Smartwatch Detection of Atrial Fibrillation Has Great Potential, Finds Study
By HospiMedica International staff writers Posted on 14 Oct 2022 |
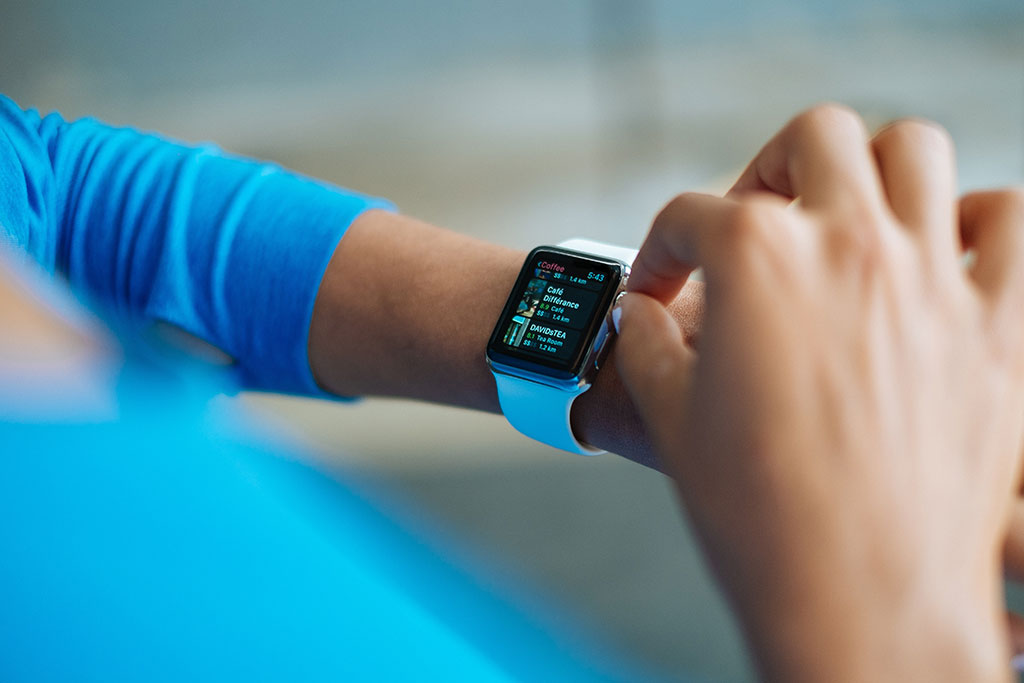
Extended cardiac monitoring in patients and the use of implantable cardiovascular electronic devices can increase detection of atrial fibrillation (AF), but the devices have limitations including short battery life and lack of immediate feedback. Can new smartphone tools that can record an electrocardiogram (ECG) strip and make an automated diagnosis overcome these limitations and facilitate timely diagnosis? In the largest study to date, researchers have found that the use of these devices is challenging in patients with abnormal ECGs, although better algorithms and machine learning may help these tools provide more accurate diagnoses.
In the first “real-world” study focusing on the use of the Apple Watch as a diagnostic tool for AF, investigators at the Bordeaux University Hospital (Bordeaux, France) looked at 734 consecutive hospitalized patients. Each patient underwent a 12-lead ECG, immediately followed by a 30-second Apple Watch recording. The smartwatch’s automated single-lead ECG AF detections were classified as “no signs of atrial fibrillation,” “atrial fibrillation,” or “inconclusive reading.” Smartwatch recordings were given to an electrophysiologist who conducted a blinded interpretation, assigning each tracing a diagnosis of “AF,” “absence of AF,” or “diagnosis unclear.” A second blinded electrophysiologist interpreted 100 randomly selected traces to determine the extent to which the observers agreed.
In approximately one in every five patients, the smartwatch ECG failed to produce an automatic diagnosis. The risk of having a false positive automated AF detection was higher for patients with premature atrial and ventricular contractions (PACs/PVCs), sinus node dysfunction, and second- or third-degree atrioventricular-block. For patients in AF, the risk of having a false negative tracing (missed AF) was higher for patients with ventricular conduction abnormalities (interventricular conduction delay) or rhythms controlled by an implanted pacemaker.
The cardiac electrophysiologists had a high level of agreement for differentiation between AF and non-AF. The smartphone app correctly identified 78% of the patients who were in AF and 81% who were not in AF. The electrophysiologists identified 97% of the patients who were in AF and 89% who were not. Patients with PVCs were three times more likely to have false positive AF diagnoses from the smartwatch ECG, and the identification of patients with atrial tachycardia (AT) and atrial flutter (AFL) was very poor.
“These observations are not surprising, as smartwatch automated detection algorithms are based solely on cycle variability,” said lead investigator Marc Strik, MD, PhD, LIRYC institute, Bordeaux University Hospital, Bordeaux, France, explaining that PVCs cause short and long cycles, which increase cycle variability. “Ideally, an algorithm would better discriminate between PVCs and AF. Any algorithm limited to the analysis of cycle variability will have poor accuracy in detecting AT/AFL. Machine learning approaches may increase smartwatch AF detection accuracy in these patients.”
“With the growing use of smartwatches in medicine, it is important to know which medical conditions and ECG abnormalities could impact and alter the detection of AF by the smartwatch in order to optimize the care of our patients,” Dr. Strik added. “Smartwatch detection of AF has great potential, but it is more challenging in patients with pre-existing cardiac disease.”
Related Links:
Bordeaux University Hospital
Latest AI News
- AI-Powered Algorithm to Revolutionize Detection of Atrial Fibrillation
- AI Diagnostic Tool Accurately Detects Valvular Disorders Often Missed by Doctors
- New Model Predicts 10 Year Breast Cancer Risk
- AI Tool Accurately Predicts Cancer Three Years Prior to Diagnosis
- Ground-Breaking Tool Predicts 10-Year Risk of Esophageal Cancer
Channels
Critical Care
view channel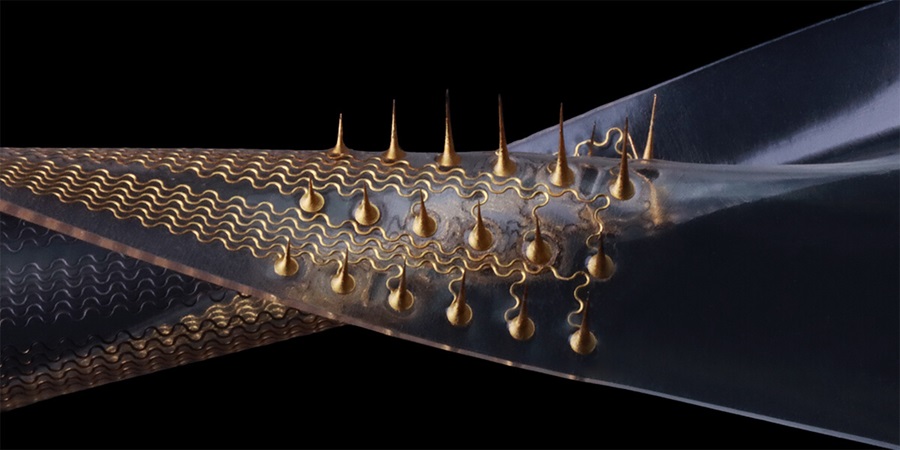
Stretchable Microneedles to Help In Accurate Tracking of Abnormalities and Identifying Rapid Treatment
The field of personalized medicine is transforming rapidly, with advancements like wearable devices and home testing kits making it increasingly easy to monitor a wide range of health metrics, from heart... Read more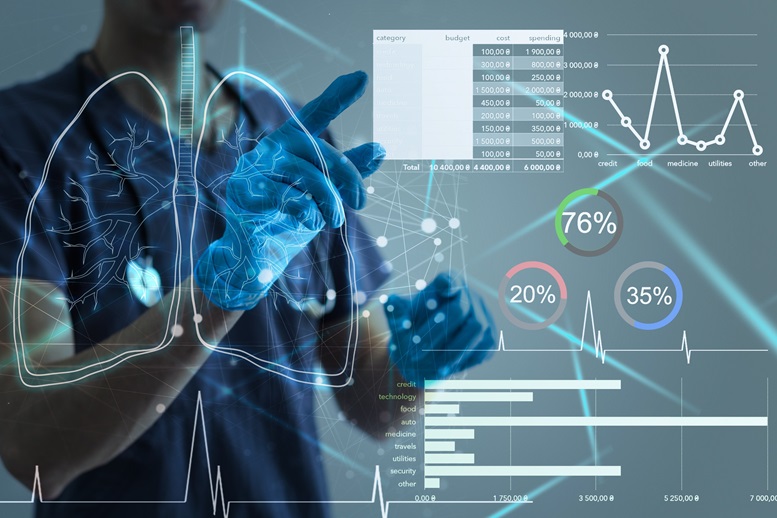
Machine Learning Tool Identifies Rare, Undiagnosed Immune Disorders from Patient EHRs
Patients suffering from rare diseases often endure extensive delays in receiving accurate diagnoses and treatments, which can lead to unnecessary tests, worsening health, psychological strain, and significant... Read more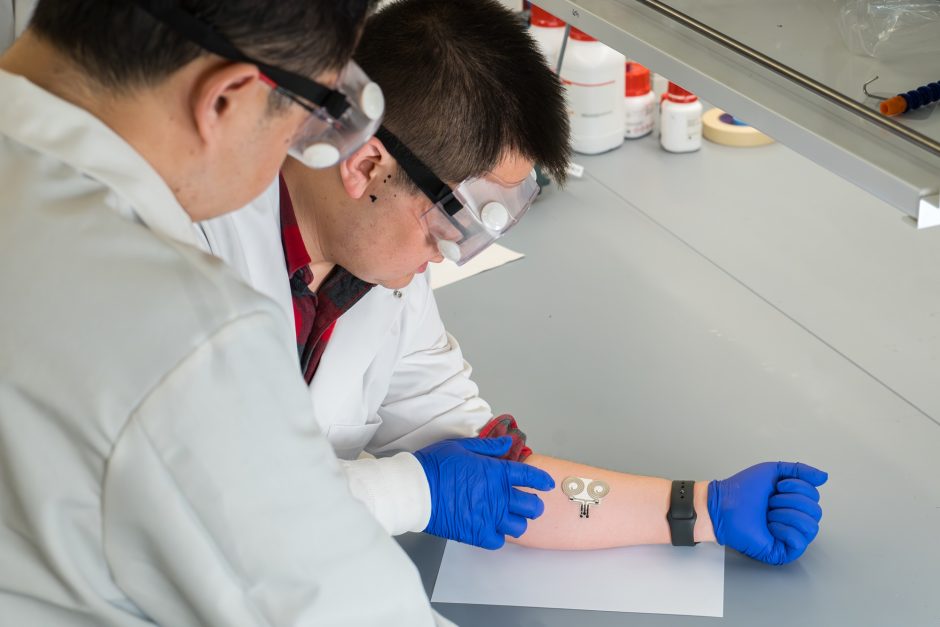
On-Skin Wearable Bioelectronic Device Paves Way for Intelligent Implants
A team of researchers at the University of Missouri (Columbia, MO, USA) has achieved a milestone in developing a state-of-the-art on-skin wearable bioelectronic device. This development comes from a lab... Read more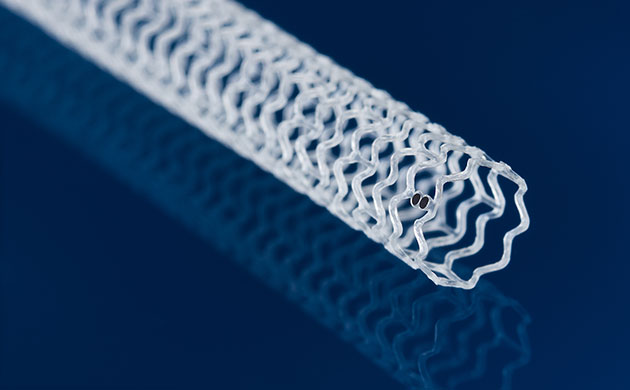
First-Of-Its-Kind Dissolvable Stent to Improve Outcomes for Patients with Severe PAD
Peripheral artery disease (PAD) affects millions and presents serious health risks, particularly its severe form, chronic limb-threatening ischemia (CLTI). CLTI develops when arteries are blocked by plaque,... Read moreSurgical Techniques
view channel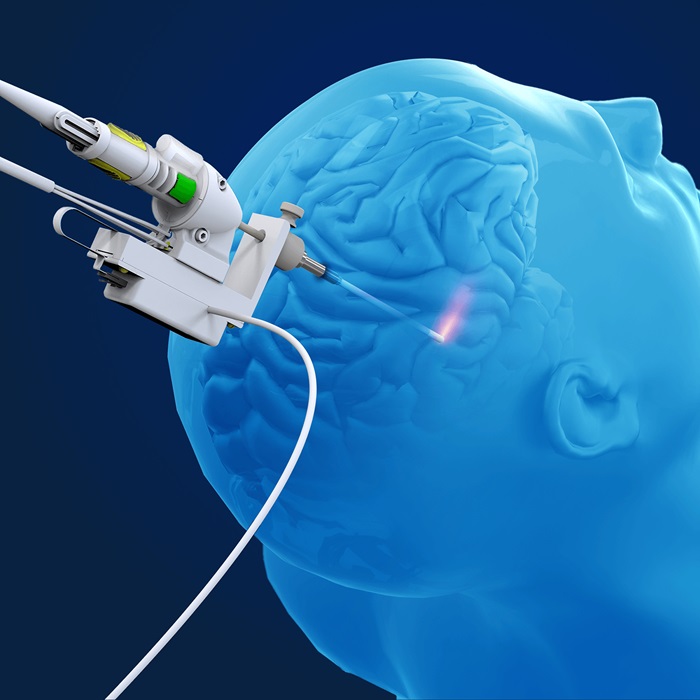
World’s Smallest Laser Probe for Brain Procedures Facilitates Ablation of Full Range of Targets
A new probe enhances the ablation capabilities for a broad spectrum of oncology and epilepsy targets, including pediatric applications, by incorporating advanced laser and cooling technologies to support... Read more.jpg)
Artificial Intelligence Broadens Diagnostic Abilities of Conventional Coronary Angiography
Coronary angiography is an essential diagnostic tool used globally to identify coronary artery disease (CAD), with millions of procedures conducted annually. Traditionally, data from coronary angiograms... Read more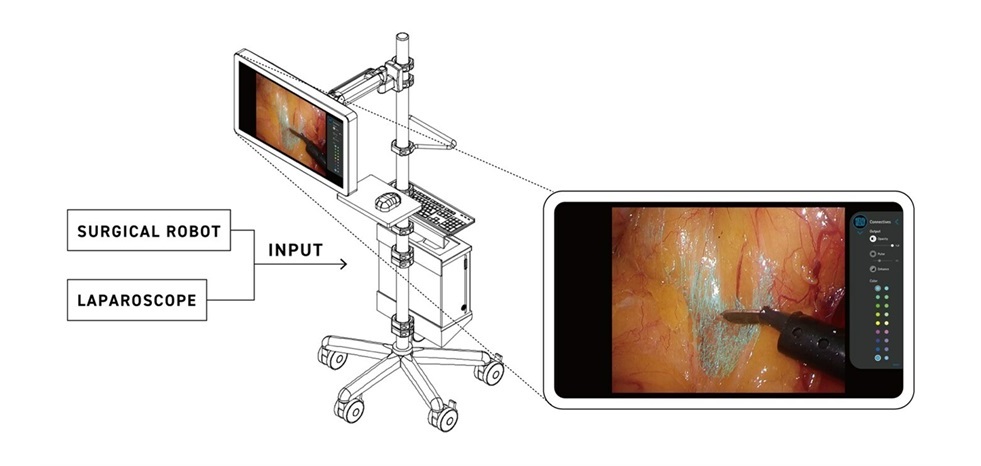
AI-Powered Surgical Visualization Tool Supports Surgeons' Visual Recognition in Real Time
Connective tissue serves as an essential landmark in surgical navigation, often referred to as the "dissection plane" or "holy plane." Its accurate identification is vital for achieving safe and effective... Read more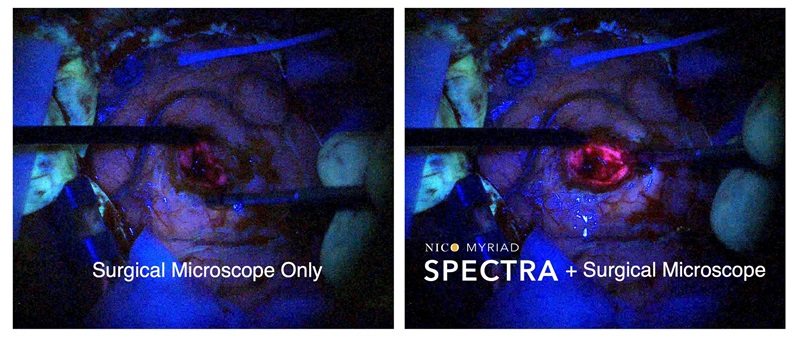
Handheld Device for Fluorescence-Guided Surgery a Game Changer for Removal of High-Grade Glioma Brain Tumors
Grade III or IV gliomas are among the most common and deadly brain tumors, with around 20,000 cases annually in the U.S. and 1.2 million globally. These tumors are very aggressive and tend to infiltrate... Read morePatient Care
view channel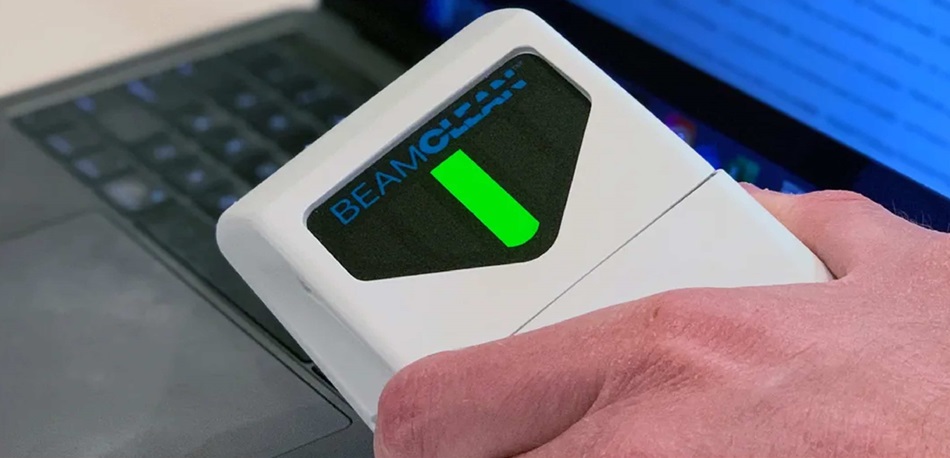
First-Of-Its-Kind Portable Germicidal Light Technology Disinfects High-Touch Clinical Surfaces in Seconds
Reducing healthcare-acquired infections (HAIs) remains a pressing issue within global healthcare systems. In the United States alone, 1.7 million patients contract HAIs annually, leading to approximately... Read more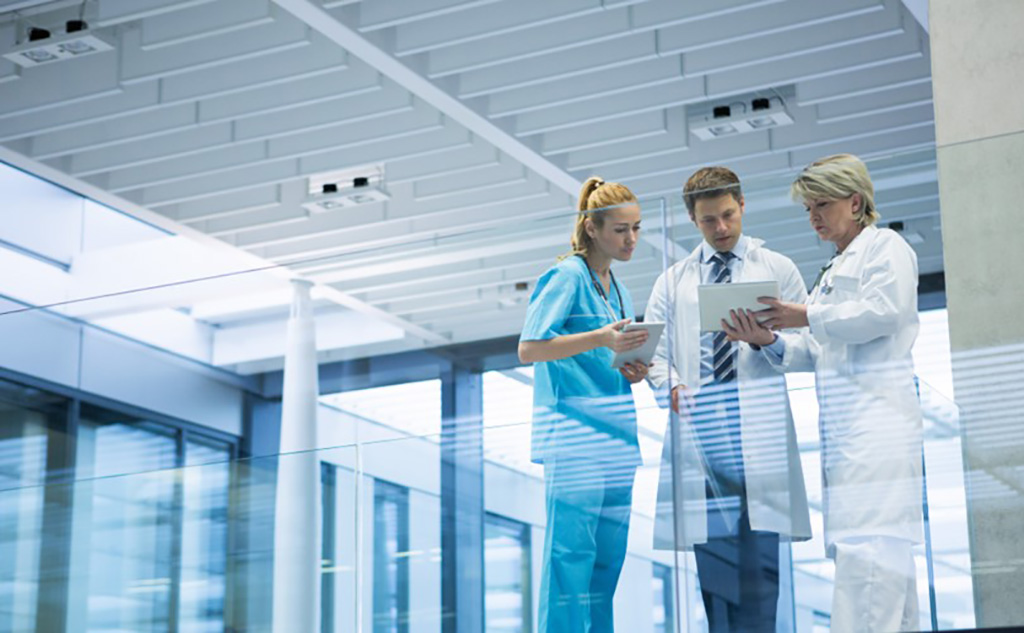
Surgical Capacity Optimization Solution Helps Hospitals Boost OR Utilization
An innovative solution has the capability to transform surgical capacity utilization by targeting the root cause of surgical block time inefficiencies. Fujitsu Limited’s (Tokyo, Japan) Surgical Capacity... Read more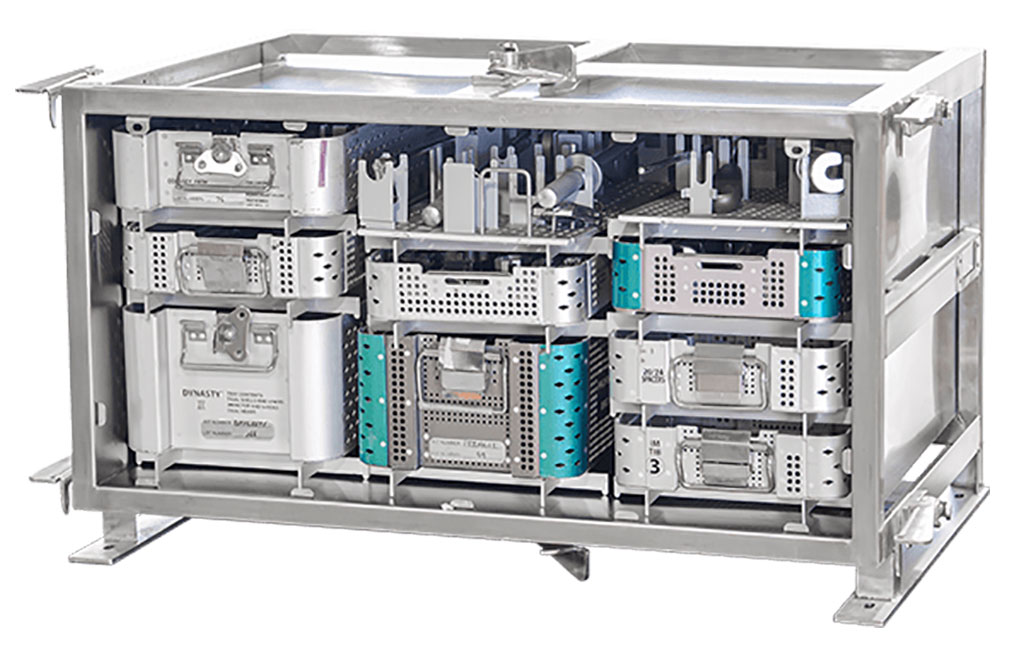
Game-Changing Innovation in Surgical Instrument Sterilization Significantly Improves OR Throughput
A groundbreaking innovation enables hospitals to significantly improve instrument processing time and throughput in operating rooms (ORs) and sterile processing departments. Turbett Surgical, Inc.... Read moreHealth IT
view channel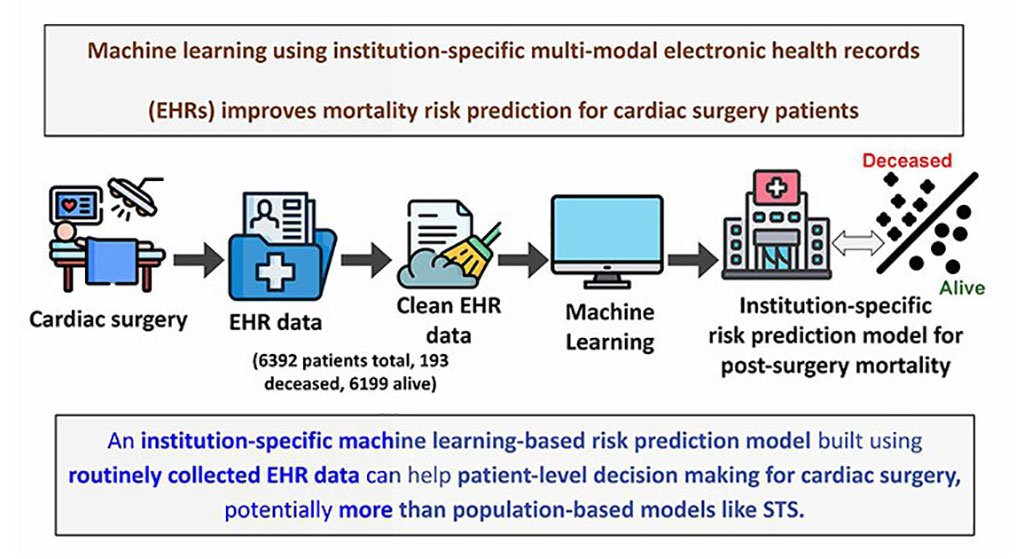
Machine Learning Model Improves Mortality Risk Prediction for Cardiac Surgery Patients
Machine learning algorithms have been deployed to create predictive models in various medical fields, with some demonstrating improved outcomes compared to their standard-of-care counterparts.... Read more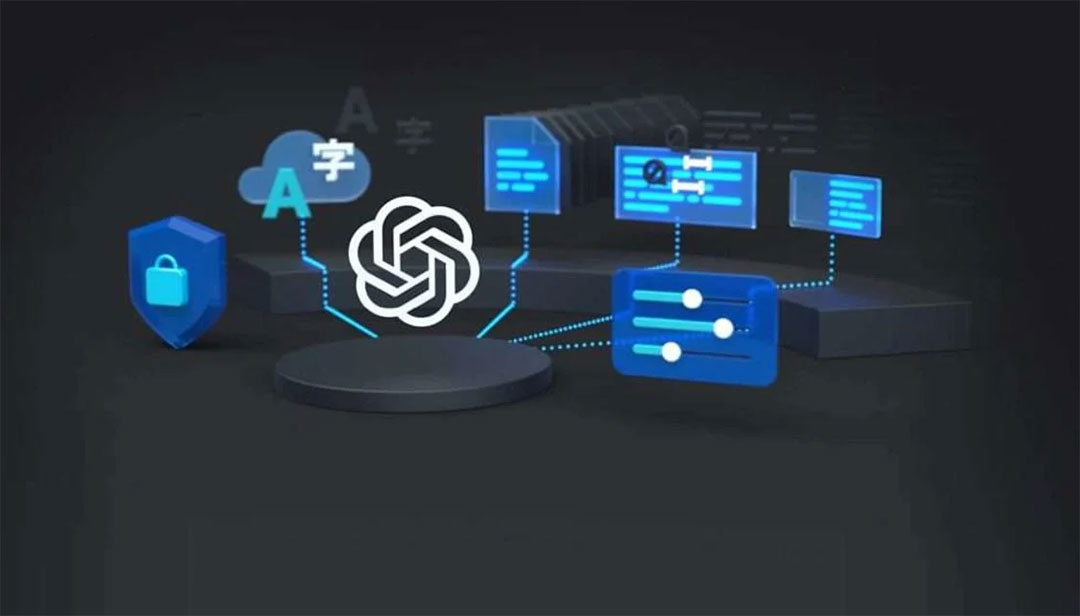
Strategic Collaboration to Develop and Integrate Generative AI into Healthcare
Top industry experts have underscored the immediate requirement for healthcare systems and hospitals to respond to severe cost and margin pressures. Close to half of U.S. hospitals ended 2022 in the red... Read more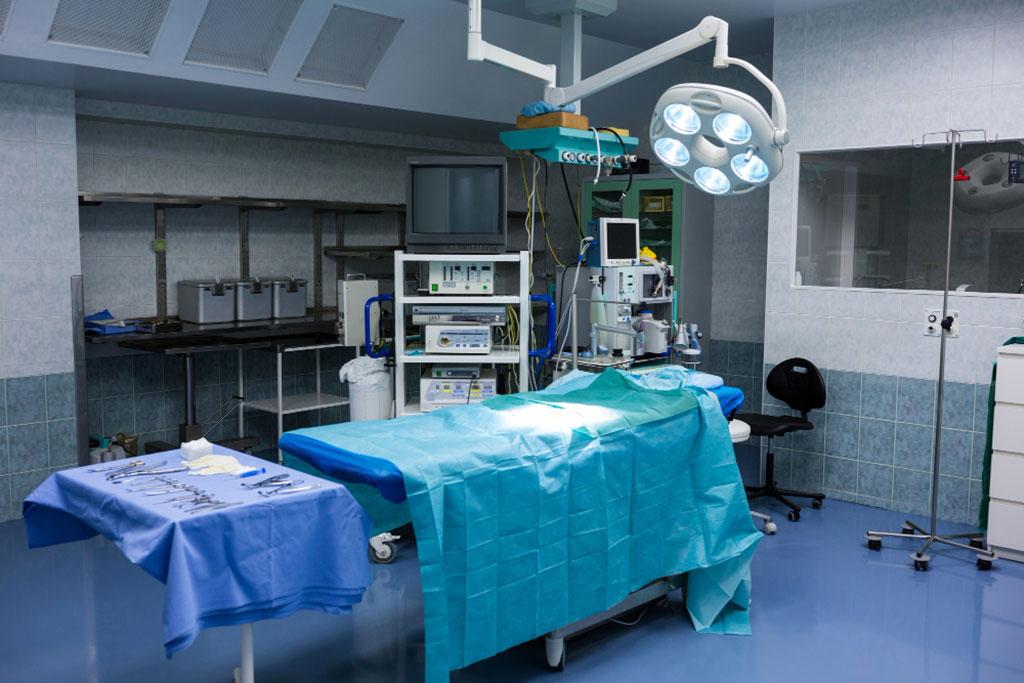
AI-Enabled Operating Rooms Solution Helps Hospitals Maximize Utilization and Unlock Capacity
For healthcare organizations, optimizing operating room (OR) utilization during prime time hours is a complex challenge. Surgeons and clinics face difficulties in finding available slots for booking cases,... Read more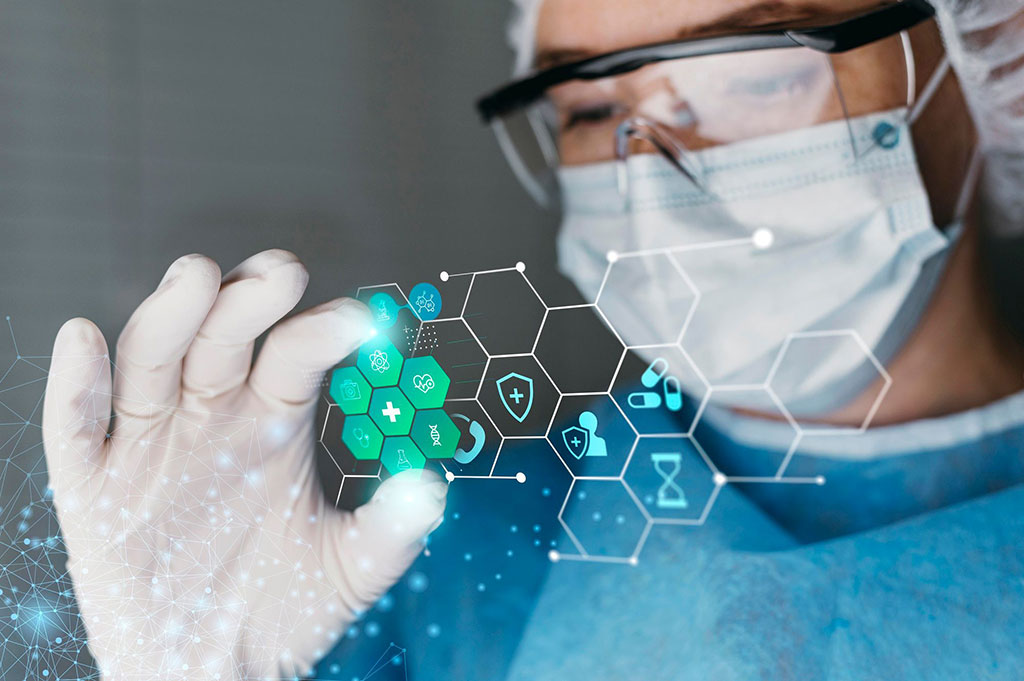
AI Predicts Pancreatic Cancer Three Years before Diagnosis from Patients’ Medical Records
Screening for common cancers like breast, cervix, and prostate cancer relies on relatively simple and highly effective techniques, such as mammograms, Pap smears, and blood tests. These methods have revolutionized... Read morePoint of Care
view channel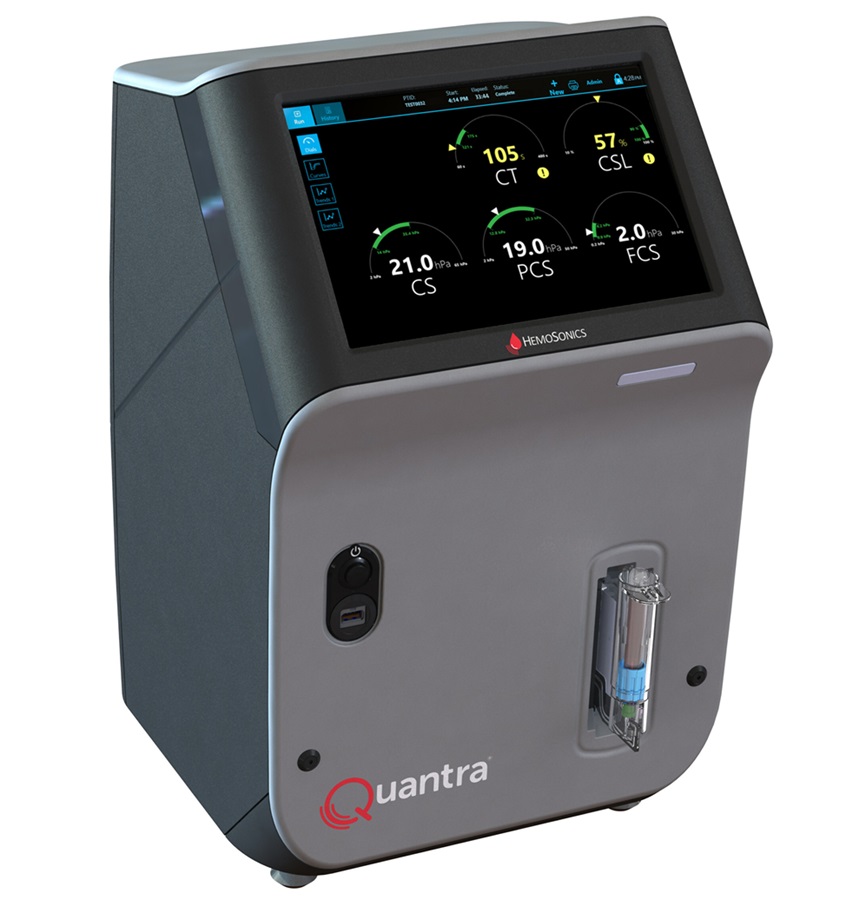
Critical Bleeding Management System to Help Hospitals Further Standardize Viscoelastic Testing
Surgical procedures are often accompanied by significant blood loss and the subsequent high likelihood of the need for allogeneic blood transfusions. These transfusions, while critical, are linked to various... Read more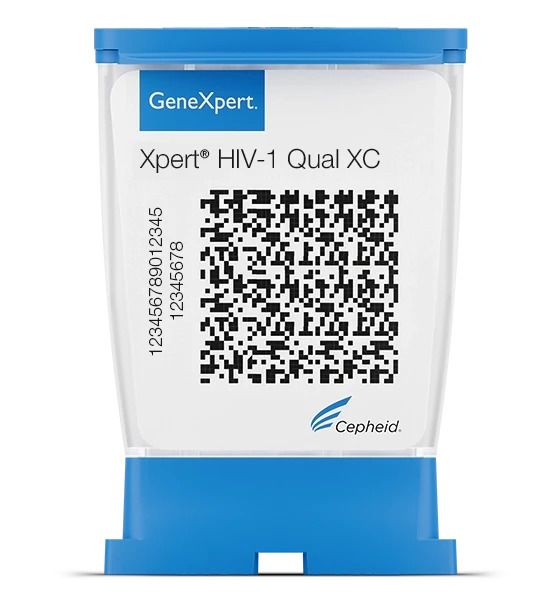
Point of Care HIV Test Enables Early Infection Diagnosis for Infants
Early diagnosis and initiation of treatment are crucial for the survival of infants infected with HIV (human immunodeficiency virus). Without treatment, approximately 50% of infants who acquire HIV during... Read more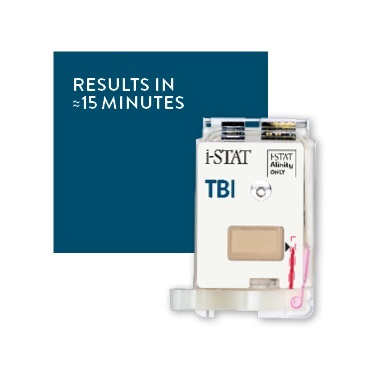
Whole Blood Rapid Test Aids Assessment of Concussion at Patient's Bedside
In the United States annually, approximately five million individuals seek emergency department care for traumatic brain injuries (TBIs), yet over half of those suspecting a concussion may never get it checked.... Read more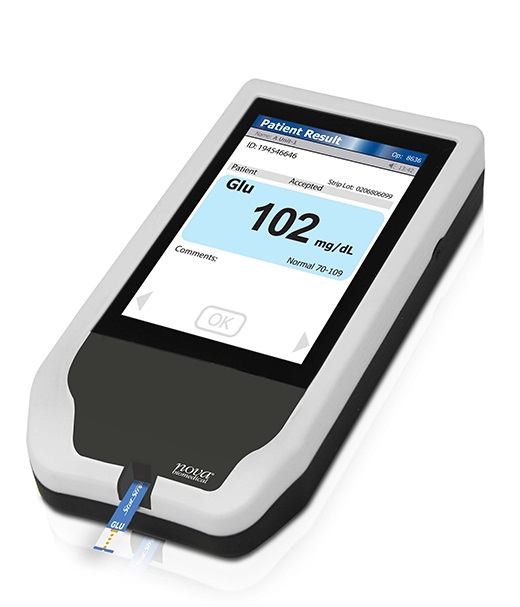
New Generation Glucose Hospital Meter System Ensures Accurate, Interference-Free and Safe Use
A new generation glucose hospital meter system now comes with several features that make hospital glucose testing easier and more secure while continuing to offer accuracy, freedom from interference, and... Read moreBusiness
view channel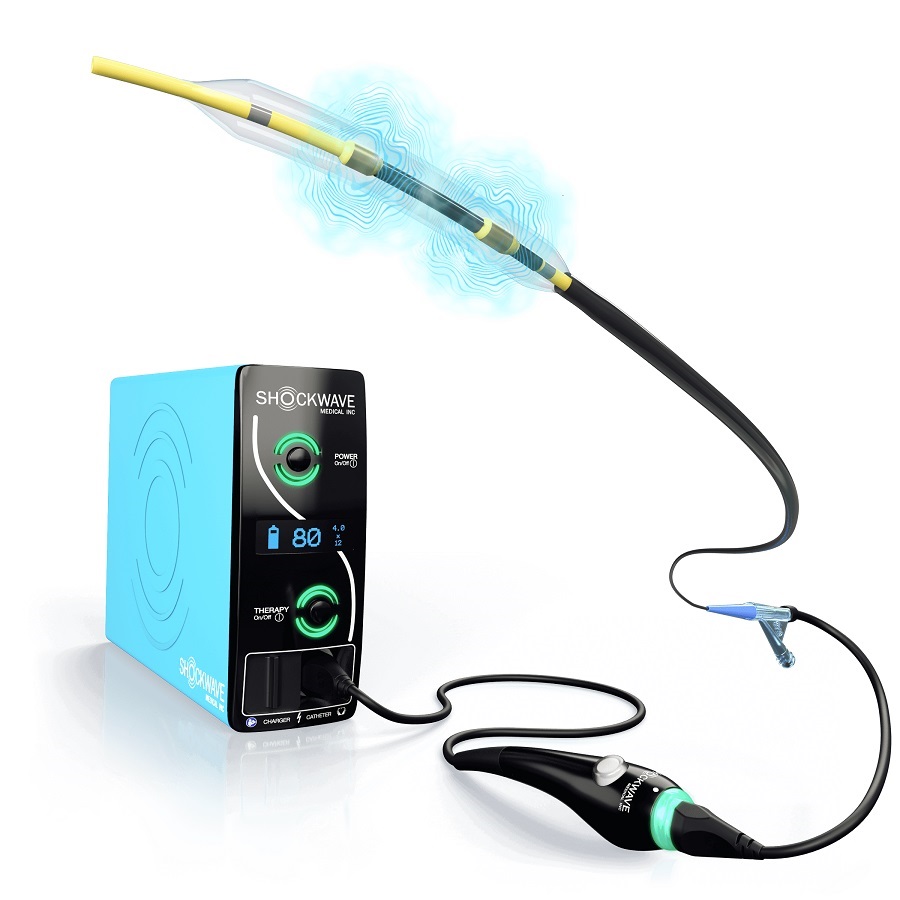
Johnson & Johnson Acquires Cardiovascular Medical Device Company Shockwave Medical
Johnson & Johnson (New Brunswick, N.J., USA) and Shockwave Medical (Santa Clara, CA, USA) have entered into a definitive agreement under which Johnson & Johnson will acquire all of Shockwave’s... Read more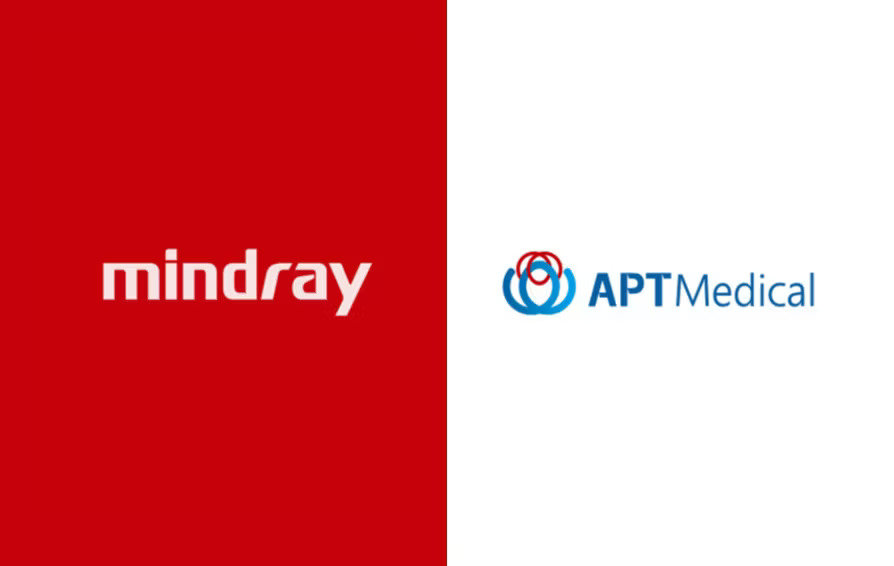