AI More Accurate at Predicting Heart Attacks than Physicians
By HospiMedica International staff writers Posted on 18 May 2019 |
Researchers from the Turku PET Centre (Turku, Finland) have developed an algorithm that “learned” how imaging data interacts by repeatedly analyzing 85 variables in 950 patients with known six-year outcomes. The algorithm then identified patterns correlating the variables to death and heart attack with more than 90% accuracy.
Doctors use risk scores to make treatment decisions, although these scores are based on just a handful of variables and often have modest accuracy in individual patients. Through repetition and adjustment, machine learning can exploit large amounts of data and identify complex patterns that may not be evident to humans.
For the study, the researchers enrolled 950 patients with chest pain who underwent the centre’s usual protocol to look for coronary artery disease. A coronary computed tomography angiography (CCTA) scan yielded 58 pieces of data on presence of coronary plaque, vessel narrowing, and calcification. Those with scans suggestive of disease underwent a positron emission tomography (PET) scan, which produced 17 variables on blood flow.
Ten clinical variables were obtained from medical records including sex, age, smoking and diabetes. During an average six-year follow-up there were 24 heart attacks and 49 deaths from any cause. The 85 variables were entered into a machine-learning algorithm called LogitBoost, which analyzed them over and over again until it found the best structure to predict who had a heart attack or died.
The predictive performance using the ten clinical variables alone (similar to current clinical practice) was modest, with an area under the curve (AUC) of 0.65 (where 1.0 is a perfect test and 0.5 is a random result). When PET data were added, AUC increased to 0.69. The predictive performance increased significantly (p=0.005) when CCTA data were added to clinical and PET data, giving an AUC 0.82 and more than 90% accuracy.
“Our study shows that very high dimensional patterns are more useful than single dimensional patterns to predict outcomes in individuals and for that we need machine learning,” said study author Dr. Luis Eduardo Juarez-Orozco. “Doctors already collect a lot of information about patients – for example those with chest pain. We found that machine learning can integrate these data and accurately predict individual risk. This should allow us to personalize treatment and ultimately lead to better outcomes for patients.”
Related Links:
Turku PET Centre
Doctors use risk scores to make treatment decisions, although these scores are based on just a handful of variables and often have modest accuracy in individual patients. Through repetition and adjustment, machine learning can exploit large amounts of data and identify complex patterns that may not be evident to humans.
For the study, the researchers enrolled 950 patients with chest pain who underwent the centre’s usual protocol to look for coronary artery disease. A coronary computed tomography angiography (CCTA) scan yielded 58 pieces of data on presence of coronary plaque, vessel narrowing, and calcification. Those with scans suggestive of disease underwent a positron emission tomography (PET) scan, which produced 17 variables on blood flow.
Ten clinical variables were obtained from medical records including sex, age, smoking and diabetes. During an average six-year follow-up there were 24 heart attacks and 49 deaths from any cause. The 85 variables were entered into a machine-learning algorithm called LogitBoost, which analyzed them over and over again until it found the best structure to predict who had a heart attack or died.
The predictive performance using the ten clinical variables alone (similar to current clinical practice) was modest, with an area under the curve (AUC) of 0.65 (where 1.0 is a perfect test and 0.5 is a random result). When PET data were added, AUC increased to 0.69. The predictive performance increased significantly (p=0.005) when CCTA data were added to clinical and PET data, giving an AUC 0.82 and more than 90% accuracy.
“Our study shows that very high dimensional patterns are more useful than single dimensional patterns to predict outcomes in individuals and for that we need machine learning,” said study author Dr. Luis Eduardo Juarez-Orozco. “Doctors already collect a lot of information about patients – for example those with chest pain. We found that machine learning can integrate these data and accurately predict individual risk. This should allow us to personalize treatment and ultimately lead to better outcomes for patients.”
Related Links:
Turku PET Centre
Latest AI News
- AI-Powered Algorithm to Revolutionize Detection of Atrial Fibrillation
- AI Diagnostic Tool Accurately Detects Valvular Disorders Often Missed by Doctors
- New Model Predicts 10 Year Breast Cancer Risk
- AI Tool Accurately Predicts Cancer Three Years Prior to Diagnosis
- Ground-Breaking Tool Predicts 10-Year Risk of Esophageal Cancer
Channels
Artificial Intelligence
view channel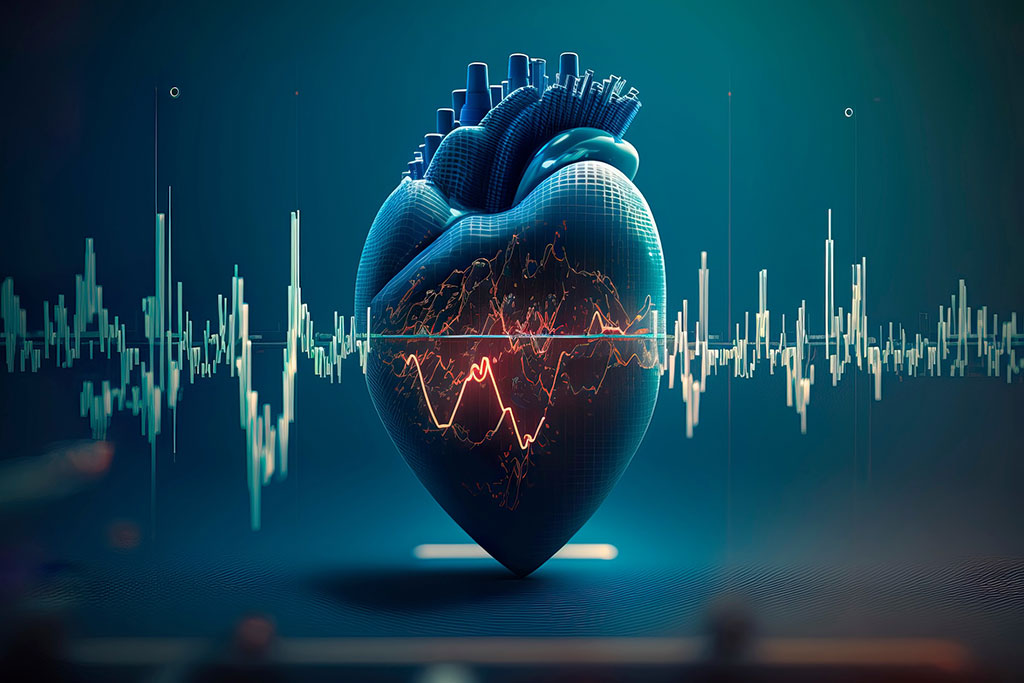
AI-Powered Algorithm to Revolutionize Detection of Atrial Fibrillation
Atrial fibrillation (AFib), a condition characterized by an irregular and often rapid heart rate, is linked to increased risks of stroke and heart failure. This is because the irregular heartbeat in AFib... Read more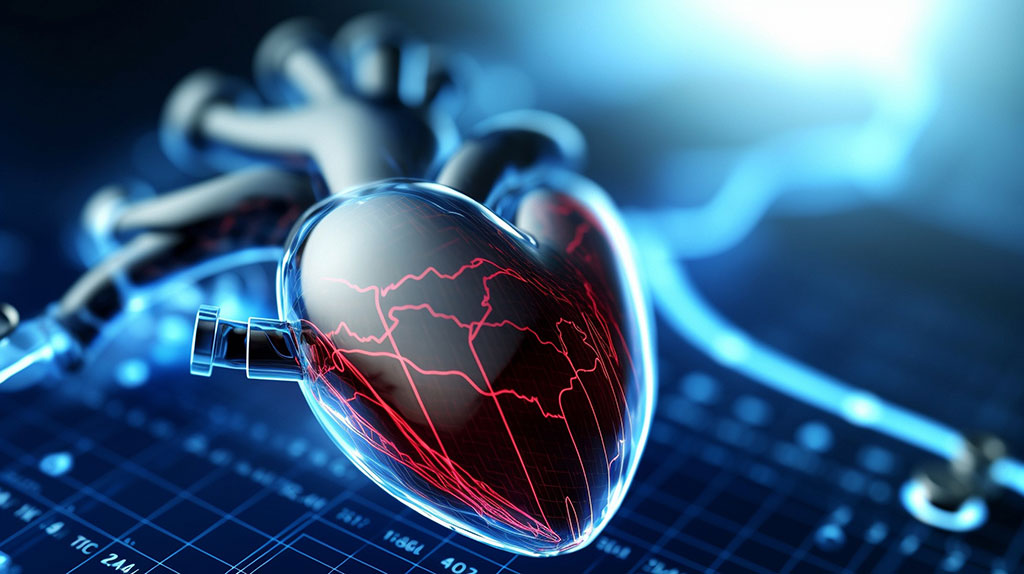
AI Diagnostic Tool Accurately Detects Valvular Disorders Often Missed by Doctors
Doctors generally use stethoscopes to listen for the characteristic lub-dub sounds made by heart valves opening and closing. They also listen for less prominent sounds that indicate problems with these valves.... Read moreCritical Care
view channel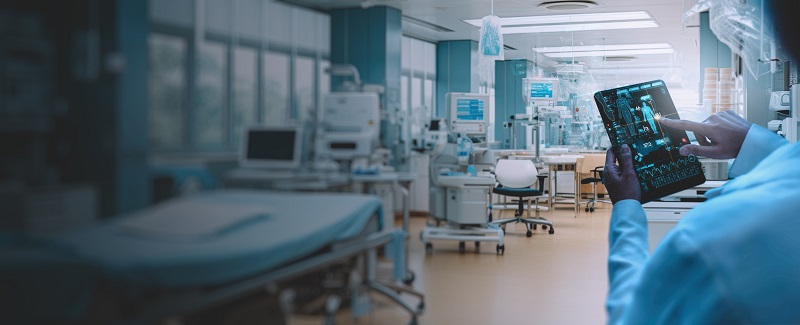
AI Can Prioritize Emergecny Department Patients Requiring Urgent Treatment
Emergency departments across the world are facing severe overcrowding and excessive demands, but a new study indicates that artificial intelligence (AI) might soon assist in prioritizing patients who require... Read more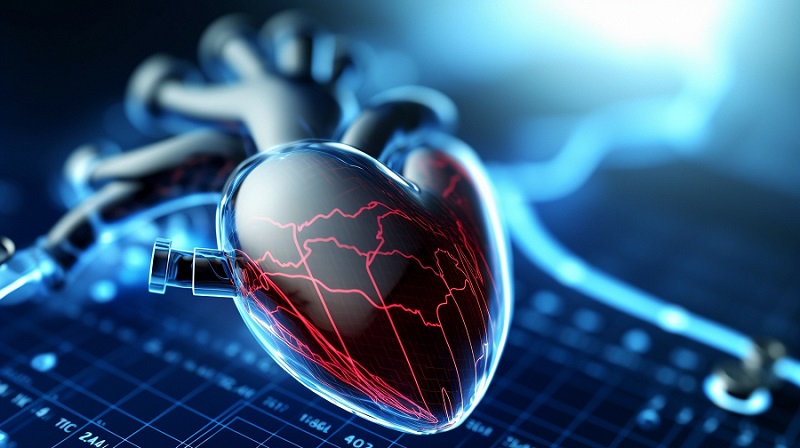
AI to Improve Diagnosis of Atrial Fibrillation
Abnormal heart rhythms frequently arise from—and contribute to—structural abnormalities in the heart. Atrial fibrillation is a specific type of abnormal rhythm that may not be consistently present, often... Read more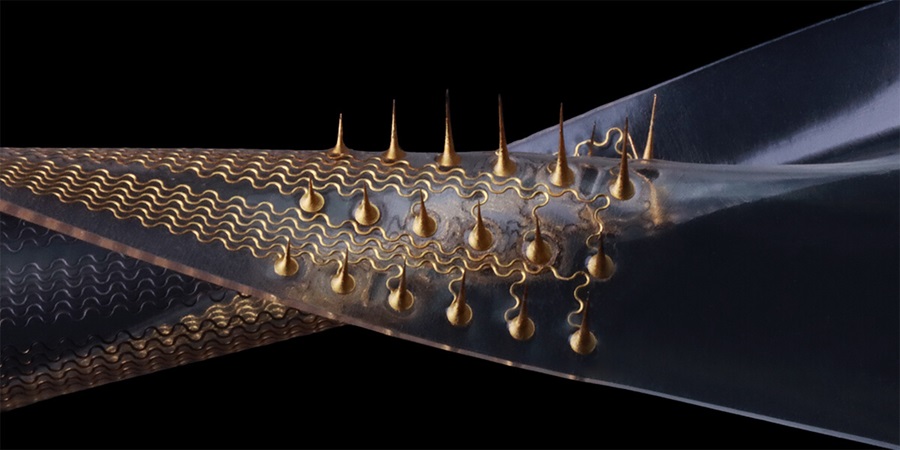
Stretchable Microneedles to Help In Accurate Tracking of Abnormalities and Identifying Rapid Treatment
The field of personalized medicine is transforming rapidly, with advancements like wearable devices and home testing kits making it increasingly easy to monitor a wide range of health metrics, from heart... Read more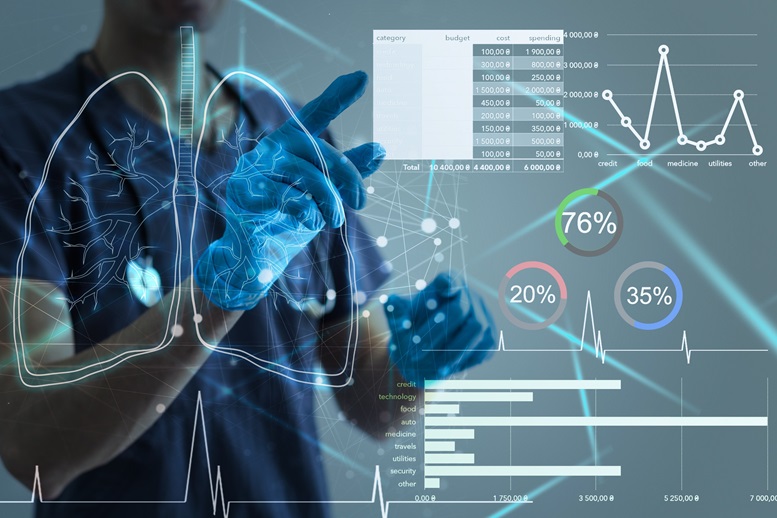
Machine Learning Tool Identifies Rare, Undiagnosed Immune Disorders from Patient EHRs
Patients suffering from rare diseases often endure extensive delays in receiving accurate diagnoses and treatments, which can lead to unnecessary tests, worsening health, psychological strain, and significant... Read moreSurgical Techniques
view channel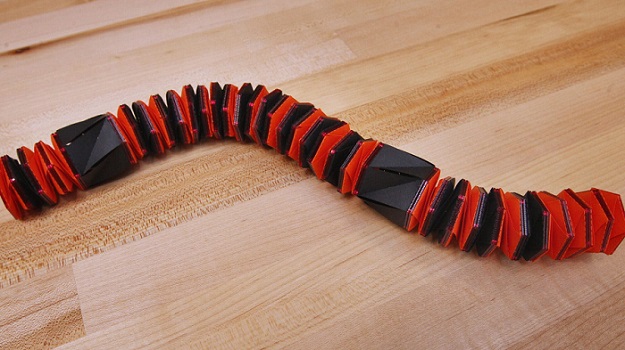
Caterpillar Robot with Built-In Steering System Crawls Easily Through Loops and Bends
Soft robots often face challenges in being guided effectively because adding steering mechanisms typically reduces their flexibility by increasing rigidity. Now, a team of engineers has combined ancient... Read more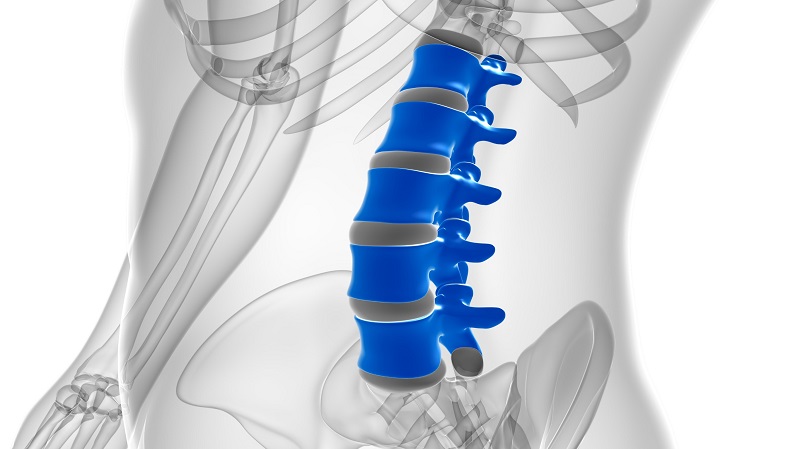
Tiny Wraparound Electronic Implants to Revolutionize Treatment of Spinal Cord Injuries
The spinal cord functions as a vital conduit, transmitting nerve impulses to and from the brain, much like a highway. When the spinal cord is damaged, this flow of information is disrupted, leading to... Read more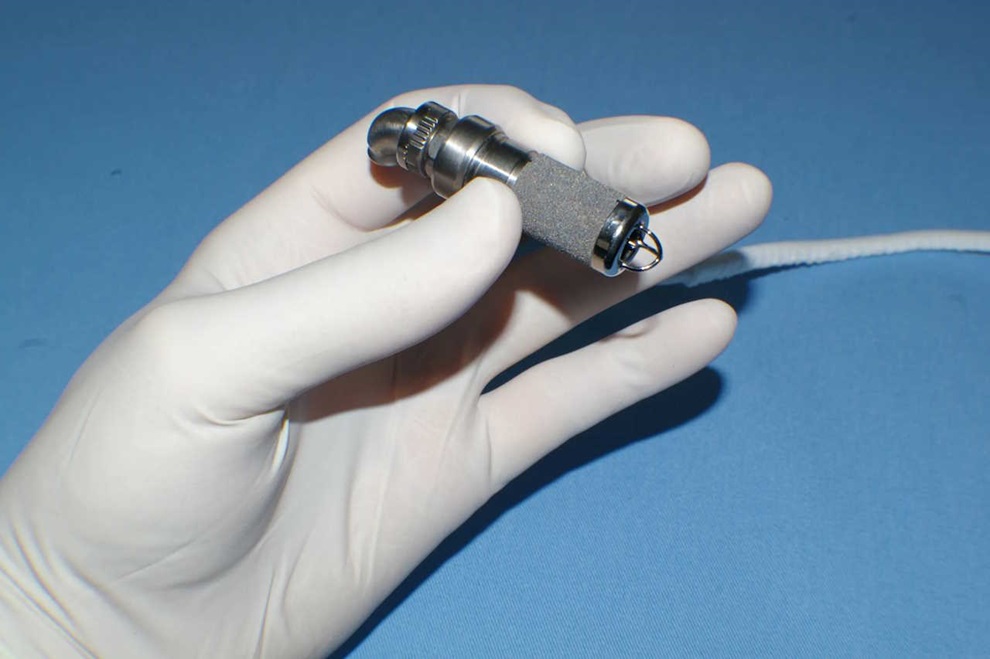
Small, Implantable Cardiac Pump to Help Children Awaiting Heart Transplant
Implantable ventricular assist devices, available for adults for over 40 years, fit inside the chest and are generally safer and easier to use than external devices. These devices enable patients to live... Read more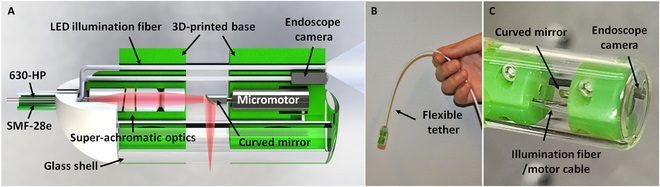
Gastrointestinal Imaging Capsule a Game-Changer in Esophagus Surveillance and Treatment
A newly-developed gastrointestinal imaging capsule is poised to be a game-changer in esophagus surveillance and interventions. The Multifunctional Ablative Gastrointestinal Imaging Capsule (MAGIC) developed... Read morePatient Care
view channel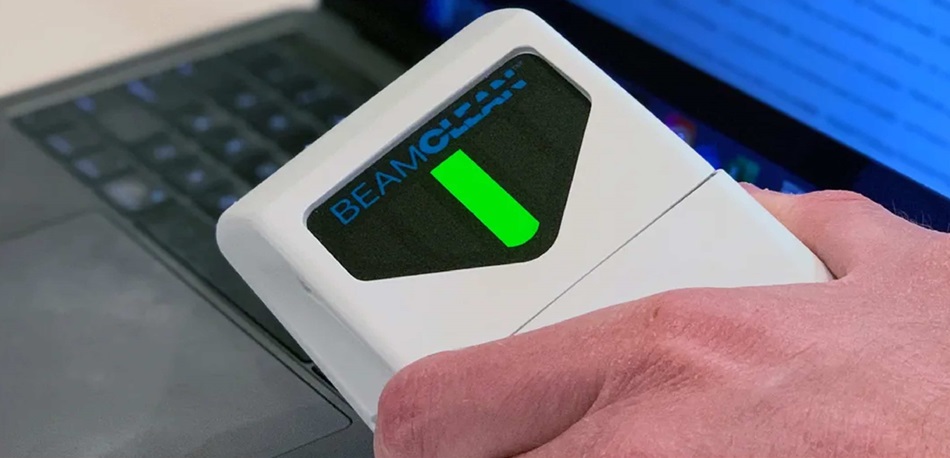
First-Of-Its-Kind Portable Germicidal Light Technology Disinfects High-Touch Clinical Surfaces in Seconds
Reducing healthcare-acquired infections (HAIs) remains a pressing issue within global healthcare systems. In the United States alone, 1.7 million patients contract HAIs annually, leading to approximately... Read more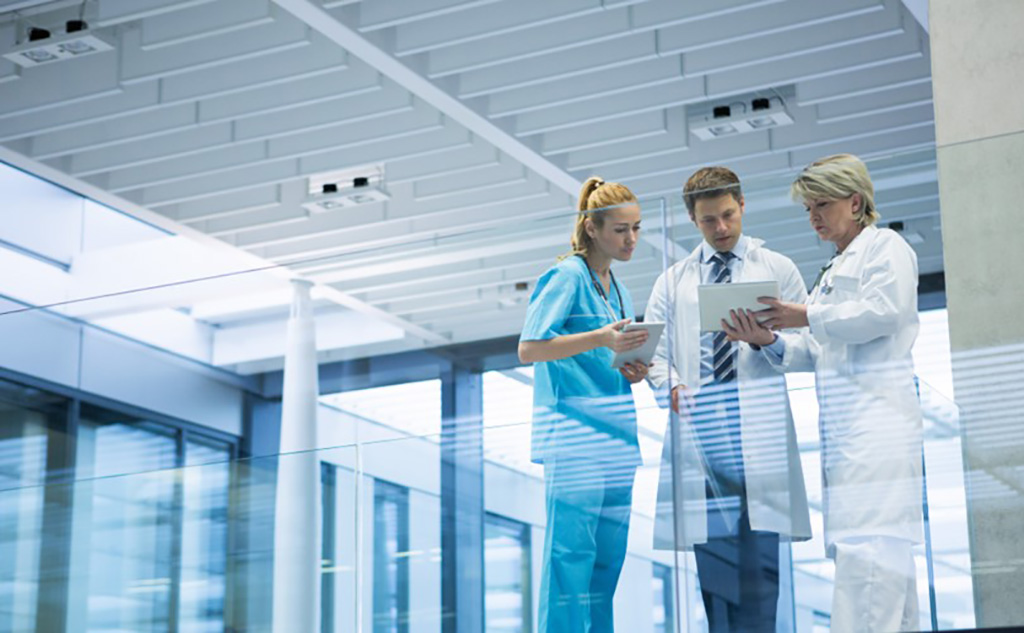
Surgical Capacity Optimization Solution Helps Hospitals Boost OR Utilization
An innovative solution has the capability to transform surgical capacity utilization by targeting the root cause of surgical block time inefficiencies. Fujitsu Limited’s (Tokyo, Japan) Surgical Capacity... Read more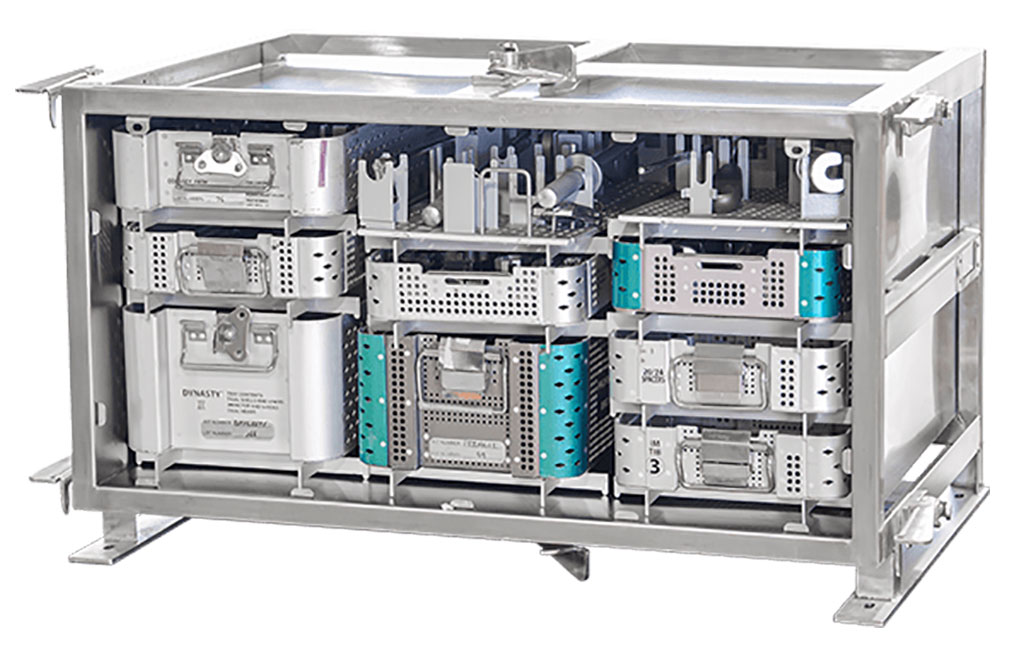
Game-Changing Innovation in Surgical Instrument Sterilization Significantly Improves OR Throughput
A groundbreaking innovation enables hospitals to significantly improve instrument processing time and throughput in operating rooms (ORs) and sterile processing departments. Turbett Surgical, Inc.... Read moreHealth IT
view channel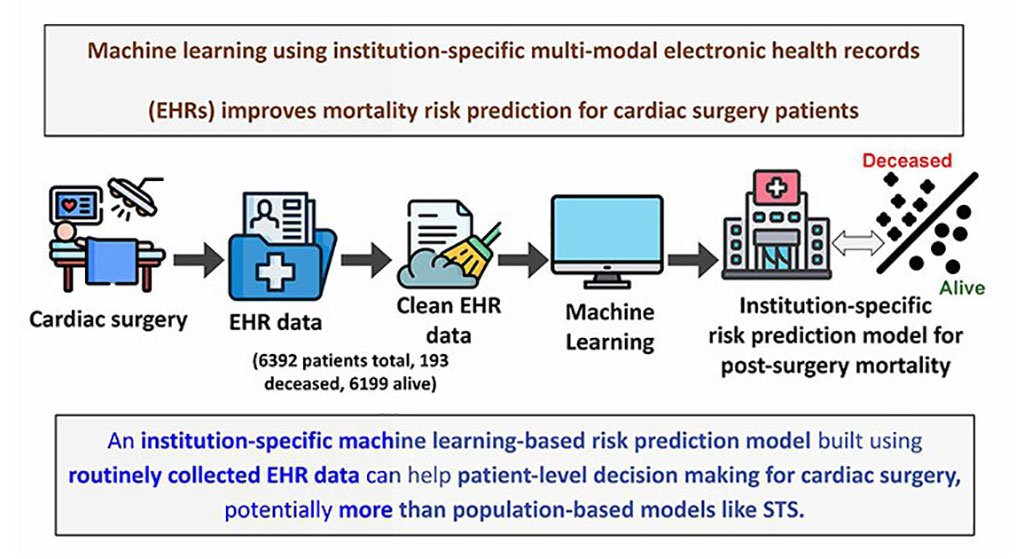
Machine Learning Model Improves Mortality Risk Prediction for Cardiac Surgery Patients
Machine learning algorithms have been deployed to create predictive models in various medical fields, with some demonstrating improved outcomes compared to their standard-of-care counterparts.... Read more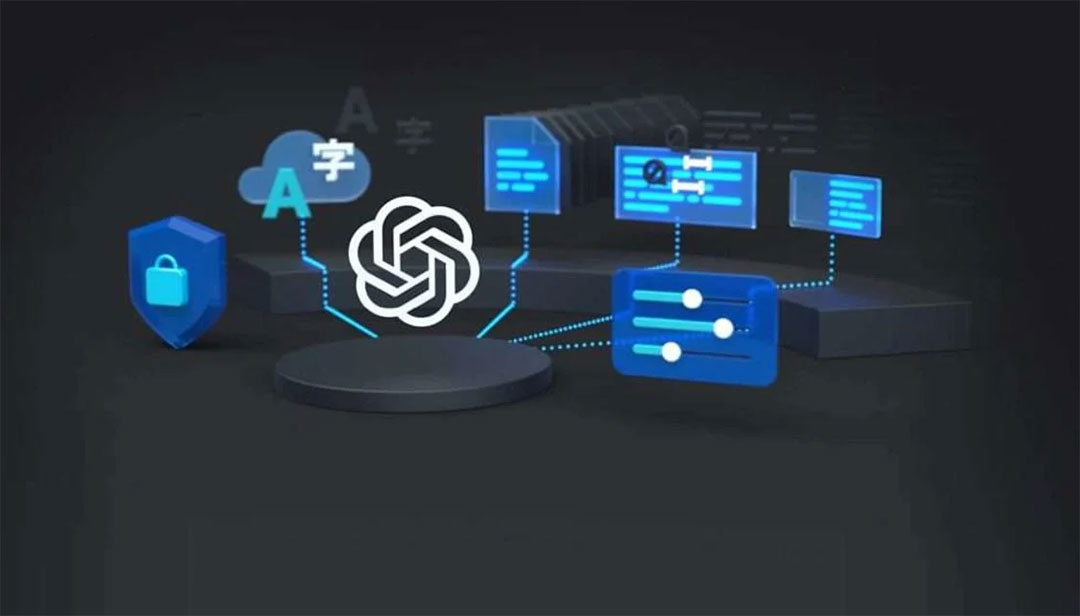
Strategic Collaboration to Develop and Integrate Generative AI into Healthcare
Top industry experts have underscored the immediate requirement for healthcare systems and hospitals to respond to severe cost and margin pressures. Close to half of U.S. hospitals ended 2022 in the red... Read more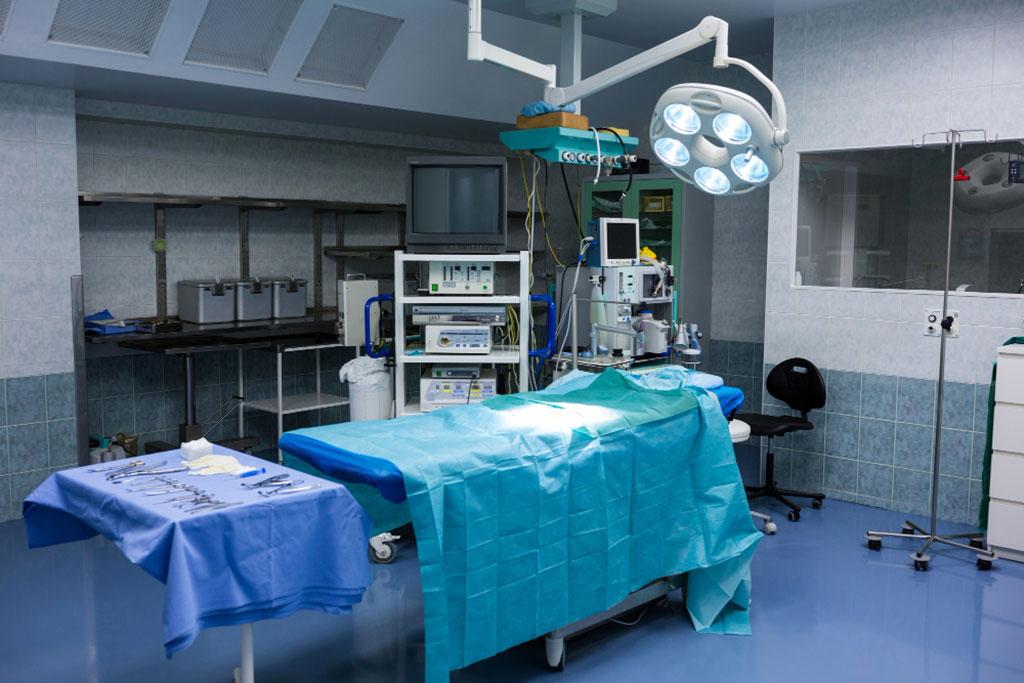
AI-Enabled Operating Rooms Solution Helps Hospitals Maximize Utilization and Unlock Capacity
For healthcare organizations, optimizing operating room (OR) utilization during prime time hours is a complex challenge. Surgeons and clinics face difficulties in finding available slots for booking cases,... Read more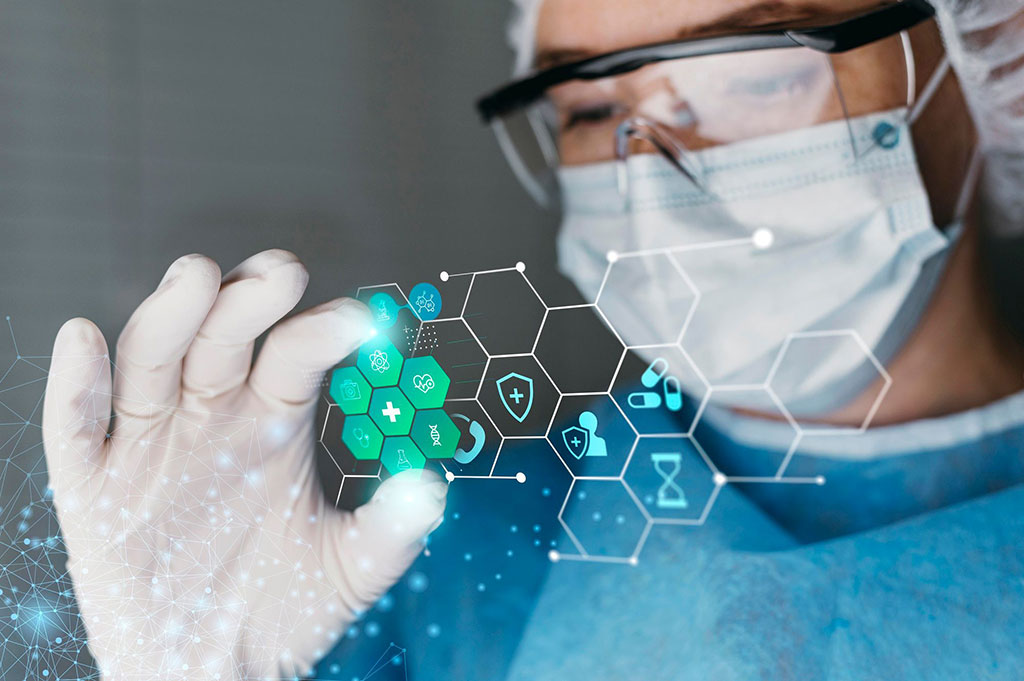
AI Predicts Pancreatic Cancer Three Years before Diagnosis from Patients’ Medical Records
Screening for common cancers like breast, cervix, and prostate cancer relies on relatively simple and highly effective techniques, such as mammograms, Pap smears, and blood tests. These methods have revolutionized... Read morePoint of Care
view channel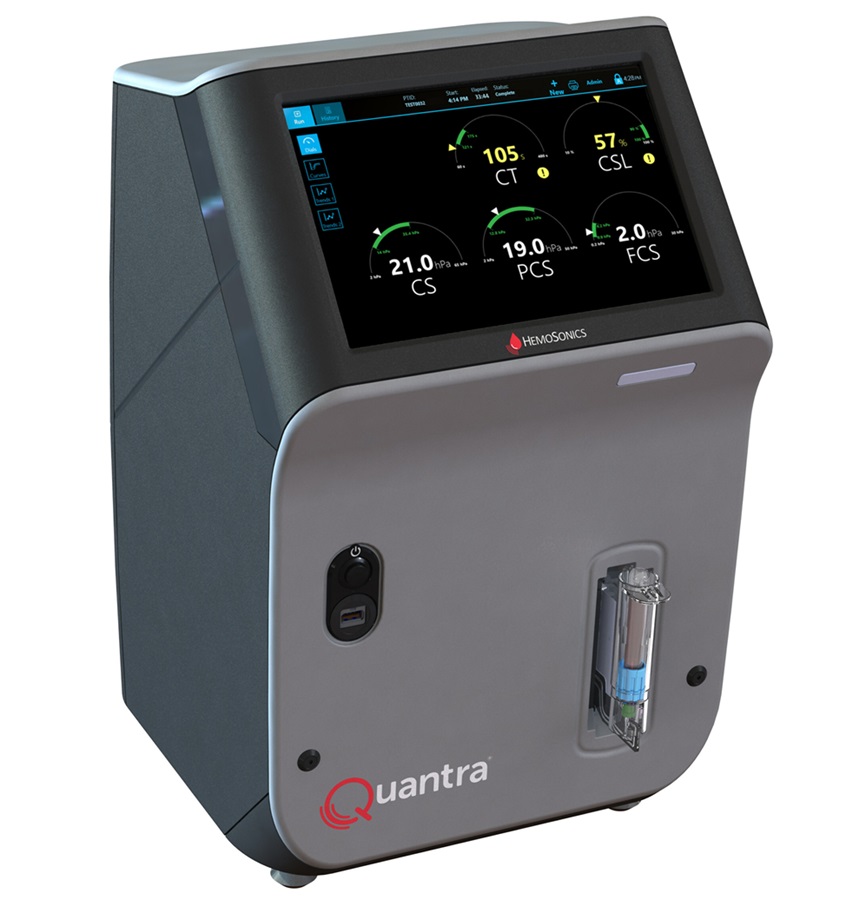
Critical Bleeding Management System to Help Hospitals Further Standardize Viscoelastic Testing
Surgical procedures are often accompanied by significant blood loss and the subsequent high likelihood of the need for allogeneic blood transfusions. These transfusions, while critical, are linked to various... Read more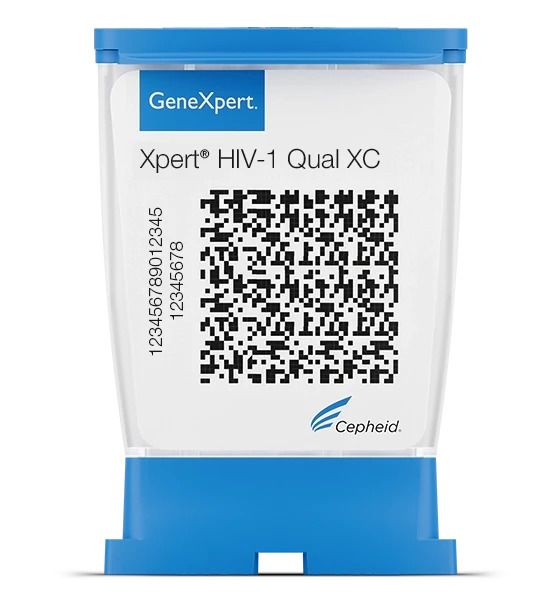
Point of Care HIV Test Enables Early Infection Diagnosis for Infants
Early diagnosis and initiation of treatment are crucial for the survival of infants infected with HIV (human immunodeficiency virus). Without treatment, approximately 50% of infants who acquire HIV during... Read more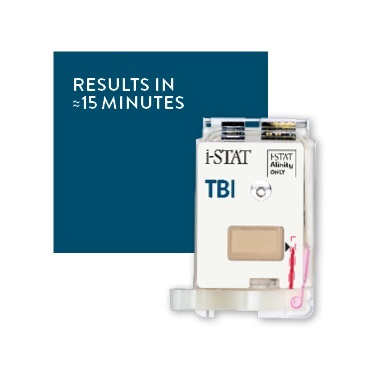
Whole Blood Rapid Test Aids Assessment of Concussion at Patient's Bedside
In the United States annually, approximately five million individuals seek emergency department care for traumatic brain injuries (TBIs), yet over half of those suspecting a concussion may never get it checked.... Read more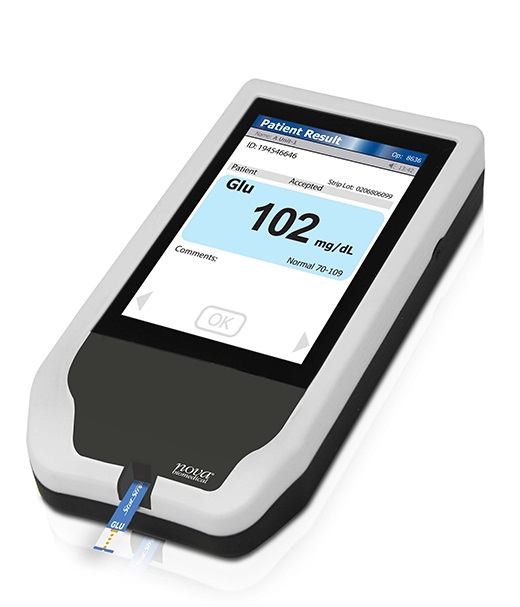
New Generation Glucose Hospital Meter System Ensures Accurate, Interference-Free and Safe Use
A new generation glucose hospital meter system now comes with several features that make hospital glucose testing easier and more secure while continuing to offer accuracy, freedom from interference, and... Read moreBusiness
view channel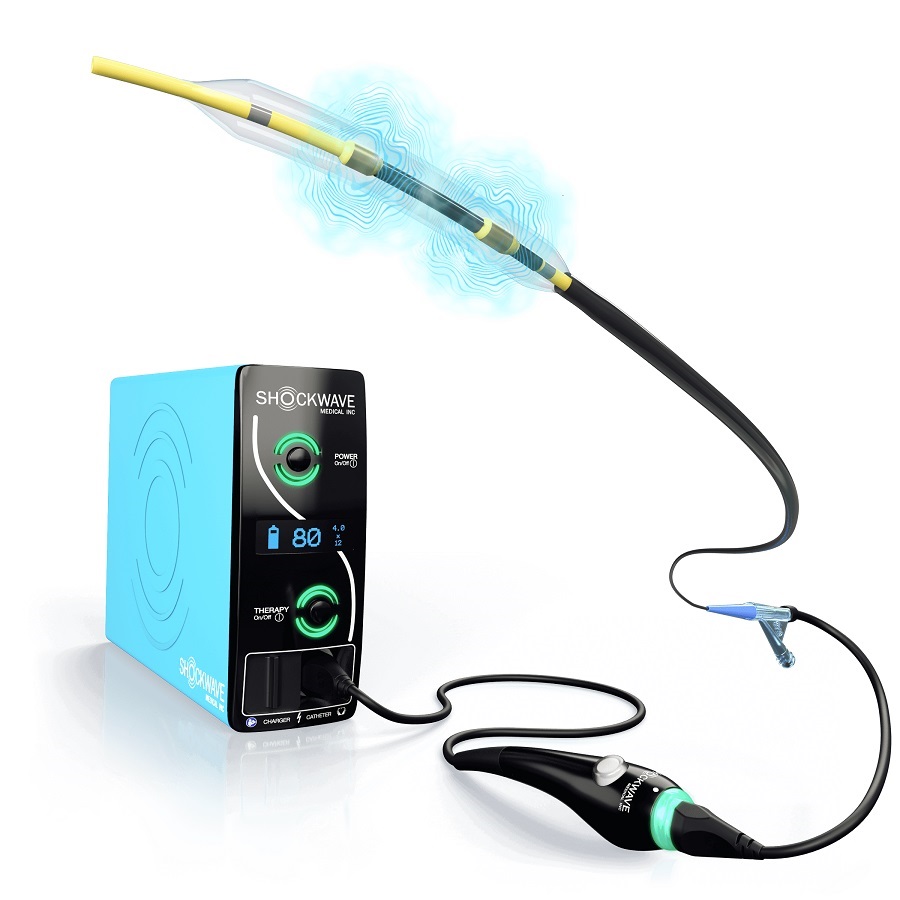
Johnson & Johnson Acquires Cardiovascular Medical Device Company Shockwave Medical
Johnson & Johnson (New Brunswick, N.J., USA) and Shockwave Medical (Santa Clara, CA, USA) have entered into a definitive agreement under which Johnson & Johnson will acquire all of Shockwave’s... Read more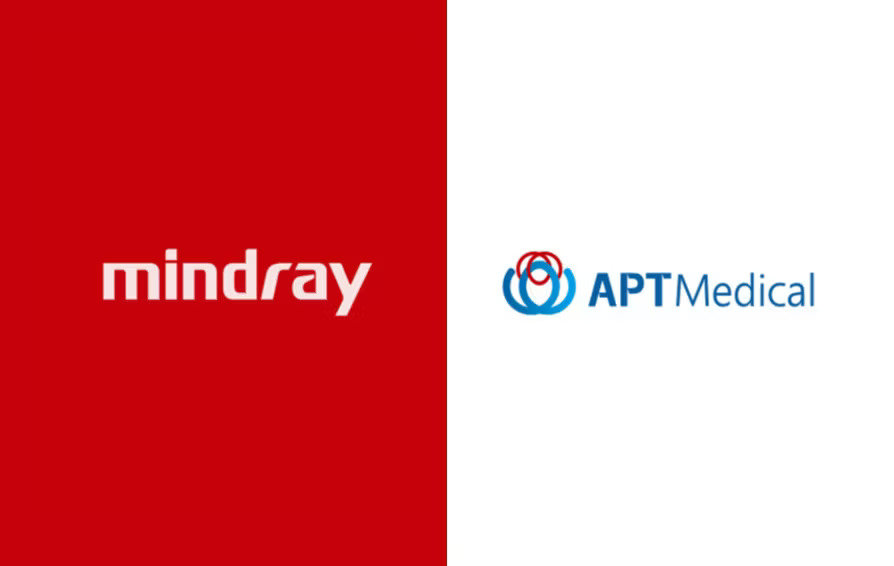