Automated CT Image-Based AI Model Predicts Disease Progression and Mortality in COVID-19 Patients
By HospiMedica International staff writers Posted on 03 May 2021 |
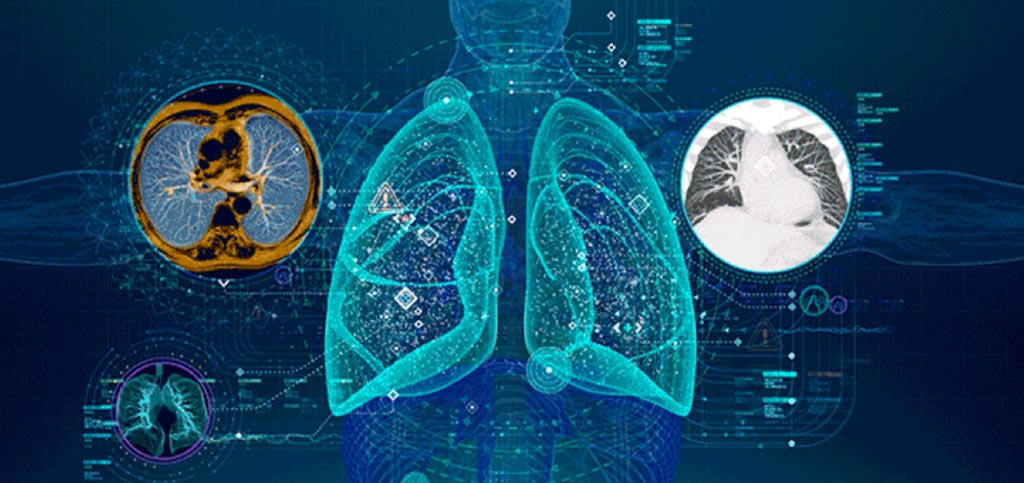
Illustration
Researchers have developed an automated image-based survival prediction model based on deep learning of chest computed tomography (CT) images for fast and accurate clinical assessment of COVID-19 progression and mortality.
In an evaluation of the model, called U-survival, developed by researchers at Brigham and Women’s Hospital (Boston, MA, USA), the results indicated that it can be used to provide automated and objective prognostic predictions for the management of COVID-19 patients.
Chest imaging can help clinicians to decide whether to admit or discharge patients with mild COVID-19 symptoms, whether to admit patients with moderate-to-severe COVID-19 symptoms to a regular ward or an intensive care unit (ICU), and to provide information about therapeutic management of hospitalized patients with moderate-to-severe COVID-19 symptoms. CT is the most sensitive chest imaging method for COVID-19.
The U-survival model integrates the image information extracted by deep learning (U-Net) directly into a Cox proportional hazards model with an elastic-net penalty (elastic-net Cox model) for performing the prognostic prediction of patients with COVID-19. After training the U-Net to perform semantic segmentation of the lung tissue patterns of chest CT images, the researchers subjected the bottleneck section of the U-Net to an elastic-net Cox model that automatically selects a sparse subset of features to build an optimal survival model for the input data. Their approach was inspired by radiomics in the sense that the researchers used an elastic-net penalty to construct a deep radiomic signature for survival analysis from a large number of features that are extracted from the images internally by the U-Net.
The researchers showed that deep learning of chest CT images can be used as an integral part of an automated image-based survival prediction model based on traditional survival analysis methodology. This made it possible to obtain complete survival information that was not available with previously proposed prediction models. In their evaluation of 383 COVID-19 positive patients from two hospitals, the U-survival model significantly outperformed existing laboratory tests and image-based visual and quantitative predictors in the prediction of the disease progression and mortality of COVID-19 patients. The results indicate that the U-survival model can be used to provide automated and objective prognostic predictions for the management of COVID-19 patients.
Related Links:
Brigham and Women’s Hospital
In an evaluation of the model, called U-survival, developed by researchers at Brigham and Women’s Hospital (Boston, MA, USA), the results indicated that it can be used to provide automated and objective prognostic predictions for the management of COVID-19 patients.
Chest imaging can help clinicians to decide whether to admit or discharge patients with mild COVID-19 symptoms, whether to admit patients with moderate-to-severe COVID-19 symptoms to a regular ward or an intensive care unit (ICU), and to provide information about therapeutic management of hospitalized patients with moderate-to-severe COVID-19 symptoms. CT is the most sensitive chest imaging method for COVID-19.
The U-survival model integrates the image information extracted by deep learning (U-Net) directly into a Cox proportional hazards model with an elastic-net penalty (elastic-net Cox model) for performing the prognostic prediction of patients with COVID-19. After training the U-Net to perform semantic segmentation of the lung tissue patterns of chest CT images, the researchers subjected the bottleneck section of the U-Net to an elastic-net Cox model that automatically selects a sparse subset of features to build an optimal survival model for the input data. Their approach was inspired by radiomics in the sense that the researchers used an elastic-net penalty to construct a deep radiomic signature for survival analysis from a large number of features that are extracted from the images internally by the U-Net.
The researchers showed that deep learning of chest CT images can be used as an integral part of an automated image-based survival prediction model based on traditional survival analysis methodology. This made it possible to obtain complete survival information that was not available with previously proposed prediction models. In their evaluation of 383 COVID-19 positive patients from two hospitals, the U-survival model significantly outperformed existing laboratory tests and image-based visual and quantitative predictors in the prediction of the disease progression and mortality of COVID-19 patients. The results indicate that the U-survival model can be used to provide automated and objective prognostic predictions for the management of COVID-19 patients.
Related Links:
Brigham and Women’s Hospital
Latest COVID-19 News
- Low-Cost System Detects SARS-CoV-2 Virus in Hospital Air Using High-Tech Bubbles
- World's First Inhalable COVID-19 Vaccine Approved in China
- COVID-19 Vaccine Patch Fights SARS-CoV-2 Variants Better than Needles
- Blood Viscosity Testing Can Predict Risk of Death in Hospitalized COVID-19 Patients
- ‘Covid Computer’ Uses AI to Detect COVID-19 from Chest CT Scans
- MRI Lung-Imaging Technique Shows Cause of Long-COVID Symptoms
- Chest CT Scans of COVID-19 Patients Could Help Distinguish Between SARS-CoV-2 Variants
- Specialized MRI Detects Lung Abnormalities in Non-Hospitalized Long COVID Patients
- AI Algorithm Identifies Hospitalized Patients at Highest Risk of Dying From COVID-19
- Sweat Sensor Detects Key Biomarkers That Provide Early Warning of COVID-19 and Flu
- Study Assesses Impact of COVID-19 on Ventilation/Perfusion Scintigraphy
- CT Imaging Study Finds Vaccination Reduces Risk of COVID-19 Associated Pulmonary Embolism
- Third Day in Hospital a ‘Tipping Point’ in Severity of COVID-19 Pneumonia
- Longer Interval Between COVID-19 Vaccines Generates Up to Nine Times as Many Antibodies
- AI Model for Monitoring COVID-19 Predicts Mortality Within First 30 Days of Admission
- AI Predicts COVID Prognosis at Near-Expert Level Based Off CT Scans
Channels
Critical Care
view channel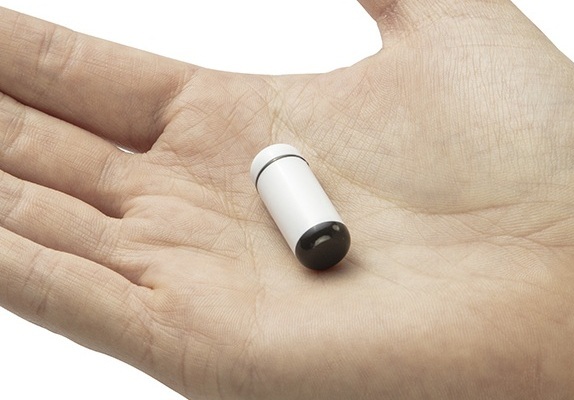
Ingestible Smart Capsule for Chemical Sensing in the Gut Moves Closer to Market
Intestinal gases are associated with several health conditions, including colon cancer, irritable bowel syndrome, and inflammatory bowel disease, and they have the potential to serve as crucial biomarkers... Read more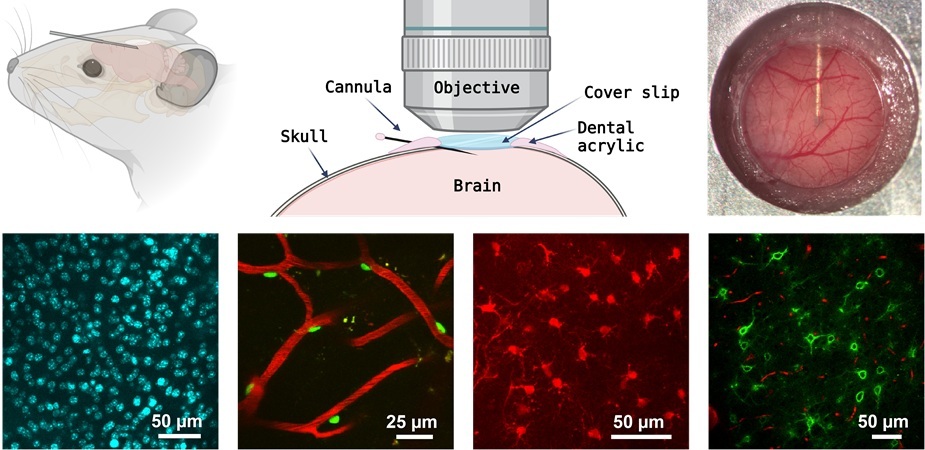
Novel Cannula Delivery System Enables Targeted Delivery of Imaging Agents and Drugs
Multiphoton microscopy has become an invaluable tool in neuroscience, allowing researchers to observe brain activity in real time with high-resolution imaging. A crucial aspect of many multiphoton microscopy... Read more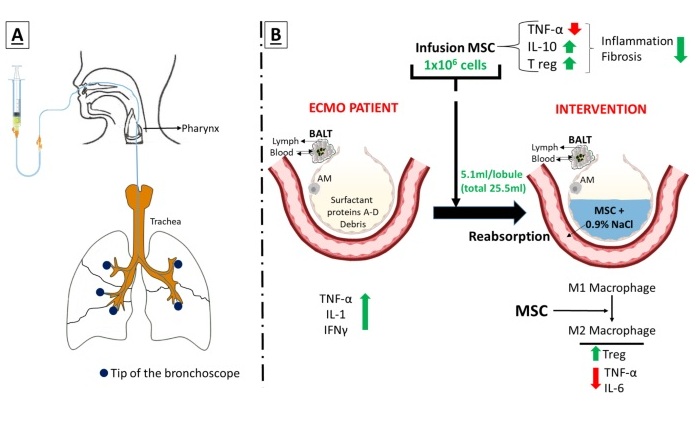
Novel Intrabronchial Method Delivers Cell Therapies in Critically Ill Patients on External Lung Support
Until now, administering cell therapies to patients on extracorporeal membrane oxygenation (ECMO)—a life-support system typically used for severe lung failure—has been nearly impossible.... Read moreSurgical Techniques
view channel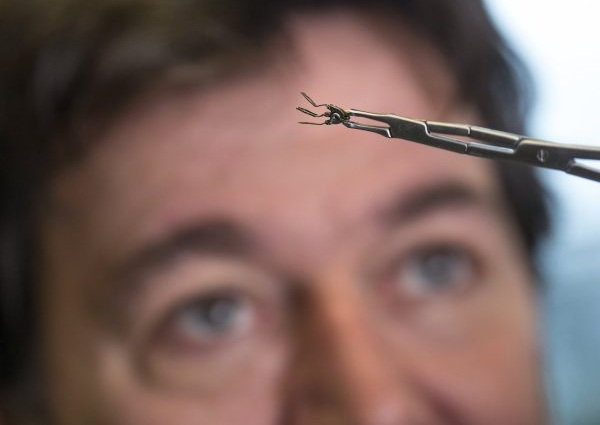
Pioneering Sutureless Coronary Bypass Technology to Eliminate Open-Chest Procedures
In patients with coronary artery disease, certain blood vessels may be narrowed or blocked, requiring a stent or a bypass (also known as diversion) to restore blood flow to the heart. Bypass surgeries... Read more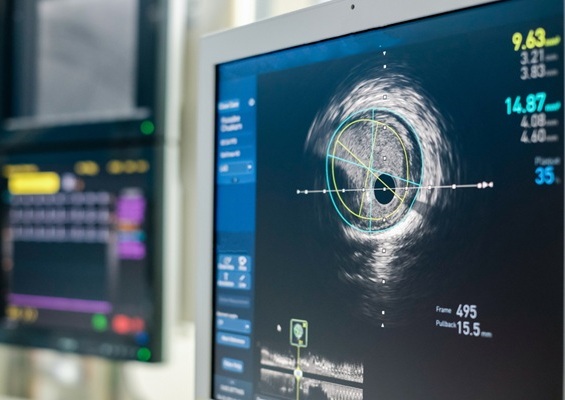
Intravascular Imaging for Guiding Stent Implantation Ensures Safer Stenting Procedures
Patients diagnosed with coronary artery disease, which is caused by plaque accumulation within the arteries leading to chest pain, shortness of breath, and potential heart attacks, frequently undergo percutaneous... Read more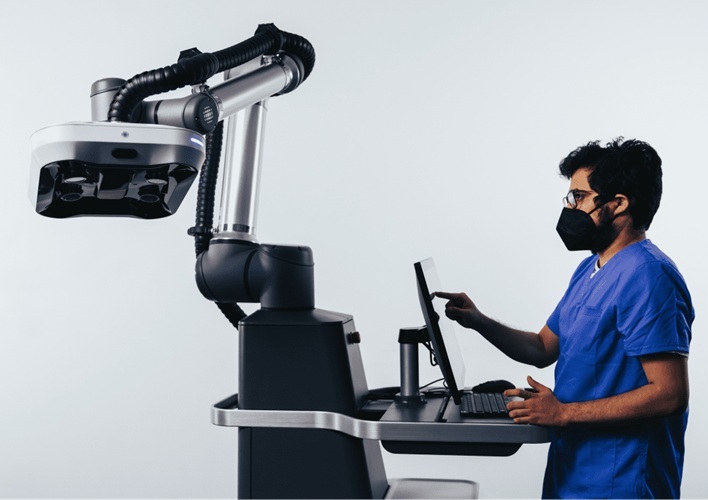
World's First AI Surgical Guidance Platform Allows Surgeons to Measure Success in Real-Time
Surgeons have always faced challenges in measuring their progress toward surgical goals during procedures. Traditionally, obtaining measurements required stepping out of the sterile environment to perform... Read morePatient Care
view channel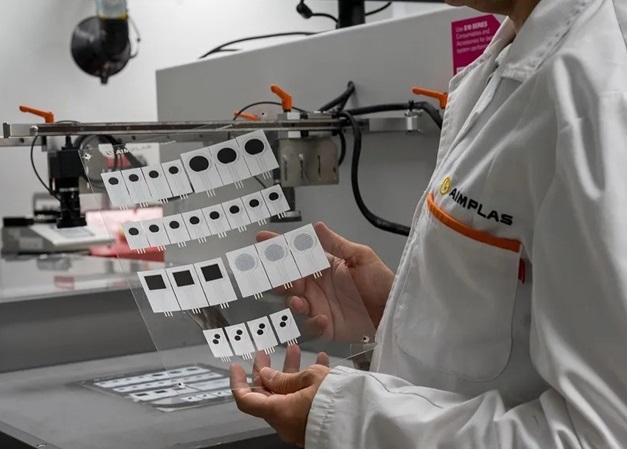
Portable Biosensor Platform to Reduce Hospital-Acquired Infections
Approximately 4 million patients in the European Union acquire healthcare-associated infections (HAIs) or nosocomial infections each year, with around 37,000 deaths directly resulting from these infections,... Read more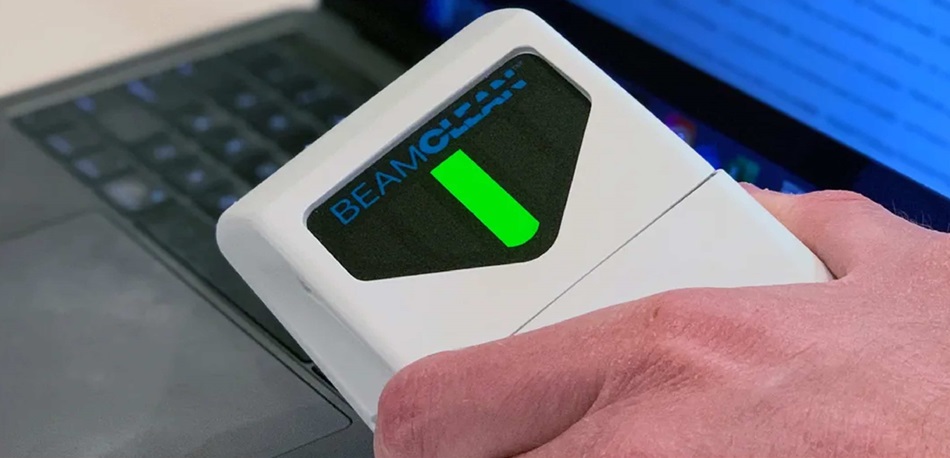
First-Of-Its-Kind Portable Germicidal Light Technology Disinfects High-Touch Clinical Surfaces in Seconds
Reducing healthcare-acquired infections (HAIs) remains a pressing issue within global healthcare systems. In the United States alone, 1.7 million patients contract HAIs annually, leading to approximately... Read more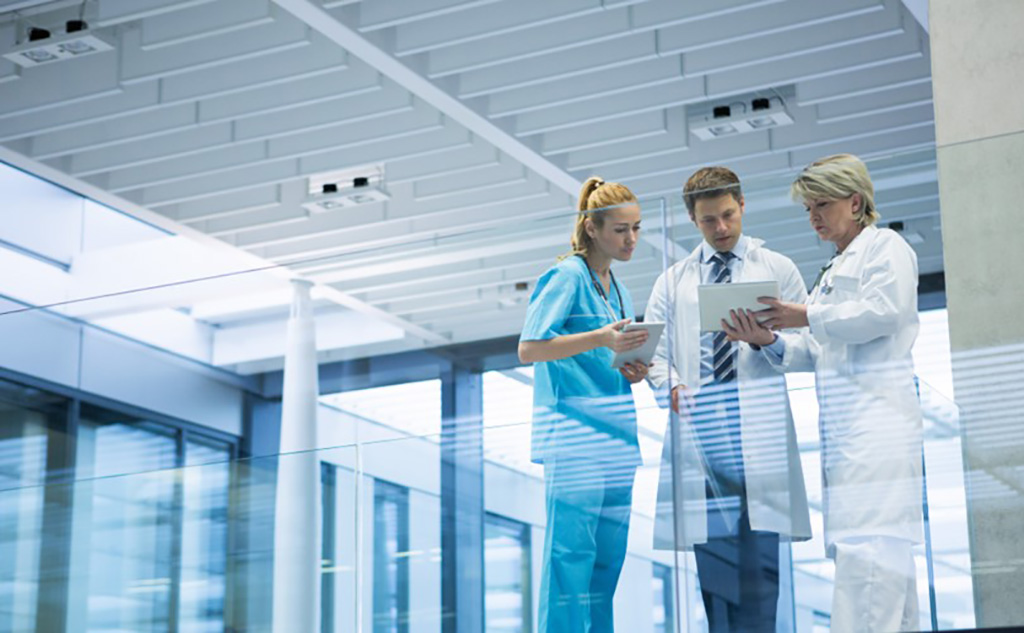
Surgical Capacity Optimization Solution Helps Hospitals Boost OR Utilization
An innovative solution has the capability to transform surgical capacity utilization by targeting the root cause of surgical block time inefficiencies. Fujitsu Limited’s (Tokyo, Japan) Surgical Capacity... Read more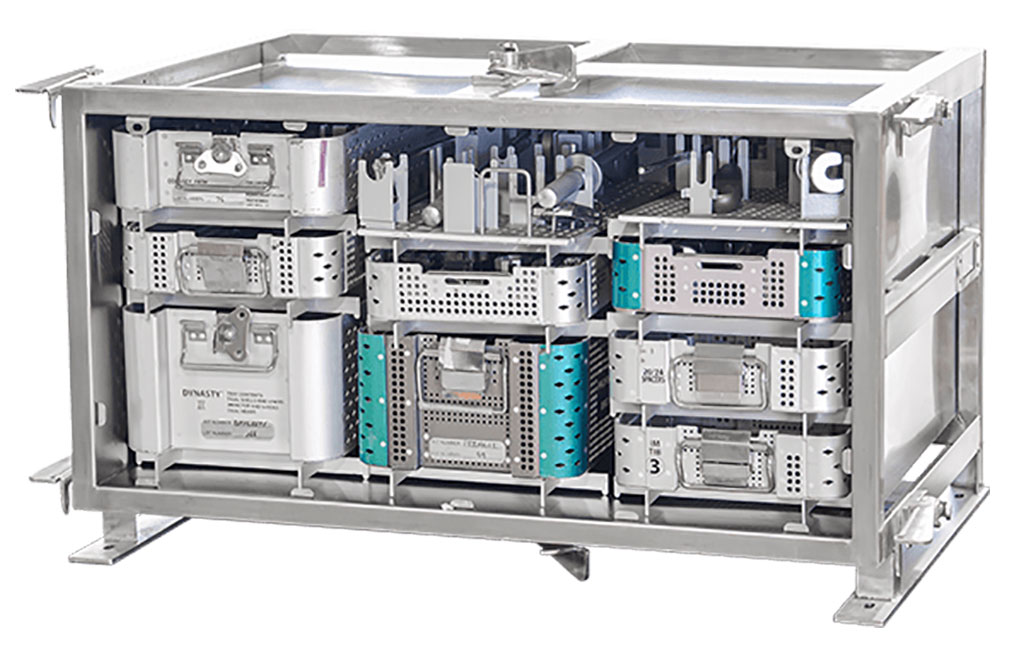
Game-Changing Innovation in Surgical Instrument Sterilization Significantly Improves OR Throughput
A groundbreaking innovation enables hospitals to significantly improve instrument processing time and throughput in operating rooms (ORs) and sterile processing departments. Turbett Surgical, Inc.... Read moreHealth IT
view channel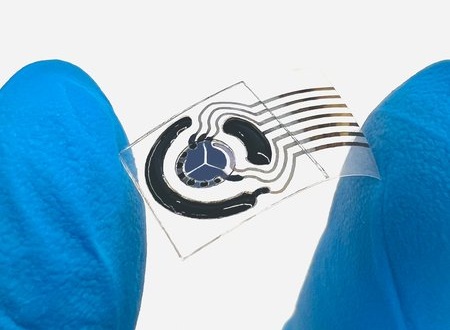
Printable Molecule-Selective Nanoparticles Enable Mass Production of Wearable Biosensors
The future of medicine is likely to focus on the personalization of healthcare—understanding exactly what an individual requires and delivering the appropriate combination of nutrients, metabolites, and... Read more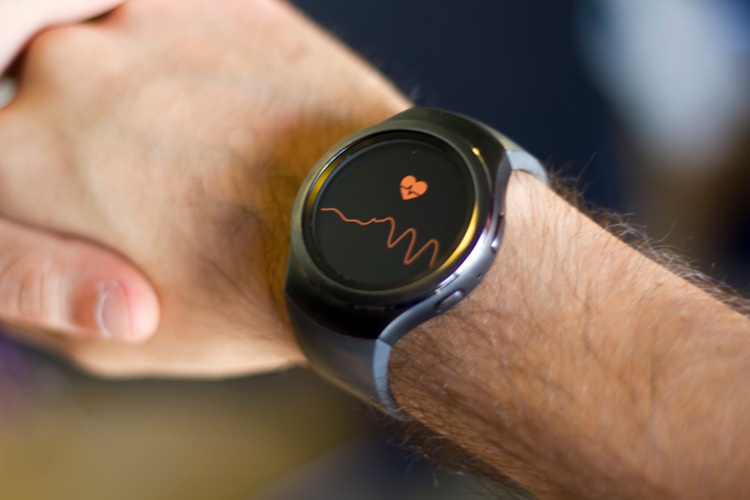
Smartwatches Could Detect Congestive Heart Failure
Diagnosing congestive heart failure (CHF) typically requires expensive and time-consuming imaging techniques like echocardiography, also known as cardiac ultrasound. Previously, detecting CHF by analyzing... Read moreBusiness
view channel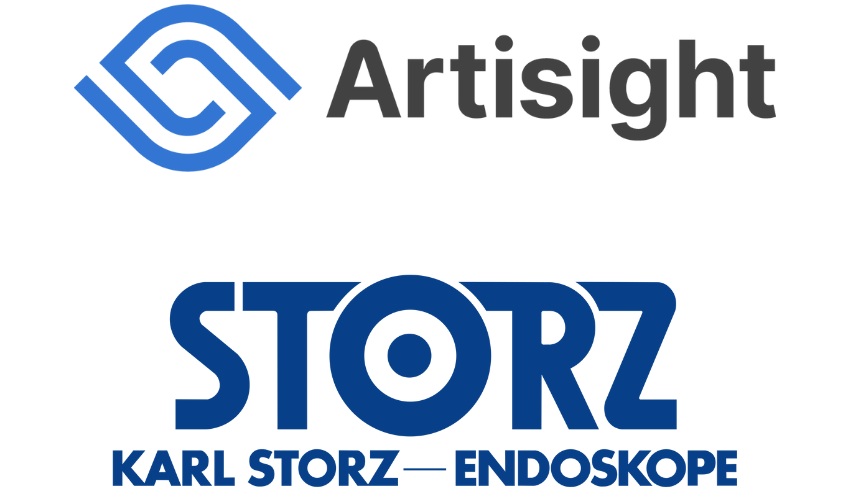
Expanded Collaboration to Transform OR Technology Through AI and Automation
The expansion of an existing collaboration between three leading companies aims to develop artificial intelligence (AI)-driven solutions for smart operating rooms with sophisticated monitoring and automation.... Read more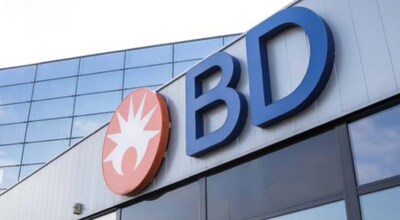