Artificial Intelligence Accurately Predicts Radiation Treatment Side Effects
By HospiMedica International staff writers Posted on 01 Oct 2019 |
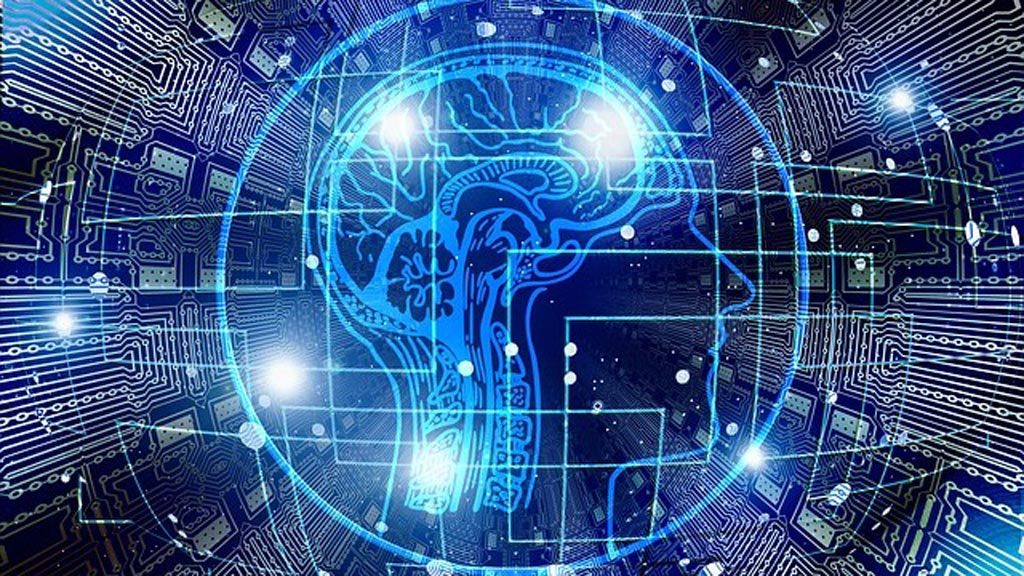
Image: New research has shown that a computer model can predict side effects associated with radiation therapy (Photo courtesy of Technology Networks).
Researchers from the University of Texas MD Anderson Cancer Center (Houston, Texas, USA) have demonstrated that a sophisticated computer model can accurately predict two of the most challenging side effects associated with radiation therapy for head and neck cancer. This precision oncology approach has the potential to better identify patients who might benefit from early interventions that could help prevent significant weight loss after treatment or reduce the need for feeding tube placement.
The team of researchers developed models to analyze large sets of data merged from three sources: electronic health records (Epic), an internal web-based charting tool (Brocade) and the record/verify system (Mosaiq). The data included more than 700 clinical and treatment variables for patients with head and neck cancer (75% male/25% female, with a median age of 62 years) who received more than 2,000 courses of radiation therapy (median dose 60 Gy) across five practice sites at MD Anderson from 2016 to 2018.
The researchers used the models to predict three endpoints: significant weight loss, feeding tube placement and unplanned hospitalizations. Results from the best-performing model were then validated against 225 subsequent consecutive radiation therapy treatments. Models with a performance rate that met a pre-specified threshold of area under the curve (AUC) of 0.70 or higher were considered clinically valid (an AUC score of 1.0 would mean the model’s predictions were 100% accurate, while a score of 0.0 would mean the predictions were never accurate). The models predicted the likelihood of significant weight loss (AUC = 0.751) and need for feeding tube placement (AUC = 0.755) with a high degree of accuracy.
“Being able to identify which patients are at greatest risk would allow radiation oncologists to take steps to prevent or mitigate these possible side effects,” said Jay Reddy, MD, PhD, an assistant professor of radiation oncology at The University of Texas MD Anderson Cancer Center and lead author on the study. “If the patient has an intermediate risk, and they might get through treatment without needing a feeding tube, we could take precautions such as setting them up with a nutritionist and providing them with nutritional supplements. If we know their risk for feeding tube placement is extremely high – a better than 50% chance they would need one – we could place it ahead of time so they wouldn’t have to be admitted to the hospital after treatment. We’d know to keep a closer eye on that patient.”
The machine learning approach cannot isolate the single-most predictive factor or combination of factors that lead to negative side effects, but can provide patients and their clinicians with a better understanding of what to expect during the course of treatment. In addition to predicting the likelihood of side effects, machine learning models could potentially predict which treatment plans would be most effective for different types of patients and allow for more personalized approaches to radiation oncology.
“Machine learning can make doctors more efficient and treatment safer by reducing the risk of error,” added Dr. Reddy. “It has the potential for influencing all aspects of radiation oncology today – anything where a computer can look at data and recognize a pattern.”
Related Links:
University of Texas MD Anderson Cancer Center
The team of researchers developed models to analyze large sets of data merged from three sources: electronic health records (Epic), an internal web-based charting tool (Brocade) and the record/verify system (Mosaiq). The data included more than 700 clinical and treatment variables for patients with head and neck cancer (75% male/25% female, with a median age of 62 years) who received more than 2,000 courses of radiation therapy (median dose 60 Gy) across five practice sites at MD Anderson from 2016 to 2018.
The researchers used the models to predict three endpoints: significant weight loss, feeding tube placement and unplanned hospitalizations. Results from the best-performing model were then validated against 225 subsequent consecutive radiation therapy treatments. Models with a performance rate that met a pre-specified threshold of area under the curve (AUC) of 0.70 or higher were considered clinically valid (an AUC score of 1.0 would mean the model’s predictions were 100% accurate, while a score of 0.0 would mean the predictions were never accurate). The models predicted the likelihood of significant weight loss (AUC = 0.751) and need for feeding tube placement (AUC = 0.755) with a high degree of accuracy.
“Being able to identify which patients are at greatest risk would allow radiation oncologists to take steps to prevent or mitigate these possible side effects,” said Jay Reddy, MD, PhD, an assistant professor of radiation oncology at The University of Texas MD Anderson Cancer Center and lead author on the study. “If the patient has an intermediate risk, and they might get through treatment without needing a feeding tube, we could take precautions such as setting them up with a nutritionist and providing them with nutritional supplements. If we know their risk for feeding tube placement is extremely high – a better than 50% chance they would need one – we could place it ahead of time so they wouldn’t have to be admitted to the hospital after treatment. We’d know to keep a closer eye on that patient.”
The machine learning approach cannot isolate the single-most predictive factor or combination of factors that lead to negative side effects, but can provide patients and their clinicians with a better understanding of what to expect during the course of treatment. In addition to predicting the likelihood of side effects, machine learning models could potentially predict which treatment plans would be most effective for different types of patients and allow for more personalized approaches to radiation oncology.
“Machine learning can make doctors more efficient and treatment safer by reducing the risk of error,” added Dr. Reddy. “It has the potential for influencing all aspects of radiation oncology today – anything where a computer can look at data and recognize a pattern.”
Related Links:
University of Texas MD Anderson Cancer Center
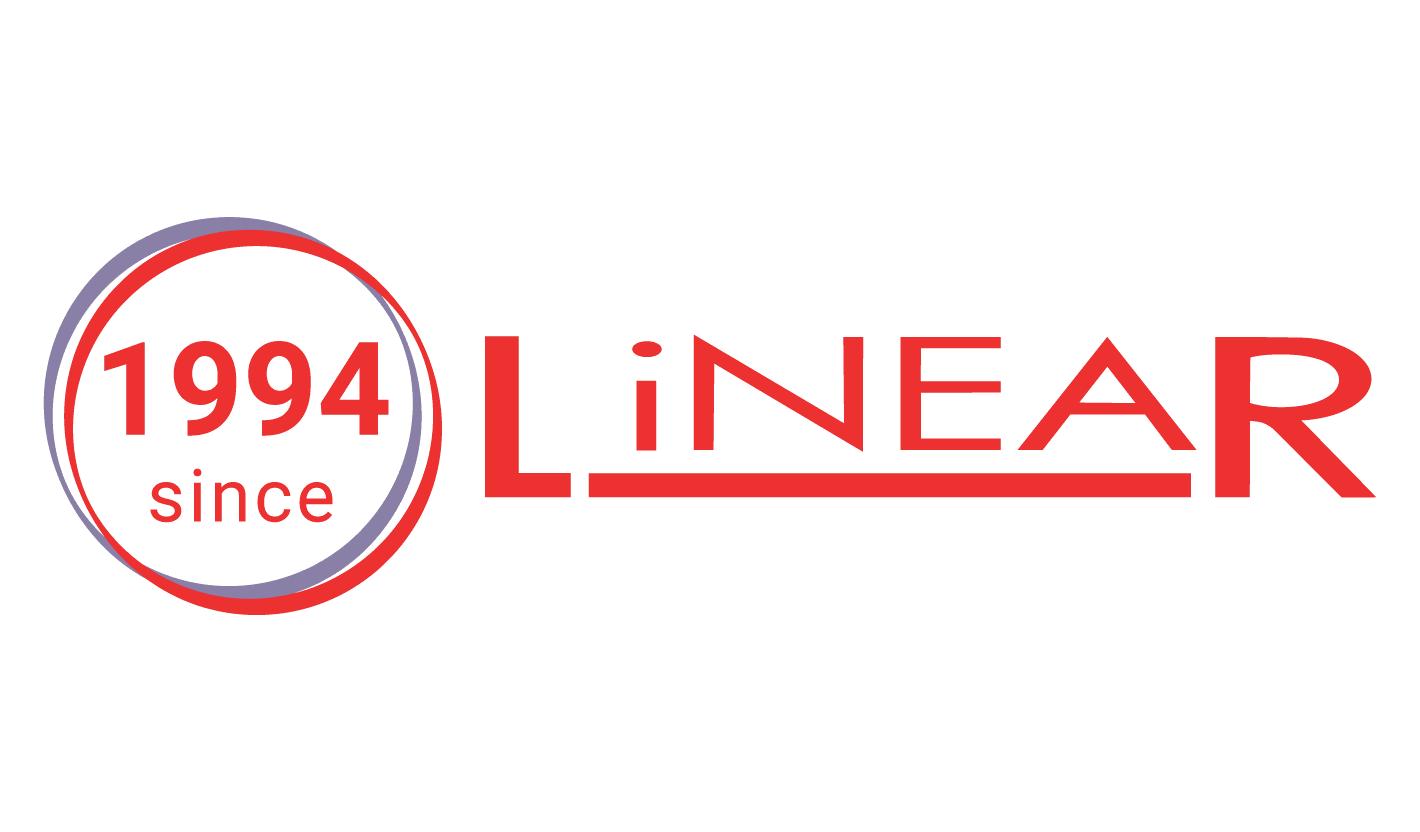
SARS‑CoV‑2/Flu A/Flu B/RSV Sample-To-Answer Test
SARS‑CoV‑2/Flu A/Flu B/RSV Cartridge (CE-IVD)
Channels
Artificial Intelligence
view channel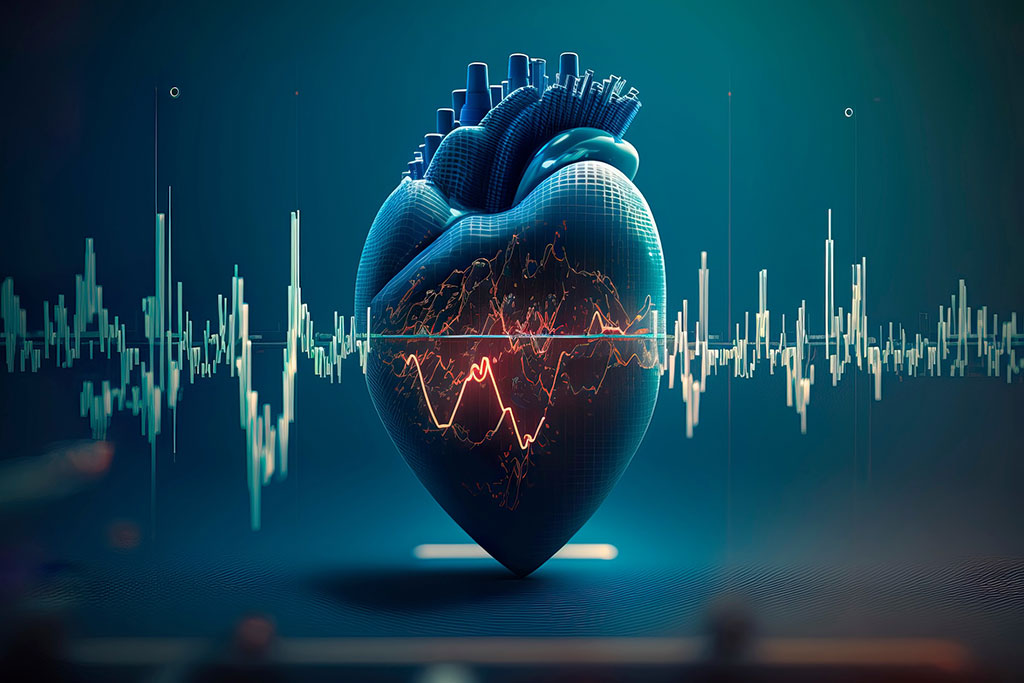
AI-Powered Algorithm to Revolutionize Detection of Atrial Fibrillation
Atrial fibrillation (AFib), a condition characterized by an irregular and often rapid heart rate, is linked to increased risks of stroke and heart failure. This is because the irregular heartbeat in AFib... Read more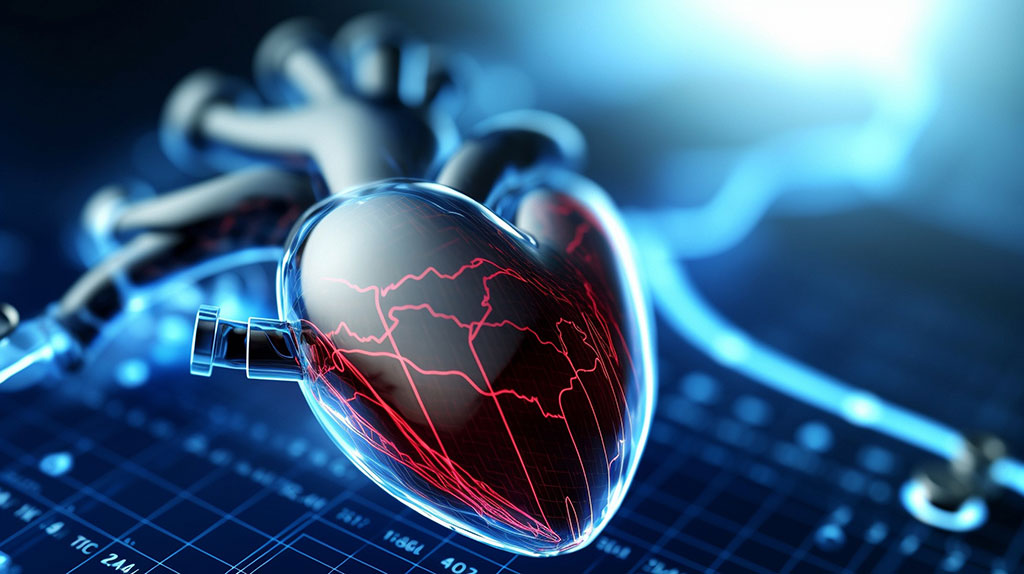
AI Diagnostic Tool Accurately Detects Valvular Disorders Often Missed by Doctors
Doctors generally use stethoscopes to listen for the characteristic lub-dub sounds made by heart valves opening and closing. They also listen for less prominent sounds that indicate problems with these valves.... Read moreCritical Care
view channel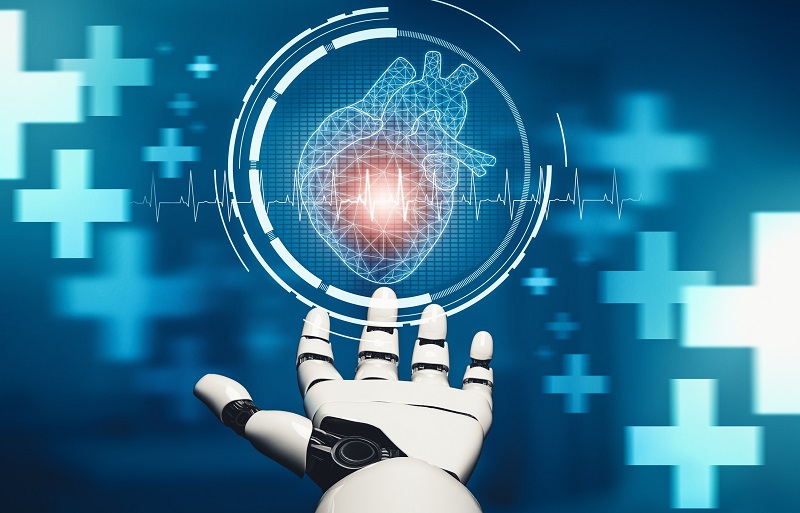
Powerful AI Risk Assessment Tool Predicts Outcomes in Heart Failure Patients
Heart failure is a serious condition where the heart cannot pump sufficient blood to meet the body's needs, leading to symptoms like fatigue, weakness, and swelling in the legs and feet, and it can ultimately... Read more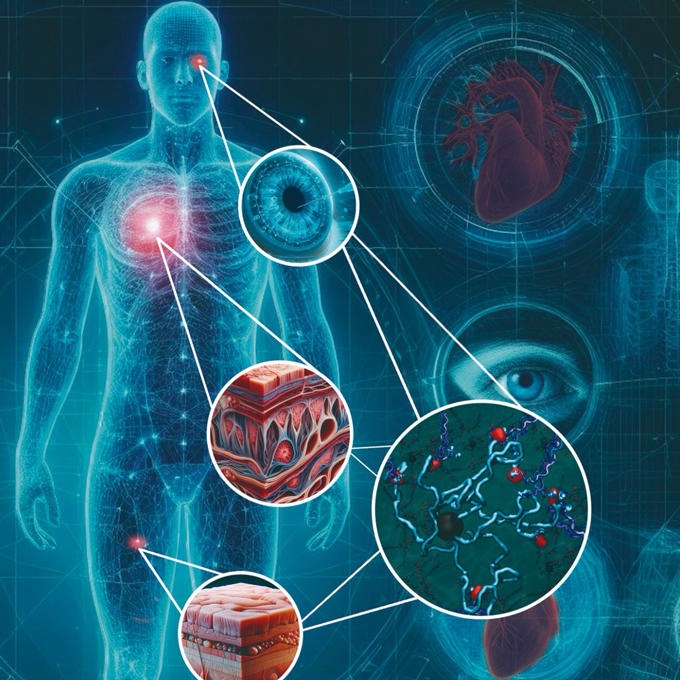
Peptide-Based Hydrogels Repair Damaged Organs and Tissues On-The-Spot
Scientists have ingeniously combined biomedical expertise with nature-inspired engineering to develop a jelly-like material that holds significant promise for immediate repairs to a wide variety of damaged... Read more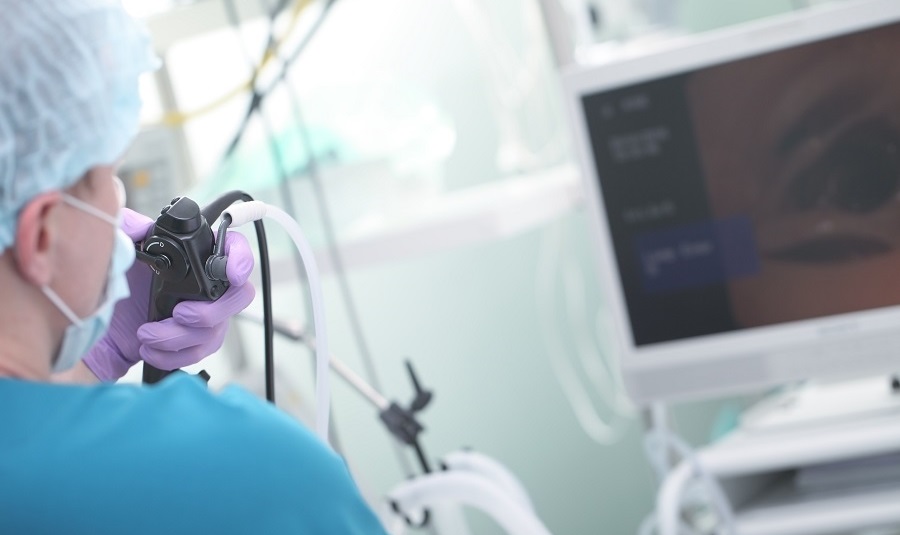
One-Hour Endoscopic Procedure Could Eliminate Need for Insulin for Type 2 Diabetes
Over 37 million Americans are diagnosed with diabetes, and more than 90% of these cases are Type 2 diabetes. This form of diabetes is most commonly seen in individuals over 45, though an increasing number... Read moreSurgical Techniques
view channel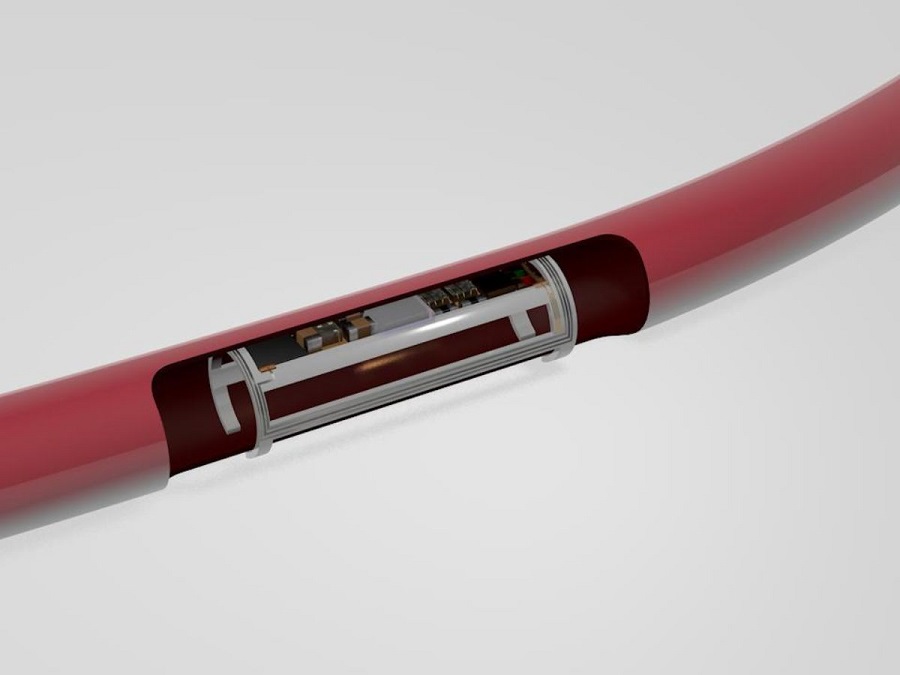
Miniaturized Implantable Multi-Sensors Device to Monitor Vessels Health
Researchers have embarked on a project to develop a multi-sensing device that can be implanted into blood vessels like peripheral veins or arteries to monitor a range of bodily parameters and overall health status.... Read more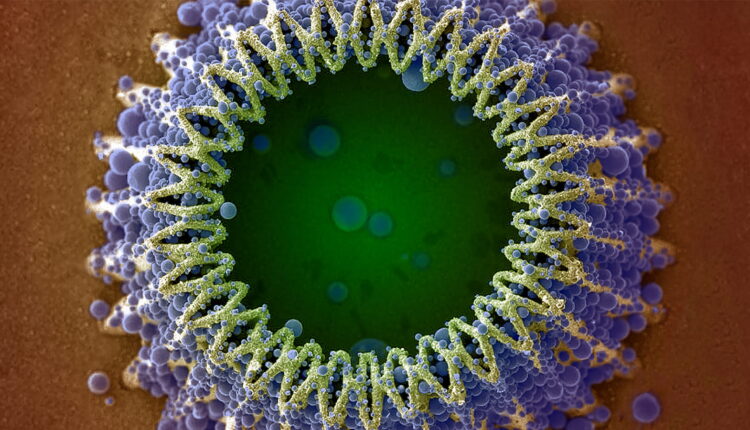
Tiny Robots Made Out Of Carbon Could Conduct Colonoscopy, Pelvic Exam or Blood Test
Researchers at the University of Alberta (Edmonton, AB, Canada) are developing cutting-edge robots so tiny that they are invisible to the naked eye but are capable of traveling through the human body to... Read more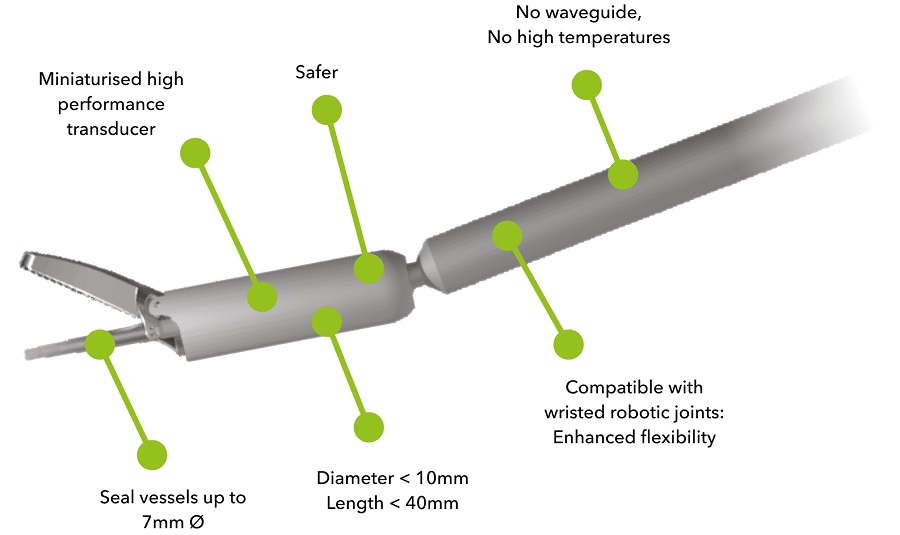
Miniaturized Ultrasonic Scalpel Enables Faster and Safer Robotic-Assisted Surgery
Robot-assisted surgery (RAS) has gained significant popularity in recent years and is now extensively used across various surgical fields such as urology, gynecology, and cardiology. These surgeries, performed... Read morePatient Care
view channel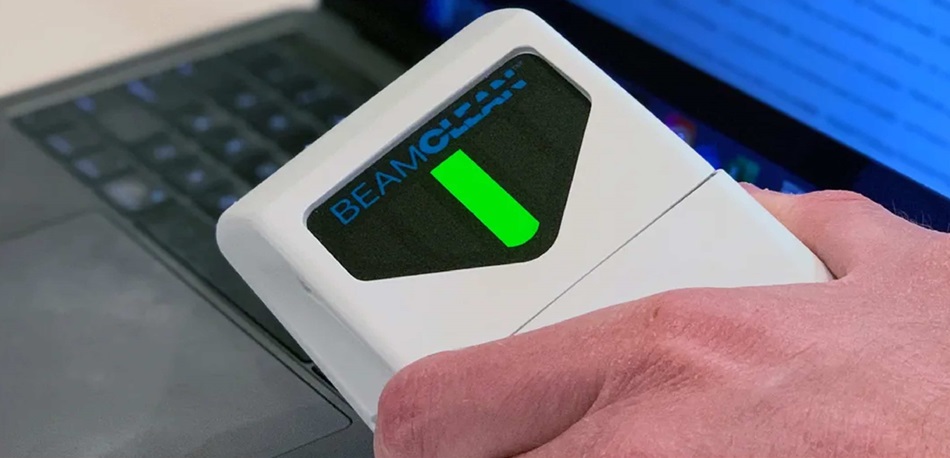
First-Of-Its-Kind Portable Germicidal Light Technology Disinfects High-Touch Clinical Surfaces in Seconds
Reducing healthcare-acquired infections (HAIs) remains a pressing issue within global healthcare systems. In the United States alone, 1.7 million patients contract HAIs annually, leading to approximately... Read more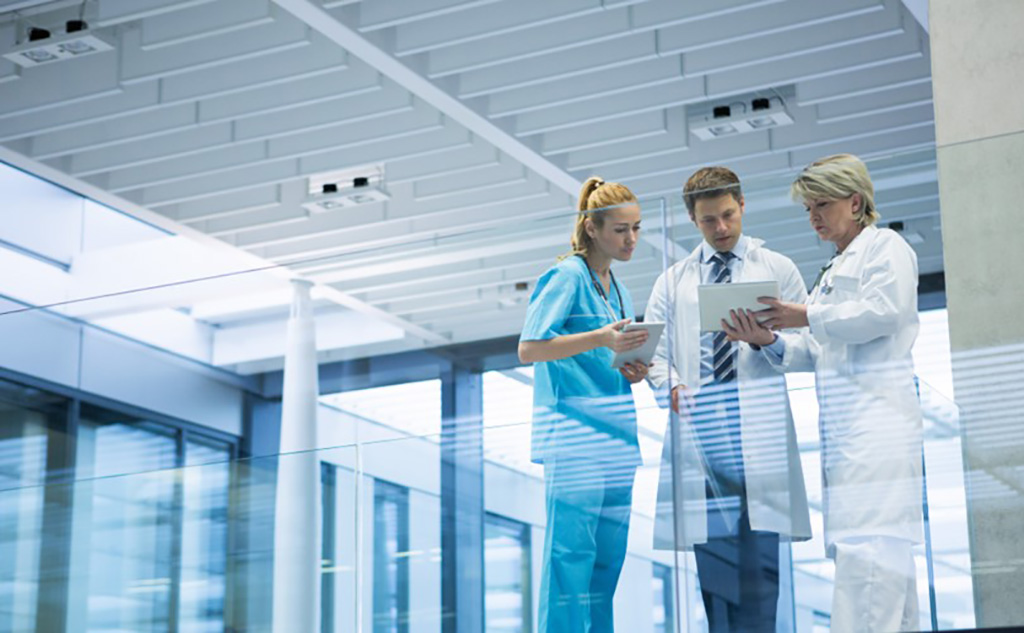
Surgical Capacity Optimization Solution Helps Hospitals Boost OR Utilization
An innovative solution has the capability to transform surgical capacity utilization by targeting the root cause of surgical block time inefficiencies. Fujitsu Limited’s (Tokyo, Japan) Surgical Capacity... Read more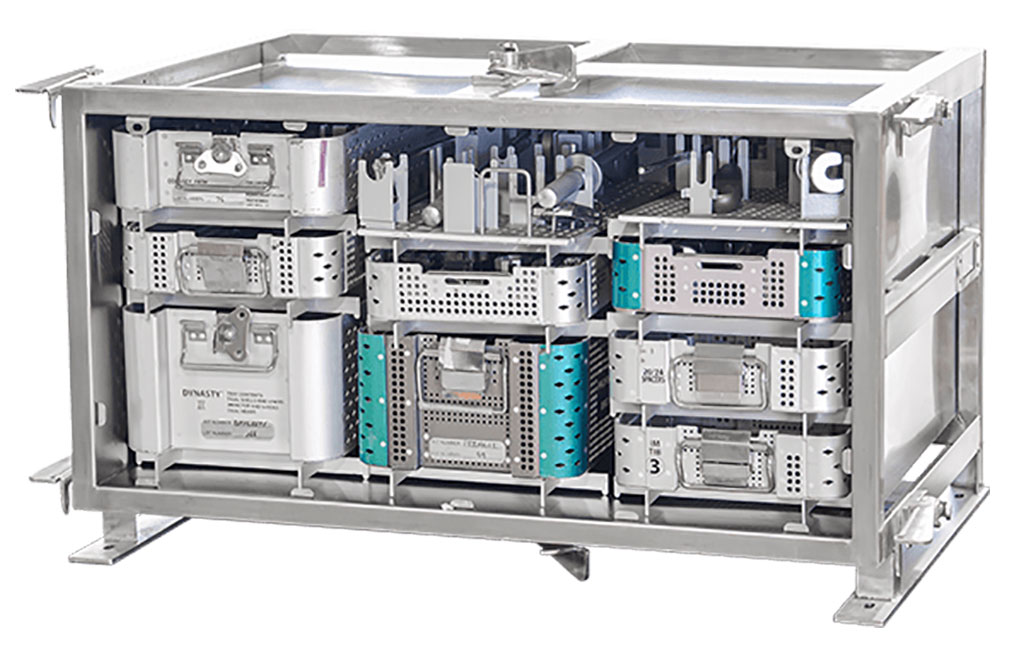
Game-Changing Innovation in Surgical Instrument Sterilization Significantly Improves OR Throughput
A groundbreaking innovation enables hospitals to significantly improve instrument processing time and throughput in operating rooms (ORs) and sterile processing departments. Turbett Surgical, Inc.... Read moreHealth IT
view channel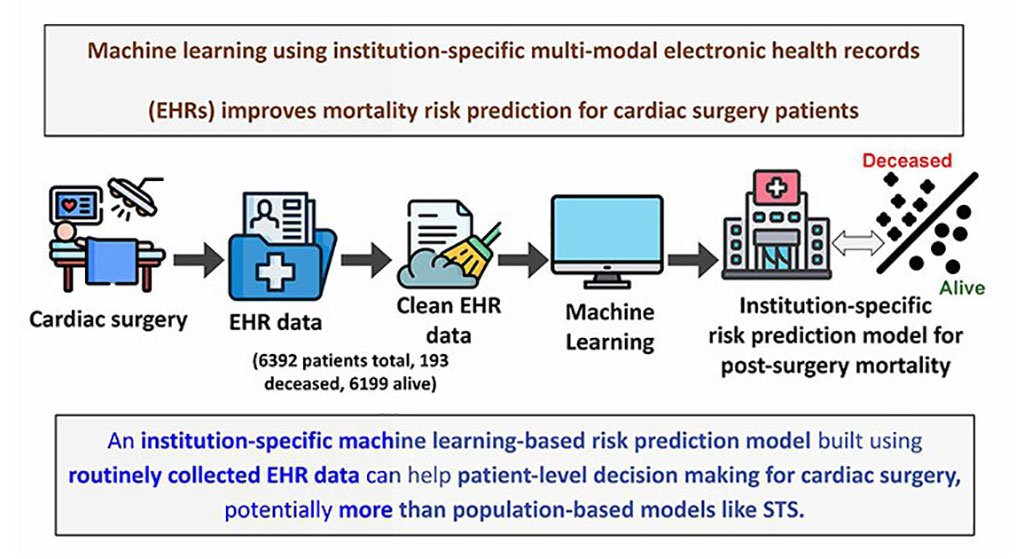
Machine Learning Model Improves Mortality Risk Prediction for Cardiac Surgery Patients
Machine learning algorithms have been deployed to create predictive models in various medical fields, with some demonstrating improved outcomes compared to their standard-of-care counterparts.... Read more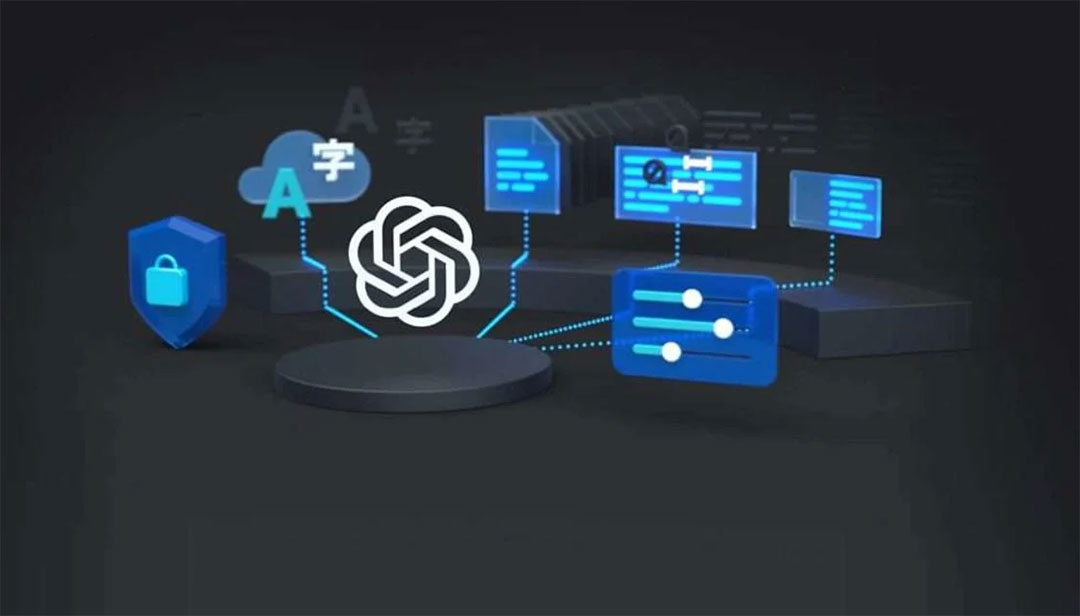
Strategic Collaboration to Develop and Integrate Generative AI into Healthcare
Top industry experts have underscored the immediate requirement for healthcare systems and hospitals to respond to severe cost and margin pressures. Close to half of U.S. hospitals ended 2022 in the red... Read more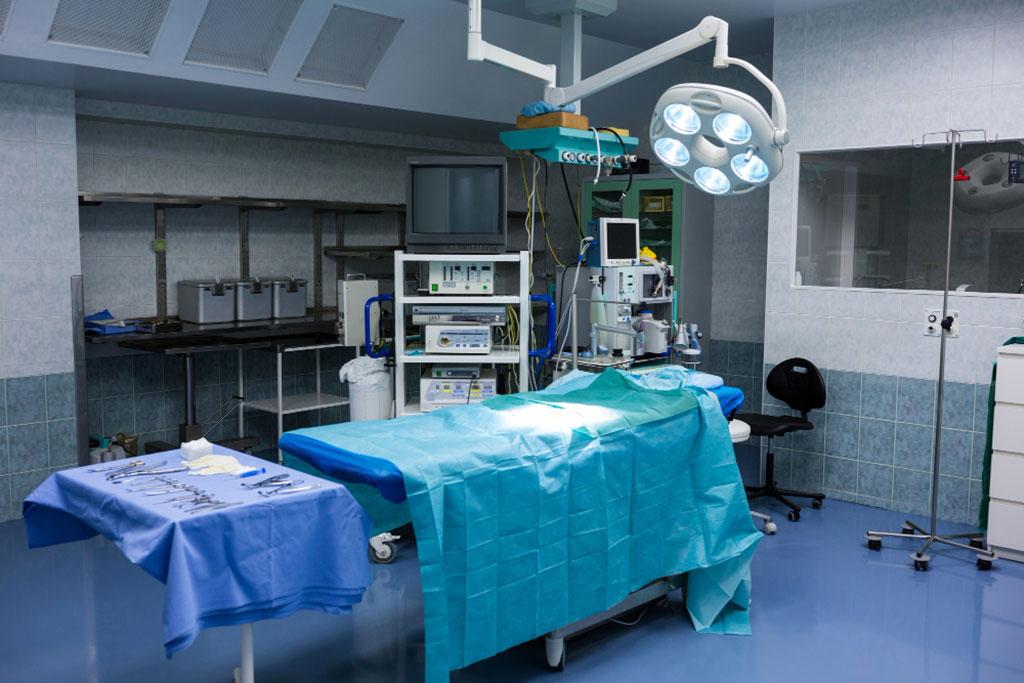
AI-Enabled Operating Rooms Solution Helps Hospitals Maximize Utilization and Unlock Capacity
For healthcare organizations, optimizing operating room (OR) utilization during prime time hours is a complex challenge. Surgeons and clinics face difficulties in finding available slots for booking cases,... Read more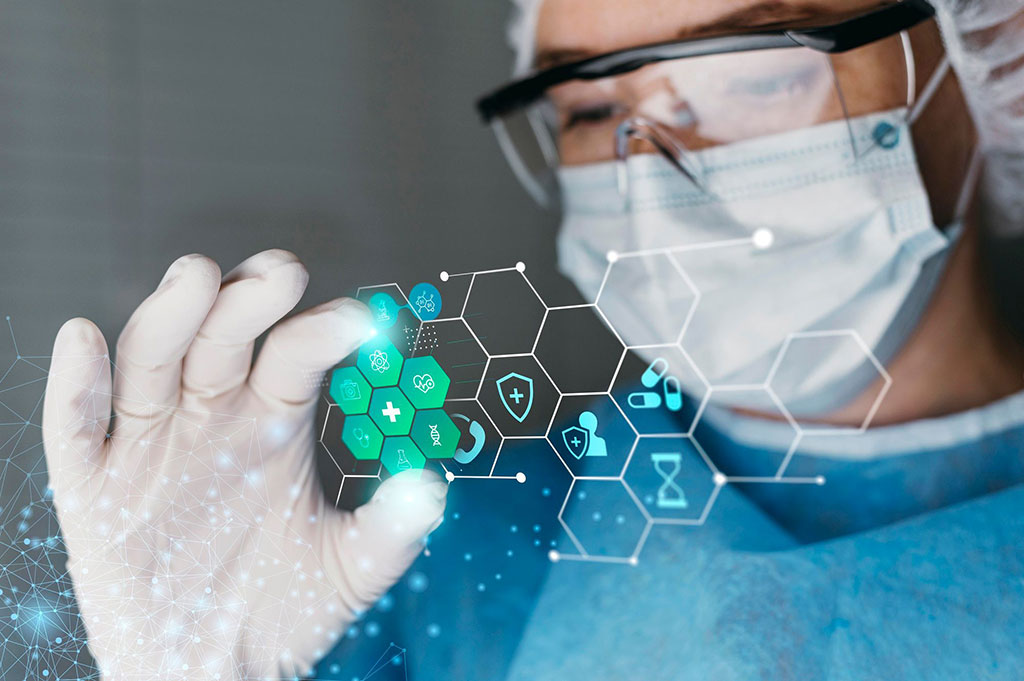
AI Predicts Pancreatic Cancer Three Years before Diagnosis from Patients’ Medical Records
Screening for common cancers like breast, cervix, and prostate cancer relies on relatively simple and highly effective techniques, such as mammograms, Pap smears, and blood tests. These methods have revolutionized... Read morePoint of Care
view channel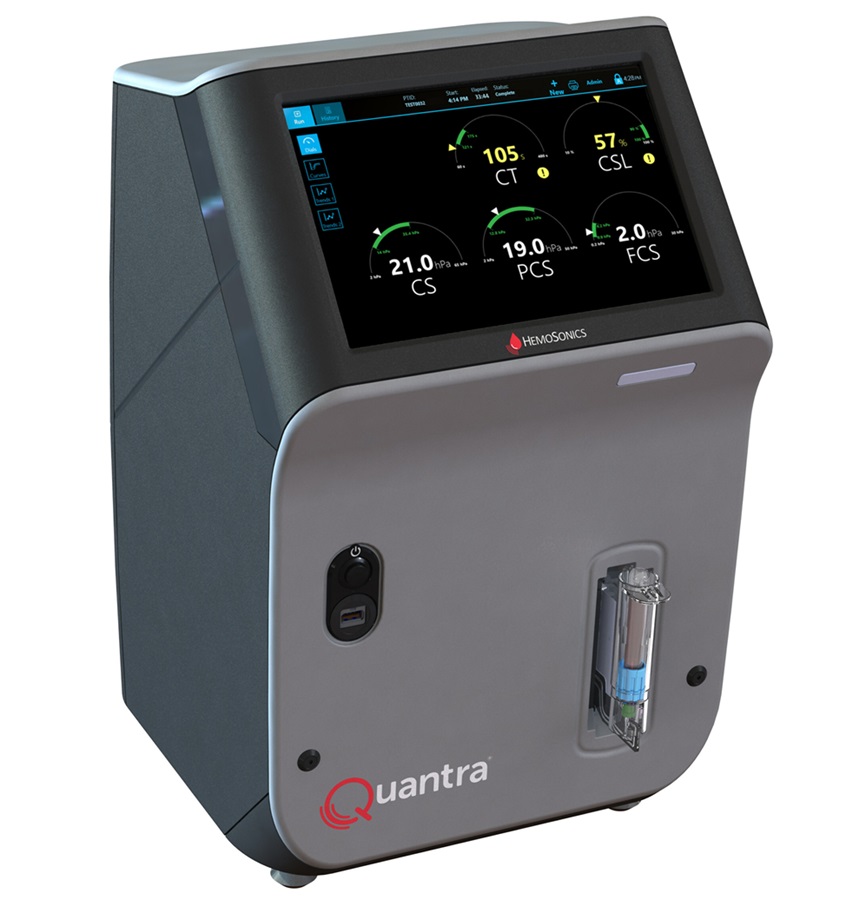
Critical Bleeding Management System to Help Hospitals Further Standardize Viscoelastic Testing
Surgical procedures are often accompanied by significant blood loss and the subsequent high likelihood of the need for allogeneic blood transfusions. These transfusions, while critical, are linked to various... Read more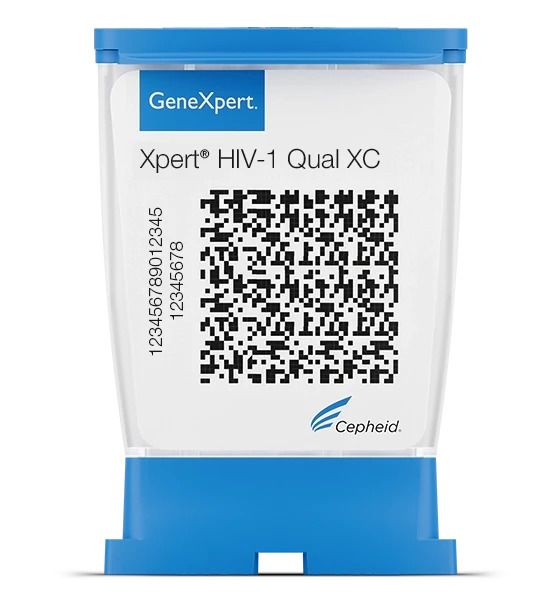
Point of Care HIV Test Enables Early Infection Diagnosis for Infants
Early diagnosis and initiation of treatment are crucial for the survival of infants infected with HIV (human immunodeficiency virus). Without treatment, approximately 50% of infants who acquire HIV during... Read more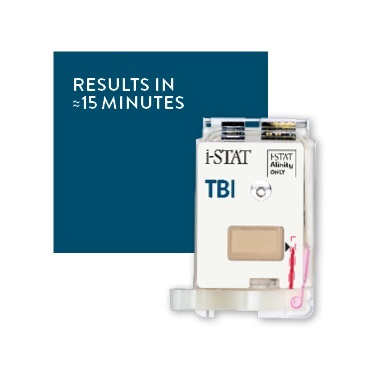
Whole Blood Rapid Test Aids Assessment of Concussion at Patient's Bedside
In the United States annually, approximately five million individuals seek emergency department care for traumatic brain injuries (TBIs), yet over half of those suspecting a concussion may never get it checked.... Read more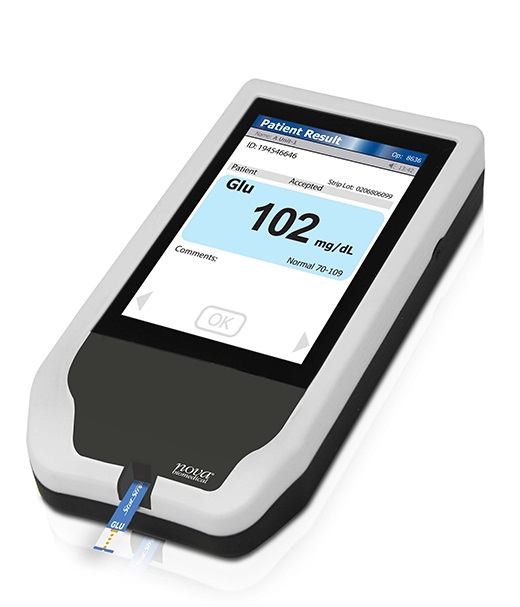
New Generation Glucose Hospital Meter System Ensures Accurate, Interference-Free and Safe Use
A new generation glucose hospital meter system now comes with several features that make hospital glucose testing easier and more secure while continuing to offer accuracy, freedom from interference, and... Read moreBusiness
view channel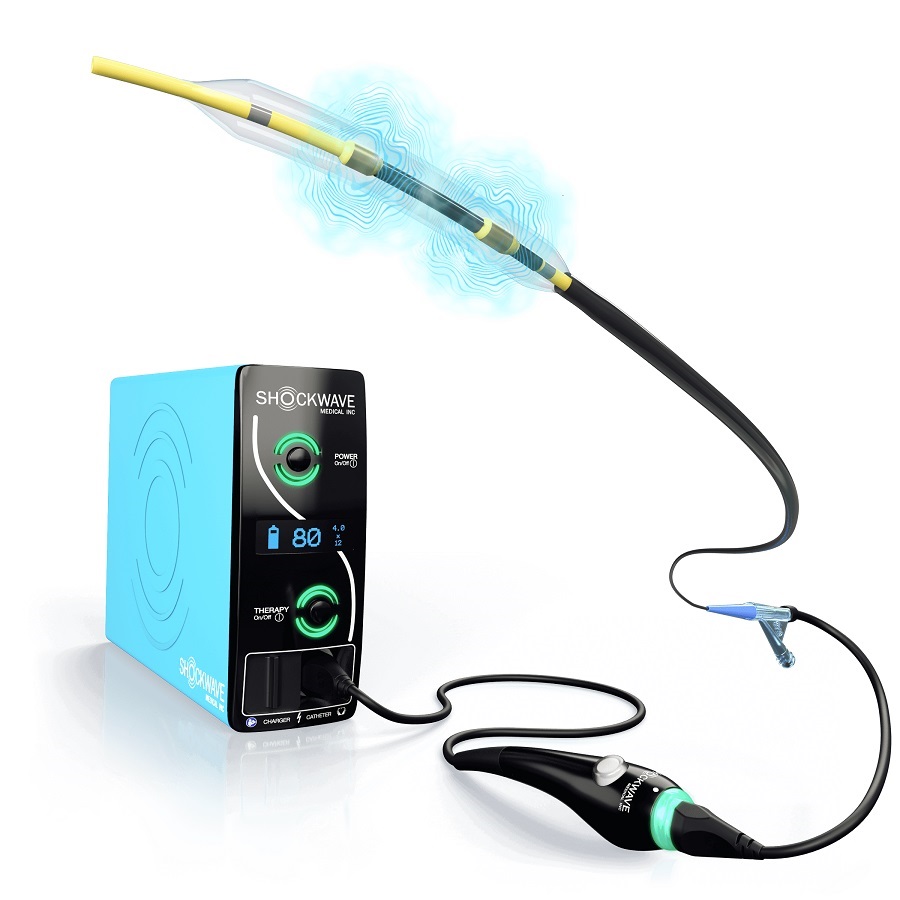
Johnson & Johnson Acquires Cardiovascular Medical Device Company Shockwave Medical
Johnson & Johnson (New Brunswick, N.J., USA) and Shockwave Medical (Santa Clara, CA, USA) have entered into a definitive agreement under which Johnson & Johnson will acquire all of Shockwave’s... Read more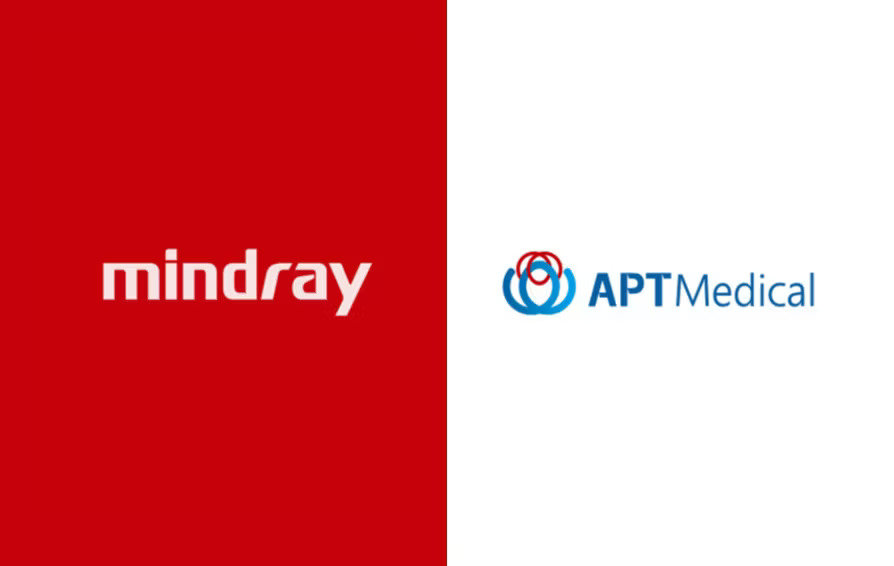