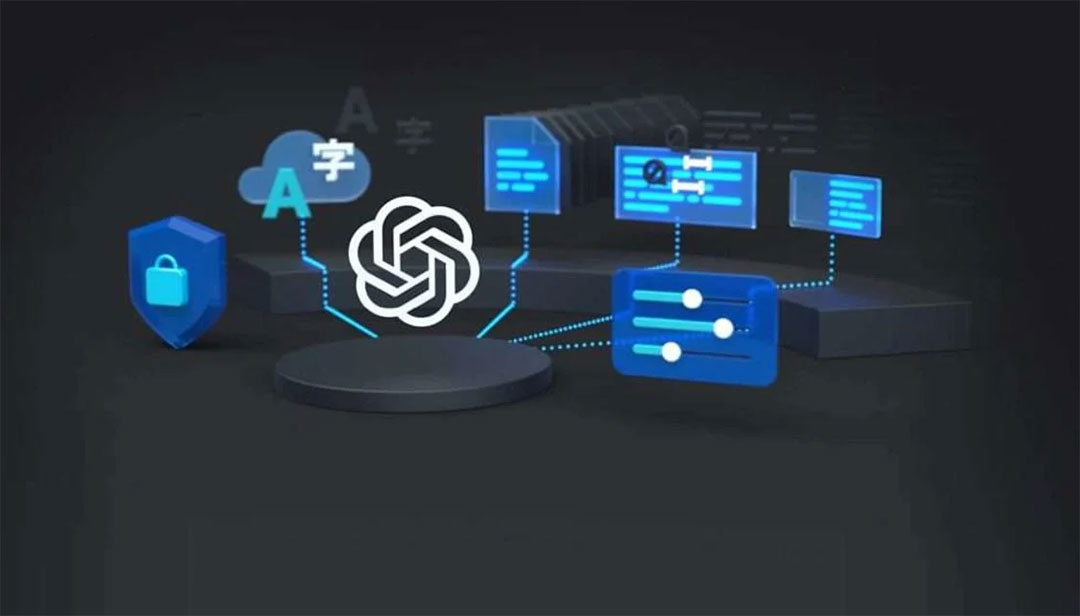
Strategic Collaboration to Develop and Integrate Generative AI into Healthcare
Top industry experts have underscored the immediate requirement for healthcare systems and hospitals to respond to severe cost and margin pressures. Close to half of U.S. hospitals ended 2022 in the red... Read more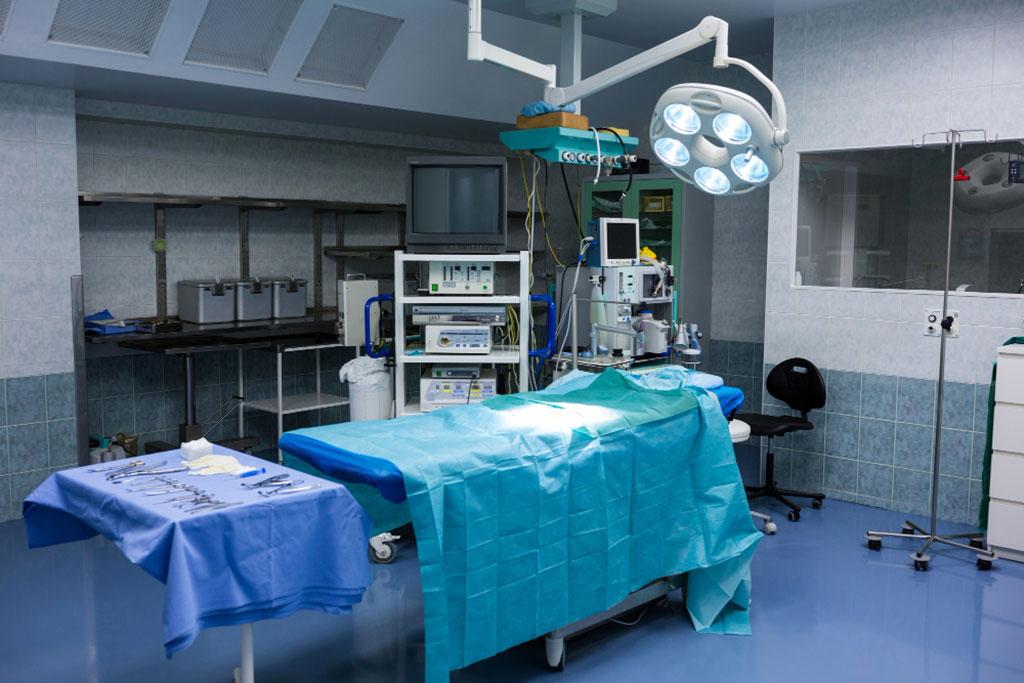
AI-Enabled Operating Rooms Solution Helps Hospitals Maximize Utilization and Unlock Capacity
For healthcare organizations, optimizing operating room (OR) utilization during prime time hours is a complex challenge. Surgeons and clinics face difficulties in finding available slots for booking cases,... Read more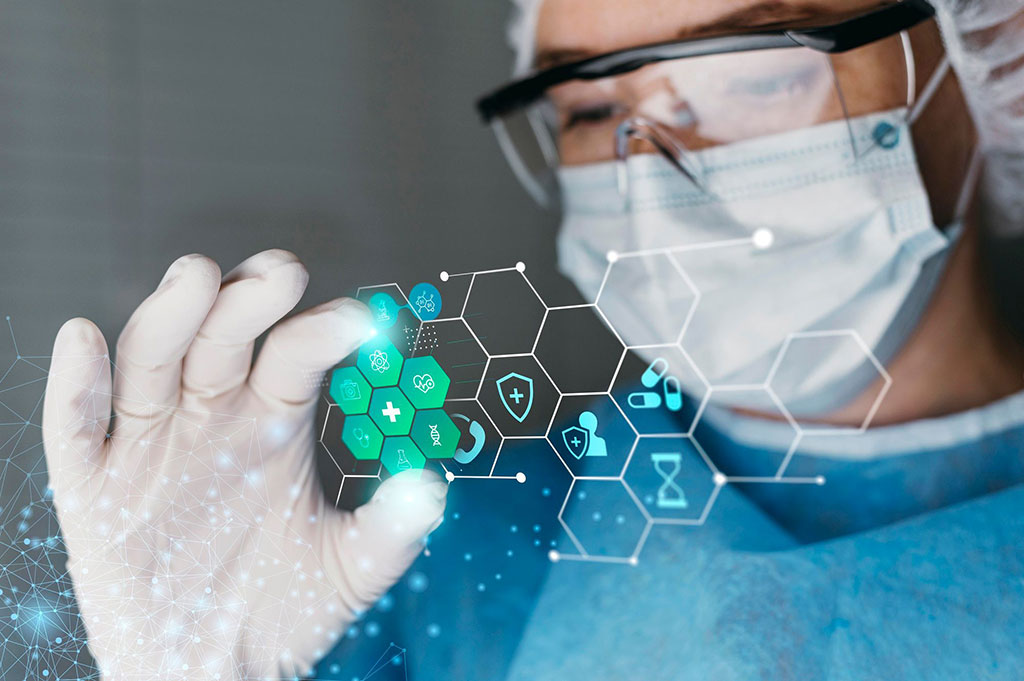
AI Predicts Pancreatic Cancer Three Years before Diagnosis from Patients’ Medical Records
Screening for common cancers like breast, cervix, and prostate cancer relies on relatively simple and highly effective techniques, such as mammograms, Pap smears, and blood tests. These methods have revolutionized... Read morePoint of Care
view channel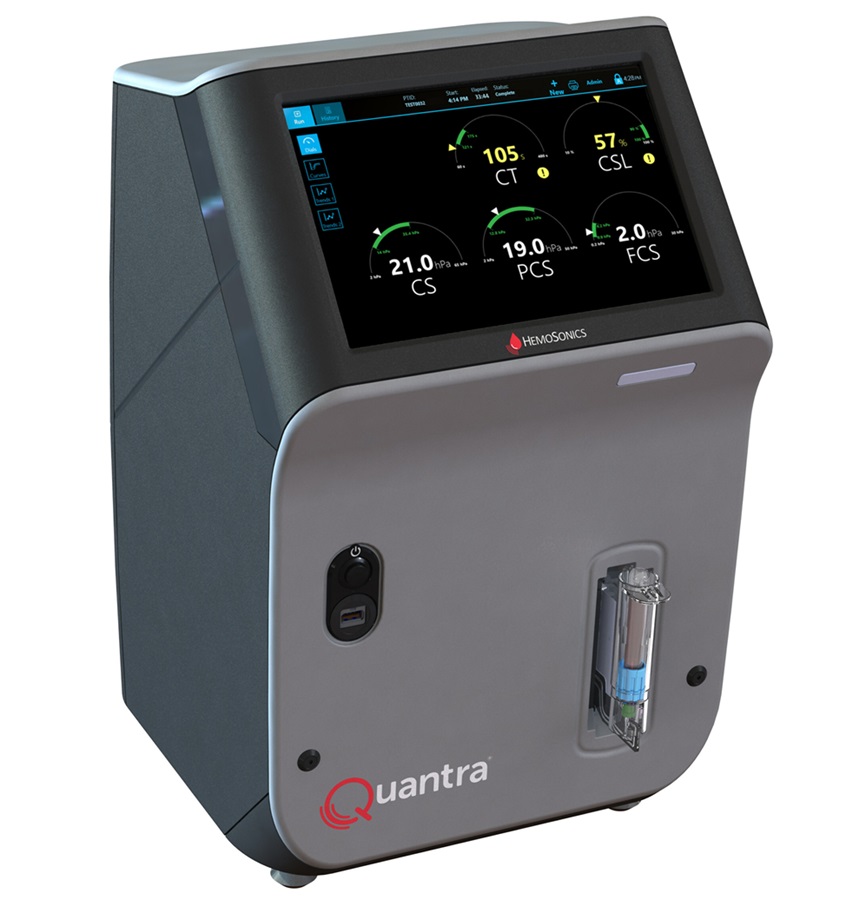
Critical Bleeding Management System to Help Hospitals Further Standardize Viscoelastic Testing
Surgical procedures are often accompanied by significant blood loss and the subsequent high likelihood of the need for allogeneic blood transfusions. These transfusions, while critical, are linked to various... Read more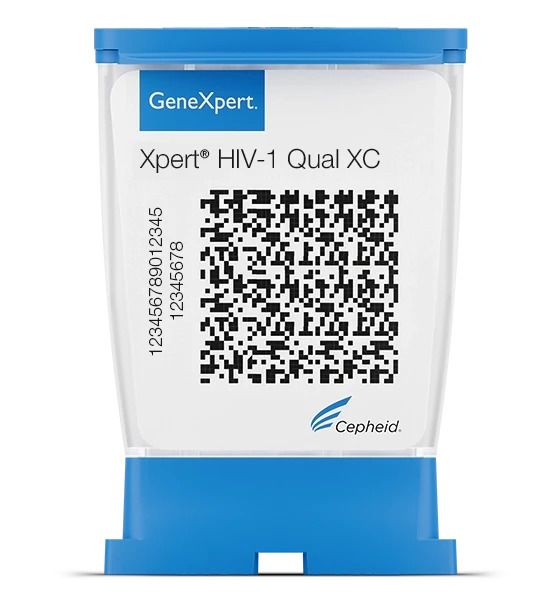
Point of Care HIV Test Enables Early Infection Diagnosis for Infants
Early diagnosis and initiation of treatment are crucial for the survival of infants infected with HIV (human immunodeficiency virus). Without treatment, approximately 50% of infants who acquire HIV during... Read more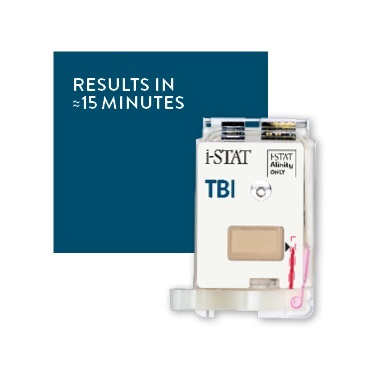
Whole Blood Rapid Test Aids Assessment of Concussion at Patient's Bedside
In the United States annually, approximately five million individuals seek emergency department care for traumatic brain injuries (TBIs), yet over half of those suspecting a concussion may never get it checked.... Read more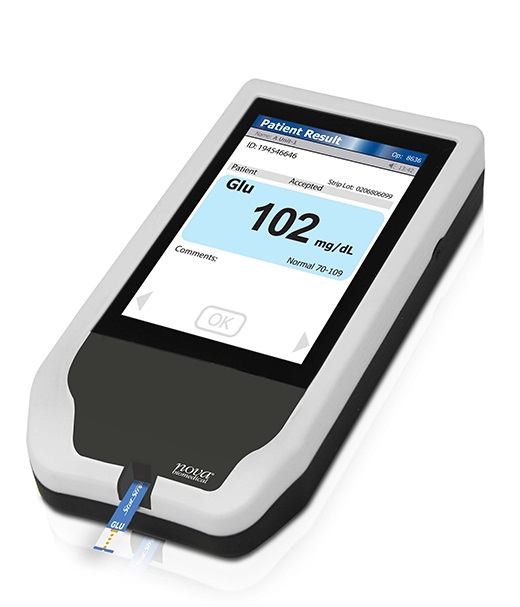
New Generation Glucose Hospital Meter System Ensures Accurate, Interference-Free and Safe Use
A new generation glucose hospital meter system now comes with several features that make hospital glucose testing easier and more secure while continuing to offer accuracy, freedom from interference, and... Read moreBusiness
view channel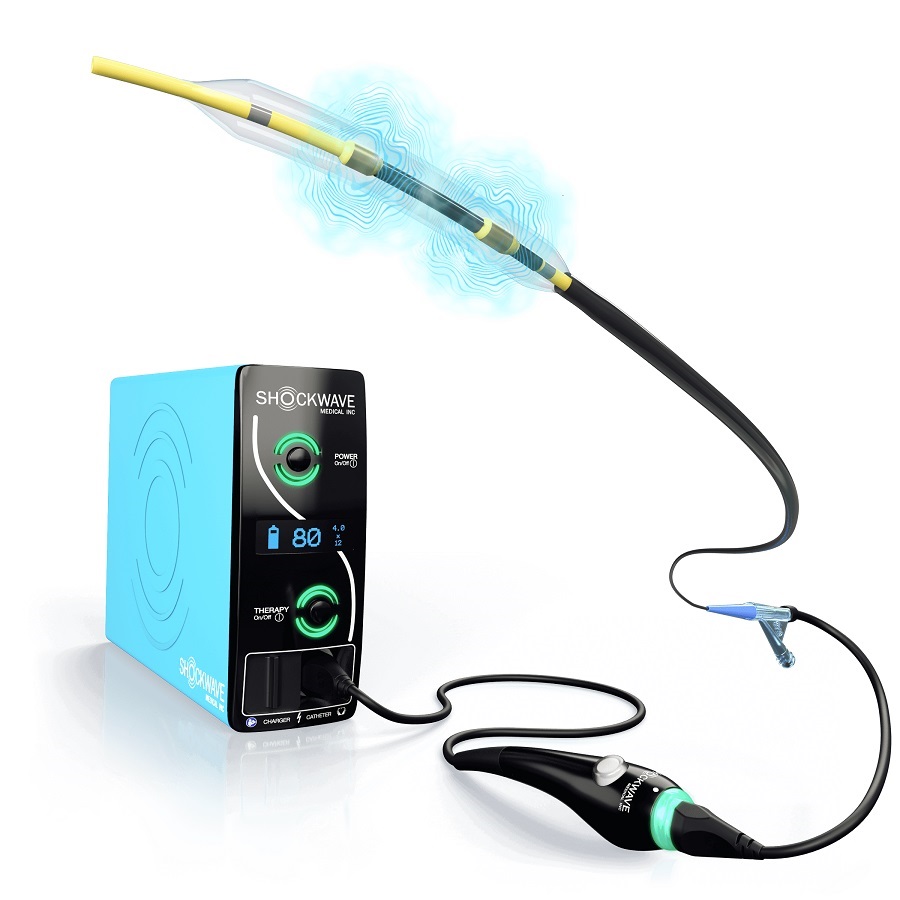
Johnson & Johnson Acquires Cardiovascular Medical Device Company Shockwave Medical
Johnson & Johnson (New Brunswick, N.J., USA) and Shockwave Medical (Santa Clara, CA, USA) have entered into a definitive agreement under which Johnson & Johnson will acquire all of Shockwave’s... Read more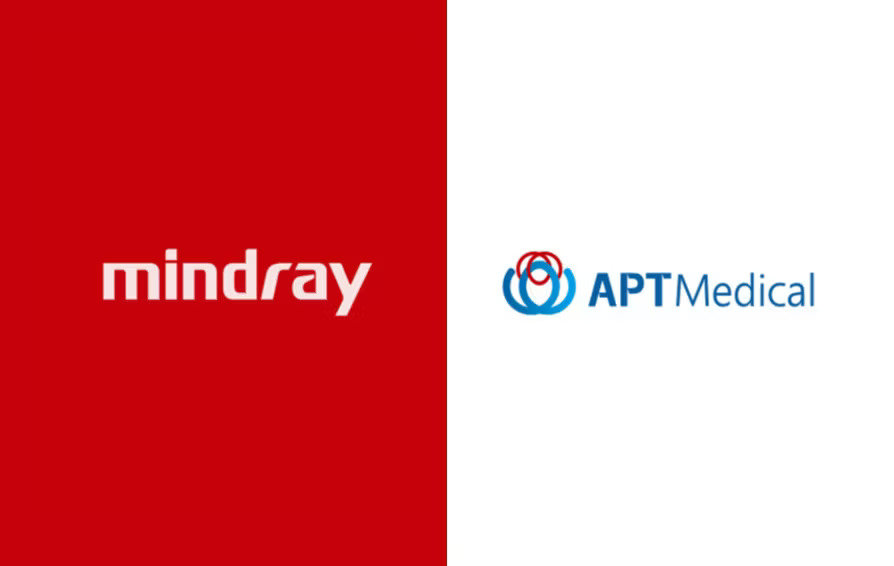