Automated CT Image-Based AI Model Predicts Disease Progression and Mortality in COVID-19 Patients
By HospiMedica International staff writers Posted on 03 May 2021 |
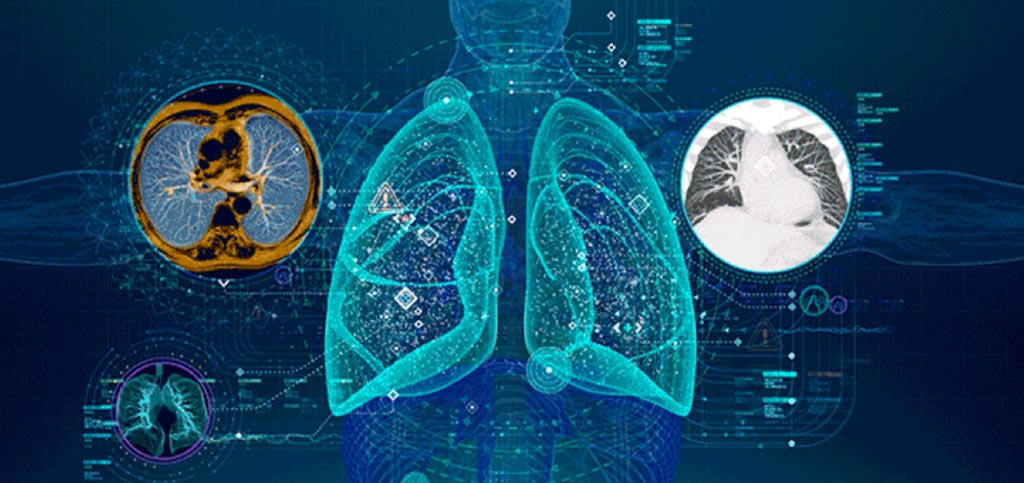
Illustration
Researchers have developed an automated image-based survival prediction model based on deep learning of chest computed tomography (CT) images for fast and accurate clinical assessment of COVID-19 progression and mortality.
In an evaluation of the model, called U-survival, developed by researchers at Brigham and Women’s Hospital (Boston, MA, USA), the results indicated that it can be used to provide automated and objective prognostic predictions for the management of COVID-19 patients.
Chest imaging can help clinicians to decide whether to admit or discharge patients with mild COVID-19 symptoms, whether to admit patients with moderate-to-severe COVID-19 symptoms to a regular ward or an intensive care unit (ICU), and to provide information about therapeutic management of hospitalized patients with moderate-to-severe COVID-19 symptoms. CT is the most sensitive chest imaging method for COVID-19.
The U-survival model integrates the image information extracted by deep learning (U-Net) directly into a Cox proportional hazards model with an elastic-net penalty (elastic-net Cox model) for performing the prognostic prediction of patients with COVID-19. After training the U-Net to perform semantic segmentation of the lung tissue patterns of chest CT images, the researchers subjected the bottleneck section of the U-Net to an elastic-net Cox model that automatically selects a sparse subset of features to build an optimal survival model for the input data. Their approach was inspired by radiomics in the sense that the researchers used an elastic-net penalty to construct a deep radiomic signature for survival analysis from a large number of features that are extracted from the images internally by the U-Net.
The researchers showed that deep learning of chest CT images can be used as an integral part of an automated image-based survival prediction model based on traditional survival analysis methodology. This made it possible to obtain complete survival information that was not available with previously proposed prediction models. In their evaluation of 383 COVID-19 positive patients from two hospitals, the U-survival model significantly outperformed existing laboratory tests and image-based visual and quantitative predictors in the prediction of the disease progression and mortality of COVID-19 patients. The results indicate that the U-survival model can be used to provide automated and objective prognostic predictions for the management of COVID-19 patients.
Related Links:
Brigham and Women’s Hospital
In an evaluation of the model, called U-survival, developed by researchers at Brigham and Women’s Hospital (Boston, MA, USA), the results indicated that it can be used to provide automated and objective prognostic predictions for the management of COVID-19 patients.
Chest imaging can help clinicians to decide whether to admit or discharge patients with mild COVID-19 symptoms, whether to admit patients with moderate-to-severe COVID-19 symptoms to a regular ward or an intensive care unit (ICU), and to provide information about therapeutic management of hospitalized patients with moderate-to-severe COVID-19 symptoms. CT is the most sensitive chest imaging method for COVID-19.
The U-survival model integrates the image information extracted by deep learning (U-Net) directly into a Cox proportional hazards model with an elastic-net penalty (elastic-net Cox model) for performing the prognostic prediction of patients with COVID-19. After training the U-Net to perform semantic segmentation of the lung tissue patterns of chest CT images, the researchers subjected the bottleneck section of the U-Net to an elastic-net Cox model that automatically selects a sparse subset of features to build an optimal survival model for the input data. Their approach was inspired by radiomics in the sense that the researchers used an elastic-net penalty to construct a deep radiomic signature for survival analysis from a large number of features that are extracted from the images internally by the U-Net.
The researchers showed that deep learning of chest CT images can be used as an integral part of an automated image-based survival prediction model based on traditional survival analysis methodology. This made it possible to obtain complete survival information that was not available with previously proposed prediction models. In their evaluation of 383 COVID-19 positive patients from two hospitals, the U-survival model significantly outperformed existing laboratory tests and image-based visual and quantitative predictors in the prediction of the disease progression and mortality of COVID-19 patients. The results indicate that the U-survival model can be used to provide automated and objective prognostic predictions for the management of COVID-19 patients.
Related Links:
Brigham and Women’s Hospital
Latest COVID-19 News
- Low-Cost System Detects SARS-CoV-2 Virus in Hospital Air Using High-Tech Bubbles
- World's First Inhalable COVID-19 Vaccine Approved in China
- COVID-19 Vaccine Patch Fights SARS-CoV-2 Variants Better than Needles
- Blood Viscosity Testing Can Predict Risk of Death in Hospitalized COVID-19 Patients
- ‘Covid Computer’ Uses AI to Detect COVID-19 from Chest CT Scans
- MRI Lung-Imaging Technique Shows Cause of Long-COVID Symptoms
- Chest CT Scans of COVID-19 Patients Could Help Distinguish Between SARS-CoV-2 Variants
- Specialized MRI Detects Lung Abnormalities in Non-Hospitalized Long COVID Patients
- AI Algorithm Identifies Hospitalized Patients at Highest Risk of Dying From COVID-19
- Sweat Sensor Detects Key Biomarkers That Provide Early Warning of COVID-19 and Flu
- Study Assesses Impact of COVID-19 on Ventilation/Perfusion Scintigraphy
- CT Imaging Study Finds Vaccination Reduces Risk of COVID-19 Associated Pulmonary Embolism
- Third Day in Hospital a ‘Tipping Point’ in Severity of COVID-19 Pneumonia
- Longer Interval Between COVID-19 Vaccines Generates Up to Nine Times as Many Antibodies
- AI Model for Monitoring COVID-19 Predicts Mortality Within First 30 Days of Admission
- AI Predicts COVID Prognosis at Near-Expert Level Based Off CT Scans
Channels
Artificial Intelligence
view channel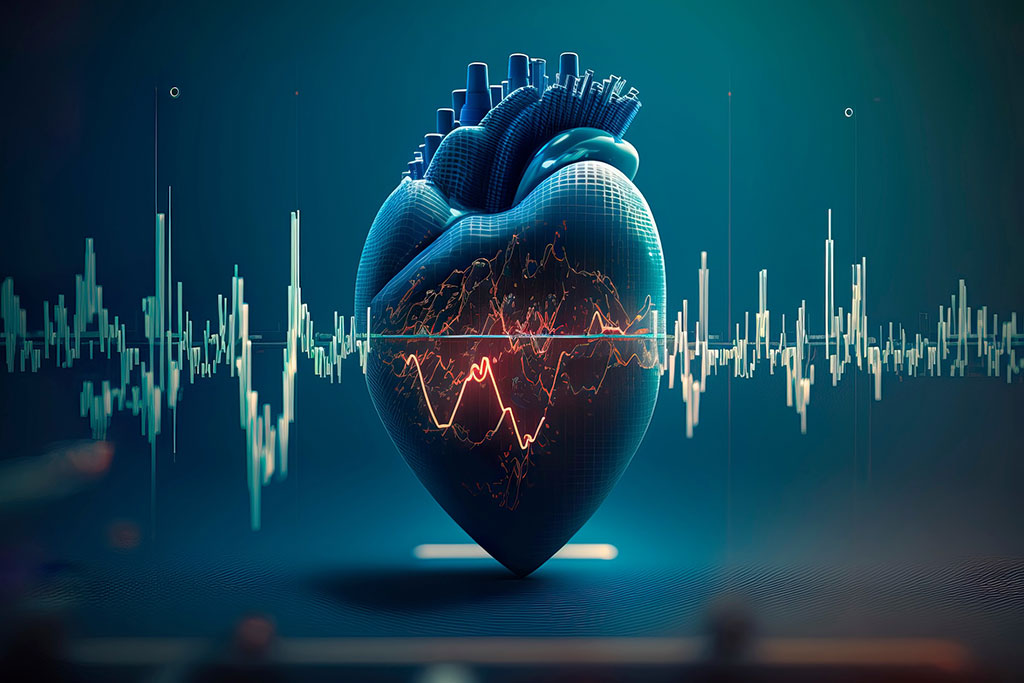
AI-Powered Algorithm to Revolutionize Detection of Atrial Fibrillation
Atrial fibrillation (AFib), a condition characterized by an irregular and often rapid heart rate, is linked to increased risks of stroke and heart failure. This is because the irregular heartbeat in AFib... Read more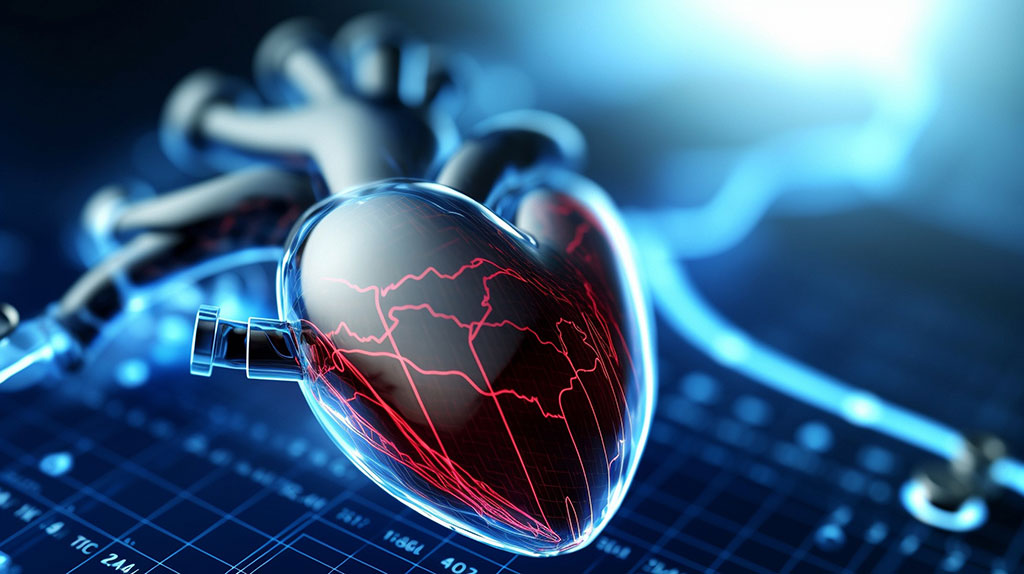
AI Diagnostic Tool Accurately Detects Valvular Disorders Often Missed by Doctors
Doctors generally use stethoscopes to listen for the characteristic lub-dub sounds made by heart valves opening and closing. They also listen for less prominent sounds that indicate problems with these valves.... Read moreCritical Care
view channel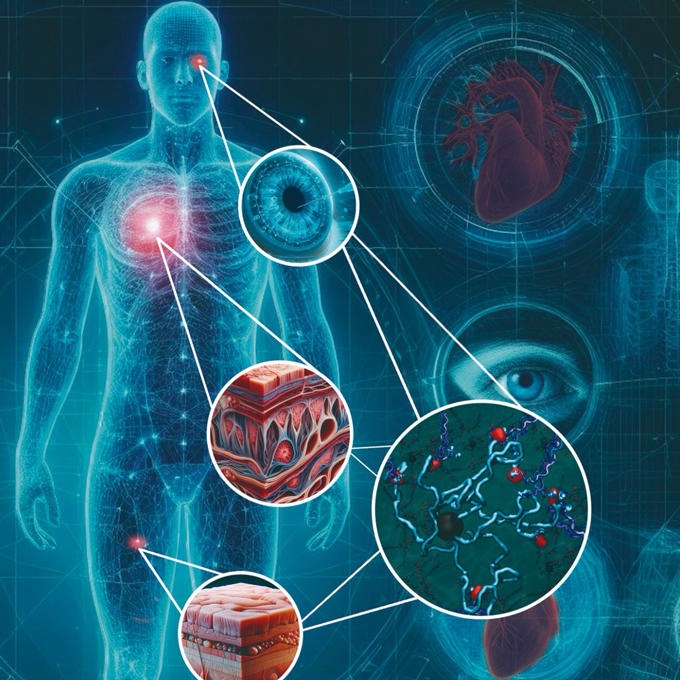
Peptide-Based Hydrogels Repair Damaged Organs and Tissues On-The-Spot
Scientists have ingeniously combined biomedical expertise with nature-inspired engineering to develop a jelly-like material that holds significant promise for immediate repairs to a wide variety of damaged... Read more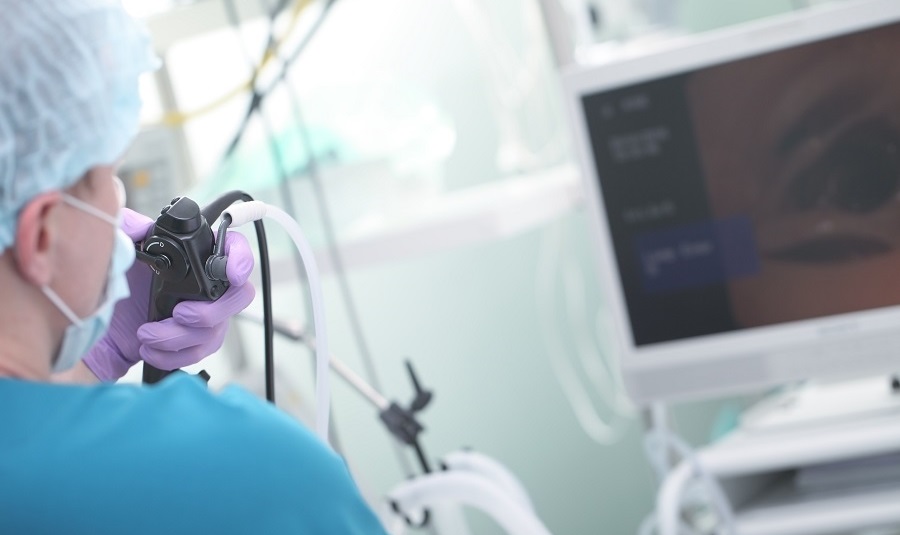
One-Hour Endoscopic Procedure Could Eliminate Need for Insulin for Type 2 Diabetes
Over 37 million Americans are diagnosed with diabetes, and more than 90% of these cases are Type 2 diabetes. This form of diabetes is most commonly seen in individuals over 45, though an increasing number... Read moreSurgical Techniques
view channel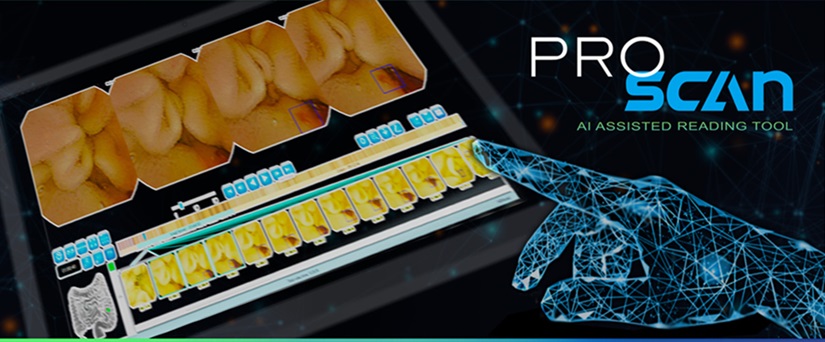
AI Assisted Reading Tool for Small Bowel Video Capsule Endoscopy Detects More Lesions
A revolutionary artificial intelligence (AI) technology that has proven faster and more accurate in diagnosing small bowel bleeding could transform gastrointestinal medicine. AnX Robotica (Plano, TX,... Read more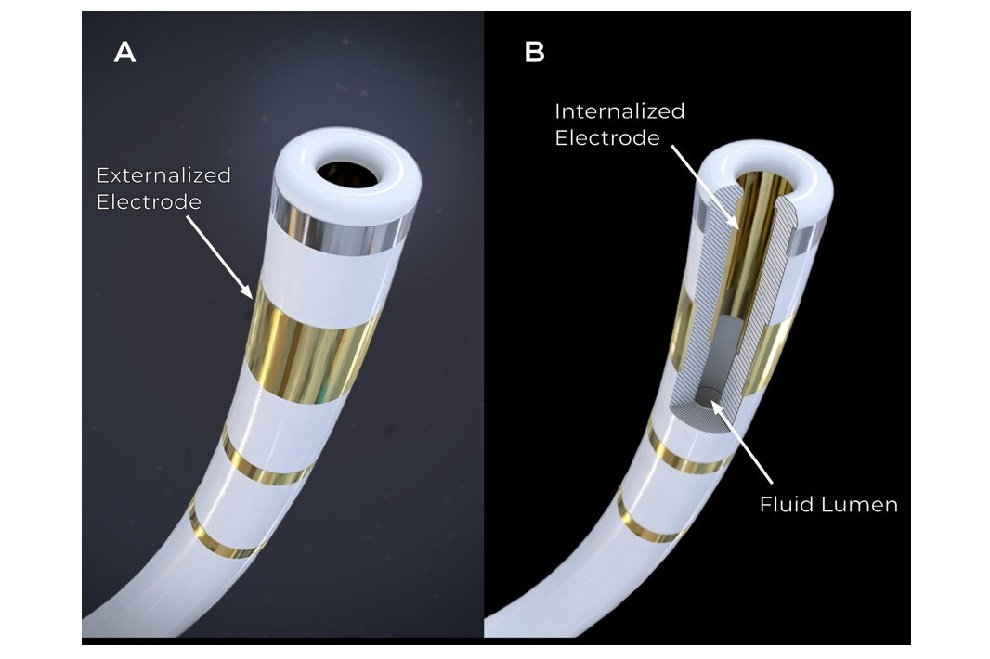
First-Ever Contact Force Pulsed Field Ablation System to Transform Treatment of Ventricular Arrhythmias
It is estimated that over 6 million patients in the US and Europe are affected by ventricular arrhythmias, which include conditions such as ventricular tachycardia (VT) and premature ventricular contractions (PVCs).... Read more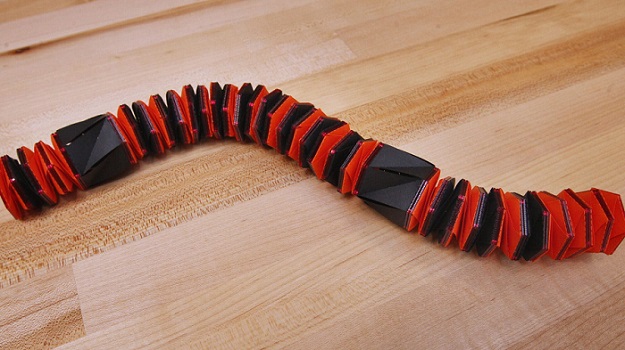
Caterpillar Robot with Built-In Steering System Crawls Easily Through Loops and Bends
Soft robots often face challenges in being guided effectively because adding steering mechanisms typically reduces their flexibility by increasing rigidity. Now, a team of engineers has combined ancient... Read more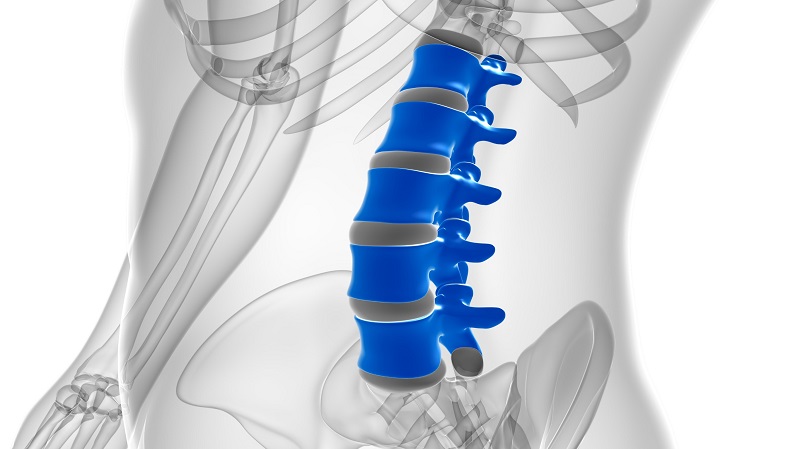
Tiny Wraparound Electronic Implants to Revolutionize Treatment of Spinal Cord Injuries
The spinal cord functions as a vital conduit, transmitting nerve impulses to and from the brain, much like a highway. When the spinal cord is damaged, this flow of information is disrupted, leading to... Read morePatient Care
view channel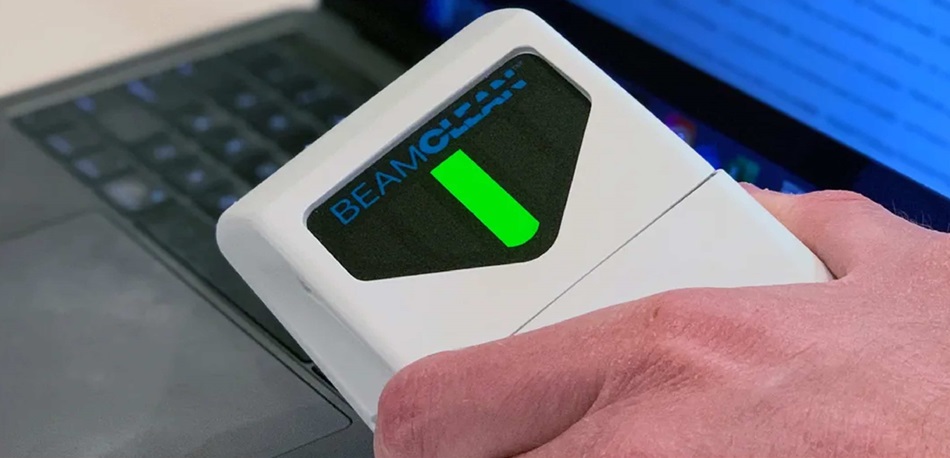
First-Of-Its-Kind Portable Germicidal Light Technology Disinfects High-Touch Clinical Surfaces in Seconds
Reducing healthcare-acquired infections (HAIs) remains a pressing issue within global healthcare systems. In the United States alone, 1.7 million patients contract HAIs annually, leading to approximately... Read more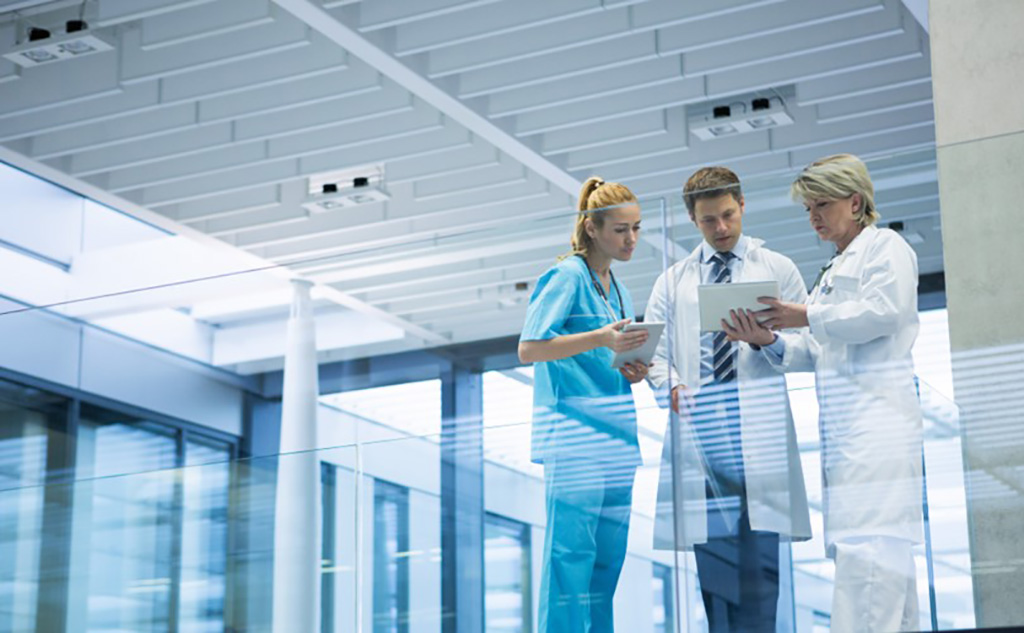
Surgical Capacity Optimization Solution Helps Hospitals Boost OR Utilization
An innovative solution has the capability to transform surgical capacity utilization by targeting the root cause of surgical block time inefficiencies. Fujitsu Limited’s (Tokyo, Japan) Surgical Capacity... Read more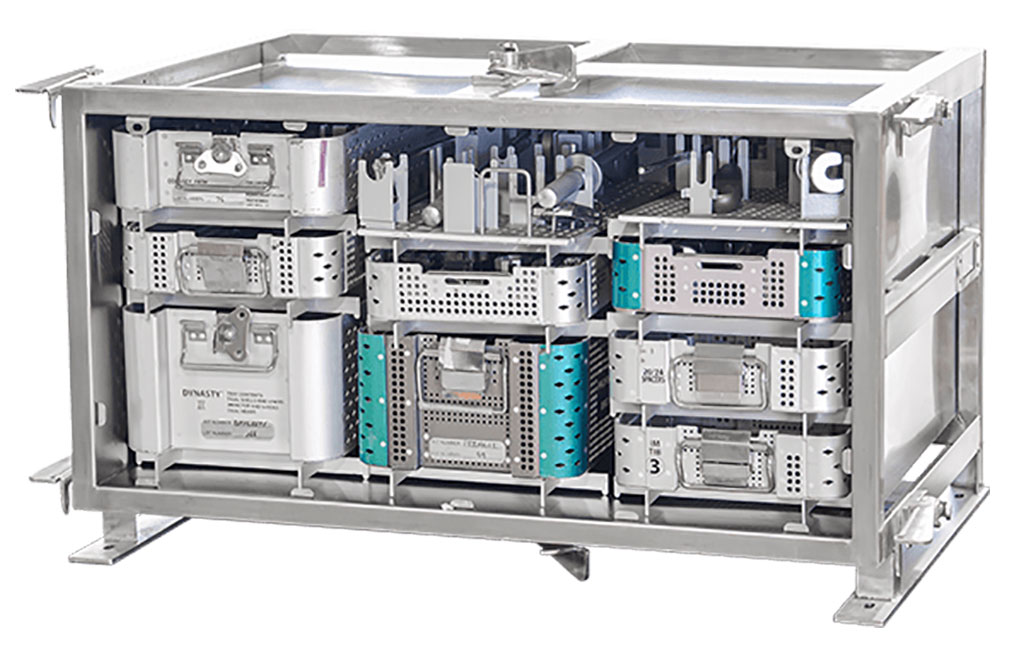
Game-Changing Innovation in Surgical Instrument Sterilization Significantly Improves OR Throughput
A groundbreaking innovation enables hospitals to significantly improve instrument processing time and throughput in operating rooms (ORs) and sterile processing departments. Turbett Surgical, Inc.... Read moreHealth IT
view channel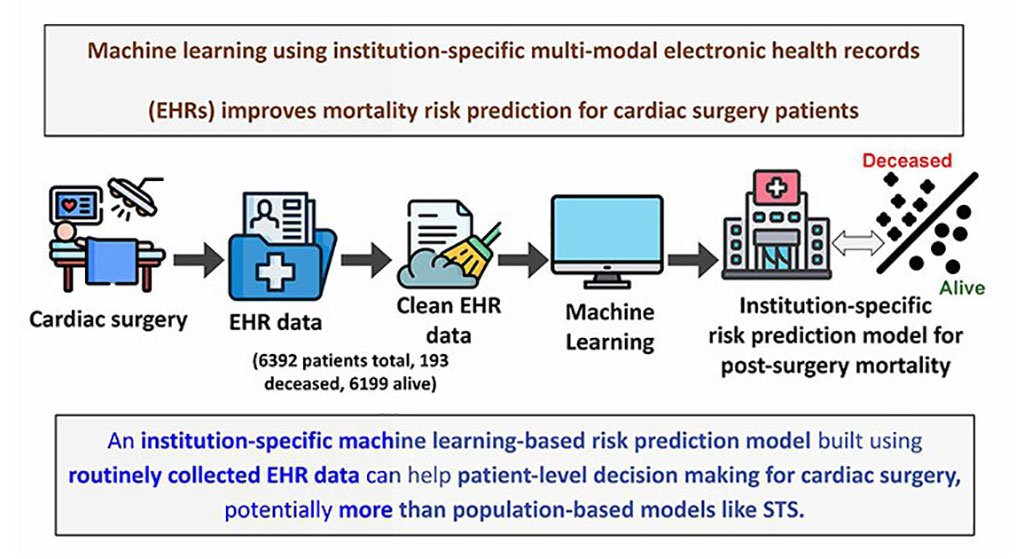
Machine Learning Model Improves Mortality Risk Prediction for Cardiac Surgery Patients
Machine learning algorithms have been deployed to create predictive models in various medical fields, with some demonstrating improved outcomes compared to their standard-of-care counterparts.... Read more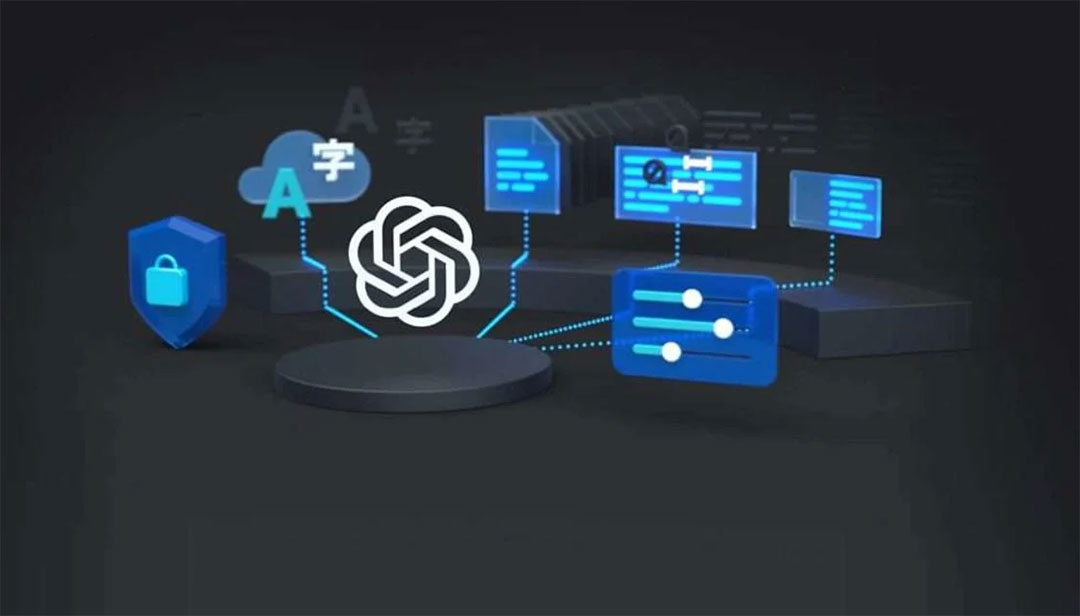
Strategic Collaboration to Develop and Integrate Generative AI into Healthcare
Top industry experts have underscored the immediate requirement for healthcare systems and hospitals to respond to severe cost and margin pressures. Close to half of U.S. hospitals ended 2022 in the red... Read more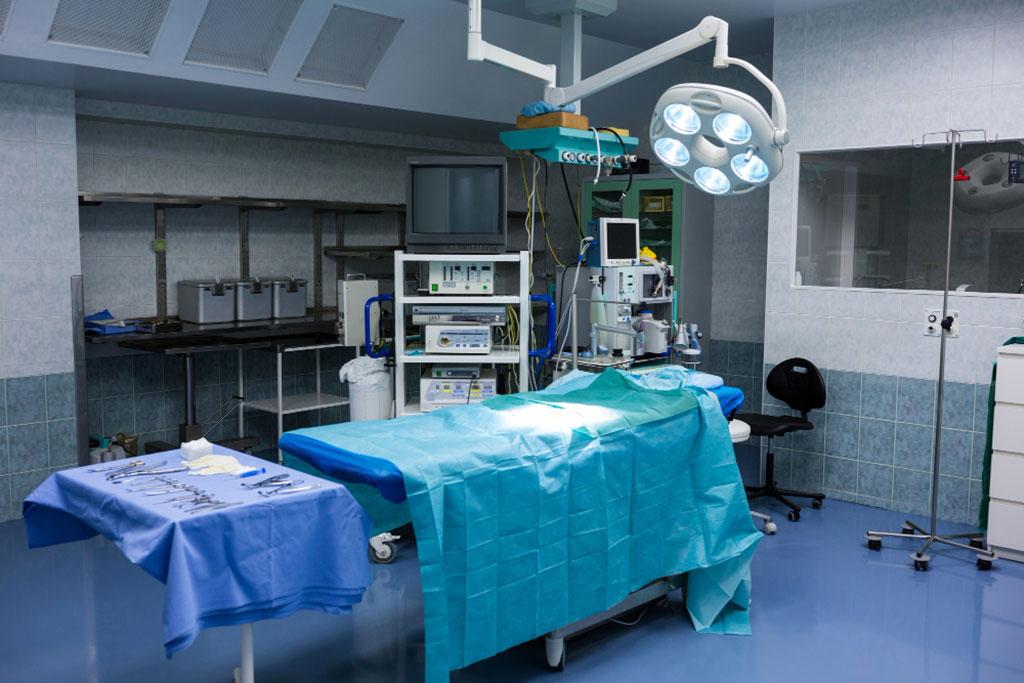
AI-Enabled Operating Rooms Solution Helps Hospitals Maximize Utilization and Unlock Capacity
For healthcare organizations, optimizing operating room (OR) utilization during prime time hours is a complex challenge. Surgeons and clinics face difficulties in finding available slots for booking cases,... Read more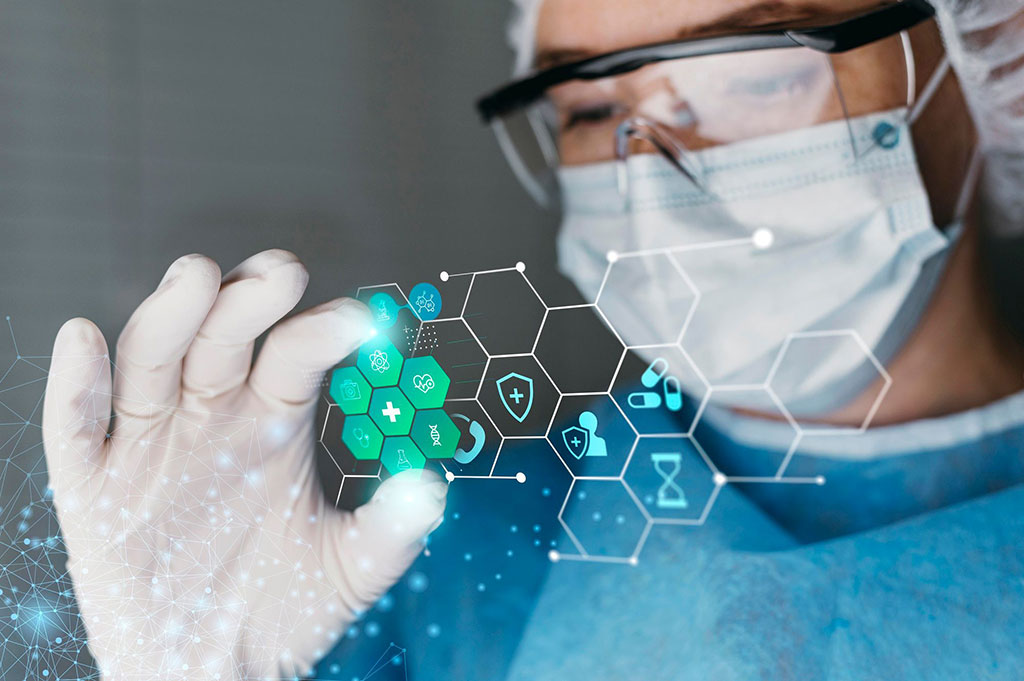
AI Predicts Pancreatic Cancer Three Years before Diagnosis from Patients’ Medical Records
Screening for common cancers like breast, cervix, and prostate cancer relies on relatively simple and highly effective techniques, such as mammograms, Pap smears, and blood tests. These methods have revolutionized... Read morePoint of Care
view channel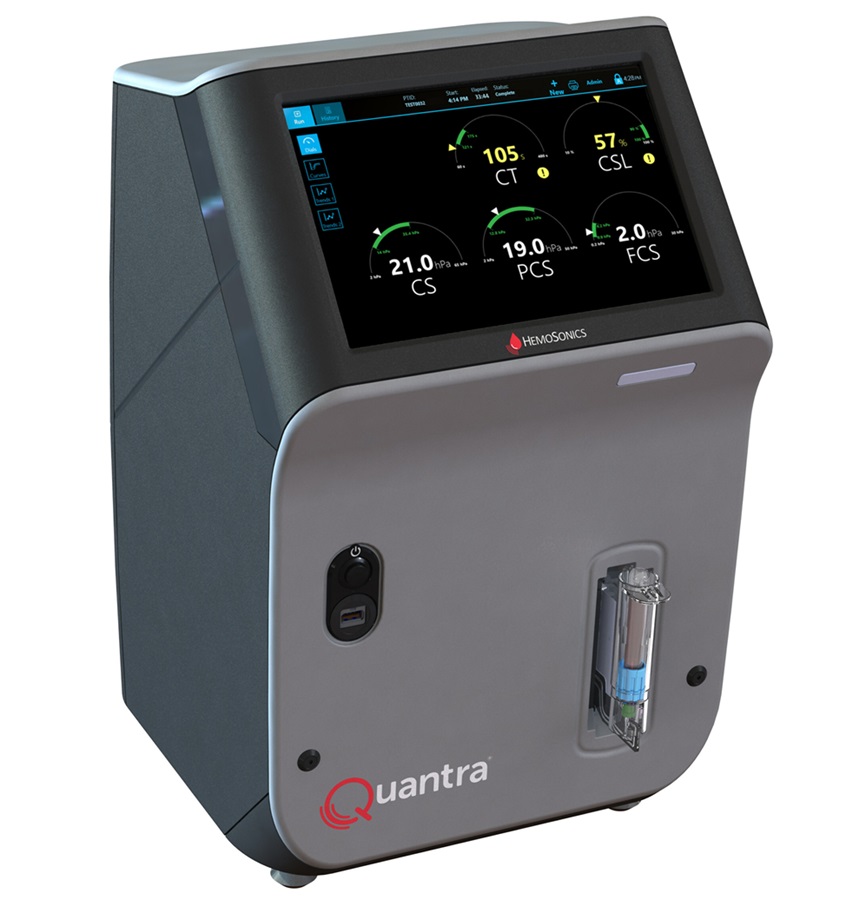
Critical Bleeding Management System to Help Hospitals Further Standardize Viscoelastic Testing
Surgical procedures are often accompanied by significant blood loss and the subsequent high likelihood of the need for allogeneic blood transfusions. These transfusions, while critical, are linked to various... Read more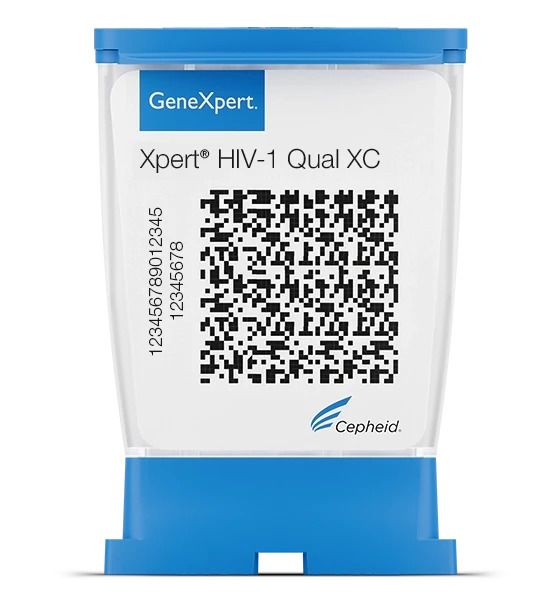
Point of Care HIV Test Enables Early Infection Diagnosis for Infants
Early diagnosis and initiation of treatment are crucial for the survival of infants infected with HIV (human immunodeficiency virus). Without treatment, approximately 50% of infants who acquire HIV during... Read more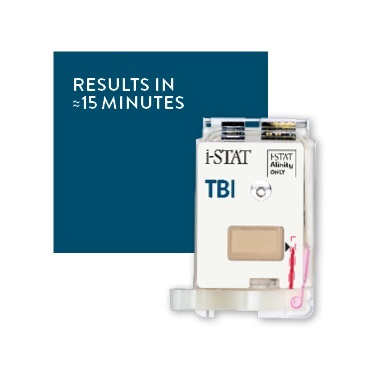
Whole Blood Rapid Test Aids Assessment of Concussion at Patient's Bedside
In the United States annually, approximately five million individuals seek emergency department care for traumatic brain injuries (TBIs), yet over half of those suspecting a concussion may never get it checked.... Read more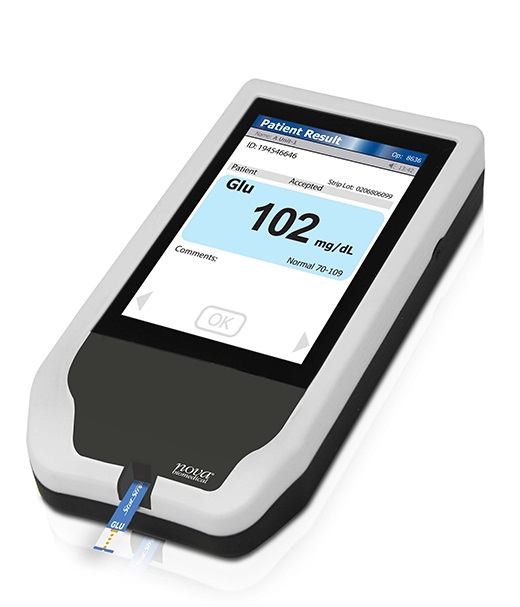
New Generation Glucose Hospital Meter System Ensures Accurate, Interference-Free and Safe Use
A new generation glucose hospital meter system now comes with several features that make hospital glucose testing easier and more secure while continuing to offer accuracy, freedom from interference, and... Read moreBusiness
view channel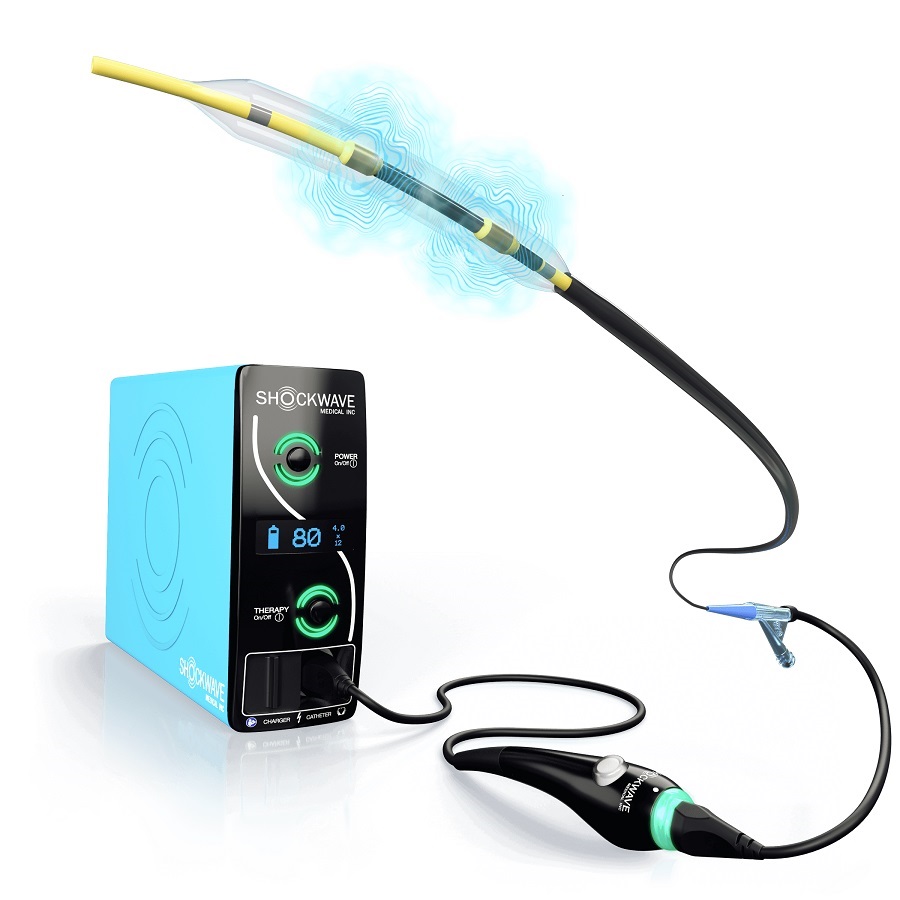
Johnson & Johnson Acquires Cardiovascular Medical Device Company Shockwave Medical
Johnson & Johnson (New Brunswick, N.J., USA) and Shockwave Medical (Santa Clara, CA, USA) have entered into a definitive agreement under which Johnson & Johnson will acquire all of Shockwave’s... Read more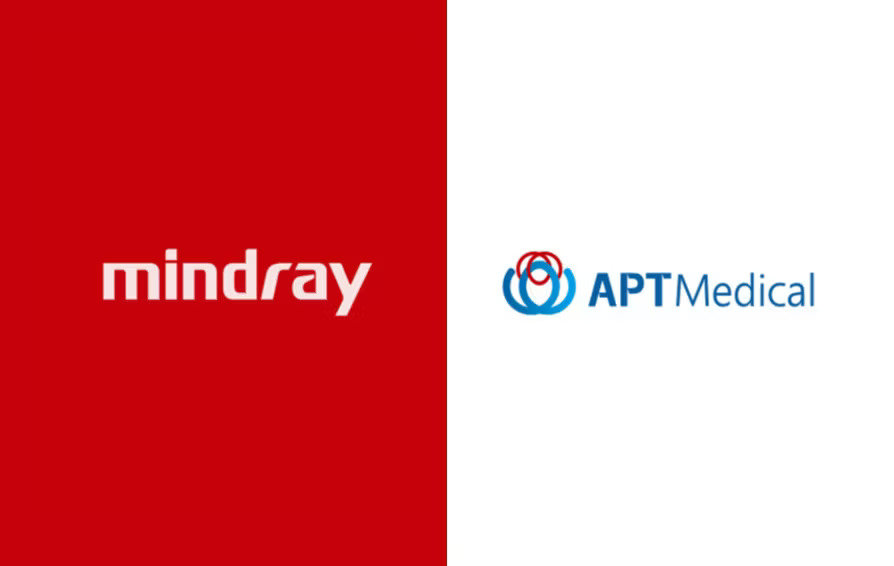