Machine Learning Model Uses Blood Tests to Predict Survival of Critically Ill COVID-19 Patients
By HospiMedica International staff writers Posted on 20 Jan 2022 |
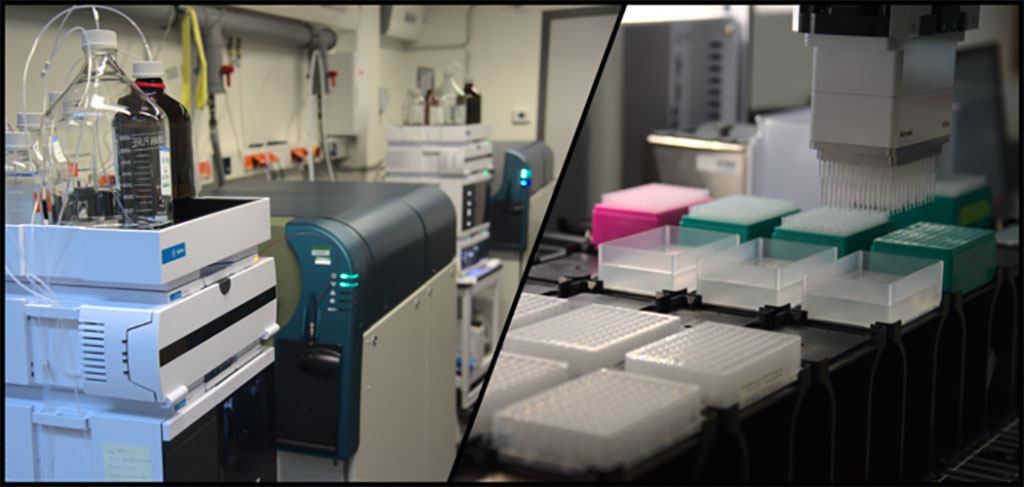
A single blood sample from a critically ill COVID-19 patient can be analyzed by a machine learning model which uses blood plasma proteins to predict survival, weeks before the outcome, according to a new study.
Scientists at the Charité-Universitätsmedizin Berlin (Berlin, Germany) have found that the levels of 14 proteins in the blood of critically ill COVID-19 patients are associated with survival. Healthcare systems around the world are struggling to accommodate high numbers of severely ill COVID-19 patients who need special medical attention, especially if they are identified as being at high risk. Clinically established risk assessments in intensive care medicine, such as the SOFA or APACHE II, show only limited reliability in predicting future disease outcomes for COVID-19.
In the new study, researchers studied the levels of 321 proteins in blood samples taken at 349 timepoints from 50 critically ill COVID-19 patients being treated in two independent health care centers in Germany and Austria. A machine learning approach was used to find associations between the measured proteins and patient survival. 15 of the patients in the cohort died; the average time from admission to death was 28 days. For patients who survived, the median time of hospitalization was 63 days.
The researchers pinpointed 14 proteins which, over time, changed in opposite directions for patients who survive compared to patients who do not survive on intensive care. The team then developed a machine learning model to predict survival based on a single time-point measurement of relevant proteins and tested the model on an independent validation cohort of 24 critically ill COVID-10 patients. The model demonstrated high predictive power on this cohort, correctly predicting the outcome for 18 of 19 patients who survived and five out of five patients who died.
The researchers concluded that blood protein tests, if validated in larger cohorts, may be useful in both identifying patients with the highest mortality risk, as well as for testing whether a given treatment changes the projected trajectory of an individual patient.
Related Links:
Charité-Universitätsmedizin Berlin
Latest COVID-19 News
- Low-Cost System Detects SARS-CoV-2 Virus in Hospital Air Using High-Tech Bubbles
- World's First Inhalable COVID-19 Vaccine Approved in China
- COVID-19 Vaccine Patch Fights SARS-CoV-2 Variants Better than Needles
- Blood Viscosity Testing Can Predict Risk of Death in Hospitalized COVID-19 Patients
- ‘Covid Computer’ Uses AI to Detect COVID-19 from Chest CT Scans
- MRI Lung-Imaging Technique Shows Cause of Long-COVID Symptoms
- Chest CT Scans of COVID-19 Patients Could Help Distinguish Between SARS-CoV-2 Variants
- Specialized MRI Detects Lung Abnormalities in Non-Hospitalized Long COVID Patients
- AI Algorithm Identifies Hospitalized Patients at Highest Risk of Dying From COVID-19
- Sweat Sensor Detects Key Biomarkers That Provide Early Warning of COVID-19 and Flu
- Study Assesses Impact of COVID-19 on Ventilation/Perfusion Scintigraphy
- CT Imaging Study Finds Vaccination Reduces Risk of COVID-19 Associated Pulmonary Embolism
- Third Day in Hospital a ‘Tipping Point’ in Severity of COVID-19 Pneumonia
- Longer Interval Between COVID-19 Vaccines Generates Up to Nine Times as Many Antibodies
- AI Model for Monitoring COVID-19 Predicts Mortality Within First 30 Days of Admission
- AI Predicts COVID Prognosis at Near-Expert Level Based Off CT Scans
Channels
Artificial Intelligence
view channel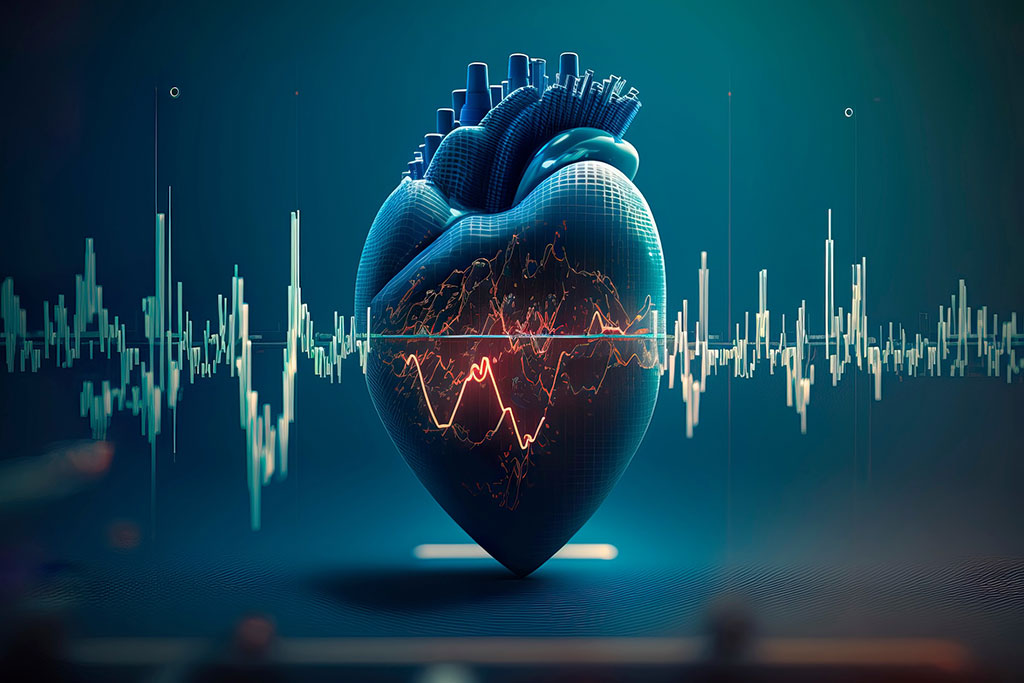
AI-Powered Algorithm to Revolutionize Detection of Atrial Fibrillation
Atrial fibrillation (AFib), a condition characterized by an irregular and often rapid heart rate, is linked to increased risks of stroke and heart failure. This is because the irregular heartbeat in AFib... Read more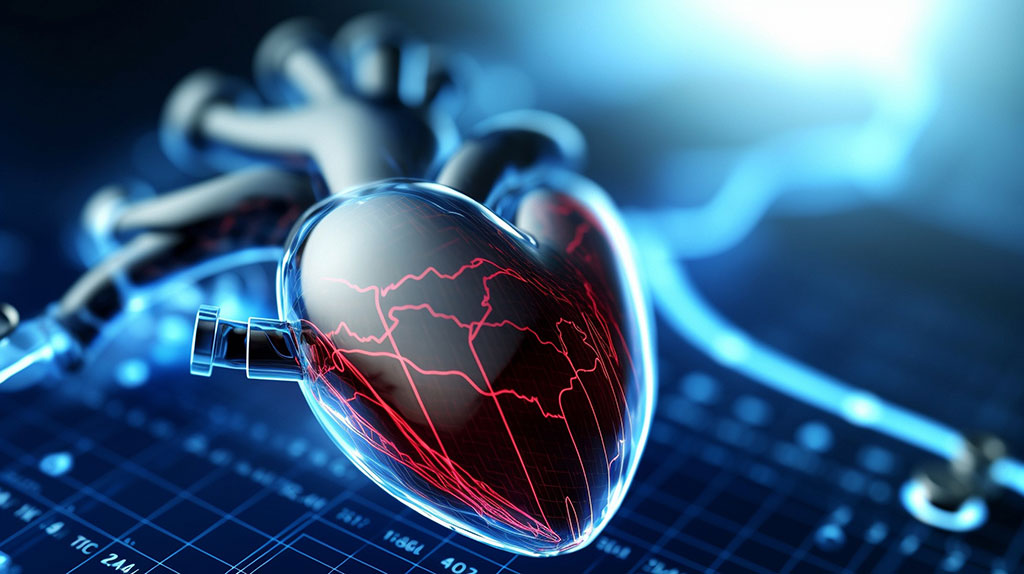
AI Diagnostic Tool Accurately Detects Valvular Disorders Often Missed by Doctors
Doctors generally use stethoscopes to listen for the characteristic lub-dub sounds made by heart valves opening and closing. They also listen for less prominent sounds that indicate problems with these valves.... Read moreCritical Care
view channel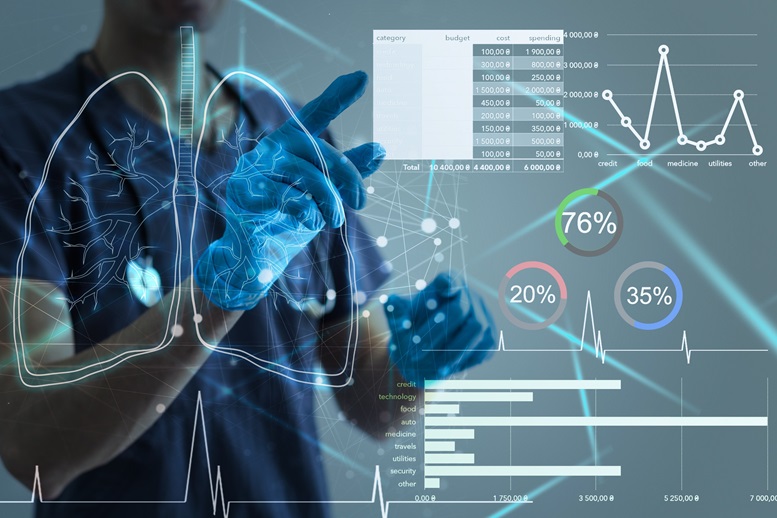
Machine Learning Tool Identifies Rare, Undiagnosed Immune Disorders from Patient EHRs
Patients suffering from rare diseases often endure extensive delays in receiving accurate diagnoses and treatments, which can lead to unnecessary tests, worsening health, psychological strain, and significant... Read more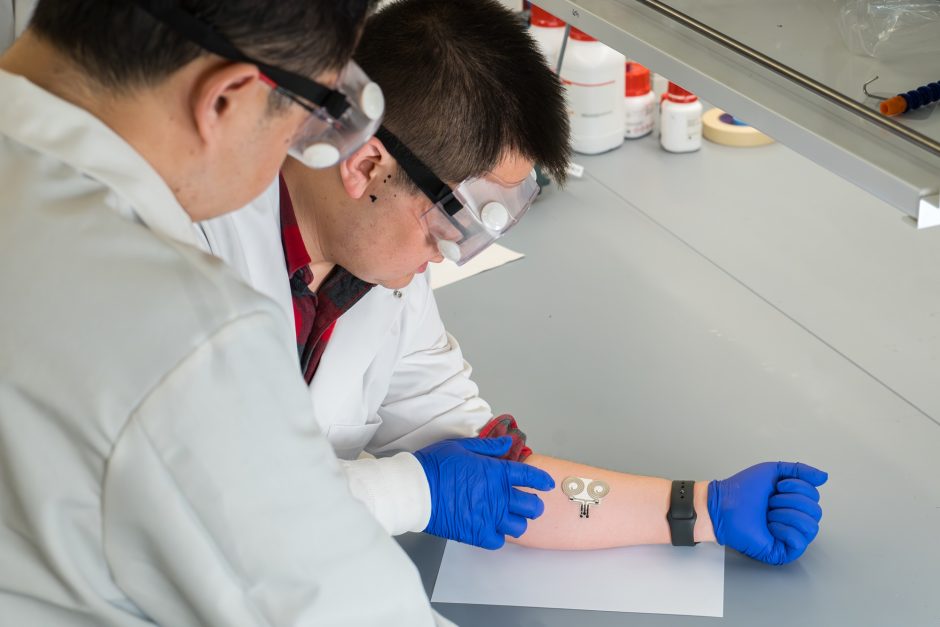
On-Skin Wearable Bioelectronic Device Paves Way for Intelligent Implants
A team of researchers at the University of Missouri (Columbia, MO, USA) has achieved a milestone in developing a state-of-the-art on-skin wearable bioelectronic device. This development comes from a lab... Read more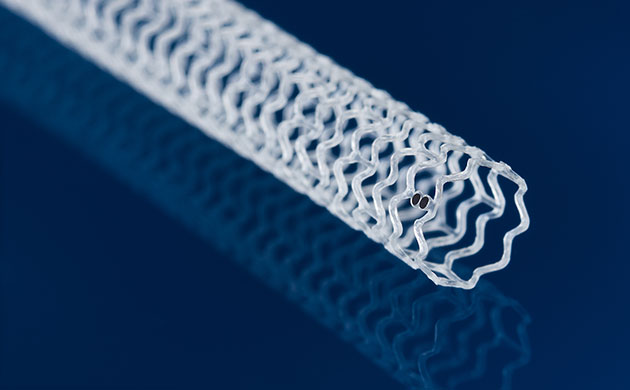
First-Of-Its-Kind Dissolvable Stent to Improve Outcomes for Patients with Severe PAD
Peripheral artery disease (PAD) affects millions and presents serious health risks, particularly its severe form, chronic limb-threatening ischemia (CLTI). CLTI develops when arteries are blocked by plaque,... Read more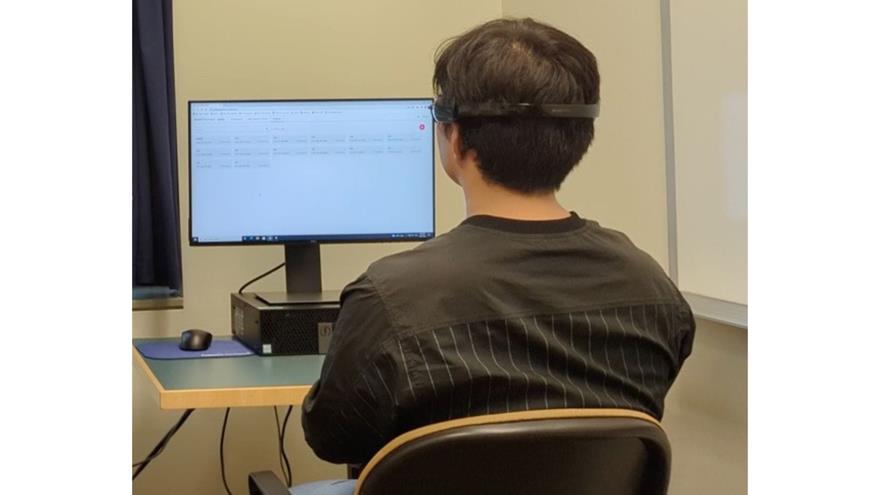
AI Brain-Age Estimation Technology Uses EEG Scans to Screen for Degenerative Diseases
As individuals age, so do their brains. Premature aging of the brain can lead to age-related conditions such as mild cognitive impairment, dementia, or Parkinson's disease. The ability to determine "brain... Read moreSurgical Techniques
view channel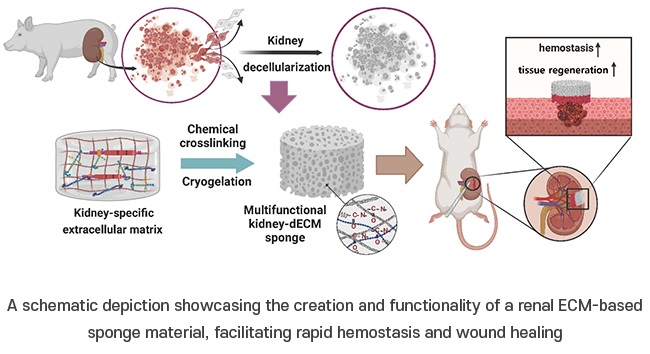
Porous Gel Sponge Facilitates Rapid Hemostasis and Wound Healing
The kidneys are essential organs that handle critical bodily functions, including waste elimination and blood pressure regulation. Often referred to as the silent organ because they typically do not manifest... Read more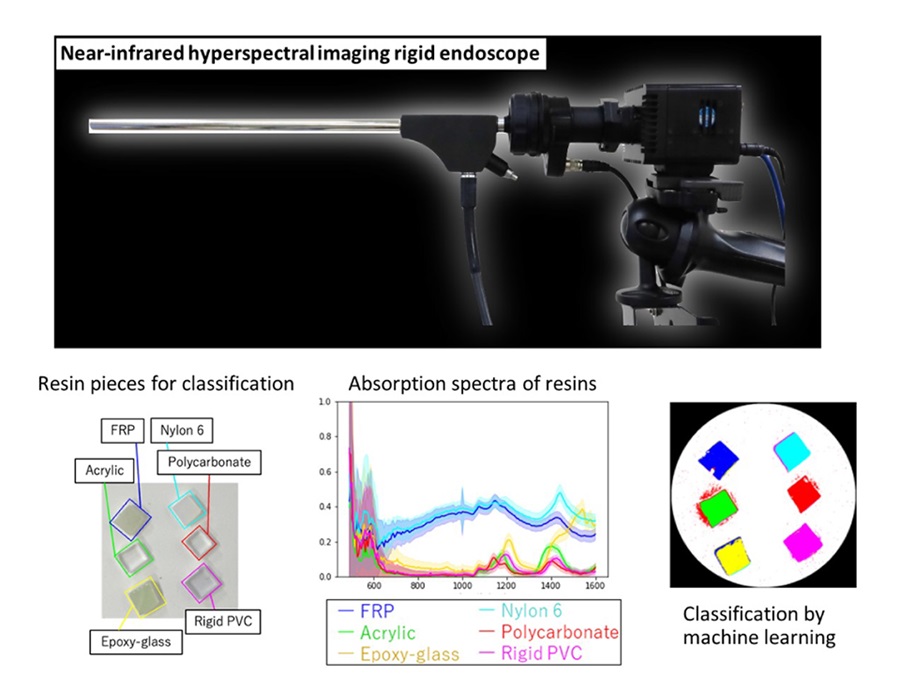
Novel Rigid Endoscope System Enables Deep Tissue Imaging During Surgery
Hyperspectral imaging (HSI) is an advanced technique that captures and processes information across a given electromagnetic spectrum. Near-infrared hyperspectral imaging (NIR-HSI) has particularly gained... Read more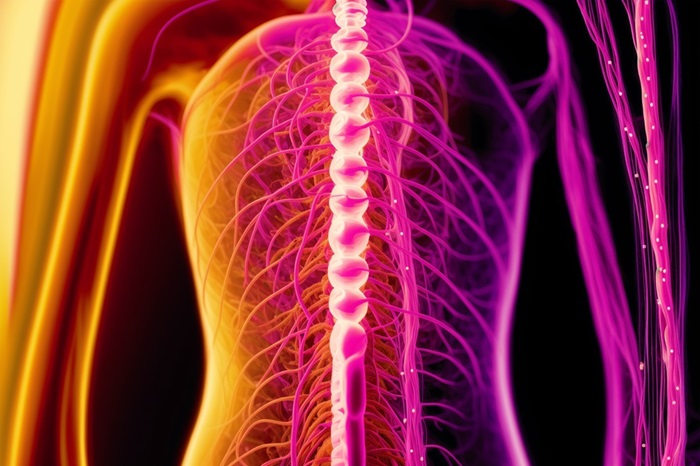
Robotic Nerve ‘Cuffs’ Could Treat Various Neurological Conditions
Electric nerve implants serve dual functions: they can either stimulate or block signals in specific nerves. For example, they may alleviate pain by inhibiting pain signals or restore movement in paralyzed... Read more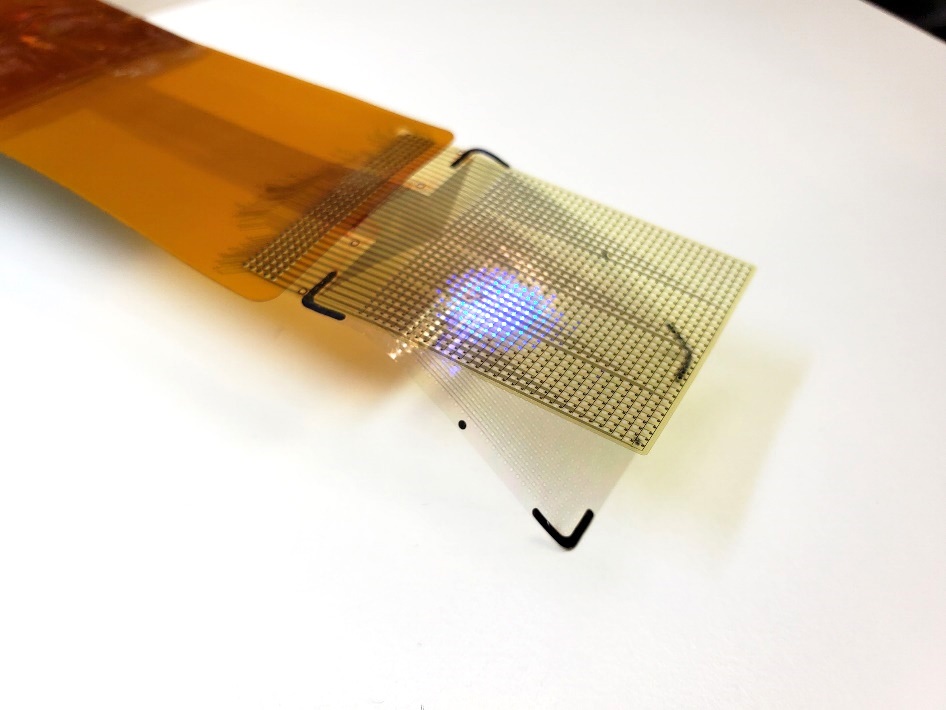
Flexible Microdisplay Visualizes Brain Activity in Real-Time To Guide Neurosurgeons
During brain surgery, neurosurgeons need to identify and preserve regions responsible for critical functions while removing harmful tissue. Traditionally, neurosurgeons rely on a team of electrophysiologists,... Read morePatient Care
view channel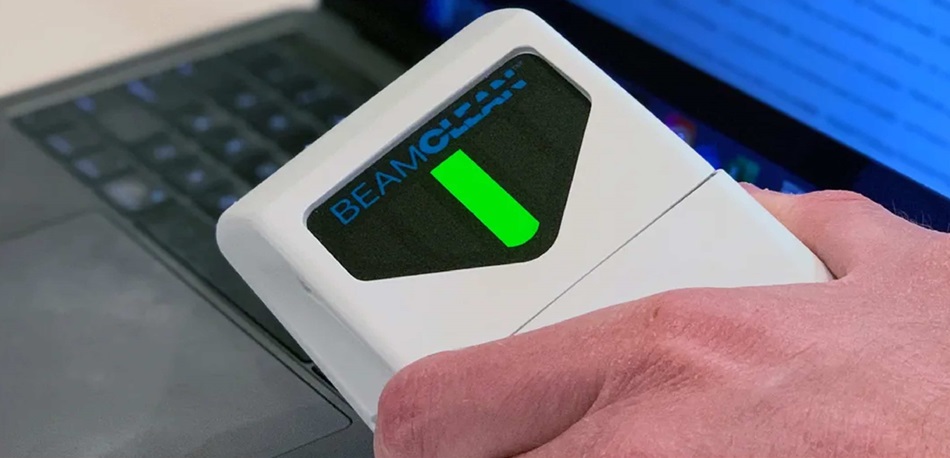
First-Of-Its-Kind Portable Germicidal Light Technology Disinfects High-Touch Clinical Surfaces in Seconds
Reducing healthcare-acquired infections (HAIs) remains a pressing issue within global healthcare systems. In the United States alone, 1.7 million patients contract HAIs annually, leading to approximately... Read more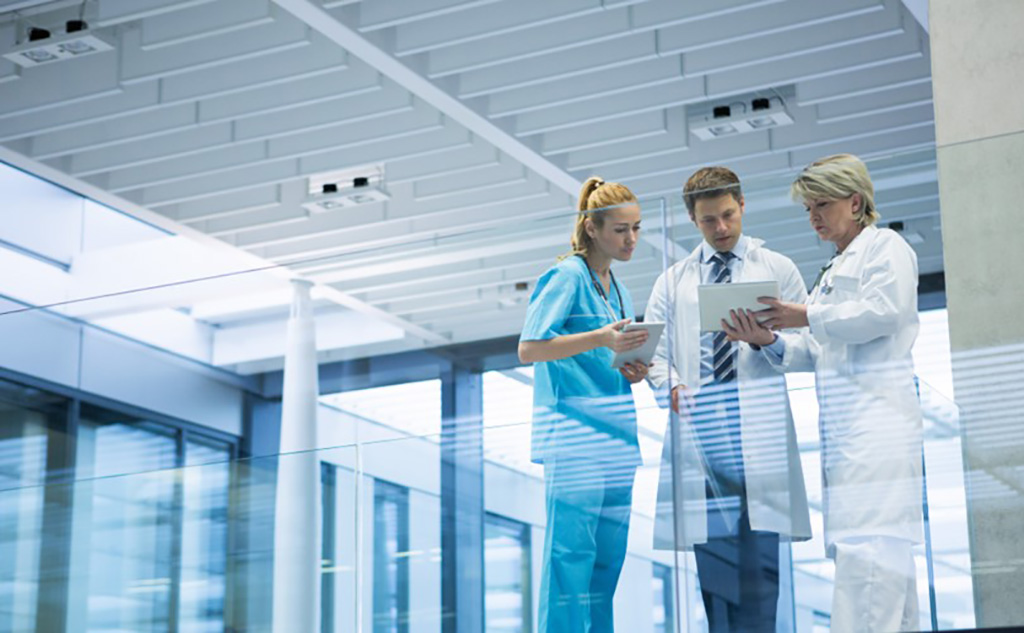
Surgical Capacity Optimization Solution Helps Hospitals Boost OR Utilization
An innovative solution has the capability to transform surgical capacity utilization by targeting the root cause of surgical block time inefficiencies. Fujitsu Limited’s (Tokyo, Japan) Surgical Capacity... Read more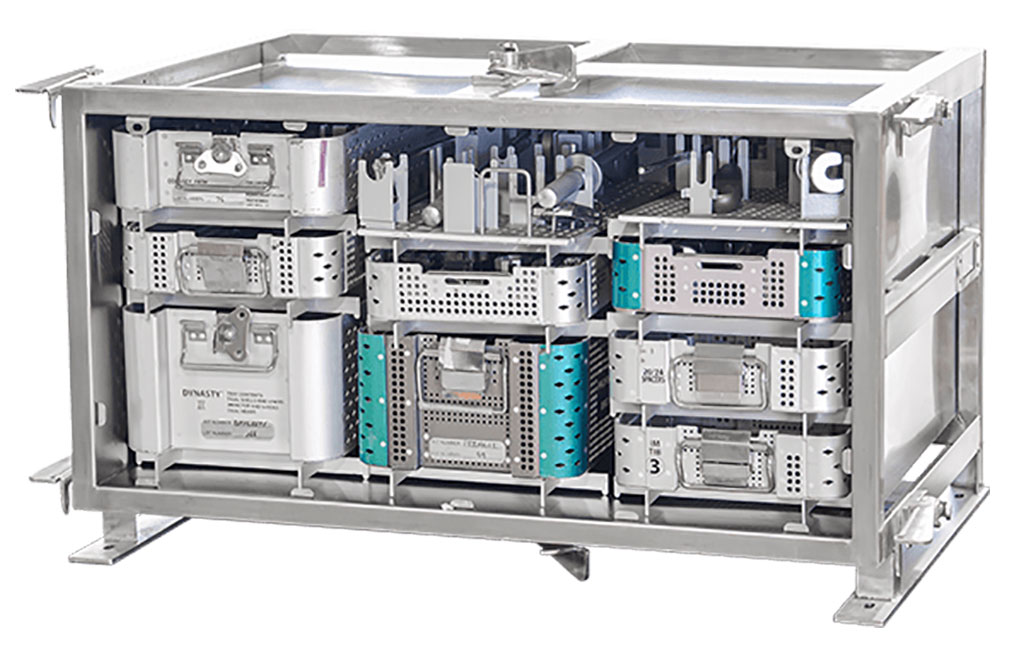
Game-Changing Innovation in Surgical Instrument Sterilization Significantly Improves OR Throughput
A groundbreaking innovation enables hospitals to significantly improve instrument processing time and throughput in operating rooms (ORs) and sterile processing departments. Turbett Surgical, Inc.... Read moreHealth IT
view channel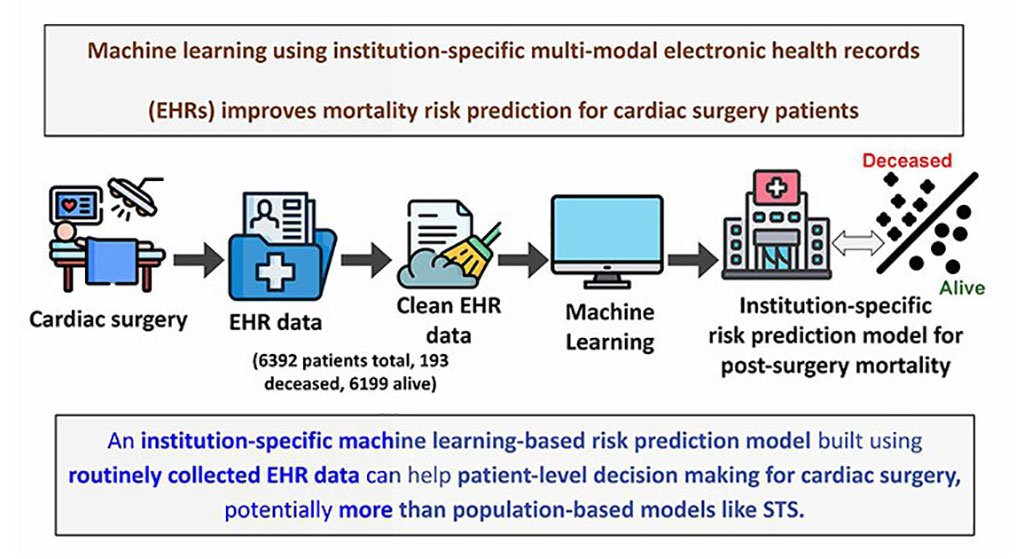
Machine Learning Model Improves Mortality Risk Prediction for Cardiac Surgery Patients
Machine learning algorithms have been deployed to create predictive models in various medical fields, with some demonstrating improved outcomes compared to their standard-of-care counterparts.... Read more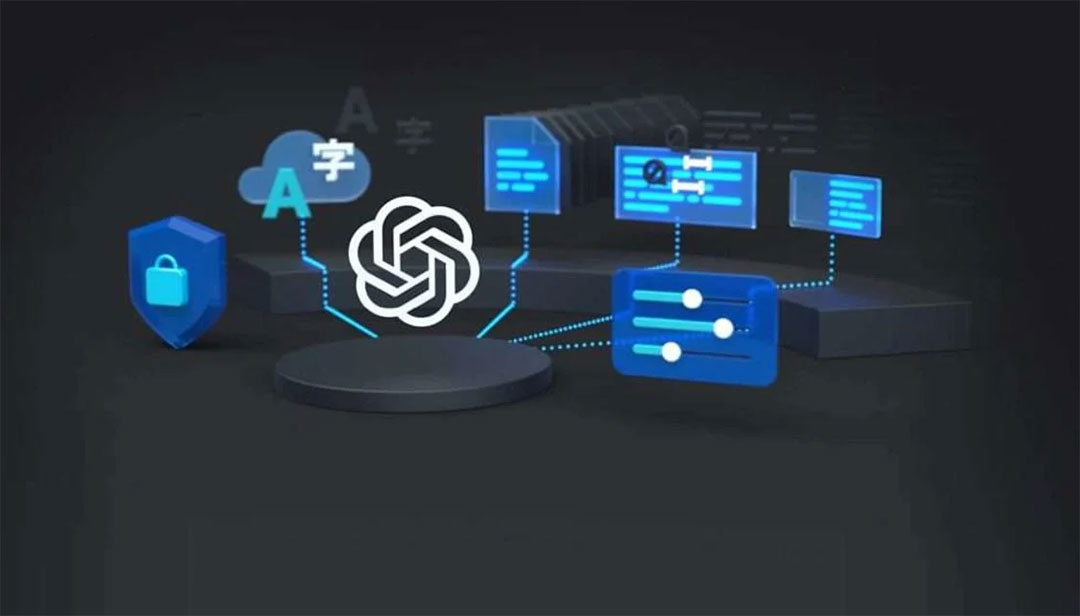
Strategic Collaboration to Develop and Integrate Generative AI into Healthcare
Top industry experts have underscored the immediate requirement for healthcare systems and hospitals to respond to severe cost and margin pressures. Close to half of U.S. hospitals ended 2022 in the red... Read more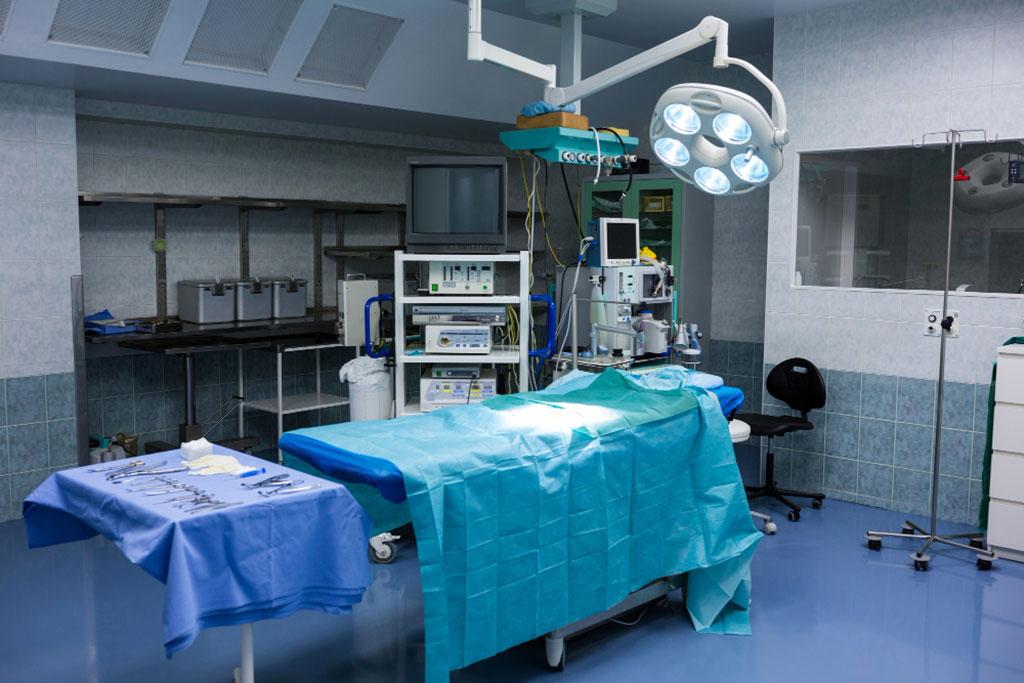
AI-Enabled Operating Rooms Solution Helps Hospitals Maximize Utilization and Unlock Capacity
For healthcare organizations, optimizing operating room (OR) utilization during prime time hours is a complex challenge. Surgeons and clinics face difficulties in finding available slots for booking cases,... Read more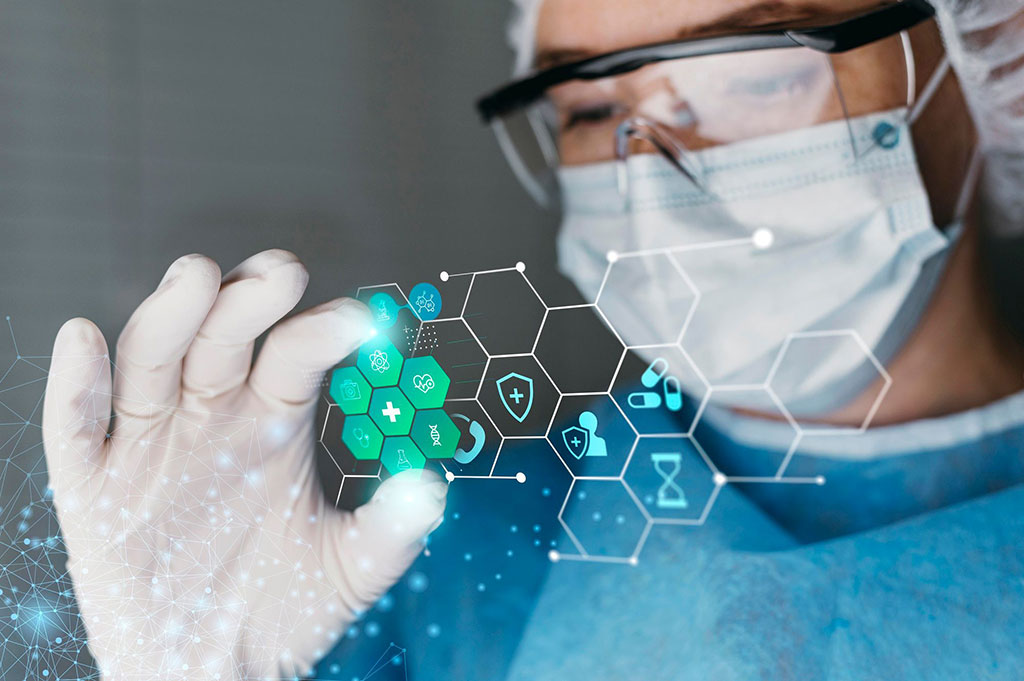
AI Predicts Pancreatic Cancer Three Years before Diagnosis from Patients’ Medical Records
Screening for common cancers like breast, cervix, and prostate cancer relies on relatively simple and highly effective techniques, such as mammograms, Pap smears, and blood tests. These methods have revolutionized... Read morePoint of Care
view channel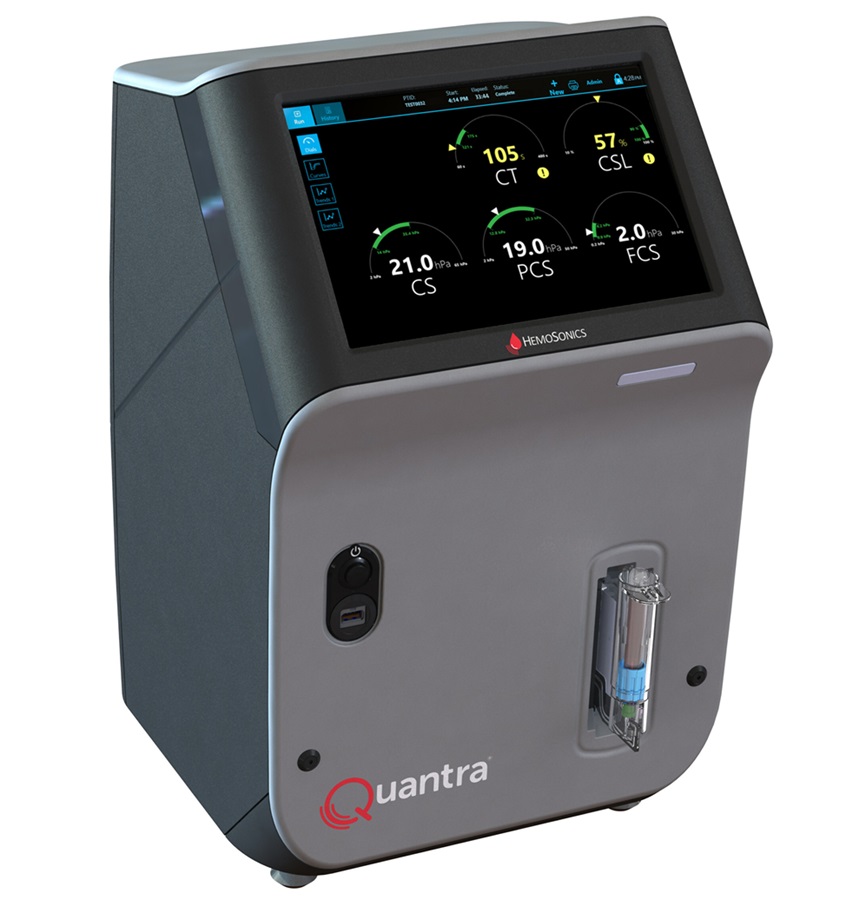
Critical Bleeding Management System to Help Hospitals Further Standardize Viscoelastic Testing
Surgical procedures are often accompanied by significant blood loss and the subsequent high likelihood of the need for allogeneic blood transfusions. These transfusions, while critical, are linked to various... Read more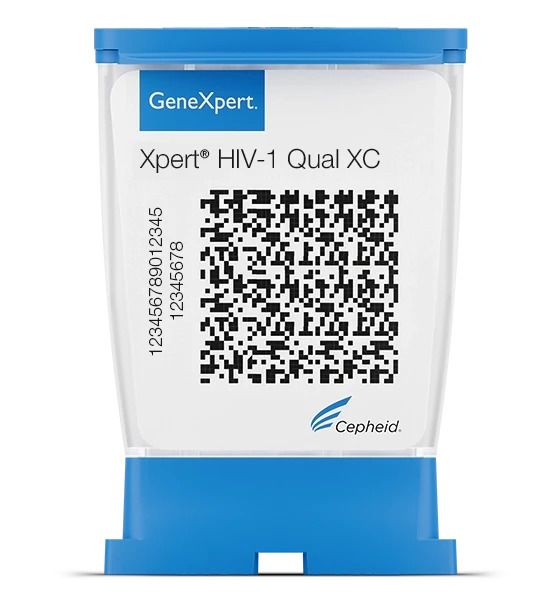
Point of Care HIV Test Enables Early Infection Diagnosis for Infants
Early diagnosis and initiation of treatment are crucial for the survival of infants infected with HIV (human immunodeficiency virus). Without treatment, approximately 50% of infants who acquire HIV during... Read more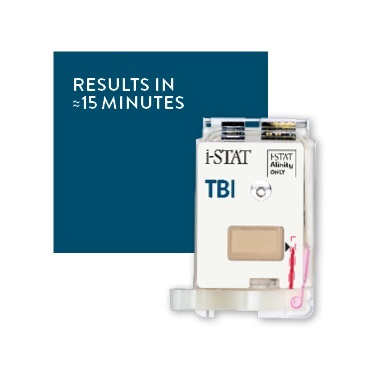
Whole Blood Rapid Test Aids Assessment of Concussion at Patient's Bedside
In the United States annually, approximately five million individuals seek emergency department care for traumatic brain injuries (TBIs), yet over half of those suspecting a concussion may never get it checked.... Read more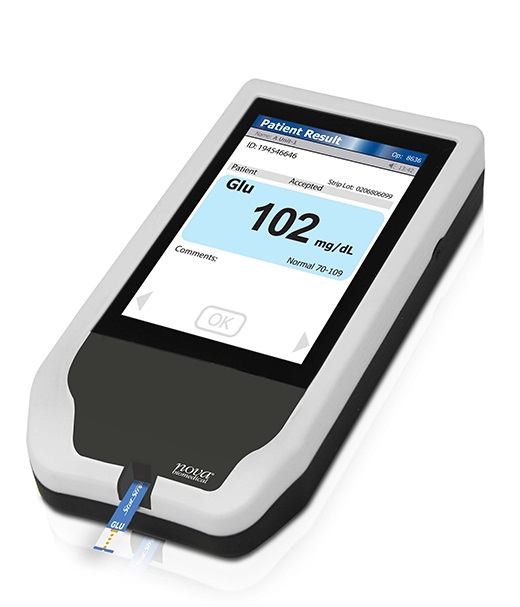
New Generation Glucose Hospital Meter System Ensures Accurate, Interference-Free and Safe Use
A new generation glucose hospital meter system now comes with several features that make hospital glucose testing easier and more secure while continuing to offer accuracy, freedom from interference, and... Read moreBusiness
view channel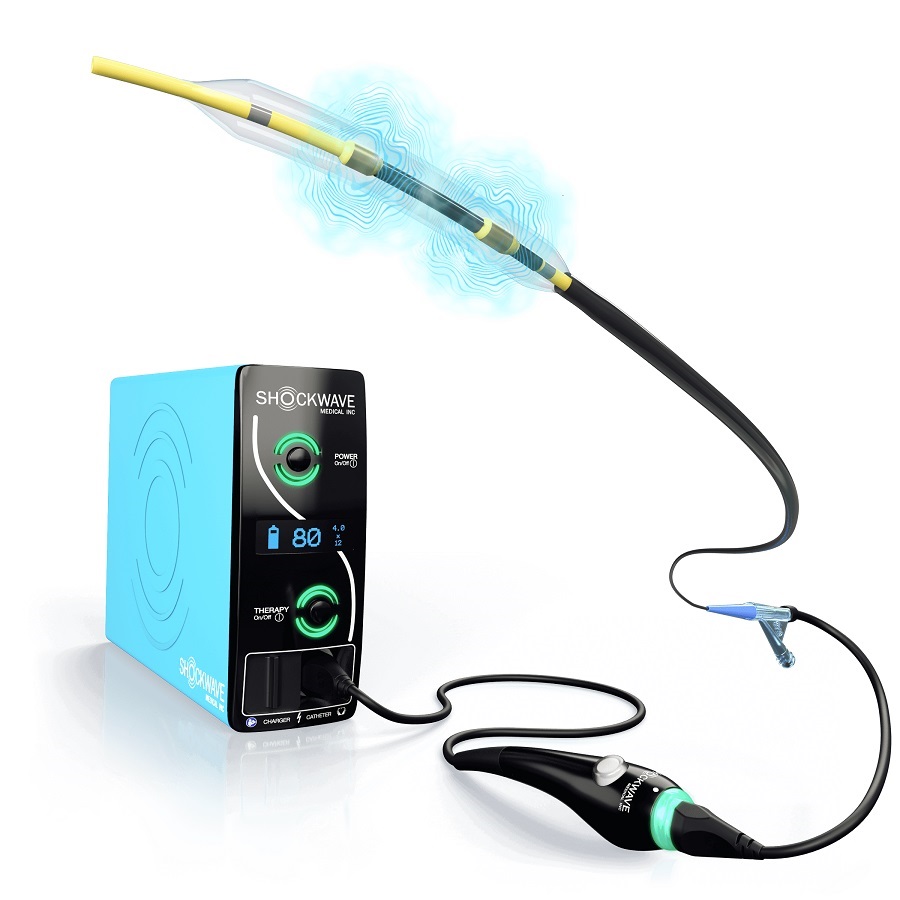
Johnson & Johnson Acquires Cardiovascular Medical Device Company Shockwave Medical
Johnson & Johnson (New Brunswick, N.J., USA) and Shockwave Medical (Santa Clara, CA, USA) have entered into a definitive agreement under which Johnson & Johnson will acquire all of Shockwave’s... Read more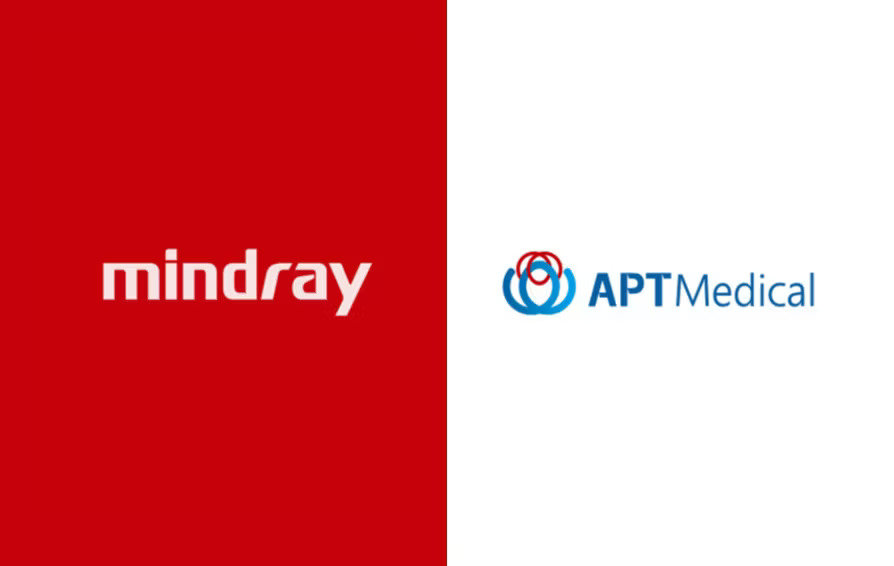